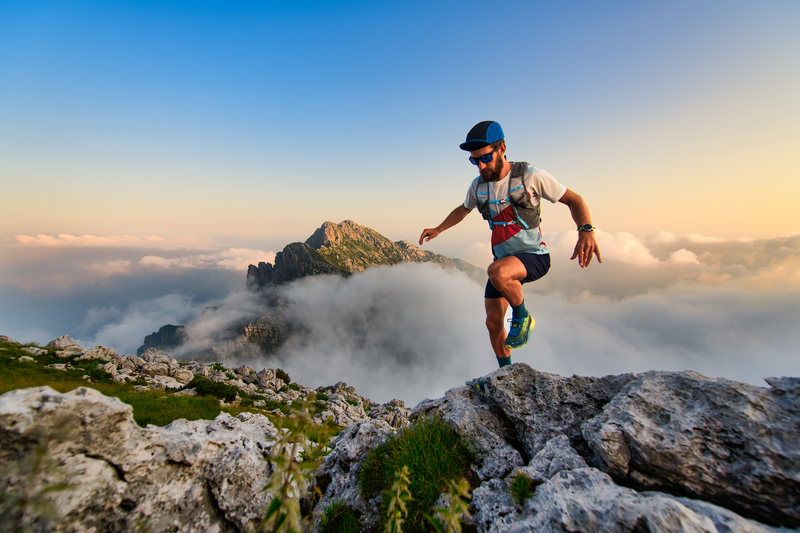
95% of researchers rate our articles as excellent or good
Learn more about the work of our research integrity team to safeguard the quality of each article we publish.
Find out more
ORIGINAL RESEARCH article
Front. Robot. AI
Sec. Field Robotics
Volume 12 - 2025 | doi: 10.3389/frobt.2025.1574161
This article is part of the Research Topic Autonomous Robotic Systems in Aquaculture: Research Challenges and Industry Needs View all articles
The final, formatted version of the article will be published soon.
You have multiple emails registered with Frontiers:
Please enter your email address:
If you already have an account, please login
You don't have a Frontiers account ? You can register here
Studies show that Atlantic salmon in captivity adjust their distribution in sea cages based on environmental gradients like temperature, waves, and photoperiod. This study used a computer vision algorithm at three marine farms to analyse fish group swimming behaviour termed "activity" (measured in percent), which includes fish abundance, speed, and shoal cohesion. The activity metric inferred the depth distribution of the main fish group and analysed its relationship with environmental conditions, as well as potential changes in response to a stressor, in this case, a storm event. During winter conditions, farms A and B showed distinct thermal stratification, with fish activity demonstrating preference for the warmer lower water column (39.6 ± 15.3% and 27.5 ± 10.2%) over the upper water column (16.3 ± 5.7% and 18 ± 3.3%; p < 0.001). At Farm C, with thermally homogenous water, fish activity was similarly distributed between the upper (18.2 ± 6.9%) and lower (17.7 ± 7.6%) water column. Severe weather increased wave heights, influencing fish horizontal distribution differently at Farms B and C. At Farm B, a deeper site, fish remained in the warmer lower water column and avoided surface waves, while at Farm C, with shallower cages, they moved toward the side of the cage nearest the centre of the farm, presumably less exposed due to nearby cages. Understanding fish behavioural responses to environmental conditions can inform management practices, while using cameras with associated algorithms offers a powerful, non-invasive tool for continuously monitoring and safeguarding fish health and welfare.
Keywords: Atlantic salmon, Aquaculture, temperature, photoperiod, storm event, fish distribution, machine learning
Received: 10 Feb 2025; Accepted: 04 Apr 2025.
Copyright: © 2025 Burke, Nikolic, Fabry, Rishi, Telfer and Rey Planellas. This is an open-access article distributed under the terms of the Creative Commons Attribution License (CC BY). The use, distribution or reproduction in other forums is permitted, provided the original author(s) or licensor are credited and that the original publication in this journal is cited, in accordance with accepted academic practice. No use, distribution or reproduction is permitted which does not comply with these terms.
* Correspondence:
Sonia Rey Planellas, University of Stirling, Stirling, United Kingdom
Disclaimer: All claims expressed in this article are solely those of the authors and do not necessarily represent those of their affiliated organizations, or those of the publisher, the editors and the reviewers. Any product that may be evaluated in this article or claim that may be made by its manufacturer is not guaranteed or endorsed by the publisher.
Research integrity at Frontiers
Learn more about the work of our research integrity team to safeguard the quality of each article we publish.