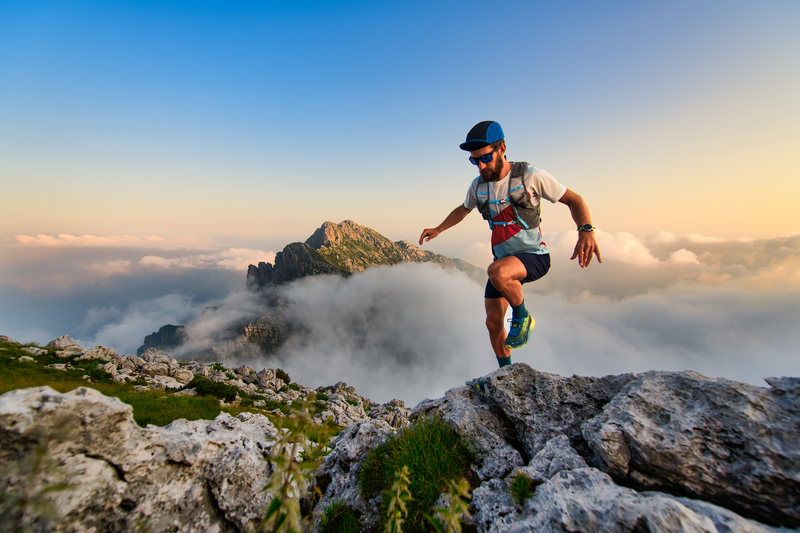
95% of researchers rate our articles as excellent or good
Learn more about the work of our research integrity team to safeguard the quality of each article we publish.
Find out more
ORIGINAL RESEARCH article
Front. Robot. AI
Sec. Human-Robot Interaction
Volume 12 - 2025 | doi: 10.3389/frobt.2025.1569476
The final, formatted version of the article will be published soon.
You have multiple emails registered with Frontiers:
Please enter your email address:
If you already have an account, please login
You don't have a Frontiers account ? You can register here
Computer-Aided Manufacturing (CAM) tools are a key component in many digital fabrication workflows, translating digital designs into machine instructions to manufacture physical objects.However, conventional CAM tools are tailored for standard manufacturing processes such as milling, turning or laser cutting, and can therefore be a limiting factor -especially for craftspeople and makers who want to employ non-standard, craft-like operations. Formalizing the tacit knowledge behind such operations to incorporate it in new CAM-routines is inherently difficult and often not feasible for the ad hoc incorporation of custom manufacturing operations in a digital fabrication workflow. In this paper, we address this gap by exploring the integration of Learning from Demonstration (LfD) into digital fabrication workflows, allowing makers to establish new manufacturing operations by providing manual demonstrations. To this end, we perform a case study on robot wood carving with hand tools, in which we integrate probabilistic movement primitives (ProMPs) into Rhino's Grasshopper environment to achieve basic CAM-like functionality.Human demonstrations of different wood carving cuts are recorded via kinesthetic teaching and modeled by a mixture of ProMPs to capture correlations between the toolpath parameters. The ProMP model is then exposed in Grasshopper, where it functions as a translator from drawing input to toolpath output. With our pipeline, makers can create simplified 2D drawings of their carving patterns with common CAD tools and then seamlessly generate skill-informed 6 degree-offreedom carving toolpaths from them, all in the same familiar CAD environment. We demonstrate our pipeline on multiple wood carving applications and discuss its limitations, including the need for iterative toolpath adjustments to address inaccuracies. Our findings illustrate the potential of LfD in augmenting CAM tools for specialized and highly customized manufacturing tasks. At the same time, the question of how to best represent carving skills for flexible and generalizable toolpath generation remains open and requires further investigation.
Keywords: Digital Fabrication, Learning from Demonstration (LFD), Computer-aided manufacturing (CAM), Robot Wood Carving, Probabilistic Movement Primitives (ProMPs), Grasshopper/Rhino Integration, Skill-Based Toolpath Generation, Human-Robot Collaboration in Fabrication
Received: 31 Jan 2025; Accepted: 26 Mar 2025.
Copyright: © 2025 Schäle, Stoelen and Kyrkjebø. This is an open-access article distributed under the terms of the Creative Commons Attribution License (CC BY). The use, distribution or reproduction in other forums is permitted, provided the original author(s) or licensor are credited and that the original publication in this journal is cited, in accordance with accepted academic practice. No use, distribution or reproduction is permitted which does not comply with these terms.
* Correspondence:
Daniel Schäle, Western Norway University of Applied Sciences, Bergen, Norway
Disclaimer: All claims expressed in this article are solely those of the authors and do not necessarily represent those of their affiliated organizations, or those of the publisher, the editors and the reviewers. Any product that may be evaluated in this article or claim that may be made by its manufacturer is not guaranteed or endorsed by the publisher.
Research integrity at Frontiers
Learn more about the work of our research integrity team to safeguard the quality of each article we publish.