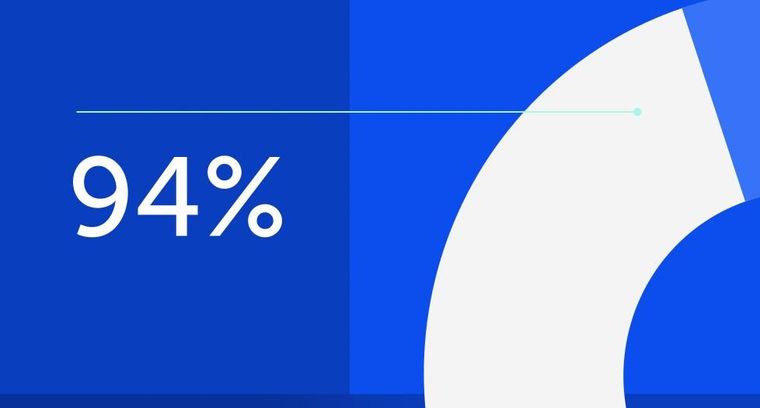
94% of researchers rate our articles as excellent or good
Learn more about the work of our research integrity team to safeguard the quality of each article we publish.
Find out more
ORIGINAL RESEARCH article
Front. Robot. AI, 01 April 2025
Sec. Human-Robot Interaction
Volume 12 - 2025 | https://doi.org/10.3389/frobt.2025.1563923
This article is part of the Research TopicPersonalized Robotics: Capturing Variability in Child–Robot Interactions in Education, Healthcare, and Daily LifeView all articles
This work aims to advance the understanding of group dynamics in robot-child interactions, focusing on whether, during a motor-imitation task led by a Nao robot, children might be influenced in their action executions by other group members - human or robotic. After testing eighteen groups of four children and teenagers, our findings indicate that participants tend to disregard the robot when it performs atypical gestures, preferring instead to imitate the actions of a human peer. Moreover, we found evidence that, in this scenario, assigning a leadership role to the robot does not, by itself, guarantee compliance from human group members; broader group dynamics must also be taken into account. Further results show that participants are significantly more likely to imitate the robot’s action when the “proactive” group members (i.e., those who initiate actions first) conform to Nao, compared to when they do not. Previous studies suggest that the mutual influence of group members can facilitate interaction with a robotic agent; however, our findings show that the presence of proactive members could also undermine the group’s conformity to the robot. Additionally, these findings highlight the importance of personalizing robots to better integrate into specific group dynamics, enhancing their ability to influence different groups effectively.
Integrating social robots into daily life is one of the ultimate goals for the Human-Robot Interaction (HRI) field, with research focusing, for instance, on robots as caregivers (Sorell and Draper, 2014) or teachers (Belpaeme et al., 2018). In pursuing this goal, researchers cannot ignore the need to provide social robots with the ability to interact with groups of people. Recognizing groups’ most relevant features and studying group dynamics - i.e., the influential interpersonal processes that occur in and between groups over time (Forsyth, 2018) - would provide insights into how to train robots to interact with groups of humans and deal with their members efficiently and naturally. Most of the knowledge in HRI focuses on dyadic interactions. However, in the last decade, non-dyadic HRI publications have increased significantly (Schneiders et al., 2022).
One area of HRI that generates significant interest and could benefit from non-dyadic research is the use of social robots in education (Belpaeme et al., 2018). Understanding the psychological and behavioral mechanisms that come into play during interactions between groups of students and robots would allow to design and deploy robots that are effective and fair as tools or companions for education. Given that students are often children or teenagers, it is crucial to conduct studies that explore group dynamics in these age groups, with a focus on how they interact with and perceive a robot inserted into their team. Additionally, previous social psychology research - e.g., Turner et al. (1994) - emphasizes the relevance of group interaction for many elements of human development, such as the formation of personal identity. Therefore, it is vital to explore these dynamics in groups of young individuals.
This research can provide valuable insights into the social factors influencing group behavior and can guide the design of robots that foster effective interactions with young individuals. In particular, we investigate how a social robot influences groups of children and teenagers during a motor imitation game. Additionally, we examine how the robot’s impact can be shaped by the social influence exerted by other group members. To do so, we propose a gamified interaction context based on an imitation task (Figure 1 shows a moment of the interaction). During the game, the robot mimed actions in a Typical or Atypical way, thereby conforming or not conforming to the generally acknowledged manner to carry out such actions (see Section 3.3 for more details).
Figure 1. A group of participants mimicking the action “exult” with Nao during the motor imitation game. The side players are imitating the Atypical gesture of the robot, while the central ones are performing the action in the Standard way.
Studying robots’ influence on young people could be relevant to educational settings, where robots might act as tutors or teachers, pushing students to explore new, unfamiliar concepts. By identifying the conditions under which students are more likely to follow a robot’s guidance, even when it introduces ideas students have never encountered before, we can develop adaptive robotic tutors that personalize their approach based on group composition, promoting more effective learning. Another possible application could be in the domain of children’s safety. Understanding how and when children follow robots, even when they exhibit atypical behaviors, could provide insights into their susceptibility. This could be particularly significant, as children and teenagers have been found to be more keen to be influenced than adults (Vollmer et al., 2018; Costanzo and Shaw, 1966). To address these issues, we formulated the following research questions:
Q1: Do participants conform to a robot’s atypical behavior during a social game?
Q2: Are the participants influenced by the other group members during a social game with a robot?
Studying groups is a complex task that cannot rely only on examining individuals separately; indeed, that would mean ignoring fundamental insights such as mutual influences and social contexts (Forsyth, 2018). Previous works have shown some of the challenges and opportunities of investigating human-robot interaction on a group level.
In group decision-making, people often rely on implicit social norms, which can lead them to adjust their choices based on others’ input (Sherif, 1936). For instance, Asch (1951) demonstrated that people tend to be influenced by others’ evaluations even when situations are not ambiguous (i.e., evaluation of the length of lines). So far, it is unclear whether the influence of robots in decision-making scenarios within groups is similar to that exerted by humans. Studies using variations of the Asch experiment have found contradictory results Vollmer et al. (2018); Salomons et al. (2018); Qin et al. (2022); Brandstetter et al. (2014). Previous results also suggest that robots can influence group dynamics when they are active participants (Oliveira et al., 2021). Moreover, some studies found that robots can be useful mediators in some conditions. Gillet et al. (2021) observed that a robot’s adaptive gaze behavior could shape the interaction among participants, leading to more participation during a group conversation; others suggest that robots could improve team performance by managing crucial team processes like conflict (Martelaro et al., 2015; Shen et al., 2018).
A factor to consider when integrating robots into human groups is the risk of group polarization. As group interaction impacts members by taking their evaluation as individuals to extremes (Moscovici and Zavalloni, 1969), integrating robots into groups could be harder than expected. A recent study found this tendency while investigating polarization in the context of a food-delivery robot: group members were highly influenced by their group mates in evaluating the robot, and they reported a lower trust towards the robot with respect to individual participants (Martinez et al., 2023). These patterns are consistent with other research showing that when people deal with robots, groups - especially cohesive ones - tend to have more negative attitudes toward the robotic devices than individuals (Preusse et al., 2021).
Group variability is an additional aspect to take into account. Social psychology research highlights how each member brings to the team a set of unique personal skills, abilities, and motivations, all of which shape how they act as team members. Researchers examined the complex interplay of personality, group composition, and performance by considering the kind of tasks the teams were performing and the personality traits of each member. They found that certain combinations of people, based on their personal motivations and personality traits, are more or less suited to specific types of tasks (Mathieu et al., 2014), which explains why different team compositions may lead to varying outcomes For instance, team members with high emotional stability help the group perform well in conjunctive tasks, but not in tasks that do not rely on coordination among members (Kramer et al., 2014). Another study evidenced that teams composed of all highly dominant individuals are less stable and less productive than groups that include a balance of dominant and less dominant members (Groysberg et al., 2011). Another example is that while a conscientious person fits in well with a team when other members are motivated to perform, that individual may not fit in less task-oriented teams Bell et al. (2015). The combination of different team members working on the same task can, therefore, lead to significantly different outcomes. This has also been observed in recent HRI studies. For instance, Gillet et al. (2022) conducted a participatory design study, asking three groups of teenagers to design and shape the behavior of the Nao robot to mediate and enhance discussion-based activities during a 2-week summer camp. They observed great differences in the designed robot behaviors and perceptions among the teams. Indeed, each team found a different use for the robot, adapting it to the group’s attitudes and identity. A recent study (Axelsson et al., 2023) found that participants’ perceptions of the robot’s current behaviors diverged between the groups tested after a group mindfulness practice in a public cafe. These results together with findings by previous social psychology research suggest that the perception and use of robots may change depending on group members’ attributes and needs, and highlight the necessity for personalized robotics. In the future, efficient and adaptable robots should be able to understand the group with which they interact and tailor their behaviors consequently.
To design robots that effectively act as tutors or peers in learning tasks, it is important to evaluate their influence on the decision-making of users. Previous research has proven that robots can impact children’s choices and behaviors in social contexts (Stower et al., 2021; Vollmer et al., 2018). However, children’s perception and preferences towards robots seem to evolve rapidly with age (Cocchella et al., 2023; Stower et al., 2021; Sciutti et al., 2014; Kahn Jr et al., 2012), making it challenging to determine the extent of a robot’s influence across different age groups. Trust-related behaviors, for instance, often rely on the development of theory of mind: as children acquire this ability, they begin to evaluate whether an agent is trustworthy based on its informational access (Di Dio et al., 2020). Geiskkovitch et al. (2019) demonstrated that young children tend to trust a robot that has previously provided correct information, aligning with earlier findings in developmental psychology about children’s trust in previously reliable human agents (Birch et al., 2008). Yet in case participants can choose between human and robotic agents, younger children (3 y. o.) display a greater inclination to trust humans over robotic play partners, while older children (7 y. o.) tend to do the opposite (Di Dio et al., 2020). Considering an older population, Vollmer et al. (2018) observed that while adults resist social pressure from a group of small humanoid robots, children aged 7 to 9 conform to the robots’ suggestions. Concerning the age group considered in this study (9–13 years), we conducted a previous experiment where groups of children and teenagers participated in a team strategy game. The robot provided advice that was sometimes correct and sometimes incorrect (Pusceddu et al., 2025). Results show that participants rarely adjust their strategies based on the robot’s advice, especially after it makes mistakes. At the group dynamics level, a potential influence emerges between human players who consistently initiate moves and the rest of the team.
Building on our prior work, we examine robot influence in a spontaneous scenario rather than one requiring strategy. Playful activities have proven to be versatile tools in HRI, as they may be customized to various participant types and used to test different aspects of interaction (Rato et al., 2023; Pasquali et al., 2021; Strohkorb et al., 2016; Pereira et al., 2012; Castellano et al., 2009), while simultaneously reducing the Hawthorne effect, distracting people from the idea that they are experiment subjects and promoting unbiased human behaviors (Chiesa and Hobbs, 2008). For this reason, we developed a dance task designed to capture immediate and natural aspects of group influence, observing whether and how often participants imitate the robot during the game.
Research about imitation on preschool-aged children (1–3 years old) suggests that they imitate human actions more frequently and with greater accuracy than those performed by robots (Sommer et al., 2020; 2021). In older age groups, studies mostly focus on children and teenagers with autism spectrum disorder. Findings suggest that imitation learning from social robots is often more engaging than from human therapists for children with autism (Zheng et al., 2014; 2016). However, results found for this specific population are not easily generalizable to typically developing children. Although it was very valuable to analyze studies about children’s imitation in order to create our experimental design, our work does not focus on imitation performance but uses the phenomenon of imitation to evaluate the influence of a robotic agent within a group setting during a dance game. More specifically, we intend to explore how a robot’s actions shape group dynamics and influence action execution among elementary and early middle school children.
The participants were students involved in an educational project called NaoToKnow, which aimed to teach primary and secondary school students how to program Nao and some general concepts about social robotics. Further details about the NaoToKnow project can be found in the Acknowledgements section. A subset of the students participating in the above-cited initiative took part in our study after the approval of the Ethical Committee of the University of Genoa (protocol n. 20220317, 03/17/2022). Through the above-cited project, at the moment of the experiments, the participants were familiar with the Nao robot and had a basic understanding of its functionality. We can assume that this familiarity with the robot helped mitigate the novelty effect that is often present in HRI studies, where participants’ behavior may be influenced by initial excitement.
Seventy-two children between nine and 14 years old participated in the study (24 males, 46 females, 2 not declared; age:
The study was conducted in a real-world setting: the experimental sessions took place in a classroom of a school in Bracciano, Italy. The Nao robot was placed on a 75-cm-tall desk in the center of the room, facing the participants. Participants stood about 1 m apart one from the other and about 3 m from the robot (see Figures 1, 2 for reference). The designated position of every participant was marked on the floor with tape (1 strip for each foot). A 4K webcam with an integrated microphone to shoot the interaction was installed on a 180-cm tall tripod. A laptop was used to manage the recordings and to store the data temporarily. See Figure 2 for a schema of the setup.
Figure 2. Schema of the setup. (1) Designated positions of the participants; (2) Experimenter position with a laptop positioned behind a separator; (3) Nao on a desk; (4) Webcam with integrated microphone on a tripod.
The activity consisted of a game in which participants and the robot had to mime four actions whose detailed description can be found at the end of this section. The task was in the participants’ mother language (Italian); it lasted about 5 min and comprised two phases. The task is summarized in the diagram in Figure 3, and a video of it is available in the supplementary material.
1. Training: As the first thing, the robot explained the rules: it would name some actions, and the participants would have to perform them. For every one of the four actions, this Training phase was divided into two steps. In the former, the robot said the action out loud (e.g., “Waving!“) without performing it, and the four participants had to act it out (No Robot Influence phase). In the latter, the robot declared that it was going to show its own interpretation of the movement and performed it; after completing the action, Nao remained silent in a resting position for about 10 seconds to allow time for participants to express possible spontaneous reactions to the robot’s gesture before moving on to the next action (Spontaneous Reaction phase). See Figure 4 for a visual description of the Training phase.
2. Music Game: Nao said: “Now we will repeat all the gestures we have just tried out at the music rhythm” and announced each action out loud; the human participants and the robot executed it together to the rhythm of the Gioca Jouer song, popular in Italy.
Figure 4. Sequential images illustrating the composition of the Training phase. (a) Nao says the action out loud (“Fly” in the depicted case). (b) The participants perform the action without knowing the robot's version of it (No Robot Influence). (c) Nao performs the gesture. The third player from the left spontaneously imitates the robot, although not required by the game's rules (Spontaneous Reaction phase).
We designed the experiment with two phases because the Training allowed to understand how the participants perform the actions without the influence of the robot, so that it could be evaluated whether, in the Music Game, after seeing the robot’s actions, they decided to imitate it.
Nao performed two actions in a Typical way and two in an Atypical one. With Typical, we indicated a gesture the robot mimes as an average child does. In contrast, we programmed Nao to perform Atypical actions in an unconventional way, dissimilar to how a person would act. The Typical actions were needed as a baseline to investigate how participants would respond to Atypical actions. This classification was based on the common association between the Gioca Jouer song and its widely recognized dance movements in Italy. We assumed that these gestures reflect a general consensus on how such actions are typically mimed. It was confirmed that the selected gestures were suitable because most participants executed them with a Standard style during the Training phase, even before observing Nao’s version of the gesture (see Section 4 for more details).
The following list contains detailed descriptions of the gestures made by the robot during the experimental tasks in the order of execution:
After the activity, participants responded to a questionnaire on a tablet through Survey Monkey1. They were asked if they had imitated the robot during the task; then, they answered an adapted version of the Group Environment Questionnaire (Carron et al., 1985), a survey created to measure group cohesion, on a five-point Likert scale. An attention-bump item (“Answer 5 to this question to demonstrate your attention”) was added to verify that the participants were focused on the task. In total, 67 answers to the questionnaire were collected, as 5 participants were excluded because of the invalid answer to the attention bump item.
We performed a post hoc analysis of the video recordings concerning annotations on objective aspects of participants’ behavior. To ensure a reliable and systematic annotation and minimize the annotators’ mistakes, the observations were independently made by two individuals chosen among the authors. Their notes were then mediated by a third person, following the approach of Waseem and Hovy (2016). In our case, the third party was a social psychologist, familiar with this methodology.
The annotators worked autonomously without influencing each other. The inter-annotator agreement resulted in an
These annotations were made not only to collect data for the analyses in this study but also to have a ground truth to be potentially used in the future to validate automatic video analysis software.
We distinguished among two types of participants’ action styles: (i) Standard: the participant performs the action in a human-like way, i.e., they conform to Nao in the Typical actions but behave differently from Nao for the Atypical actions; (ii) Non-standard: the participant performs the Atypical actions in the same way as Nao in the Atypical actions; the participant performs the Typical action in a non-expected way. Mixed executions, i.e., the participant performs the gesture partially in a Standard way, partially in an Non-standard one, were considered Non-standard. There were no cases in which children performed Non-standard gestures in a manner different from the Atypical gestures shown by NAO.
For every action in both the Training and the Music Game phases, the following annotations were made for each human participant:
1. The action style they used to perform the action;
2. Whether they were the first player to start the action;
3. Whether they spontaneously imitated the robot after it demonstrated its version of the action (in the Spontaneous Reaction phase).
From item (1) of the list, we computed the frequency of possible action styles for every player and group in every task’s phase, to gain an insight about their tendency to conform to the others. Through (2), we could identify which participant was the initiator for every trial and compute the occurrence of starting-as-first times for every player, hence assessing their proactiveness. Item (3) provided instead an indirect measure of the engagement level of the participant, who spontaneously decides to imitate the robot.
From the observation of group behaviors, we noticed that some groups presented a player keener on starting the action without waiting for the others. We hypothesized that the presence of such a player could drive some dynamics of the whole group and somehow influence the decision to imitate the robot.
We then formulated a criterion to distinguish these groups from the others. We only considered the rounds of Atypical gestures because they were the trials in which it was more evident whether the players conformed to other members, including the robot. We identified as Strong Proactive Player (SPP) groups the teams in which one member initiated the gesture first at least 75% of the time, of which at least one solo. Sometimes more than one player started the action together.
The results of the survey reveal that, when the participants were asked whether they imitated the robot during the Music Game, 69.8% of them answered “yes”, 22.2% “sometimes”, and 7.9% “no”. According to the annotations, this is in line with participants’ actual behavior for the Standard actions in the Music Game phase, in which they acted as the robot in 98.6%. However, in only 43.8% of the Atypical trials, in the Music Game phase, participants performed the Atypical actions in a Non-standard way (i.e., in the same way or partially as Nao).
In the Training phase, before seeing Nao’s version of the actions, children mostly performed the gestures in a Standard way (88.2%) with respect to a Non-standard style (11.8%), thus validating our design choices for Nao’s execution of the Typical and Atypical actions. Additionally, if participants perform the gestures in a Standard manner before observing the robot’s actions, it demonstrates that the robot’s Atypical actions are distinct from their usual behavior, thereby justifying the selection of these Atypical actions.
Significant differences between the Training (No Robot Influence) and Music Game phases (after having seen Nao’s action) were found for the Atypical gestures, where the Non-standard executions of the participants doubled in the latter phase, with respect to the Training (Non-standard executions in Training: 21.5%, in Music Game: 43.8%. McNemar’s tests:
Figure 5. Comparison of players’ performance of Typical and Atypical gesture styles before (Training) and after (Music Game) exposure to Nao’s version. The plot highlights shifts in gesture style preferences before and after exposure to the robot’s action style, suggesting potential influence on participants’ behavior.
In the Spontaneous Reaction phase, participants naturally mimicked the robot in 35.4% of the trials, even though it was not part of the game’s rules. With 47.2% of trials, “drive” (Standard) was the most spontaneously imitated gesture, followed by “wave” (40.2%, Standard), “fly” (29.2%, Atypical), and “exult” (25.0%, Atypical). A Binomial Logistic Regression was conducted to verify if imitating the robot in the Spontaneous Imitation phase could predict the behavior in the Music Game phase for the Atypical actions, but the test did not produce any significant statistical results
We investigated whether, in trials where the robot performs the Atypical action (2 Atypical rounds per group, hence 36 Atypical rounds in total), players’ execution could be mediated by the presence of an initiator (i.e., a player who started the action before the other human participants). To do so, we analyzed every trial of the Atypical gestures (“Fly” and “Exult”) in the Music Game phase in which there was an initiator. These were 19 out of 36 rounds; in the remaining trials, more than one player started the action simultaneously. Since there are three potential “imitators” in each round, we conducted these analyses on a sample size of
Figure 6. Mosaic representations of the contingency tables illustrating the association between initiators/Proactive Players and other players’ action styles. The width of each segment corresponds to the relative frequency of occurrences. Chi-square tests results indicate a significant relationship between initiator/Proactive Player and other players’ actions, suggesting a potential influence of the former on subsequent players’ actions. (a) Single instance behavior. Representations of the association between initiators and other players' action styles. (b) Group behavior. Representations of the association between Proactive Players and other members’ action styles.
In light of these results, further considerations can be made regarding the imitation of Nao by the participants. In Figure 6a, it can be observed that, among the 36.8% of cases in which the initiator performs a Non-standard action, the other players match the Non-standard execution the 26.3% of the time, relative to these cases (as indicated by the orange box), with the remaining 10.5% where the other players perform the action in a Standard way (grey box, bottom right). An additional representation of these data can be found in the pie chart to the left of Figure 7: when the initiator makes a Non-standard reproduction of an Atypical action, 71.5% of the other members do the same. It can therefore be stated that players perform the action like the robot more frequently if the initiator has done the same. The opposite is also true: when the initiator decides not to imitate the robot, the other players do the same (blue box).
The groups of participants attended the same school class. We could not know in detail the dynamics of pre-existing relationships among group members, but to acquire a general idea of their cohesiveness, we ran Group Environment Questionnaires. A One-Sample t-test against the neutral value (3, on a 1-5 Likert scale) revealed that, on average, participants perceive their team as cohesive
According to the group categorization presented in the Analyses, we identified 10 Strong Proactive Player (SPP) groups out of 18. For each SPP group, we identified one Proactive Player (see Section 3.5.2 for further details).
Similarly to the Single Instance Behavior analyses, we focused on the SPP groups during Nao’s execution of Atypical actions in the Music Game phase (10 SPP groups with 3 non-Proactive Players, 2 rounds, hence
After evaluating the affinity between the Proactive Player and the other players, we now assess the execution of the participants’ action styles in relation to that of the robot. The results presented in Figure 6b show that when the Proactive Player decides not to imitate Nao (60.0% of the instances), the other players do the same in the majority of cases (45.0%, blue box), and less frequently decide to imitate the robot anyway (15.0%, grey box at the top left). From the same figure, it can be seen that when the Proactive Player conforms to Nao (40.0%), players tend to imitate the robot’s actions more frequently (28.3% out of 40%, orange box), and perform a Standard reproduction less frequently (11.7% out of 40.0%, bottom right grey box); indeed, the orange box is larger than the grey box at the bottom right. For clarity, this data is also presented in Figure 7, which shows that when the Proactive Player performs an Atypical action like the robot (Non-standard reproduction), the other players do the same in 70.8% of cases.
This work investigates the influence exerted by human peers and the robot Nao on the behavior of the group members during a motor imitation game. In particular, (1) we explored the influence of the robot on group members, investigating whether participants conform to the unconventional behavior of a robot during a social game; (2) we then studied if the influence of the robot on group members might be mediated by human peers.
The study was conducted in a natural school setting rather than a controlled laboratory environment. This approach offers the advantage of observing children in a familiar context, likely leading to more authentic behavior even though it makes obtaining precise measurements more difficult.
In the questionnaire, most of the participants declared to have imitated the robot during the game, but they did not entirely. On one side, the children might feel they have imitated because, in the Standard cases, they performed the action like the robot. However, a disparity was highlighted between the self-reported measures and the actual observation of the Atypical actions. In the questionnaire, participants declared to have imitated the robot more with respect to the video observations. We hypothesize that participants might not want to disclose their lack of willingness to imitate the robot, suggesting they chose the answer they judged as “right”. This might be due to social desirability, i.e., the tendency for individuals to respond in culturally sanctioned ways (Stöber, 2001; Grimm, 2010). We also hypothesize that this behavior might be attributed to the experiments taking place in a school where students are continuously evaluated. Another possible explanation for this result is that participants did not perceive Nao’s Atypical actions as different or interpreted them merely as mistakes by the robot. As a result, they may have been following what they believed the robot intended to do rather than what it actually did. Even though they did not imitate Nao as much as they declared, some showed engagement and curiosity towards the robot as demonstrated by their propensity to imitate it spontaneously; indeed, in the Spontaneous Reaction phase, they imitated the robot’s actions even when it was not required by the game’s rules.
In the vast majority of the trials, participants performed the actions with a Standard style in the Training phase, before seeing Nao’s version. It was then verified that the chosen gestures provided a solid baseline to evaluate Typical and Atypical action styles.
It was unexpected that, despite the robot being positioned in a leadership-like role—placed on the teacher’s desk (“head-of-the-table effect” (Forsyth, 2018)), dictating rules, and guiding the interaction—participants imitated its movements in fewer than half of the trials. These findings might suggest that young people are unwilling to follow a robotic leader if they perceive its indications as unusual or out of their comfort zone. Another reason for this behavior could be that, even though we intended to put Nao in a leadership position, participants did not perceive it as such, similar to a previous study Alves-Oliveira et al. (2016), in which students evaluated Nao as a peer, even though it was acting as a teacher. Another possible explanation for this behavior, similar to the one mentioned above regarding the discrepancy between actual and self-reported measures, is that children may not have fully noticed the deviation from the Typical actions or may have perceived it simply as a mistake by Nao. As a result, they might have been following what they believed Nao intended to do rather than what it actually did. Future studies should investigate this by, for example, asking children whether they noticed that Nao performed the actions incorrectly and why they believed this to be the case. An additional interpretation of why participants rarely performed atypical actions is that they might have felt uncomfortable doing so, as such movements might contrast with group social norms and could elicit ostracism (Forsyth, 2018). This feeling could be heightened if group members perceive their group as heterogeneous since exclusionary reactions are more likely in such cases (Gerard, 1953). However, given that participants reported a high level of group cohesion in the Group Environment Questionnaire, we can reasonably exclude heterogeneity as a contributing factor. Future studies should incorporate a scale to measure social desirability to determine whether this factor could be correlated to the observed behavior (Stöber, 2001).
Results show that initiators influenced others’ behaviors. We found that players made the same action as the robot significantly more often when the initiator conformed to Nao in comparison to when they did not. The same effect was found considering the SPP groups only, referring to a single Proactive Player for each group in place of one initiator for each trial. These findings might indicate that the initiator’s behavior influences the tendency of the other members to conform to Nao and that, in front of the unconventional behavior of a social robot, young participants prefer to imitate a human peer rather than conform to the robotic member of the group. Interestingly, similar results were found in our previous study in a strategy game scenario (Pusceddu et al., 2025), thus suggesting that taking the initiative is a leadership trait (King, 2010; Yoo and Alavi, 2004), despite of the task type.
This observation is relevant for HRI because the acceptance and interaction with a robotic agent, which can be challenging for shy or distrustful children or teenagers, could be enhanced by inserting them in groups with peers who are more willing and predisposed to accept or follow the robot. This could also be reflected in educational contexts. If a robot tries to guide children through unfamiliar concepts or atypical behaviors, it might not be listened to by the students; this would limit its effectiveness as an educational tool. However, a robot tutor aligning with the tendencies of proactive students could enhance engagement, making it a more effective tool for promoting learning. These results could also be relevant in the field of children’s safety. The findings suggest that children and teenagers rarely follow a robot that displays atypical behavior. However, they tend to follow proactive members of the group, which could pose a risk if those proactive members were to rely on a robot with unusual behavior without questioning it. Thus, these findings open up possibilities for leveraging robots’ influence in groups for children’s benefit, but also raise concerns about how this influence could have negative effects.
To investigate this effect deeper, in future studies, it would be useful to observe how participants would behave in the presence of a proactive player acting atypically and a robot acting in a standard way. Furthermore, groups with a Strong Proactive Player might have other peculiarities worth studying. For example, the presence of a proactive player might impact the group’s cohesion. From another perspective, in groups without proactive human members, a robot exhibiting proactive qualities could play a valuable role in improving team performance and unity.
In general, dividing groups into categories might help better understand their different strengths and needs. Based on this study, a possible criterion for categorization could be the presence or absence of proactive group members. This kind of classification could allow researchers to design and personalize robots to better align with the dynamics of each group to help improve performance and enjoyment.
This study also presents some limitations. For instance, the actions pool was limited to only four, due to time constraints. Testing a wider variety of actions would be of interest and could provide stronger conclusions. In addition, as the groups were not perfectly homogeneous with respect to age and gender and because the sample size was limited, it was not possible to perform reliable analyses to test for any effects of the subjects’ age and gender. As a future improvement, the manual annotation analysis could be substituted with automatic computer vision software, using the annotations of this study as ground truth to test the algorithms. Automating the annotations would not only simplify and accelerate the analyses for researchers but also open the possibility for real-time behavioral analysis. This advancement could enable robots to dynamically monitor group behaviors, including those of proactive participants, and adapt their actions accordingly.
In conclusion, this study explores how, in playful contexts with children and teenagers, it is not enough to assign a leadership role to the robot to ensure children’s compliance with the robotic partner: group dynamics must be considered. Despite some limitations, our results add a new dimension to this research, showing that the presence of proactive group members can influence their peers, potentially enhancing or undermining the group’s conformity to the robot.
The raw data supporting the conclusions of this article will be made available by the authors, without undue reservation.
The studies involving humans were approved by Ethical Committee of University of Genoa (protocol no. 20220317, 03/17/2022). The studies were conducted in accordance with the local legislation and institutional requirements. Written informed consent for participation in this study was provided by the participants’ legal guardians/next of kin.
GP: Conceptualization, Data curation, Investigation, Methodology, Software, Writing – original draft, Writing – review and editing, Formal Analysis, Project administration, Resources, Visualization. FC: Conceptualization, Data curation, Investigation, Methodology, Writing – review and editing, Formal Analysis, Resources, Visualization. MB: Conceptualization, Funding acquisition, Investigation, Methodology, Project administration, Resources, Writing – review and editing, Data curation. GB: Conceptualization, Investigation, Methodology, Writing – review and editing. LL: Conceptualization, Methodology, Writing – review and editing. FR: Conceptualization, Investigation, Methodology, Supervision, Writing – review and editing. MC: Conceptualization, Resources, Supervision, Writing – review and editing. AS: Conceptualization, Methodology, Supervision, Writing – review and editing.
The author(s) declare that financial support was received for the research and/or publication of this article. The NaoToKnow project was funded under the PNSD national call for proposals “STEAM Methodologies” and implemented by the national network of school Institutes “ARETE+4Nao”, of which the Istituto Comprensivo “Goffredo Mameli” of Palestrina (RM) directed by DS Professor Ester Corsi is the leader, involving twenty-three Comprehensive Institutes in the Italian regions of Lazio, Abruzzo, Campania, Sardinia, Lombardy, Piedmont and Emilia-Romagna.
Thanks to Scuola di Robotica for the support, with particular reference to Filippo Michela Bogliolo, Gianluca Pedemonte, and Emanuele Micheli.
The authors declare that the research was conducted in the absence of any commercial or financial relationships that could be construed as a potential conflict of interest.
The author(s) declared that they were an editorial board member of Frontiers, at the time of submission. This had no impact on the peer review process and the final decision.
The author(s) declare that Generative AI was used in the creation of this manuscript. While preparing this work, the authors used Grammarly and GPT 4.0 to check the grammar and improve the manuscript’s readability. After using these tools, the authors reviewed and edited the content as needed and took full responsibility for the publication’s content.
All claims expressed in this article are solely those of the authors and do not necessarily represent those of their affiliated organizations, or those of the publisher, the editors and the reviewers. Any product that may be evaluated in this article, or claim that may be made by its manufacturer, is not guaranteed or endorsed by the publisher.
The Supplementary Material for this article can be found online at: https://www.frontiersin.org/articles/10.3389/frobt.2025.1563923/full#supplementary-material
Alves-Oliveira, P., Sequeira, P., and Paiva, A. (2016). “The role that an educational robot plays,” in 2016 25th IEEE International symposium on robot and human interactive communication, 817–822.
Asch, S. E. (1951). Effects of group pressure upon the modification and distortion of judgments. Groups, Leadersh. men, 177–190.
Axelsson, M., Spitale, M., and Gunes, H. (2023). “Robotic coaches delivering group mindfulness practice at a public cafe,” in Companion of the 2023 ACM/IEEE international conference on human-robot interaction, 86–90.
Bell, S. T., Brown, S. G., Abben, D. R., and Outland, N. B. (2015). Team composition issues for future space exploration: a review and directions for future research. Aerosp. Med. Hum. Perform. 86, 548–556. doi:10.3357/amhp.4195.2015
Belpaeme, T., Kennedy, J., Ramachandran, A., Scassellati, B., and Tanaka, F. (2018). Social robots for education: a review. Sci. robotics 3, eaat5954. doi:10.1126/scirobotics.aat5954
Birch, S. A., Vauthier, S. A., and Bloom, P. (2008). Three-and four-year-olds spontaneously use others’ past performance to guide their learning. Cognition 107, 1018–1034. doi:10.1016/j.cognition.2007.12.008
Brandstetter, J., Rácz, P., Beckner, C., Sandoval, E. B., Hay, J., and Bartneck, C. (2014). “A peer pressure experiment: recreation of the asch conformity experiment with robots,” in 2014 IEEE/RSJ international Conference on intelligent Robots and systems (IEEE), 1335–1340.
Carron, A. V., Widmeyer, W. N., and Brawley, L. R. (1985). The development of an instrument to assess cohesion in sport teams: the group environment questionnaire. J. Sport Exerc. Psychol. 7, 244–266. doi:10.1123/jsp.7.3.244
Castellano, G., Pereira, A., Leite, I., Paiva, A., and McOwan, P. W. (2009). “Detecting user engagement with a robot companion using task and social interaction-based features,” in Proceedings of the 2009 international conference on multimodal interfaces, 119–126.
Chiesa, M., and Hobbs, S. (2008). Making sense of social research: how useful is the hawthorne effect? Eur. J. Soc. Psychol. 38, 67–74. doi:10.1002/ejsp.401
Cocchella, F., Pusceddu, G., Belgiovine, G., Bogliolo, M., Lastrico, L., Casadio, M., et al. (2023). “At school with a robot: Italian students’ perception of robotics during an educational program,” in 2023 32nd IEEE international conference on robot and human interactive communication (Busan, Republic of Korea: RO-MAN), 1413–1419. doi:10.1109/RO-MAN57019.2023.10309379
Costanzo, P. R., and Shaw, M. E. (1966). Conformity as a function of age level. Child. Dev. 37, 967–975. doi:10.2307/1126618
Di Dio, C., Manzi, F., Peretti, G., Cangelosi, A., Harris, P. L., Massaro, D., et al. (2020). Shall i trust you? from child–robot interaction to trusting relationships. Front. Psychol. 11, 469. doi:10.3389/fpsyg.2020.00469
Geiskkovitch, D. Y., Thiessen, R., Young, J. E., and Glenwright, M. R. (2019). “What? that’s not a chair!: how robot informational errors affect children’s trust towards robots,” in 2019 14th ACM/IEEE international Conference on human-robot interaction (HRI) (IEEE), 48–56.
Gerard, H. B. (1953). The effect of different dimensions of disagreement on the communication process in small groups. Hum. Relat. 6, 249–271. doi:10.1177/001872675300600303
Gillet, S., Cumbal, R., Pereira, A., Lopes, J., Engwall, O., and Leite, I. (2021). “Robot gaze can mediate participation imbalance in groups with different skill levels,” in Proceedings of the 2021 ACM/IEEE international conference on human-robot interaction, 303–311.
Gillet, S., Winkle, K., Belgiovine, G., and Leite, I. (2022). “Ice-breakers, turn-takers and fun-makers: exploring robots for groups with teenagers,” in 2022 31st IEEE international conference on robot and human interactive communication (RO-MAN IEEE), 1474–1481.
Groysberg, B., Polzer, J. T., and Elfenbein, H. A. (2011). Too many cooks spoil the broth: how high-status individuals decrease group effectiveness. Organ. Sci. 22, 722–737. doi:10.1287/orsc.1100.0547
Kahn, Jr P. H., Kanda, T., Ishiguro, H., Freier, N. G., Severson, R. L., Gill, B. T., et al. (2012). “robovie, you’ll have to go into the closet now”: children’s social and moral relationships with a humanoid robot. Dev. Psychol. 48, 303–314. doi:10.1037/a0027033
King, A. J. (2010). Follow me! i’ma leader if you do; i’ma failed initiator if you don’t. Behav. Process. 84, 671–674. doi:10.1016/j.beproc.2010.03.006
Kramer, A., Bhave, D. P., and Johnson, T. D. (2014). Personality and group performance: the importance of personality composition and work tasks. Personality Individ. Differ. 58, 132–137. doi:10.1016/j.paid.2013.10.019
Martelaro, N., Jung, M., and Hinds, P. (2015). “Using robots to moderate team conflict: the case of repairing violations,” in Proceedings of the tenth annual ACM/IEEE international conference on (New York, NY: Association for Computing Machinery), 271.
Martinez, J. E., VanLeeuwen, D., Stringam, B. B., and Fraune, M. R. (2023). “Hey? ! what did you think about that robot? groups polarize users’ acceptance and trust of food delivery robots,” in Proceedings of the 2023 ACM/IEEE international conference on human-robot interaction, 417–427.
Mathieu, J. E., Tannenbaum, S. I., Donsbach, J. S., and Alliger, G. M. (2014). A review and integration of team composition models: moving toward a dynamic and temporal framework. J. Manag. 40, 130–160. doi:10.1177/0149206313503014
Moscovici, S., and Zavalloni, M. (1969). The group as a polarizer of attitudes. J. personality Soc. Psychol. 12, 125–135. doi:10.1037/h0027568
Oliveira, R., Arriaga, P., and Paiva, A. (2021). Human-robot interaction in groups: methodological and research practices. Multimodal Technol. Interact. 5, 59. doi:10.3390/mti5100059
Pasquali, D., Gonzalez-Billandon, J., Rea, F., Sandini, G., and Sciutti, A. (2021). Magic icub: a humanoid robot autonomously catching your lies in a card game, 293–302.
Pereira, A., Prada, R., and Paiva, A. (2012). “Socially present board game opponents,” in International conference on advances in computer entertainment technology (Springer), 101–116.
Preusse, H., Skulsky, R., Fraune, M. R., and Stringam, B. B. (2021). Together we can figure it out: groups find hospitality robots easier to use and interact with them more than individuals. Front. Robotics AI 8, 730399. doi:10.3389/frobt.2021.730399
Pusceddu, G., Sangineto, M., Cocchella, F., Bogliolo, M., Belgiovine, G., Lastrico, L., et al. (2025). “Exploring children’s strategies in responses to robot’s advice during a group task with icub and nao,” in 16th international conference on social robotics. (in press).
Qin, X., Chen, C., Yam, K. C., Cao, L., Li, W., Guan, J., et al. (2022). Adults still can’t resist: a social robot can induce normative conformity. Comput. Hum. Behav. 127, 107041. doi:10.1016/j.chb.2021.107041
Rato, D., Correia, F., Pereira, A., and Prada, R. (2023). Robots in games. Int. J. Soc. Robotics 15, 37–57. doi:10.1007/s12369-022-00944-4
Salomons, N., Van Der Linden, M., Strohkorb Sebo, S., and Scassellati, B. (2018). “Humans conform to robots: disambiguating trust, truth, and conformity,” in Proceedings of the 2018 acm/ieee international conference on human-robot interaction, 187–195.
Schneiders, E., Cheon, E., Kjeldskov, J., Rehm, M., and Skov, M. B. (2022). Non-dyadic interaction: a literature review of 15 years of human-robot interaction conference publications. ACM Trans. Human-Robot Interact. (THRI) 11, 1–32. doi:10.1145/3488242
Sciutti, A., Rea, F., and Sandini, G. (2014). “When you are young,(robot’s) looks matter. developmental changes in the desired properties of a robot friend,” in The 23rd IEEE international symposium on robot and human interactive communication (IEEE), 567–573.
Shen, S., Slovak, P., and Jung, M. F. (2018). “stop. i see a conflict happening.” a robot mediator for young children’s interpersonal conflict resolution. Proc. 2018 ACM/IEEE Int. Conf. human-robot Interact., 69–77. doi:10.1145/3171221.3171248
Sherif, M. (1936). The Psychology of social Norms.Muzafer sherif. Am. J. Sociol. 42, 420–421. doi:10.1086/217439
Sommer, K., Davidson, R., Armitage, K. L., Slaughter, V., Wiles, J., and Nielsen, M. (2020). Preschool children overimitate robots, but do so less than they overimitate humans. J. Exp. Child Psychol. 191, 104702. doi:10.1016/j.jecp.2019.104702
Sommer, K., Slaughter, V., Wiles, J., Owen, K., Chiba, A. A., Forster, D., et al. (2021). Can a robot teach me that? children’s ability to imitate robots. J. Exp. Child Psychol. 203, 105040. doi:10.1016/j.jecp.2020.105040
Sorell, T., and Draper, H. (2014). Robot carers, ethics, and older people. Ethics Inf. Technol. 16, 183–195. doi:10.1007/s10676-014-9344-7
Stöber, J. (2001). The social desirability scale-17 (sds-17): convergent validity, discriminant validity, and relationship with age. Eur. J. Psychol. Assess. 17, 222–232. doi:10.1027//1015-5759.17.3.222
Stower, R., Calvo-Barajas, N., Castellano, G., and Kappas, A. (2021). A meta-analysis on children’s trust in social robots. Int. J. Soc. Robotics 13, 1979–2001. doi:10.1007/s12369-020-00736-8
Strohkorb, S., Fukuto, E., Warren, N., Taylor, C., Berry, B., and Scassellati, B. (2016). “Improving human-human collaboration between children with a social robot,” in 2016 25th IEEE international symposium on robot and human interactive communication (New York, NY: IEEE Press), 551–556.
Turner, J. C., Oakes, P. J., Haslam, S. A., and McGarty, C. (1994). Self and collective: cognition and social context. Personality Soc. Psychol. Bull. 20, 454–463. doi:10.1177/0146167294205002
Vollmer, A.-L., Read, R., Trippas, D., and Belpaeme, T. (2018). Children conform, adults resist: a robot group induced peer pressure on normative social conformity. Sci. robotics 3, eaat7111. doi:10.1126/scirobotics.aat7111
Waseem, Z., and Hovy, D. (2016). “Hateful symbols or hateful people? predictive features for hate speech detection on twitter,” in Proceedings of the NAACL student research workshop, 88–93.
Yoo, Y., and Alavi, M. (2004). Emergent leadership in virtual teams: what do emergent leaders do? Inf. Organ. 14, 27–58. doi:10.1016/j.infoandorg.2003.11.001
Zheng, Z., Das, S., Young, E. M., Swanson, A., Warren, Z., and Sarkar, N. (2014). “Autonomous robot-mediated imitation learning for children with autism,” in 2014 IEEE international conference on robotics and automation (ICRA), 2707–2712. doi:10.1109/ICRA.2014.6907247
Keywords: child-robot interaction, group-robot interaction, group dynamics, robot influence, imitation, robots at school
Citation: Pusceddu G, Cocchella F, Bogliolo M, Belgiovine G, Lastrico L, Rea F, Casadio M and Sciutti A (2025) Who will you imitate? Studying reciprocal influence in children-robot groups during an imitation game. Front. Robot. AI 12:1563923. doi: 10.3389/frobt.2025.1563923
Received: 20 January 2025; Accepted: 21 March 2025;
Published: 01 April 2025.
Edited by:
Mike Ligthart, VU Amsterdam, NetherlandsReviewed by:
Isabel Neto, Technical University of Lisbon, PortugalCopyright © 2025 Pusceddu, Cocchella, Bogliolo, Belgiovine, Lastrico, Rea, Casadio and Sciutti. This is an open-access article distributed under the terms of the Creative Commons Attribution License (CC BY). The use, distribution or reproduction in other forums is permitted, provided the original author(s) and the copyright owner(s) are credited and that the original publication in this journal is cited, in accordance with accepted academic practice. No use, distribution or reproduction is permitted which does not comply with these terms.
*Correspondence: Giulia Pusceddu, Z2l1bGlhLnB1c2NlZGR1QGlpdC5pdA==
Disclaimer: All claims expressed in this article are solely those of the authors and do not necessarily represent those of their affiliated organizations, or those of the publisher, the editors and the reviewers. Any product that may be evaluated in this article or claim that may be made by its manufacturer is not guaranteed or endorsed by the publisher.
Research integrity at Frontiers
Learn more about the work of our research integrity team to safeguard the quality of each article we publish.