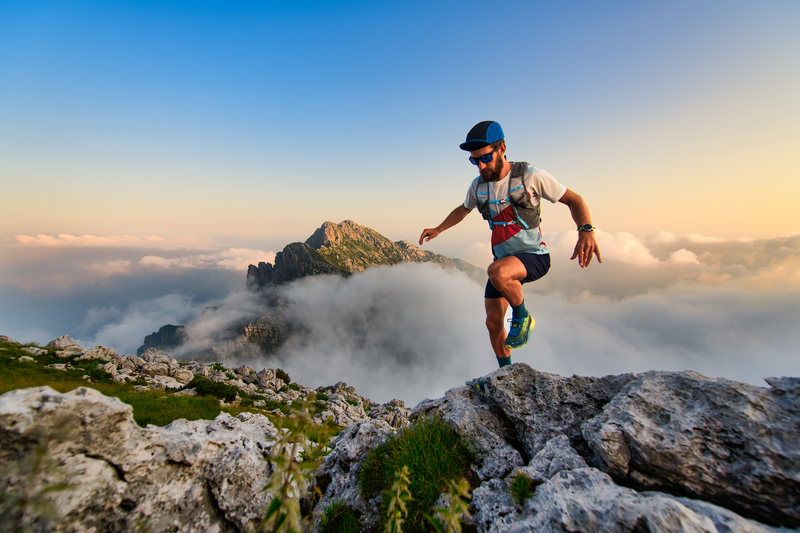
95% of researchers rate our articles as excellent or good
Learn more about the work of our research integrity team to safeguard the quality of each article we publish.
Find out more
ORIGINAL RESEARCH article
Front. Robot. AI
Sec. Computational Intelligence in Robotics
Volume 12 - 2025 | doi: 10.3389/frobt.2025.1441801
This article is part of the Research Topic Integrative Approaches with BCI and Robotics for Improved Human Interaction View all articles
The final, formatted version of the article will be published soon.
You have multiple emails registered with Frontiers:
Please enter your email address:
If you already have an account, please login
You don't have a Frontiers account ? You can register here
Piloting an aircraft is a cognitive task which requires continuous verbal, visual and auditory attentions (e.g Air Traffic Control Communication etc.). Increase or decrease in mental workload from a specific level can alter auditory and visual attention resulting in pilot errors. The objective of this research is to monitor pilot's mental workload with the help of advance machine learning techniques to achieve improved accuracy compared to previous studies. Electroencephalogram (EEG) data were recorded from twenty-two pilots operating under visual flight rules (VFR) conditions using a six dry-electrode enobio neuroelectrics system, and the riemannian artifact subspace reconstruction (rASR) filter was used for data cleaning. Information gain (IG) attribute evaluator has been used to select 25 optimal features out of 72 power spectral and statistical extracted features. In this study 15 classifiers have been used for classification purpose. Multinomial logistic regression with ridge estimator has been selected, achieving a significant mean accuracy of 84.6% on the dataset from 17 subjects. Data were initially collected from 22 subjects, but five were excluded due to data synchronization issues. This work has several limitations like the author did not counter balance the order of scenario, could not control all the variables such as wind conditions, workload was not stationary in each leg of the flight pattern. This study demonstrates that multinomial logistic regression with ridge estimator shows the significant classification accuracy (p<0.05) and effectively detects pilot mental workload in real flight scenarios.
Keywords: Dry-electrode EEG, Real flight conditions, Artifact Subspace Reconstruction (ASR), Auditory Attention, Enobio Neuroelectrics system, Passive Brain Computer Interface
Received: 31 May 2024; Accepted: 24 Mar 2025.
Copyright: © 2025 Haseeb, Nadeem, Sultana, Naseer, Gilani and Dehais. This is an open-access article distributed under the terms of the Creative Commons Attribution License (CC BY). The use, distribution or reproduction in other forums is permitted, provided the original author(s) or licensor are credited and that the original publication in this journal is cited, in accordance with accepted academic practice. No use, distribution or reproduction is permitted which does not comply with these terms.
* Correspondence:
Noman Naseer, Air University, Islamabad, 44000, Islamabad, Pakistan
Disclaimer: All claims expressed in this article are solely those of the authors and do not necessarily represent those of their affiliated organizations, or those of the publisher, the editors and the reviewers. Any product that may be evaluated in this article or claim that may be made by its manufacturer is not guaranteed or endorsed by the publisher.
Research integrity at Frontiers
Learn more about the work of our research integrity team to safeguard the quality of each article we publish.