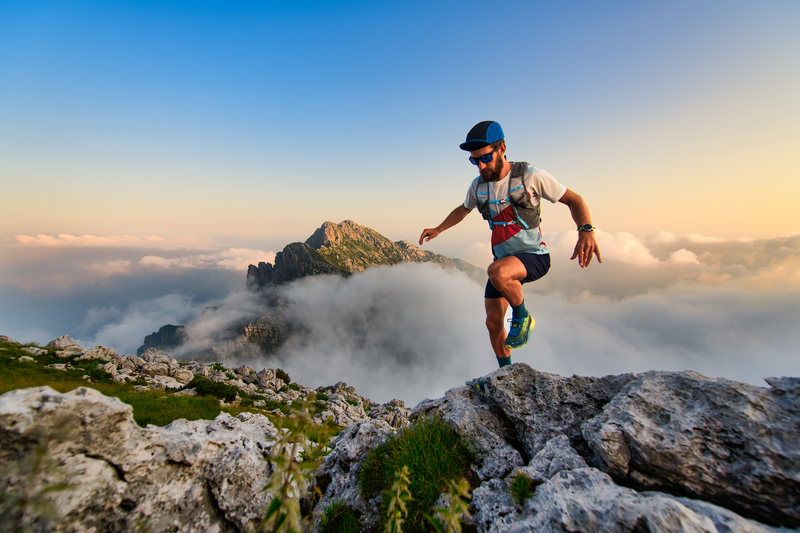
94% of researchers rate our articles as excellent or good
Learn more about the work of our research integrity team to safeguard the quality of each article we publish.
Find out more
EDITORIAL article
Front. Robot. AI , 11 July 2022
Sec. Robotic Control Systems
Volume 9 - 2022 | https://doi.org/10.3389/frobt.2022.973208
This article is part of the Research Topic Current Challenges and Future Developments in Robot Grasping View all 6 articles
Editorial on the Research Topic
Current Challenges and Future Developments in Robot Grasping
Robot grasping describes a robot’s ability to grasp and manipulate objects which is one of the most desired capabilities for a fully functional robot system in many applications. However, grasping is one of the hardest problems in robotics since it has to deal with complex perception issues, planning and executing difficult and careful interactions, and requires advanced devices and reasoning.
As a consequence, it is a field which has attracted a great deal of interest and research over the last decades, which has resulted in many advances and an active area of research and development. In the last few years, research related to robot grasping has focused on two main aspects. The first is the application of deep learning techniques to robot grasping in order to apprehend and overcome most of the difficult cognitive problems. These applications have not only addressed the perception, but also the planning and control aspects of grasping. The second aspect is the design of novel grippers or robot hands applying principles of soft mechanisms, with the purpose of providing more adaptable and versatile devices. Nevertheless, other more traditional research lines are still present and should not be neglected in a complete view of robot grasping. These include perception, mostly vision but also haptics; planning, which involves grasp synthesis, analysis and metrics; control and specific applications.
The papers collected in this Research Topic provide a good example of the broad spectrum of areas of research that still present a lot of challenges. The first paper, with title “DGCM-Net: Dense Geometrical Correspondence Matching Network for Incremental Experience-Based Robotic Grasping,” by Patten et al. presents a method for grasping novel objects by learning from previous successful experiences. The successful grasps are stored in a database, encoding objects with similar geometries close to each other in a feature space. Then, looking for a grasp for a new object is based on a nearest neighbour search in this feature space and an adaptation of the existing grasps to the new object. Specific techniques are developed as part of the contribution to deal with this problem. The approach also allows an improvement in the grasp success rate when experience is accumulated. Real experiments are reported to validate the proposed approach.
The following paper “Leveraging Human Perception in Robot Grasping and Manipulation Through Crowdsourcing and Gamification” by Gorjup et al. presents a framework that combines crowdsourcing and gamification to leverage human intelligence, enhancing the object recognition and attribute estimation processes of robot grasping. The framework is aimed to facilitate the assignment of semantic attributes of visual instances of objects. This is done by a crowdsourcing framework where different human subjects collaborate in the recognition of such attributes. The relevant contribution of the paper is the introduction of a gamified interface to ease and motivate the task of the human collaborators. The paper presents two cases that demonstrate the usefulness of the approach: the control of an exoskeleton while manipulating objects and the object recognition and grasping for a dual-arm service robot.
The third paper, with title “Aiding Grasp Synthesis for Novel Objects Using Heuristic-Based and Data-Driven Active Vision Methods,” by Natarajan et al., presents an approach to optimise a sequence of viewpoints of an object to efficiently collect the necessary data for the grasp synthesis using a two-finger parallel gripper. The paper describes two optimization methods, based on heuristics and considering a depth camera mounted on the robot arm. The work includes an extensive set of simulated and real experiments to validate relevant statistical results and comparisons with baseline methods.
The next work “Grasp Stability Prediction for a Dexterous Robotic Hand Combining Depth Vision and Haptic Bayesian Exploration” also addresses the issue of stability prediction for grasp selection, but from a different point of view. Siddiqui et al. propose a framework for determining stable and safe grasping actions on unknown or un-modelled objects, based on a multimodal visual and tactile exploration. A system composed by camera, articulated arm and dexterous hand with tactile feedback is used in the final robot experiments, building upon simulated tests for initial safety and performance assessment. Visual analysis through an RGB-D sensor is employed to establish initial contact points based on an estimated quality metric on the visible object surface. Tactile exploration is performed starting from the moment the hand touches the object and different exploration methods (standard and unscented Bayesian Optimisation and uniform grid search) are employed to find a stable contact area for the fingers in order to achieve a reliable grip. Results show that probabilistic methods, particularly the unscented Bayesian Optimisation, can provide confident stability predictions after only a few nexploratory observations.
Finally, Meattini et al. on their work “Simulative Evaluation Of A Joint-Cartesian Hybrid Motion Mapping For Robot Hands Based On Spatial In-Hand Information,” proposed a way of combining two approaches to improve the control of the robot hands when using teleoperation exploiting the spatial information available in-hand related to the thumb-fingers relative position, for combining joint and Cartesian mappings. This allows to improve the performance of a large range of grasps both volar (where the preservation of finger shapes is more important) and precision grips (where the preservation of fingertip positions is more important). The evaluation was done using simulation of four well-known robot hands showing that both approaches were capable of successfully performing the mapping of finger shapes and fingertip positions, on both anthropomorphic and non-anthropomorphic robot hands. It will be interesting to see in future works the performance of these experiments with real robot hands to investigate the specific telemanipulation improvements using the proposed mapping.
This Research Topic of papers provides a useful insight on recent, diverse, approaches to the realm of robot grasping and its most recent advances. It also outlines important scientific challenges that are still open and provide interesting opportunities for further research and progress.
AM, BL, EC and RS have contributed to the writing of the editorial and have managed the review of at least one paper of the Research Topic.
This work was partially supported by the Spanish Government through the project PID2020‐114819GB‐I00 and PDC2021‐121011‐I00, by Generalitat Valenciana through project PROMETEO/2020/034 and by Universitat Jaume I through project UJI‐B2021‐27.
Author BL was employed by the company Shadow Robot Company.
The remaining authors declare that the research was conducted in the absence of any commercial or financial relationships that could be construed as a potential conflict of interest.
All claims expressed in this article are solely those of the authors and do not necessarily represent those of their affiliated organizations, or those of the publisher, the editors and the reviewers. Any product that may be evaluated in this article, or claim that may be made by its manufacturer, is not guaranteed or endorsed by the publisher.
Keywords: robotics, grasping, manipulation, robot grasp planning, robot learning
Citation: Morales A, León B, Chinellato E and Suárez R (2022) Editorial: Current Challenges and Future Developments in Robot Grasping. Front. Robot. AI 9:973208. doi: 10.3389/frobt.2022.973208
Received: 19 June 2022; Accepted: 21 June 2022;
Published: 11 July 2022.
Edited and reviewed by:
Kostas J. Kyriakopoulos, National Technical University of Athens, GreeceCopyright © 2022 Morales, León, Chinellato and Suárez. This is an open-access article distributed under the terms of the Creative Commons Attribution License (CC BY). The use, distribution or reproduction in other forums is permitted, provided the original author(s) and the copyright owner(s) are credited and that the original publication in this journal is cited, in accordance with accepted academic practice. No use, distribution or reproduction is permitted which does not comply with these terms.
*Correspondence: Antonio Morales, bW9yYWxlc0B1amkuZXM=
Disclaimer: All claims expressed in this article are solely those of the authors and do not necessarily represent those of their affiliated organizations, or those of the publisher, the editors and the reviewers. Any product that may be evaluated in this article or claim that may be made by its manufacturer is not guaranteed or endorsed by the publisher.
Research integrity at Frontiers
Learn more about the work of our research integrity team to safeguard the quality of each article we publish.