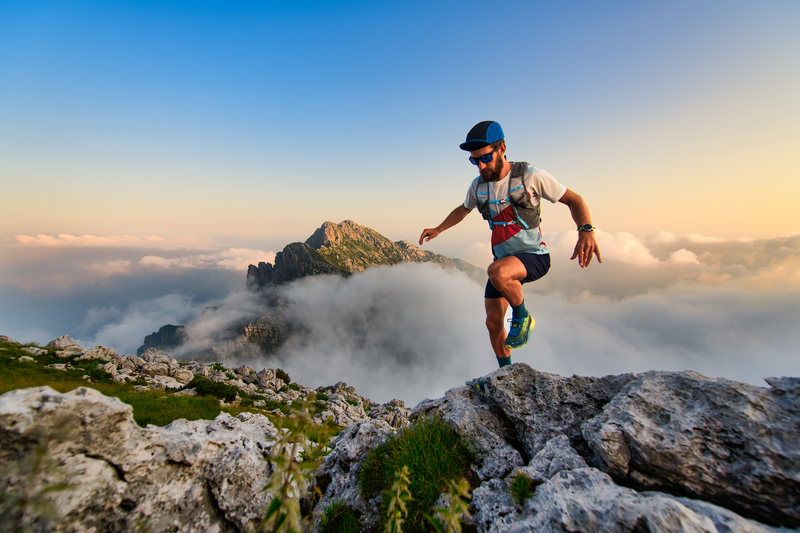
95% of researchers rate our articles as excellent or good
Learn more about the work of our research integrity team to safeguard the quality of each article we publish.
Find out more
EDITORIAL article
Front. Robot. AI , 22 June 2022
Sec. Biomedical Robotics
Volume 9 - 2022 | https://doi.org/10.3389/frobt.2022.920655
This article is part of the Research Topic Next Generation User-Adaptive Wearable Robots View all 8 articles
Editorial on the Research Topic
Next Generation User-Adaptive Wearable Robots
Wearable robots, including powered exoskeletons and robotic prostheses, have created new possibilities for mobility augmentation and restoration among individuals with a variety of movement disorders, including spinal cord injury, stroke, amputation, and other neurological conditions (Esquenazi et al., 2017; Rodríguez-Fernández et al., 2021). Exoskeleton technology has progressed to create utility for unimpaired individuals by supporting load carriage, reducing joint loading or improving metabolic efficiency (Sawicki et al., 2020). Despite this progress, exoskeleton deployment in real-world, community environments remains limited. While there are multiple barriers to ubiquitous exoskeleton use, a key lynchpin is development of robust control systems that adapt to user intent, support the variety of mobility tasks that may be encountered, and account for variation in the user’s voluntary effort across such tasks.
This Research Topic highlights recent advances toward the development of such adaptive control systems for wearable robotics. The Research Topic presents three manuscripts detailing design and evaluation of hybrid exoskeletons combining functional electrical stimulation (FES) with powered exoskeletons, one that was evaluated in individuals with spinal cord injury (Nandor et al.) and two that were validated in able-bodied individuals (Molazadeh et al.; Chang et al.). One study presents a pediatric exoskeleton that provides adaptive assistance to knee extension to alleviate crouch and its evaluation in a child with cerebral palsy (Chen et al.). Two manuscripts present novel controllers which leverage reinforcement learning and their evaluation in simulation: one for assisting squatting motion (Luo et al.) and one for bipedal exoskeleton walking in three dimensions (Liu et al.). The final manuscript evaluates the fusion of surface electromyography (EMG) and muscle sonography to estimate limb movement in a variety of locomotor tasks (Rabe and Fey).
Hybrid exoskeletons are an attractive option for use in individuals with paralysis because they can potentially provide the therapeutic benefits of muscle activation and the enhanced mobility from electromechanical actuators. These systems fit particularly well with the focus of this research topic; FES-induced muscle fatigue results in unpredictable torque output from stimulated muscles and thereby necessitates an adaptive approach for supplementing motion with actuators. In this Research Topic, Nandor et al. introduce a novel Motor Assisted Hybrid Neuroprosthesis (MAHNP) with actuated hip and knee joints and a distributed control architecture that integrates the exoskeleton with customized FES systems. A supervisory gait event detector split the gait cycle into four discrete states. The hip and/or knee motors could be activated with bursts of torque to assist the stimulation-driven limb motion. The system was evaluated in two participants with SCI, each with different implanted stimulation systems. Each showed improvements in step length and gait speed when walking with exoskeleton assistance, demonstrating the utility of this muscle first hybrid approach. Molazadeh et al. introduced a robust adaptive strategy for shared control in a hybrid exoskeleton. The strategy used a neural network-based iterative learning controller combined with model predictive control to adaptively distribute torque generation between surface FES and motors at the knee. When evaluated in a sit-to-stand task, this shared control paradigm improved joint trajectory tracking at the hip and knee across multiple task repetitions, demonstrating the utility of the iterative learning process in allocating torque generation between FES and motors. The controller was stable and robust despite disturbance uncertainty, suggesting its future utility across a variety of movements. In yet another approach, Chang et al. implemented a combination of different controllers for cooperative use of FES and electric motors at the hip and knee for trajectory tracking during treadmill walking. The novelty here was combining trajectory tracking via FES and stiffness tracking using a cable-driven exoskeleton at the knee, while only motorized assistance was used at the hip. The controller was evaluated in three able-bodied individuals and showed capability to track target trajectories at both low and high speeds.
Chen et al. present a robotic exoskeleton designed for ambulatory children with crouch gait, another pathology that fits well with the aims of this topic. Rather than replacing lost function, the goal of this exoskeleton was assistance and rehabilitation. They introduced an adaptive controller that provided knee extensor torque proportional to the user’s instantaneous volitional effort. Their evaluation in one child with cerebral palsy showed the adaptive control improved crouch with less reduction in gait speed compared to non-adaptive assistance.
Luo et al. introduce design and numerical simulation of their reinforcement-based learning controller for cooperative assistance during a squatting motion. They use a multi-layer perceptron neural network, trained on a reference squatting motion with and without perturbations. The neural network outputs target joint positions, which are tracked by the actuators at the hip, knee and ankle using a proportional derivative controller. The controller was verified in multiple simulations that included perturbations and human-exoskeleton interactions, suggesting this approach may be extendable to other movements. However, they offer a word of caution that their modeling only included a passive muscle response. Thus, future work should focus on verification in users who retain some ability to generate movements themselves. Liu et al. paired reinforcement learning with two different artificial neural network controllers and evaluated them in a three-dimensional bipedal model of a human and exoskeleton. Their results showed a global neural network controller that encompasses the entire walking motion, versus local controllers for stance and swing, provided the most stable control. A key implication is their architecture doesn’t require a detailed dynamic model of the exoskeleton user, potentially expediting the transition of this controller into practice.
Rabe and Fey present a novel method of fusing surface EMG with sonography to continuously predict hip, knee, and ankle joint angles and velocities. Their results show that this fusion improves tracking during various walking tasks, including level ground, stair, and ramp ambulation. This approach holds promise for future robotic controllers as it enables movement tracking without instrumenting individual joints.
In summary, we believe this research topic contributes to the existing literature regarding adaptive control methods for wearable robotics and will pave the way for future advancements toward real-world functionality. Continued advancements in deep neural networks and machine learning (Laschowski et al., 2021), switched control theory (Sheng et al., 2021), and bioinspired control algorithms (Alibeji et al., 2018) have potential to address the challenges remaining for intent-detection, environment classification and exoskeleton control during various locomotion modes. Although the focus of this editorial was on mobility, the approaches introduced here may be applicable in upper extremity (UE) exoskeletons as well. Similarly, previously developed methods for robust and adaptive control of UE exoskeletons (Kiguchi et al., 2005; Brahmi et al., 2018) could be explored in lower limb exoskeletons. In addition, multi-modal approaches including those that leverage ultrasound imaging for intent prediction (Dhawan et al., 2019; Zhang et al., 2021) and muscle in the loop control of exoskeletons can increase adaptability during real-world use.
All authors listed have made a substantial, direct, and intellectual contribution to the work and approved it for publication.
TB was supported by the Intramural Research Program of the NIH Clinical Center. NS was supported by NSF grant 1646009 and NSF CAREER award 2126570. SS was supported by NSF grant 1646204 and NIH grant U01EB027601. HS was supported by NSF CAREER award CMMI 1944655 and NIDILRR grant 90DPGE0011.
The authors declare that the research was conducted in the absence of any commercial or financial relationships that could be construed as a potential conflict of interest.
All claims expressed in this article are solely those of the authors and do not necessarily represent those of their affiliated organizations, or those of the publisher, the editors and the reviewers. Any product that may be evaluated in this article, or claim that may be made by its manufacturer, is not guaranteed or endorsed by the publisher.
Alibeji, N. A., Molazadeh, V., Moore-Clingenpeel, F., and Sharma, N. (2018). A Muscle Synergy-Inspired Control Design to Coordinate Functional Electrical Stimulation and a Powered Exoskeleton: Artificial Generation of Synergies to Reduce Input Dimensionality. IEEE Control Syst. 38 (6), 35–60. doi:10.1109/mcs.2018.2866603
Brahmi, B., Saad, M., Ochoa-Luna, C., Rahman, M. H., and Brahmi, A. (2018). Adaptive Tracking Control of an Exoskeleton Robot with Uncertain Dynamics Based on Estimated Time-Delay Control. IEEE/ASME Trans. Mechatron. 23 (2), 575–585. doi:10.1109/tmech.2018.2808235
Dhawan, A. S., Mukherjee, B., Patwardhan, S., Akhlaghi, N., Diao, G., Levay, G., et al. (2019). Proprioceptive Sonomyographic Control: A Novel Method for Intuitive and Proportional Control of Multiple Degrees-Of-Freedom for Individuals with Upper Extremity Limb Loss. Sci. Rep. 9 (1), 9499. doi:10.1038/s41598-019-45459-7
Esquenazi, A., Talaty, M., and Jayaraman, A. (2017). Powered Exoskeletons for Walking Assistance in Persons with Central Nervous System Injuries: A Narrative Review. PM&R 9 (1), 46–62. doi:10.1016/j.pmrj.2016.07.534
Kiguchi, K., Rahman, M. H., and Yamaguchi, T. (2005). “Adaptation Strategy for the 3DOF Exoskeleton for Upper-Limb Motion Assist,” in Proceedings of the 2005 IEEE International Conference on Robotics and Automation (Barcelona, Spain: IEEE), 2296–2301.
Laschowski, B., McNally, W., Wong, A., and McPhee, J. (2021). Environment Classification for Robotic Leg Prostheses and Exoskeletons Using Deep Convolutional Neural Networks. Front. Neurorobot. 15, 730965. doi:10.3389/fnbot.2021.730965
Rodríguez-Fernández, A., Lobo-Prat, J., and Font-Llagunes, J. M. (2021). Systematic Review on Wearable Lower-Limb Exoskeletons for Gait Training in Neuromuscular Impairments. J. NeuroEngineering Rehabil. 18 (1), 22. doi:10.1186/s12984-021-00815-5
Sawicki, G. S., Beck, O. N., Kang, I., and Young, A. J. (2020). The Exoskeleton Expansion: Improving Walking and Running Economy. J. NeuroEngineering Rehabil. 17 (1), 25. doi:10.1186/s12984-020-00663-9
Sheng, Z., Sun, Z., Molazadeh, V., and Sharma, N. (2021). Switched Control of an N-Degree-Of-Freedom Input Delayed Wearable Robotic System. Automatica 125, 109455. doi:10.1016/j.automatica.2020.109455
Keywords: exoskeleton, wearable robot, rehabilitation, human-in-the loop, functional electric stimulation
Citation: Bulea TC, Sharma N, Sikdar S and Su H (2022) Editorial: Next Generation User-Adaptive Wearable Robots. Front. Robot. AI 9:920655. doi: 10.3389/frobt.2022.920655
Received: 14 April 2022; Accepted: 26 May 2022;
Published: 22 June 2022.
Edited and reviewed by:
Mohammad Habibur Rahman, University of Wisconsin–Milwaukee, United StatesCopyright © 2022 Bulea, Sharma, Sikdar and Su. This is an open-access article distributed under the terms of the Creative Commons Attribution License (CC BY). The use, distribution or reproduction in other forums is permitted, provided the original author(s) and the copyright owner(s) are credited and that the original publication in this journal is cited, in accordance with accepted academic practice. No use, distribution or reproduction is permitted which does not comply with these terms.
*Correspondence: Thomas C. Bulea, dGhvbWFzLmJ1bGVhQG5paC5nb3Y=
Disclaimer: All claims expressed in this article are solely those of the authors and do not necessarily represent those of their affiliated organizations, or those of the publisher, the editors and the reviewers. Any product that may be evaluated in this article or claim that may be made by its manufacturer is not guaranteed or endorsed by the publisher.
Research integrity at Frontiers
Learn more about the work of our research integrity team to safeguard the quality of each article we publish.