- 1Social Robotics Lab, Department of Computer Science, Yale University, New Haven, CT, United States
- 2Ball Aerospace, Boston, MA, United States
- 3Yale Center for Emotional Intelligence, Department of Psychology, Yale University, New Haven, CT, United States
- 4Air Force Research Laboratory, Dayton, OH, United States
- 5iThrive, Boston, MA, United States
Role-play scenarios have been considered a successful learning space for children to develop their social and emotional abilities. In this paper, we investigate whether socially assistive robots in role-playing settings are as effective with small groups of children as they are with a single child and whether individual factors such as gender, grade level (first vs. second), perception of the robots (peer vs. adult), and empathy level (low vs. high) play a role in these two interaction contexts. We conducted a three-week repeated exposure experiment where 40 children interacted with socially assistive robotic characters that acted out interactive stories around words that contribute to expanding children’s emotional vocabulary. Our results showed that although participants who interacted alone with the robots recalled the stories better than participants in the group condition, no significant differences were found in children’s emotional interpretation of the narratives. With regard to individual differences, we found that a single child setting appeared more appropriate to first graders than a group setting, empathy level is an important predictor for emotional understanding of the narratives, and children’s performance varies depending on their perception of the robots (peer vs. adult) in the two conditions.
1. Introduction
The typical use case for socially assistive robotics applications involves one robot and one user (Tapus et al., 2007). As assistive technology becomes more sophisticated, and as robots are being used more broadly in interventions, there arises a need to explore other types of interactions. Contrasting the typical “one robot to one user” and “one robot to many users” situations, there are cases where it is desirable to have multiple robots interacting with one user or multiple users. As an example, consider the case of role-playing activities in emotionally charged domains (e.g., bullying prevention, domestic violence, or hostage scenarios). In these cases, taking an active role in the interaction may bring about undesirable consequences, while observing the interaction might serve as a learning experience. Here, robots offer an inexpensive alternative to human actors, displaying controlled behavior across interventions with different trainees.
Our goal is to use socially assistive robots to help children build their emotional intelligence skills through interactive storytelling activities. Storytelling is an effective tool for creating a memorable and creative learning space where children can develop cognitive skills (e.g., structured oral summaries, listening, and verbal aptitude), while also bolstering social-emotional abilities (e.g., perspective-taking, mental state inference). Narrative recall, for example, prompts children to logically reconstruct a series of events, while explaining behavior and attributing mental and emotional states to story characters (Capps et al., 2000; John et al., 2003; McCabe et al., 2008). As this is a novel research direction, several questions can be posed. What is the effect of having multiple robots in the scene or, more importantly, what is the optimal context of interaction for these interventions? Should the interaction context focus on groups of children (as in traditional role-playing activities) or should we aim for single child interactions, following the current trend in socially assistive robotics?
In our previous work, we began addressing the question of whether socially assistive robots are as effective with small groups of children as they are with a single child (Leite et al., 2015a). While we found that the interaction context can impact children’s learning, we anticipate that it might not be the only contributing factor. Previous research exploring the effects of single versus small group learning with technology points out that the two different contexts can be affected by learner characteristics such as gender, grade level, and ability level (Lou et al., 2001). A recent Human–Robot Interaction (HRI) study suggests that children behave differently when interacting alone or in dyads with a social robot (Baxter et al., 2013). However, it remains unknown whether interaction context and individual factors impact the effectiveness of robot interventions in terms of how much users can learn or recall from the interaction. In this paper, we extend previous work by investigating whether individual human factors play a role in different interaction contexts (single vs. group). For example, do individual differences such as gender or empathy level influence the optimal interaction context for socially assistive robotics interventions?
To address these questions, we developed an interactive narrative scenario where a pair of robotic characters played out stories centered around words that contribute to expanding children’s emotional vocabulary (Rivers et al., 2013). To evaluate the effects of interaction context (single vs. group), we conducted a three-week repeated exposure study where children interacted with the robots either alone or in small groups, and then were individually asked questions on the interaction they had just witnessed. We analyzed interview responses in order to measure participants’ story recall and emotional understanding abilities and looked into individual differences that might affect these measures. Our results show that although children interacting alone with the robots were able to recall the narrative more accurately, no significant differences were found in the understanding of the emotional context of the stories. Furthermore, we found that individual differences such as grade, empathy level, and perception of the robots are important predictors of the optimal interaction for students. We discuss these implications for the future design of robot technology in learning environments.
2. Background
2.1. Learning Alone or in Small Groups
Educational research highlights the benefits of learning in small groups as compared to learning alone (Pai et al., 2015). These findings also apply to learning activities supported by computers (Dillenbourg, 1999; Lou et al., 2001). It has long been acknowledged that groups outperform individuals in a variety of learning tasks such as concept attainment, creativity, and problem solving (Hill, 1982). More recently, Schultze et al. (2012) conducted a controlled experiment to show that groups perform better than individuals in quantitative judgments. Interestingly, the authors attribute this finding to within-group interactions instead of weighting the individual judgment of each group member. A situation in which “two or more people learn or attempt to learn something together” is often referred to as collaborative learning (Dillenbourg, 1999).
It is important to note, however, that most of these findings were obtained with adults. Additionally, as previously stated, interaction context (i.e., whether students are alone or in small groups) is not the only factor that influences learning. In a meta-review focusing on computer-supported learning, Lou et al. (2001) enumerated several learning characteristics that can affect learning as much as interaction context. Factors such as ability level, gender, grade, or past experience with computers are among the most common individual differences that affect learning.
2.2. Individual Differences in Narrative Recall and Understanding
Research has shown that a child’s ability to reconstruct a cohesive and nuanced narrative develops with age (Griffith et al., 1986; Crais and Lorch, 1994; Bliss et al., 1998; John et al., 2003). While three-year-olds tend to focus on an isolated event within a narrative, by the time a child is five, the capacity to create a more structured narrative with a logical sequence of story events is already developed (John et al., 2003). Among seven to eleven-year-olds, Griffith et al. (1986) found more story inaccuracies in older children’s retellings as their narratives became longer. A central notion of the current study is to increase emotional understanding by allowing children to see the impact of their decisions play out in the story. As such, knowing at what age children begin to develop the capacity to attribute meaning to characters’ behaviors is important. While the foundations of a story – story setting, opening scene, and story conclusion – are typically included in narratives of children aged four to six, the presence of a character’s thoughts and intentions within a narrative takes longer to develop (Morrow, 1985). Identifying more overt story structure elements may be easier for children than attributing meaning, intentions, and emotions to a character’s behavior (Renz et al., 2003). A study carried out by Camras and Allison (1985) found that when emotion-laden stories are given to children from kindergarten to second grade, the accuracy of children’s emotion labeling improved with age.
Several authors have found gender differences in narrative recall and understanding. For example, research shows that females are more verbal than males (Smedler and Törestad, 1996; Buckner and Fivush, 1998; Crow et al., 1998) and excel on verbal tasks (Bolla-Wilson and Bleecker, 1986; Capitani et al., 1998), while males are more successful at spatial tasks (Maccoby and Jacklin, 1974; Linn and Petersen, 1985; Iaria et al., 2003). However, Andreano and Cahill (2009) found that gender differences are more nuanced and extensive, with females outperforming males in spatial, autobiographical abilities, and general episodic memory. In a test of verbal learning among children aged 5–16, females outperformed males in long-term memory recall and delayed recognition, while males produced more intrusion errors (Kramer et al., 1997). Yet, Maccoby and Jacklin (1974) concluded that “the two genders show a remarkable degree of similarity in the basic intellectual processes of perception, learning, and memory.” Additionally, females tend to generate more accurate (Pohl et al., 2005), detailed (Ross and Holmberg, 1992), and exhaustive narratives that take social context and emotions into account (Buckner and Fivush, 1998; John et al., 2003). Females are also generally thought to be more emotive both verbally (Smedler and Törestad, 1996) and non-verbally (Briton and Hall, 1995). Gender differences in emotional dialog and understanding are broadly attributed to the view that, beginning in early childhood, girls are socialized to be more emotionally attuned and, therefore, more skilled at perspective-taking (Hoffman, 1977; Greif et al., 1981; Dunn et al., 1987).
2.3. Individual Differences in Emotional Intelligence
Emotions are functional and impact our attention, memory, and learning (Rivers et al., 2013). Emotional intelligence (EI) is defined as “the ability to monitor one’s own and other’s feelings and emotions, to discriminate among them and to use this information to guide one’s thinking and action” (Salovey and Mayer, 1990). Previous research has determined that a child’s emotional understanding advances with age (Pons et al., 2004; Harris, 2008). The ability to recognize basic emotions and understand that emotions is affected by external causes, which is generally established by the age of 3–4 (Yuill, 1984; Denham, 1986). Between 3 and 6 years, children begin to understand how emotions are impacted by desires, beliefs, and time (Harris, 1983; Yuill, 1984), while children aged 6–7 begin to explore strategies for emotion regulation (Harris, 1989).
In terms of gender, Petrides and Furnham (2000) concluded that females scored higher than males on the “social skills” factor of measured trait EI, and a cross-cultural study carried out by Collis (1996) found that females had higher empathy than males at the first-grade level. These results are reinforced with findings from a meta-analysis of 16 studies in which females scored higher on self-reported empathy (Eisenberg and Lennon, 1983).
3. Related Work
In this section, we review previous research in the three main research thrusts that inform this work: robots for education, multiparty interactions, and individual differences in HRI.
3.1. Robots As Educational Tools
Kim and Baylor (2006) posit that the use of non-human pedagogical agents as learning companions creates the best possible environment for learning for a child. Virtual agents are designed to provide the user with the most interactive experience possible; however, research by Bainbridge et al. (2011) indicated that physical presence matters in addition to embodiment, with participants in a task rating an overall more positive interaction when the robot was physically embodied rather than virtually embodied. Furthermore, Leyzberg et al. (2012) found that the students who showed the greatest measurable learning gains in a cognitive skill learning task were those who interacted with a physically embodied robot tutor (a Keepon robot), as compared to a video-represented robot and a disembodied voice.
Research by Mercer (1996) supports talk as a social mode of thinking, with talk in the interaction between learners beneficial to educational activities. However, Mercer identifies the need for focused direction from a teaching figure for the interaction to be as effective as possible. In line with these findings, Saerbeck et al. (2010) showed the positive effects of social robots in language learning, especially when the robot was programmed with appropriate socially supportive behaviors.
A great deal of research has been conducted into the use of artificial characters in the context of educational interactive storytelling with children. Embodied conversational agents are structured using a foundation of human-human conversation, creating agents that appear on a screen and interact with a human user (Cassell, 2000). Interactive narratives, where users can influence the storyline through actions and interact with the characters, result in engaging experiences (Schoenau-Fog, 2011) and increase a user’s desire to keep interacting with the system (Kelleher et al., 2007; Hoffman et al., 2008). FearNot is a virtual simulation with different bullying episodes where a child can take an active role in the story by advising the victim on possible coping strategies to handle the bullying situation. An extensive evaluation of this software in schools showed promising results on the use of such tools in bullying prevention (Vannini et al., 2011). Although some authors have explored the idea of robots as actors (Bruce et al., 2000; Breazeal et al., 2003; Hoffman et al., 2008; Lu and Smart, 2011), most of the interactive storytelling applications so far are designed for virtual environments.
3.2. Multiparty Interactions with Robots
Research on design and evaluation of robots that interact with groups of users has become very prominent in the past few years in several application domains such as education (Kanda et al., 2007; Al Moubayed et al., 2012; Foster et al., 2012; Gomez et al., 2012; Johansson et al., 2013; Bohus et al., 2014; Pereira et al., 2014). Despite this trend, few authors investigated differences between one single user and a group of users interacting with a robot in the same setting.
One of the exceptions is Baxter et al. (2013), who reported a preliminary analysis that consisted of a single child or a pair of children interacting with a robot in a sorting game. Their observations indicate differences between the two conditions: when alone with the robot, children seem to treat it more as a social entity (e.g., engage in turn-taking and shared gaze with the robot), while these behaviors are less common when another peer is in the room.
Shahid et al. (2014) conducted a cross-cultural examination of variation between interactions in children who either played a game alone, with a robot, or with another child. They found that children both enjoyed playing more and were more expressive when they played with the robot, as compared to when they played alone; unsurprisingly, children who played with a friend showed the highest levels of enjoyment of all groups.
With this previous research serving as foundation, one of the goals of our work is to investigate whether interactions with robots in a group setting could benefit information retainment and emotional understanding. However, in addition to interaction context (single vs. group), there might be other individual factors contributing to these differences.
3.3. Individual Differences in Human–Robot Interaction
One of the underlying aims of studying individual differences in HRI is personalization. Understanding how different user groups perceive and react to robots, and adapting the robot’s behavior accordingly, can result in more effective and natural interactions. Andrist et al. (2015) provided one of the first empirical validations on the positive effects of personalization. In a controlled study where a social robot matched each participant’s extroversion personality dimension through gaze, they showed that introverted subjects had a marginally significant preference for the robot displaying introverted behaviors and that both introverts and extroverts showed higher compliance when interacting with the robot that matched their personality dimension.
Most of the research reporting individual differences in HRI so far has mainly focused on gender (Mutlu et al., 2006; Nomura et al., 2008; Schermerhorn et al., 2008; MacDorman and Entezari, 2015) and certain personality traits such as extroversion and agreeableness (Walters et al., 2005; Syrdal et al., 2007; Takayama and Pantofaru, 2009; Andrist et al., 2015), but there are also studies exploring other factors such as pet ownership (Takayama and Pantofaru, 2009) and perception of robots (Nomura et al., 2008; Schermerhorn et al., 2008; Mumm and Mutlu, 2011; MacDorman and Entezari, 2015).
Gender seems to play an important role in individual’s perceptions and attitudes toward robots. In a study where a storytelling robot recited a fairy tale to two participants, Mutlu et al. (2006) manipulated the robot’s gaze behavior by having the robot look at one of the participants 80% of the time. This manipulation had a significant interaction effect on gender, with males who were looked at more rating the robot more positively, and females who were looked at less rating the robot more positively. More recently, the same authors investigated gender differences (among other factors) in a scenario where the robot was able to monitor participants’ attention using brain electrophysiology and adapt its behavior accordingly (Szafir and Mutlu, 2012). Females interacting with the adaptive robot gave higher ratings in rapport toward the robot and self-motivation, while no significant differences were found for males on the same measures. In the studies conducted to validate the Negative Attitudes Toward Robots Scale (NARS) and Robot Anxiety Scale (RAS), Nomura et al. (2008) found several gender effects. For instance, males with higher NARS and RAS scores talked less to the robot and avoided touching it. Schermerhorn et al. (2008) also reported gender effects on people’s ratings of social presence toward robots, with males perceiving a robot as more human-like and females perceiving it as more machine-like and less socially desirable. These findings are in line with results obtained by MacDorman and Entezari (2015) in their investigation into whether individual differences can predict sensitivity to the uncanny valley. They found significant correlations between gender and android eerie ratings; females in this study perceived android robots as more eerie than males.
Individual differences have been explored as a way to better understand proxemic preferences between people and robots. Walters et al. (2005) investigated the effects of people’s personality traits on their comfortable social distances while approaching a robot. Results showed that more proactive people felt more comfortable standing further away from the robot. In a follow-up study (Syrdal et al., 2007), researchers from the same group found that people with high extroversion and low conscientiousness scores let a robot get closer when they were in control of the robot, as opposed to when they believed the robot to be in control of itself. Takayama and Pantofaru (2009) confirmed the hypothesis that pet owners felt more comfortable with being closer to robots, a result that also held true for people with past experience with robots. Additionally, they found that proxemic comfort levels were related to the agreeableness personality trait, with more agreeable people experiencing higher levels of comfort closer to a robot than participants rated as less agreeable in the personality questionnaire. More recently, Mumm and Mutlu (2011) reported significant differences in the effects of gender on proxemics, with males distancing themselves significantly further than females. Another interesting factor that played an effect in this study was robot likability: people who reported disliking the robot positioned themselves further away in a condition where the robot tried to establish mutual eye gaze with the subjects.
4. Research Questions and Hypotheses
The main goal of the research presented in this paper is to investigate whether the social context of the interaction, i.e., children interacting with robots alone or in a small group, has an impact on information recall and understanding of the learning content. Our research goals can be translated into two main research questions:
RQ1 How does interaction context impact children’s information recall?
RQ1 How does interaction context impact children’s emotional understanding and vocabulary?
As previously outlined, socially assistive robotic applications are typically one-on-one, but educational research suggests that learning gains may increase in a group setting (Hill, 1982; Pai et al., 2015). To further understand these questions, we explored individual factors that may impact how children perform in learning environments and/or how users perceive the robots. Considering previous findings on individual differences presented in sections 2 and 3, as well as our particular application domain, we took into account gender, grade level (first vs. second), perception of the robot (peer vs. adult), and empathy level (low vs. high). To further explore the human individual factors that influence recall and understanding in single versus group interactions, we outlined the following hypotheses:
H1 Second graders will achieve higher performance than first graders in narrative recall and emotional understanding.
In comparison to first graders, second graders are more developmentally advanced both cognitively and emotionally, so we hypothesize that second-grade students will perform better since narrative recall abilities and emotional understanding tend to develop with age (Morrow, 1985; Crais and Lorch, 1994; Bliss et al., 1998; John et al., 2003). Although a number of previous studies report age instead of grade, we determined that grade is more fitting for our study as the social and emotional learning curriculum (see section 5.2) employed in the school where our study was conducted is grade-dependent. For this reason, we predict that grade level could be a better explanatory factor than age.
H2 Females will achieve higher performance than males in narrative recall and emotional understanding.
Previous research has shown that females tend to tell more accurate (Pohl et al., 2005) and detailed (Ross and Holmberg, 1992) narratives, while accounting for the emotions of the narrative characters more often (Buckner and Fivush, 1998; John et al., 2003). Additionally, several authors found that females scored higher in emotional intelligence tests (Collis, 1996; Petrides and Furnham, 2000). For these reasons, we hypothesize that females will perform better than their male counterparts.
H3 Higher empathy students will achieve higher scores in emotional understanding.
Because we anticipate a positive correlation between high empathy and high emotional intelligence (Salovey and Mayer, 1990), we hypothesize that individuals with higher empathy will be better at emotional understanding.
H4 Children’s perceived role of the robot will affect their narrative recall and emotional understanding abilities.
Considering the extensive HRI literature showing that perception of robots changes how individuals perform and interact with them (Nomura et al., 2008; Mumm and Mutlu, 2011; MacDorman and Entezari, 2015), we expect perception of robots to affect our main measures. As most robots used in educational domains are viewed by students as either peers or adult tutors (Mubin et al., 2013), we will gage perception within these two opposite roles.
5. Affective Narratives with Robotic Characters
We developed an interactive narrative system such that any number of robotic characters can act out stories defined in a script. This system prompts children to control the actions of one of the robots at specific moments, allowing the child to see the impact of their decision on the course of the story. By exploring all the different options in these interactive scenarios, children have the opportunity to see how the effects of their decisions play out before them, without the cost of first having to make these decisions in the real world. This section describes the architecture of this system and introduces RULER, a validated framework for promoting emotional literacy that inspired the interactive stories developed for this system.
5.1. System Architecture
The central component of the narrative system is the story manager, which interprets the story scripts and communicates with the robot controller modules and the tablet (see diagram in Figure 1). The scripts contain, in a representation that can be interpreted by the story manager, every possible scene episode. A scene contains the dialog lines of each robot and a list of the next scene options that can be selected by the user. Each dialog line contains an identifier of the robot playing that line, the path to a sound file, and a descriptor of a non-verbal behavior for the robot to display while “saying” that line (e.g., happy, bouncing). When the robots finish playing out a scene, the next story options are presented on the tablet as text with an accompanying illustration. When the user selects a new story option on the tablet, the story manager loads that scene and begins sending commands to the robots based on the scene dialog lines.
The system was implemented on Robot Operating System (ROS) (Quigley et al., 2009). The story manager is a ROS node that publishes messages subscribed by the active robot controller nodes. Each robot controller node is instantiated with a robotID parameter, so that each node can ignore the messages directed to the other characters in the scene. The tablet communicates with the story manager module using a TCP socket connection over Wi-Fi.
The robot platforms used in this implementation were two MyKeepon robots (see Figure 2) with programmable servos controlled by an Arduino board (Admoni et al., 2015). MyKeepon is a 32 cm tall, snowman-like robot with three dots representing eyes and a nose. Despite their minimal appearance, these robots have been shown to elicit social responses from children (Kozima et al., 2009). Each robot has four degrees of freedom: it can pan to the sides, roll to the sides, tilt forward and backward, and bop up and down. To complement the prerecorded utterances, we developed several non-verbal behaviors such as idling, talking, and bouncing. All the story authoring was done in the script files, except the robot animations and tablet artwork. In addition to increased modularity, this design choice allows non-expert users (e.g., teachers) to develop new content for the system.
5.2. The RULER Framework
RULER is a validated framework rooted in emotional intelligence theory (Salovey and Mayer, 1990) and research on emotional development (Denham, 1998) that is designed to promote and teach emotional intelligence skills. Through a comprehensive approach that is integrated into existing academic curriculum, RULER focuses on skill-building lessons and activities around Recognizing, Understanding, Labeling, Expressing, and Regulating emotions in socially appropriate ways (Rivers et al., 2013). Understanding the significance of emotional states guides attention, decision-making, and behavioral responses, and is necessary in order to navigate the social world (Lopes et al., 2005; Brackett et al., 2011).
This study employs components of RULER, including the Mood Meter, a tool that students and educators use as a way to identify and label their emotional state, and the Feeling Words Curriculum, a tool that centers on fostering an extensive feelings vocabulary that can be applied in students’ everyday lives. The story scripts are grounded in the Feeling Words Curriculum and are intended to encourage participants to choose the most appropriate story choice after considering the impact of each option. Our target age group was first to second graders (6–8 years old). Prior to beginning the study, we gathered feedback from elementary school teachers to ensure that the vocabulary and difficulty levels of story comprehension were age-appropriate. A summary of the scenes forming the scripts of each session is displayed in Table 1. All three stories followed the same structure: introduction scene, followed by three options. Each option impacted the story and the characters’ emotional state in different ways.
6. Experimental Method
In order to investigate the research questions and hypotheses outlined earlier, we conducted a user study using the system described in the previous section.
6.1. Participants
The participants in the study were first- and second-grade students from an elementary school where RULER had been implemented. A total of 46 participants were recruited in the school where the study was conducted, but six participants were excluded for various reasons (i.e., technical problems in collecting data or participants missing school). For this analysis, we considered a total of 40 children (22 females, 18 males) between the age of 6 and 8 years (mean (M) = 7.53, standard deviation (SD) = 0.51). Out of these 40 students, 21 were first graders and 19 were second graders.
Ethnicity, as reported by guardians, was as follows: 17.5% African American, 17.5% Caucasian, 25% Hispanic, and 27.5% reported more than one ethnicity (12.5% missing data). The annual income reported by guardians was as follows: 30% in $0–$20,000, 42.5% in $20,000–$50,000, and 10% in the $50,000–$100,000 range (17.5% missing data).
6.2. Design
We used a between-subjects design with participants randomly sorted into one of two conditions: single (one participant interacted alone with the robots) or group (three participants interacted with the robots at the same time). We studied groups of three children as three members is the smallest number of members considered to be a group (Moreland, 2010). Our main dependent metrics focused on participants’ recall abilities and emotional interpretation of the narrative choices.
Each participant or group of participants interacted with the robots three times, once per week. Participants in the group condition always interacted with the robots in the same groups. The design choice to use repeated interactions was not to measure learning gains over time, but to ensure that the results were not affected by a novelty effect that robots often evoke in children (Leite et al., 2013).
6.3. Procedure
The study was approved by an Institutional Review Board. Parental consent forms were distributed in classrooms that had agreed to participate in the study. Participants were randomly assigned to either the single condition (19 participants) or group condition (21 participants). Each session lasted approximately 30 min with each participant. The participant first interacted with the robots either alone or in a small group (approximately 15 min), and then was interviewed individually by an experimenter (approximately 15 min).
Participants were escorted from class by a guide who explained that they were going to interact with robots and then would be asked questions about the interaction. In addition to parental consent, the child was introduced to the experimenter and asked for verbal assent. The experimenter began by introducing the participants to Leo and Berry, the two main characters (MyKeepon robots) in the study. The first half of each session involved the participants interacting with the robots as the robots autonomously role-played a scenario centered around a RULER feeling word. After observing the scenario introduction, participants were presented with three different options. Participants were instructed to first select the option they thought was the best choice and were told they would then have the opportunity to choose the other two options. In the group condition, participants were asked to make a joint decision. The experimenter was present in the room at all times, but was outside participants’ line of sight.
After interacting with the robots, participants were interviewed by additional experimenters. The interviews had the same format for both conditions, which means that even participants in the group condition were interviewed individually. Interviews were conducted in nearby rooms. Experimenters followed a protocol that asked the same series of questions (one open-ended question, followed by two direct questions) for each of the four scenes (i.e., Introduction, Option A, Option B, and Option C) that comprised one session. The same three repeated questions were asked in the following order:
1. What happened after you chose <option>?
2. After you chose <option>, what color of the Mood Meter do you think <character> was in?
3. What word would you use to describe how <character> was feeling?
These questions were repeated for a total of 36 times (3 questions × 4 scenes per session × 3 sessions) over the course of the study. If a participant remained silent for more than 10 s after being asked a question, the experimenter asked, “Would you like me to repeat the question or would you like to move on?” The interviewer used small cards with artwork representing the different scene choices similar to the ones that appeared on the tablet near the robots. Interviews were audio-recorded and transcribed verbatim for coding.
All three sessions followed the same format (i.e., robot interaction followed by the series of interview questions). Additionally, in the second session we employed an adapted version of Bryant’s Empathy Scale (Bryant, 1982) to measure children’s empathy index, and in the third session we measured perception of the robots (peer vs. adult) using a scale specifically developed for this study. For the empathy assessment, the interviewer asked participants to sort each one of the scale items, printed on small cards, between two boxes, “me” or “not me.” A similar box task procedure was followed in the third session for collecting perception of the robots, but this time children were asked to sort cards with activities they would like to do with Leo and Berry.
6.4. Interview Coding
6.4.1. Word Count
The number of words uttered by each participant during the interview was counted using an automated script. Placeholders such as “umm” or “uhh” did not contribute toward word count. This metric was mainly used as a manipulation check for the other measures.
6.4.2. Story Recall
Responses to the open-ended question “What happened after you chose <option>?” were used to measure story recall through the Narrative Structure Score (NSS). Similar recall metrics have been previously used in HRI studies with adults (Szafir and Mutlu, 2012).
We followed the coding scheme used in previous research by McGuigan and Salmon (2006) and McCartney and Nelson (1981), in which participants’ verbal responses to open-ended questions were coded for the presence or absence of core characters (e.g., Leo, Berry) and core ideas (e.g., Leo does not know anyone, everyone is staring at Leo’s clothes). This score provides a snapshot of the participants’ “ability to logically recount the fundamental plot elements of the story.” For session S and participant i, NSS was computed using the following formula:
A perfect NSS of 1.0 would indicate that the participant mentioned all the core characters and main ideas in all four open-ended questions of that interview. The first mention of core characters and core ideas was given a point each, with additional mentions not counted. The sum of core characters and core ideas for each interview session were combined to generate the Narrative Structure Score. The average number of characters in each story was three (Leo, Berry, and Marlow or the teacher), while the number of core ideas varied depending on the difficulty of the story, ranging from an average of four in the easiest story to six in the hardest.
6.4.3. Emotional Understanding
The Emotional Understanding Score (EUS) represents participants’ ability to correctly recognize and label character’s emotional states, a fundamental skill of emotional intelligence (Brackett et al., 2011; Castillo et al., 2013). Responses to the two direct questions “After you chose <option>, what color of the Mood Meter do you think <character> was in?” and “What word would you use to describe how <character> was feeling?” were coded based on RULER concepts and combined to comprise EUS.
Appropriate responses for the first question were based on the Mood Meter colors and included Yellow (pleasant, high energy), Green (pleasant, low energy), Blue (unpleasant, low energy), or Red (unpleasant, high energy), depending on the emotional state of the robots at specific points in the role-play. Responses to the second direct question were based on the RULER Feeling Words Curriculum with potential appropriate responses being words such as excited (pleasant, high energy), calm (pleasant, low energy), upset (unpleasant, low energy), or angry (unpleasant, high energy), depending on which color quadrant the participant “plotted” the character. Since participants were recruited from schools implementing RULER, they use the Mood Meter daily and are accustomed to these types of questions. Most participants answered with one or two words when asked to describe the character’s feelings.
For the ColorScore, participants received +1 if the correct Mood Meter color was provided, and −1 if an incorrect color was given. In the FeelingWordScore, participants received +1 or −1 depending on whether the feeling word provided was appropriate or not. If participants provided additional appropriate or inappropriate feeling words, they were given +0.5 or −0.5 points for each, respectively. The total EUS was calculated using the following formula:
Higher EUS means that participants were able to more accurately identify the Mood Meter color and corresponding feeling word associated with the character’s emotional state. For each interview session, EUS scores for each scene were summed to calculate an aggregate EUS score.
6.5. Reliability between Coders
Two researchers independently coded the interview transcriptions from the three sessions according to the coding scheme described in the previous section. Both coders first coded the interviews from the excluded participants to become familiar with the coding scheme. Once agreement between coders was reached, coding began on the remaining data. Coding was completed for the 120 collected interviews (40 participants × 3 sessions), overlapping 25% (30 interviews) as a reliability check.
Reliability analysis between the two coders was performed using the Intraclass Correlation Coefficient test for absolute agreement using a two-way random model. All the coded variables for each interview session had high reliabilities. The lowest agreement was found in the number of correct feeling words (ICC(2, 1) = 0.85, p < 0.001), while the highest agreement was related to the total number of core characters mentioned by each child during one interview session (ICC(2, 1) = 0.94, p < 0.001). Given the high agreement between the two coders in the overlapping 30 interviews, data from one coder were randomly selected to be used for analyses.
6.6. Data Analysis Plan
We first calculated the story recall and emotional understanding metrics according to the formulas described above. Narrative Structure Score (NSS) and Emotional Understanding Score (EUS) were computed for each participant in every session (1, 2, and 3) and averaged across the three sessions. The empathy and perception of the robots indices were also calculated and a median split was used to categorize participants in two empathy levels (low vs. high) and perception of the robots (peer vs. adult). With regard to the empathy scale, 19 participants were classified in the low empathy category and 21 were classified in the high empathy category. Regarding perception of the robots, 19 children perceived the robots more as adults and 21 perceived the robots more as peers.
Our data analysis consisted of two main steps. First, we explored our main research questions (RQ1 and RQ2) about how interaction context (single vs. group) affects story recall and emotional understanding. We started by testing the difference between the two study conditions collapsed across the three sessions using between-subjects univariate analyses of variance (ANOVA). Next, ANOVA models were conducted with interaction context (single vs. group) as the between-subjects factor and session (1, 2, and 3) as the within-subjects factor. For all the dependent measures, we planned to test the single versus group differences in each session.
We then tested our formulated hypotheses to identify which individual factors (grade: first or second; gender: female or male; empathy: low or high; and perception of the robots: peer or adult) play a major role in our measures of interest. There are not enough children in our study for an analysis including all the individual factors in the same model. As a compromise, we explored the impact of interaction type (single vs. group) and one individual difference variable at a time on participants’ story recall and emotional understanding abilities. Separate planned comparisons from ANOVA models are reported below.
7. Results
The results concerning our research questions (RQ1 and RQ2) on the effects of interaction context are presented in the first subsection, and the results on the hypotheses about individual differences (H1 to H4) are reported in the second subsection.
Before analyzing our two main measures of interest, story recall and emotional understanding, we examined whether there were any differences between single and group conditions in the number of words spoken by the participants during the interview sessions. An ANOVA model was run with the number of words spoken as the dependent measure. No significant difference was found, which indicates that overall, there was no significant difference in word count between the two groups. The average number of words per interview was 124.82 (standard error (SE) = 16.01). This result is important because it serves as a manipulation check for other reported findings.
7.1. Effects of Interaction Context
7.1.1. Story Recall
We investigated the impact of interaction context (single vs. group) on participants’ story recall abilities (RQ1), measured by the Narrative Structure Score (NSS). An ANOVA model was run with NSS as the dependent measure. We found a significant effect of interaction context (collapsed across sessions), with students interacting alone with the robots achieving higher scores on narrative structure (M = 0.49, SE = 0.03) than the group condition (M = 0.38, SE = 0.02), F(1, 28) = 7.71, p = 0.01, and η2 = 0.22 (see Figure 3).
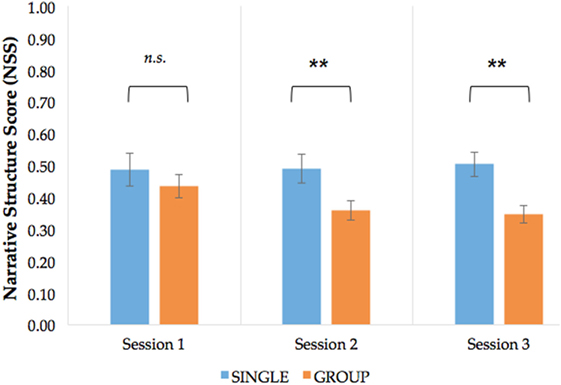
Figure 3. Average Narrative Structure Scores (NSS) for participants in each condition on every interaction session. **p < 0.01 and n.s. non-significant differences.
Planned comparisons were conducted to test the role of interaction context in each particular session. No significant differences were found for session 1. For session 2, students in the single condition (M = 0.49, SE = 0.05) had a higher NSS than the students in the group condition (M = 0.36, SE = 0.03), F(1, 36) = 7.35, p = 0.01, and η2 = 0.17. Similarly, for session 3, students in the single condition (M = 0.50, SE = 0.04) had a higher score than in the group condition (M = 0.35, SE = 0.03), F(1, 38) = 6.59, p = 0.01, and η2 = 0.15.
These findings suggest that overall, the narrative story-related recall rate was higher in the single versus the group interaction with the robots. In the easiest session (session 1), there was no effect on interaction context, but during the more difficult sessions (sessions 2 and 3), students were found to perform better in individual than group level interactions.
7.1.2. Emotional Understanding
To investigate our second research question (RQ2), we tested whether students’ emotional understanding differed in the single versus group condition. The ANOVA model with EUS as the dependent measure suggested that there was no effect of interaction context. The effect of session was significant F(2, 62) = 7.39, p = 0.001, and η2 = 0.19, which aligns with our expectation given that the three sessions had different levels of difficulty (see Figure 4). Planned comparisons also yielded no significant differences between single versus group in any of the three sessions. In sum, the degree of emotional understanding did not seem to be affected by the type of interaction in this setting, but varied across sessions with different levels of difficulty.
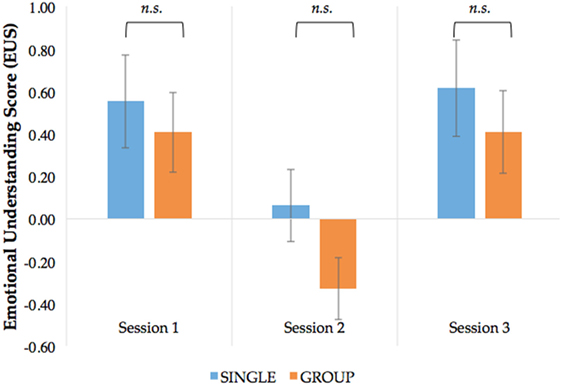
Figure 4. Average Emotional Understanding Scores (EUS) for participants in each condition for sessions 1 (easy), 2 (advanced), and 3 (medium). No significant differences (n.s.) were found between conditions.
7.2. Effects of Individual Differences
7.2.1. Grade
We tested how grade level (first vs. second) and interaction context influenced NSS (see Figure 5). Planned comparisons suggested that first graders scored higher in narrative structure when interacting alone with the robots than in the group condition, F(1, 36) = 4.44, p = 0.04, and η2 = 0.11. However, for second graders, this effect was non-significant.
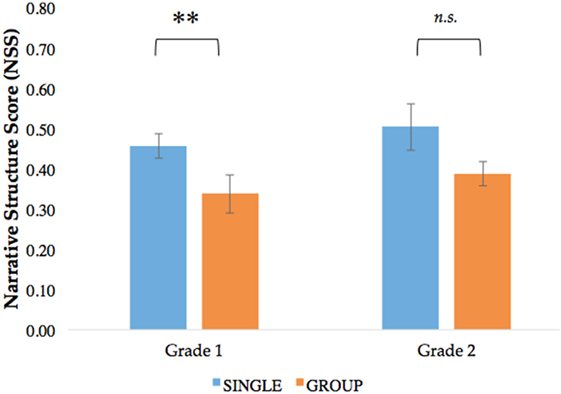
Figure 5. Average Narrative Structure Scores (NSS) for participants in each condition and grade. **p < 0.01.
A similar trend was found with EUS as an outcome, as depicted in Figure 6. Planned comparisons suggested that for the first graders, emotional understanding is higher in the single than in the group condition, F(1, 36) = 4.45, p = 0.04, and η2 = 0.11, but no significant differences were found for second graders. These results support Hypothesis 1, in which we predicted that second graders would have higher performance than first graders.
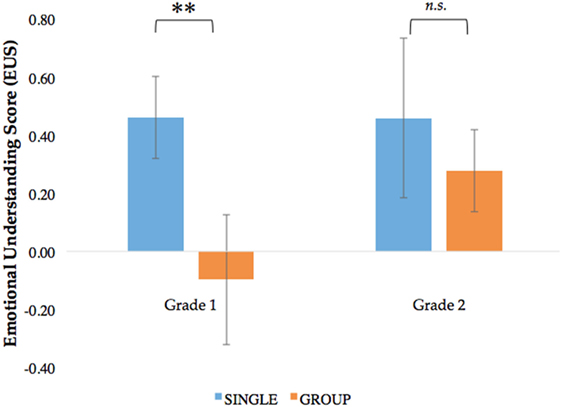
Figure 6. Average Emotional Understanding Scores (EUS) for participants in each condition and grade. **p < 0.01.
7.2.2. Gender
Hypothesis 2, which predicted that females would achieve higher performance scores, was not supported. We started by testing how gender differences and interaction context influenced NSS. Planned comparisons revealed only a marginal significance, with females in the single condition recalling more story events than in the group condition, F(1, 36) = 4.09, p = 0.05, and η2 = 0.10. No significant differences were found for male students between the single versus group conditions for this variable.
Similarly, no overall significant gender differences were found in emotional understanding (EUS) or with single vs. group interaction contexts.
7.2.3. Empathy
Hypothesis 3 predicted that higher empathy students would achieve higher scores in emotional understanding. Overall, high empathy students had significantly higher EUS than low empathy students, F(1, 36) = 4.58, p = 0.04, and η2 = 0.11, confirming our hypothesis (see Figure 7). Furthermore, in the single condition, high empathy students performed higher on emotional understanding than those with low empathy, F(1, 36) = 4.14, p = 0.049, and η2 = 0.10.
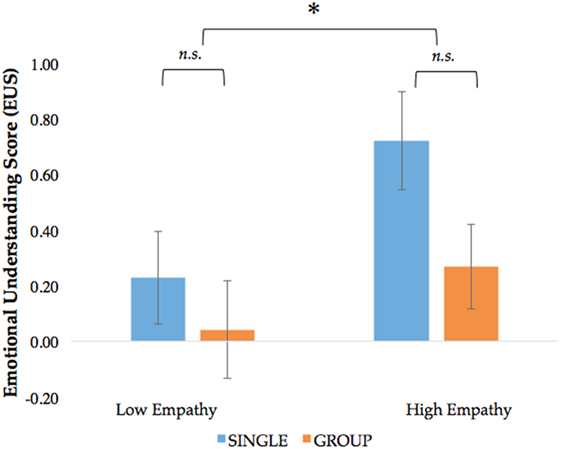
Figure 7. Average Emotional Understanding Scores (EUS) for participants in each condition and empathy level. *p < 0.05.
Planned comparisons suggested that among low empathy individuals, those in the single condition had a higher NSS than those in the group condition, F(1, 36) = 5.98, p = 0.02, and η2 = 0.14 (see Figure 8).
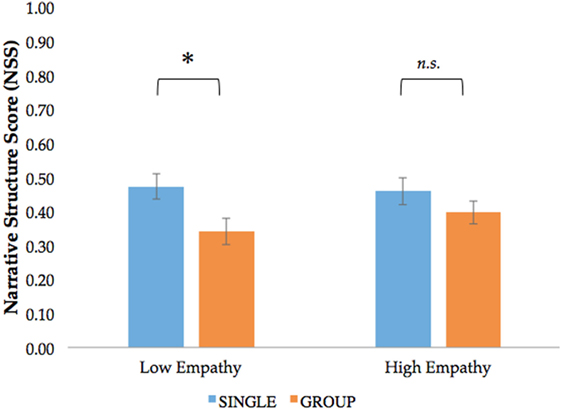
Figure 8. Average Narrative Structure Scores (NSS) for participants in each condition and empathy level. *p < 0.05.
7.2.4. Perception of the Robots
Finally, we investigated Hypothesis 4, in which we expected the perceived role of the robot to affect children’s recall and understanding abilities. Planned contrasts suggested that those who perceived the robots as adults recalled more story events when alone than in a group, F(1, 36) = 11.54, p = 0.002, and η2 = 0.24 (see Figure 9). However, for participants who perceived robots as peers, no significant differences were found between interaction context. Among the participants in the group condition, those who perceived robots as peers (rather than adults) had higher NSS, F(1, 36) = 4.26, p = 0.046, and η2 = 0.11.
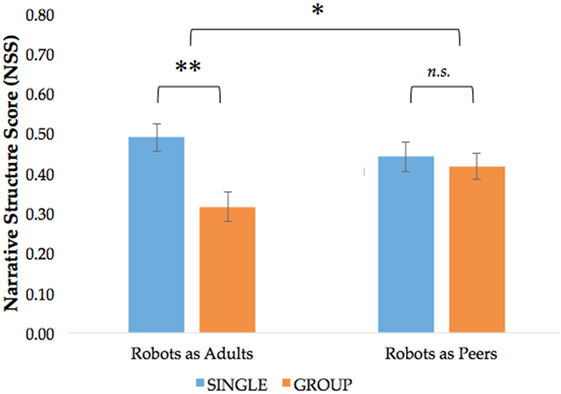
Figure 9. Average Narrative Structure Scores (NSS) for participants in each condition and perception of the robots (peer vs. adult). **p < 0.01 and *p < 0.05.
Perception of the robots in single versus group interactions did not seem to predict the emotional understanding of the students (EUS), as none of the planned comparisons were significant. Therefore, Hypothesis 4 was only partially supported.
8. Discussion
We separate this discussion into the two main steps of our analysis: exploratory analysis of interaction context and effects of individual differences in children’s story recall and emotional understanding.
8.1. Effects of Interaction Context (RQ1)
Our study yielded interesting findings about the effects of interaction context on children’s recall and understanding abilities. Participants interacting with the robots alone were able to recall the narrative structure (i.e., core ideas and characters) significantly better than participants in the group condition.
We offer three possible interpretations from these results. First, while the child was solely responsible for all choices when interacting alone, decisions were shared when in the group, thereby affecting how the interaction was experienced. A second interpretation is that in individual interactions, children may be more attentive since social standing in relation to their peers is not a factor. Third, the peers might simply be more distracting.
At first glance, our results may seem to contradict previous findings highlighting the benefits of learning in small groups (Hill, 1982; Pai et al., 2015). However, recalling story details is different than increasing learning gains. In fact, no significant differences were found between groups in our main learning metric, Emotional Understanding Score (participants’ ability to interpret the stories using the concepts of the RULER framework), despite average individual condition scores being slightly higher for every session. Other than session 2, which had the most difficult story content, all participants performed quite well despite the type of interaction in which they participated. One possible explanation, in line with the findings from Shahid et al. (2014), is that participants in the individual condition might have benefited from some of the effects of a group setting since they were interacting with multiple autonomous agents (the two robots), but further research is needed to verify this. Moreover, several authors argue that group interaction and subsequent learning gains do not necessarily occur just because learners are in a group (Kreijns et al., 2003). An analysis of the participants’ behavior while in the group during the interaction could clarify these alternative explanations.
8.2. Effects of Individual Differences (RQ2)
Our hypotheses about individual differences proved to be useful to further understand the effects of children interacting with robots alone or in small groups. The results suggest that interaction context is not the only relevant predictor for children’s success in story recall and emotional understanding.
Grade level, for example, seems a good predictor of children’s recall and understanding in these two contexts (H1). First graders interacting alone with the robots scored higher on our two main metrics (NSS and EUS) than first graders in the group condition, but no significant differences were found in second graders. While a more comprehensive analysis is necessary to validate this result, our trend suggests that first graders might not have developed the necessary skills to learn in small groups, but second graders (and potentially higher grade levels) are ready to do so.
Contrasting previous research, no significant gender differences were found in our data and, therefore, were unable to validate H2. In the existing HRI studies where gender differences were found, participants were adults and most of the effects were related to preferences rather than performance. While other reasons like a different robot or type of task might explain this result, one possibility is that children at this age might not have developed gender bias. The previous literature suggesting gender differences in narrative accuracy and emotional understanding in children might not apply as much to the present generation, as gender neutrality is currently promoted more widely in classrooms. In fact, one of the most recent meta-reviews in this area concludes that there is little evidence for gender differences in episodic memory (Andreano and Cahill, 2009).
Recall abilities seem to be affected by empathy levels in specific interaction contexts, with lower empathy individuals scoring higher on story recall in the single condition compared to the group condition (H3). A possible explanation is that lower empathy students need to be in a less distracting environment to achieve similar recall as high empathy students. Not surprisingly, our hypothesis confirmed the relation between high empathy and higher emotional understanding (Salovey and Mayer, 1990).
Like in other HRI experiments, the way participants perceived the robots had an impact on the collected measures (H4). In this domain, higher story recall is more likely to occur when participants perceive the robots as adults while interacting alone with the robot and when they perceive the robots as peers while interacting in small groups. More importantly, these findings suggest that researchers should design robot behaviors tailored to a specific interaction context and make sure that the robot’s behavior is coherent with the role they are trying to portray (e.g., teacher or peer).
9. Implications for Future Research
There are potential implications for the future design of socially assistive robotic scenarios based on the results obtained in this study. First, considering how well children reacted to the robots and reflected on the different choices in the postinterviews in both study conditions, affective interactive narratives using multiple robots seem to be a promising approach in socially assistive robotics.
Regarding the optimal type of interaction for these interventions, while single interactions seem to be slightly more effective in the short-term, group interventions might be more suitable in the long-term. Previous research has shown that children have more fun interacting with robots in groups rather than alone (Shahid et al., 2014). Since levels of engagement are positively correlated with students’ motivation for pursuing learning goals (Ryan and Deci, 2000), influence concentration, and foster group discussions (Walberg, 1990), future research in this area should study the effects of different interaction contexts in long-term exposure to robots.
Our results have also shown that specific interaction contexts might be more suitable for particular children based on their individual differences such as grade, empathy level, and the way they perceive the robots. Therefore, in order to maximize recall and understanding gains, it might be necessary to implement more sophisticated perception and adaptation mechanisms in the robots. For example, the robots should be able to detect disengagement and employ recovery mechanisms to keep children focused in the interaction, particularly in group settings (Leite et al., 2015b). Similarly, as group interactions seem more effective when participants perceive the robots as peers, the robots could portray different roles depending on whether they were interacting with one single child or a small group.
10. Conclusion
The effective acquisition of social and emotional skills requires constant practice in diverse hypothetical situations. In this paper, we proposed a novel approach where multiple socially assistive robots are used in interactive role-playing activities with children. The robots acted as interactive puppets; children could control the actions of one of the robots and see the impact of the selected actions on the course of the story. Using this scenario, we investigated the effects of interaction context (single child versus small groups) and individual factors (grade, gender, empathy level, and perception of the robots) on children’s story recall and emotional interpretation of three interactive stories.
Results from this repeated interaction study showed that although participants who interacted alone with the robot remembered the stories better than participants in the group condition, no significant differences were found in children’s emotional interpretation of the narratives. This latter metric was fairly high for all participants, except in the session with the hardest story content. To further understand these results, we investigated the effects of participants’ individual differences in the two interaction contexts for these metrics. We found that single settings seem more appropriate to first graders than groups, empathy is a very important predictor for emotional understanding of the narratives, and children’s performance varies depending on the way they perceive the robots (peer vs. adult) in the two interaction contexts. In addition to the promising results of this study, further research is required to more thoroughly understand how context of interaction affects children’s learning gains in longer-term interactions with socially assistive robots, as well as how participants’ individual differences interplay with each other.
Author Contributions
All the authors contributed to the system and experimental design. IL, MM, ML, DU, and NS ran the experiment and conducted data analysis.
Conflict of Interest Statement
The authors declare that the research was conducted in the absence of any commercial or financial relationships that could be construed as a potential conflict of interest.
Acknowledgments
We thank Bethel Assefa for assistance in data collection and coding, Rachel Protacio and Jennifer Allen for recording the voices for the robots, Emily Lennon for artwork creation, and the students and staff from the school where the study was conducted.
Funding
This work was supported by the NSF Expedition in Computing Grant #1139078 and SRA International (US Air Force) Grant #13-004807.
References
Admoni, H., Nawroj, A., Leite, I., Ye, Z., Hayes, B., Rozga, A., et al. (2015). Mykeepon Project. Available at: http://hennyadmoni.com/keepon
Al Moubayed, S., Beskow, J., Skantze, G., and Granstrom, B. (2012). “Furhat: a back-projected human-like robot head for multiparty human-machine interaction,” in Cognitive Behavioural Systems, Volume 7403 of Lecture Notes in Computer Science, eds A. Esposito, A. Esposito, A. Vinciarelli, R. Hoffmann, and V. Muller (Berlin, Heidelberg: Springer), 114–130.
Andreano, J. M., and Cahill, L. (2009). Sex influences on the neurobiology of learning and memory. Learn. Mem. 16, 248–266. doi: 10.1101/lm.918309
Andrist, S., Mutlu, B., and Tapus, A. (2015). “Look like me: matching robot personality via gaze to increase motivation,” in Proceedings of the 33rd Annual ACM Conference on Human Factors in Computing Systems, CHI ’15 (New York, NY: ACM), 3603–3612.
Bainbridge, W. A., Hart, J. W., Kim, E. S., and Scassellati, B. (2011). The benefits of interactions with physically present robots over video-displayed agents. Int. J. Soc. Robot. 3, 41–52. doi:10.1007/s12369-010-0082-7
Baxter, P., de Greeff, J., and Belpaeme, T. (2013). “Do children behave differently with a social robot if with peers?” in International Conf. on Social Robotics (ICSR 2013) (Bristol: Springer).
Bliss, L. S., McCabe, A., and Miranda, A. E. (1998). Narrative assessment profile: discourse analysis for school-age children. J. Commun. Disord. 31, 347–363. doi:10.1016/S0021-9924(98)00009-4
Bohus, D., Saw, C. W., and Horvitz, E. (2014). “Directions robot: in-the-wild experiences and lessons learned,” in Proceedings of the 2014 International Conference on Autonomous Agents and Multi-Agent Systems, AAMAS’14 (Richland, SC: International Foundation for Autonomous Agents and Multiagent Systems), 637–644.
Bolla-Wilson, K., and Bleecker, M. L. (1986). Influence of verbal intelligence, sex, age, and education on the rey auditory verbal learning test. Dev. Neuropsychol. 2, 203–211. doi:10.1080/87565648609540342
Brackett, M. A., Rivers, S. E., and Salovey, P. (2011). Emotional intelligence: implications for personal, social, academic, and workplace success. Soc. Personal. Psychol. Compass 5, 88–103. doi:10.1111/j.1751-9004.2010.00334.x
Breazeal, C., Brooks, A., Gray, J., Hancher, M., McBean, J., Stiehl, D., et al. (2003). Interactive robot theatre. Commun. ACM 46, 76–85. doi:10.1145/792704.792733
Briton, N. J., and Hall, J. A. (1995). Beliefs about female and male nonverbal communication. Sex Roles 32, 79–90. doi:10.1007/BF01544758
Bruce, A., Knight, J., Listopad, S., Magerko, B., and Nourbakhsh, I. (2000). “Robot improv: using drama to create believable agents,” in Proc. of the Int. Conf. on Robotics and Automation, ICRA’00 (San Francisco, CA: IEEE), 4002–4008.
Bryant, B. K. (1982). An index of empathy for children and adolescents. Child Dev. 53, 413–425. doi:10.2307/1128984
Buckner, J. P., and Fivush, R. (1998). Gender and self in children’s autobiographical narratives. Appl. Cogn. Psychol. 12, 407–429. doi:10.1002/(SICI)1099-0720(199808)12:4<407::AID-ACP575>3.0.CO;2-7
Camras, L. A., and Allison, K. (1985). Children’s understanding of emotional facial expressions and verbal labels. J. Nonverbal Behav. 9, 84–94. doi:10.1007/BF00987140
Capitani, E., Laiacona, M., and Basso, A. (1998). Phonetically cued word-fluency, gender differences and aging: a reappraisal. Cortex 34, 779–783. doi:10.1016/S0010-9452(08)70781-0
Capps, L., Losh, M., and Thurber, C. (2000). The frog ate the bug and made his mouth sad: narrative competence in children with autism. J. Abnorm. Child Psychol. 28, 193–204. doi:10.1023/A:1005126915631
Castillo, R., Fernández-Berrocal, P., and Brackett, M. A. (2013). Enhancing teacher effectiveness in Spain: a pilot study of the RULER approach to social and emotional learning. J. Educ. Train. Stud. 1, 263–272. doi:10.11114/jets.v1i2.203
Collis, B. (1996). The internet as an educational innovation: lessons from experience with computer implementation. Educ. Technol. 36, 21–30.
Crais, E. R., and Lorch, N. (1994). Oral narratives in school-age children. Top. Lang. Disord. 14, 13–28. doi:10.1097/00011363-199405000-00004
Crow, T., Crow, L., Done, D., and Leask, S. (1998). Relative hand skill predicts academic ability: global deficits at the point of hemispheric indecision. Neuropsychologia 36, 1275–1282. doi:10.1016/S0028-3932(98)00039-6
Denham, S. A. (1986). Social cognition, prosocial behavior, and emotion in preschoolers: contextual validation. Child Dev. 57, 194–201. doi:10.2307/1130651
Dillenbourg, P. (1999). What do you mean by collaborative learning? Collab. Learn. Cogn. Comput. Approaches 1–19.
Dunn, J., Bretherton, I., and Munn, P. (1987). Conversations about feeling states between mothers and their young children. Dev. Psychol. 23, 132. doi:10.1037/0012-1649.23.1.132
Eisenberg, N., and Lennon, R. (1983). Sex differences in empathy and related capacities. Psychol. Bull. 94, 100. doi:10.1037/0033-2909.94.1.100
Foster, M. E., Gaschler, A., Giuliani, M., Isard, A., Pateraki, M., and Petrick, R. P. (2012). “Two people walk into a bar: dynamic multi-party social interaction with a robot agent,” in Proceedings of the 14th ACM International Conference on Multimodal Interaction, ICMI ’12 (New York, NY: ACM), 3–10.
Gomez, R., Kawahara, T., Nakamura, K., and Nakadai, K. (2012). “Multi-party human-robot interaction with distant-talking speech recognition,” in Proceedings of the Seventh Annual ACM/IEEE International Conference on Human-Robot Interaction, HRI ’12 (New York, NY: ACM), 439–446.
Greif, E., Alvarez, M., and Ulman, K. (1981). Recognizing emotions in other people: sex differences in socialization. Poster Presented at the Biennial Meeting of the Society for Research in Child Development, Boston, MA.
Griffith, P. L., Ripich, D. N., and Dastoli, S. L. (1986). Story structure, cohesion, and propositions in story recalls by learning-disabled and nondisabled children. J. Psycholinguist. Res. 15, 539–555. doi:10.1007/BF01067635
Harris, P. L. (1983). Children’s understanding of the link between situation and emotion. J. Exp. Child Psychol. 36, 490–509. doi:10.1016/0022-0965(83)90048-6
Harris, P. L. (1989). Children and Emotion: The Development of Psychological Understanding. Basil Blackwell.
Hill, G. W. (1982). Group versus individual performance: are n+1 heads better than one? Psychol. Bull. 91, 517. doi:10.1037/0033-2909.91.3.517
Hoffman, G., Kubat, R., and Breazeal, C. (2008). “A hybrid control system for puppeteering a live robotic stage actor,” in Proc. of RO-MAN 2008 (IEEE), 354–359.
Hoffman, M. L. (1977). Sex differences in empathy and related behaviors. Psychol. Bull. 84, 712. doi:10.1037/0033-2909.84.4.712
Iaria, G., Petrides, M., Dagher, A., Pike, B., and Bohbot, V. D. (2003). Cognitive strategies dependent on the hippocampus and caudate nucleus in human navigation: variability and change with practice. J. Neurosci. 23, 5945–5952.
Johansson, M., Skantze, G., and Gustafson, J. (2013). “Head pose patterns in multiparty human-robot team-building interactions,” in Social Robotics, Volume 8239 of Lecture Notes in Computer Science, eds G. Herrmann, M. Pearson, A. Lenz, P. Bremner, A. Spiers, and U. Leonards (New York, NY: Springer), 351–360.
John, S. F., Lui, M., and Tannock, R. (2003). Children’s story retelling and comprehension using a new narrative resource. Can. J. Sch. Psychol. 18, 91–113. doi:10.1177/082957350301800105
Kanda, T., Sato, R., Saiwaki, N., and Ishiguro, H. (2007). A two-month field trial in an elementary school for long-term human-robot interaction. IEEE Trans. Robot. 23, 962–971. doi:10.1109/TRO.2007.904904
Kelleher, C., Pausch, R., and Kiesler, S. (2007). “Storytelling Alice motivates middle school girls to learn computer programming,” in Proc. of the SIGCHI Conf. on Human Factors in Computing Systems, CHI ’07 (New York, NY: ACM), 1455–1464.
Kim, Y., and Baylor, A. L. (2006). A social-cognitive framework for pedagogical agents as learning companions. Educ. Technol. Res. Dev. 54, 569–596. doi:10.1007/s11423-006-0637-3
Kozima, H., Michalowski, M., and Nakagawa, C. (2009). Keepon: a playful robot for research, therapy, and entertainment. Int. J. Soc. Robot. 1, 3–18. doi:10.1007/s12369-008-0009-8
Kramer, J. H., Delis, D. C., Kaplan, E., O’Donnell, L., and Prifitera, A. (1997). Developmental sex differences in verbal learning. Neuropsychology 11, 577. doi:10.1037/0894-4105.11.4.577
Kreijns, K., Kirschner, P. A., and Jochems, W. (2003). Identifying the pitfalls for social interaction in computer-supported collaborative learning environments: a review of the research. Comput. Human Behav. 19, 335–353. doi:10.1016/S0747-5632(02)00057-2
Leite, I., Martinho, C., and Paiva, A. (2013). Social robots for long-term interaction: a survey. Int. J. Soc. Robot. 5, 291–308. doi:10.1007/s12369-013-0178-y
Leite, I., McCoy, M., Lohani, M., Ullman, D., Salomons, N., Stokes, C., et al. (2015a). “Emotional storytelling in the classroom: individual versus group interaction between children and robots,” in Proceedings of the Tenth Annual ACM/IEEE International Conference on Human-Robot Interaction, HRI ’15 (New York, NY: ACM), 75–82.
Leite, I., McCoy, M., Ullman, D., Salomons, N., and Scassellati, B. (2015b). “Comparing models of disengagement in individual and group interactions,” in Proceedings of the Tenth Annual ACM/IEEE International Conference on Human-Robot Interaction, HRI ’15 (New York, NY: ACM), 99–105.
Leyzberg, D., Spaulding, S., Toneva, M., and Scassellati, B. (2012). “The physical presence of a robot tutor increases cognitive learning gains,” in Proc. of the 34th Annual Conf. of the Cognitive Science Society (Austin, TX: Cognitive Science Society).
Linn, M. C., and Petersen, A. C. (1985). Emergence and characterization of sex differences in spatial ability: a meta-analysis. Child Dev. 56, 1479–1498. doi:10.2307/1130467
Lopes, P. N., Salovey, P., Coté, S., and Beers, M. (2005). Emotion regulation abilities and the quality of social interaction. Emotion 5, 113–118. doi:10.1037/1528-3542.5.1.113
Lou, Y., Abrami, P. C., and d’ÄôApollonia, S. (2001). Small group and individual learning with technology: a meta-analysis. Rev. Educ. Res. 71, 449–521. doi:10.3102/00346543071003449
Maccoby, E. E., and Jacklin, C. N. (1974). The Psychology of Sex Differences, Vol. 1. Stanford University Press.
MacDorman, K. F., and Entezari, S. O. (2015). Individual differences predict sensitivity to the uncanny valley. Interact. Stud. 16, 141–172. doi:10.1075/is.16.2.01mac
McCabe, A., Bliss, L., Barra, G., and Bennett, M. (2008). Comparison of personal versus fictional narratives of children with language impairment. Am. J. Speech Lang. Pathol. 17, 194–206. doi:10.1044/1058-0360(2008/019)
McCartney, K. A., and Nelson, K. (1981). Children’s use of scripts in story recall. Discourse Process. 4, 59–70. doi:10.1080/01638538109544506
McGuigan, F., and Salmon, K. (2006). The influence of talking on showing and telling: adult-child talk and children’s verbal and nonverbal event recall. Appll. Cogn. Psychol. 20, 365–81. doi:10.1002/acp.1183
Mercer, N. (1996). The quality of talk in children’s collaborative activity in the classroom. Learn. Instr. 6, 359–377. doi:10.1016/S0959-4752(96)00021-7
Moreland, R. L. (2010). Are dyads really groups? Small Group Res. 41, 251–267. doi:10.1177/1046496409358618
Morrow, D. G. (1985). Prominent characters and events organize narrative understanding. J. Mem. Lang. 24, 304–319. doi:10.1016/0749-596X(85)90030-0
Mubin, O., Stevens, C. J., Shahid, S., Al Mahmud, A., and Dong, J.-J. (2013). A review of the applicability of robots in education. J. Technol. Educ. Learn. 1, 209–215. doi:10.2316/Journal.209.2013.1.209-0015
Mumm, J., and Mutlu, B. (2011). “Human-robot proxemics: physical and psychological distancing in human-robot interaction,” in Proceedings of the 6th International Conference on Human-Robot Interaction, HRI ’11 (New York, NY: ACM), 331–338.
Mutlu, B., Forlizzi, J., and Hodgins, J. (2006). “A storytelling robot: modeling and evaluation of human-like gaze behavior,” in Humanoid Robots, 2006 6th IEEE-RAS International Conference on (Genova, Italy: IEEE), 518–523.
Nomura, T., Kanda, T., Suzuki, T., and Kato, K. (2008). Prediction of human behavior in human–robot interaction using psychological scales for anxiety and negative attitudes toward robots. IEEE Trans. Robot. 24, 442–451. doi:10.1109/TRO.2007.914004
Pai, H.-H., Sears, D., and Maeda, Y. (2015). Effects of small-group learning on transfer: a meta-analysis. Educ. Psychol. Rev. 27, 79–102. doi:10.1007/s10648-014-9260-8
Pereira, A., Prada, R., and Paiva, A. (2014). “Improving social presence in human-agent interaction,” in Proceedings of the SIGCHI Conference on Human Factors in Computing Systems, CHI ’14 (New York, NY: ACM), 1449–1458.
Petrides, K., and Furnham, A. (2000). On the dimensional structure of emotional intelligence. Pers. Individ. Dif. 29, 313–320. doi:10.1016/S0191-8869(99)00195-6
Pohl, R. F., Bender, M., and Lachmann, G. (2005). Autobiographical memory and social skills of men and women. Appl. Cogn. Psychol. 19, 745–760. doi:10.1002/acp.1104
Pons, F., Harris, P. L., and de Rosnay, M. (2004). Emotion comprehension between 3 and 11 years: developmental periods and hierarchical organization. Eur. J. Dev. Psychol. 1, 127–152. doi:10.1080/17405620344000022
Quigley, M., Conley, K., Gerkey, B. P., Faust, J., Foote, T., Leibs, J., et al. (2009). “Ros: an open-source robot operating system,” in ICRA Workshop on Open Source Software (Kobe, Japan).
Renz, K., Lorch, E. P., Milich, R., Lemberger, C., Bodner, A., and Welsh, R. (2003). On-line story representation in boys with attention deficit hyperactivity disorder. J. Abnorm. Child Psychol. 31, 93–104. doi:10.1023/A:1021777417160
Rivers, S. E., Brackett, M. A., Reyes, M. R., Elbertson, N. A., and Salovey, P. (2013). Improving the social and emotional climate of classrooms: a clustered randomized controlled trial testing the RULER approach. Prev. Sci. 14, 77–87. doi:10.1007/s11121-012-0305-2
Ross, M., and Holmberg, D. (1992). Are wives’ memories for events in relationships more vivid than their husbands’ memories? J. Soc. Pers. Relat. 9, 585–604. doi:10.1177/0265407592094007
Ryan, R. M., and Deci, E. L. (2000). Self-determination theory and the facilitation of intrinsic motivation, social development, and well-being. Am. Psychol. 55, 68. doi:10.1037/0003-066X.55.1.68
Saerbeck, M., Schut, T., Bartneck, C., and Janse, M. D. (2010). “Expressive robots in education: varying the degree of social supportive behavior of a robotic tutor,” in Proceedings of the SIGCHI Conference on Human Factors in Computing Systems, CHI ’10 (New York, NY: ACM), 1613–1622.
Salovey, P., and Mayer, J. D. (1990). Emotional intelligence. Imagination Cogn. Pers. 9, 185–211. doi:10.2190/DUGG-P24E-52WK-6CDG
Schermerhorn, P., Scheutz, M., and Crowell, C. R. (2008). “Robot social presence and gender: do females view robots differently than males?” in Proceedings of the 3rd ACM/IEEE International Conference on Human Robot Interaction (Amsterdam, The Netherlands: ACM), 263–270.
Schoenau-Fog, H. (2011). “Hooked! – evaluating engagement as continuation desire in interactive narratives,” in Proceedings of the 4th International Conference on Interactive Digital Storytelling (Berlin, Heidelberg: Springer-Verlag), 219–230.
Schultze, T., Mojzisch, A., and Schulz-Hardt, S. (2012). Why groups perform better than individuals at quantitative judgment tasks: group-to-individual transfer as an alternative to differential weighting. Organ. Behav. Hum. Decis. Process 118, 24–36. doi:10.1016/j.obhdp.2011.12.006
Shahid, S., Krahmer, E., and Swerts, M. (2014). Child-robot interaction across cultures: how does playing a game with a social robot compare to playing a game alone or with a friend? Comput. Human Behav. 40, 86–100. doi:10.1016/j.chb.2014.07.043
Smedler, A.-C., and Törestad, B. (1996). Verbal intelligence: a key to basic skills? Educ. Stud. 22, 343–356. doi:10.1080/0305569960220304
Syrdal, D. S., Koay, K. L., Walters, M. L., and Dautenhahn, K. (2007). “A personalized robot companion? The role of individual differences on spatial preferences in hri scenarios,” in Robot and Human Interactive Communication, 2007. RO-MAN 2007. The 16th IEEE International Symposium on (IEEE), 1143–1148.
Szafir, D., and Mutlu, B. (2012). “Pay attention! Designing adaptive agents that monitor and improve user engagement,” in Proc. of the SIGCHI Conf. on Human Factors in Computing Systems, CHI ’12 (New York, NY: ACM), 11–20.
Takayama, L., and Pantofaru, C. (2009). “Influences on proxemic behaviors in human-robot interaction,” in Intelligent Robots and Systems, 2009. IROS 2009. IEEE/RSJ International Conference on (St. Louis, MO: IEEE), 5495–5502.
Tapus, A., Matarić, M., and Scassellatti, B. (2007). The grand challenges in socially assistive robotics. IEEE Robot. Auto. Mag. 14, 35–42. doi:10.1109/MRA.2007.339605
Vannini, N., Enz, S., Sapouna, M., Wolke, D., Watson, S., Woods, S., et al. (2011). Fearnot! Computer-based anti-bullying programme designed to foster peer intervention. Eur. J. Psychol. Educ. 26, 21–44. doi:10.1007/s10212-010-0035-4
Walberg, H. J. (1990). Productive teaching and instruction: assessing the knowledge base. Phi Delta Kappan. 71, 470–478.
Walters, M. L., Dautenhahn, K., Te Boekhorst, R., Koay, K. L., Kaouri, C., Woods, S., et al. (2005). “The influence of subjects’ personality traits on personal spatial zones in a human-robot interaction experiment,” in Robot and Human Interactive Communication, 2005. ROMAN 2005. IEEE International Workshop on (IEEE), 347–352.
Keywords: socially assistive robotics, emotional intelligence, individual differences, multiparty interaction
Citation: Leite I, McCoy M, Lohani M, Ullman D, Salomons N, Stokes C, Rivers S and Scassellati B (2017) Narratives with Robots: The Impact of Interaction Context and Individual Differences on Story Recall and Emotional Understanding. Front. Robot. AI 4:29. doi: 10.3389/frobt.2017.00029
Received: 30 December 2016; Accepted: 12 June 2017;
Published: 12 July 2017
Edited by:
Hatice Gunes, University of Cambridge, United KingdomReviewed by:
Erol Sahin, Middle East Technical University, TurkeyRodolphe Gelin, Aldebaran Robotics, France
Copyright: © 2017 Leite, McCoy, Lohani, Ullman, Salomons, Stokes, Rivers and Scassellati. This is an open-access article distributed under the terms of the Creative Commons Attribution License (CC BY). The use, distribution or reproduction in other forums is permitted, provided the original author(s) or licensor are credited and that the original publication in this journal is cited, in accordance with accepted academic practice. No use, distribution or reproduction is permitted which does not comply with these terms.
*Correspondence: Iolanda Leite, aW9sYW5kYUBrdGguc2U=