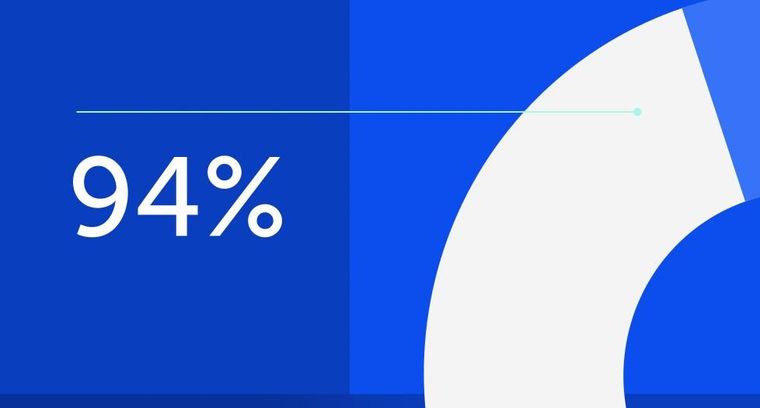
94% of researchers rate our articles as excellent or good
Learn more about the work of our research integrity team to safeguard the quality of each article we publish.
Find out more
ORIGINAL RESEARCH article
Front. Robot. AI, 31 May 2017
Sec. Humanoid Robotics
Volume 4 - 2017 | https://doi.org/10.3389/frobt.2017.00021
This article is part of the Research TopicAffective and Social Signals for HRIView all 14 articles
We conducted a user study for which we purposefully programmed faulty behavior into a robot’s routine. It was our aim to explore if participants rate the faulty robot different from an error-free robot and which reactions people show in interaction with a faulty robot. The study was based on our previous research on robot errors where we detected typical error situations and the resulting social signals of our participants during social human–robot interaction. In contrast to our previous work, where we studied video material in which robot errors occurred unintentionally, in the herein reported user study, we purposefully elicited robot errors to further explore the human interaction partners’ social signals following a robot error. Our participants interacted with a human-like NAO, and the robot either performed faulty or free from error. First, the robot asked the participants a set of predefined questions and then it asked them to complete a couple of LEGO building tasks. After the interaction, we asked the participants to rate the robot’s anthropomorphism, likability, and perceived intelligence. We also interviewed the participants on their opinion about the interaction. Additionally, we video-coded the social signals the participants showed during their interaction with the robot as well as the answers they provided the robot with. Our results show that participants liked the faulty robot significantly better than the robot that interacted flawlessly. We did not find significant differences in people’s ratings of the robot’s anthropomorphism and perceived intelligence. The qualitative data confirmed the questionnaire results in showing that although the participants recognized the robot’s mistakes, they did not necessarily reject the erroneous robot. The annotations of the video data further showed that gaze shifts (e.g., from an object to the robot or vice versa) and laughter are typical reactions to unexpected robot behavior. In contrast to existing research, we assess dimensions of user experience that have not been considered so far and we analyze the reactions users express when a robot makes a mistake. Our results show that decoding a human’s social signals can help the robot understand that there is an error and subsequently react accordingly.
Social robots are not yet in a technical state where they operate free from errors. Nevertheless, most research approaches act on the assumption of robots performing faultlessly. This results in a confined standpoint, in which the created scenarios are considered as gold standard. Alternatives resulting from unforeseeable conditions that develop during an experiment are often not further regarded or simply excluded. It lies within the nature of thorough scientific research to pursue a strict code of conduct. However, we suppose that faulty instances of human–robot interaction (HRI) are nevertheless full with knowledge that can help us further improve the interactional quality in new dimensions. We think that because most research focuses on perfect interaction, many potentially crucial aspects are overlooked.
Research that is specifically directed at exploring erroneous instances of interaction could be useful to further refine the quality of HRI. For example, a robot that understands that there is a problem in the interaction by correctly interpreting the user’s social signals could let the user know that it understands the problem and actively apply error recovery strategies. Knowing the severity of an error could further be helpful for the robot in finding the adequate corrective action.
Since robots in HRI are social actors, they elicit mental models and expectations known from human–human interaction (HHI) (Lohse, 2011). One aspect we know from HHI is that imperfections make human social actors more likeable and more believable. The psychological phenomenon Pratfall Effect states that people’s attractiveness increases when they commit a mistake. Aronson et al. (1966) suggest that superior people may be viewed as superhuman and distant while a mistake would make them seem more human. Similarly, one could argue that robots are often seen as impeccable, since this is how they are presented in the media (Bruckenberger et al., 2013). Especially, people who have not interacted with robots themselves build their mental models and expectations about robots from those media. Moreover, experience with technology in general is mostly based on interaction with consumer products, such as smartphones or TVs. Those products are very common and need to work more or less error-free in order to get accepted on the market. For example, a TV which has problems in sound will not survive long on the market. People expect technology they paid for to work without errors. What makes the interaction with social robots different is that a TV is not seen as a social actor, in contrast to a social robot. This might result in people assuming robots to be without fail, which makes them likewise seem distant (Pratfall Effect). Robots that commit errors, on the other hand, could then be viewed as more human-like and, in subsequence, more likeable. With their study on an erroneous robot in a competitive game-play scenario Ragni et al. (2016) provided additional evidence that people consider robots in general as competent, functional, and intelligent.
In our effort to embrace the imperfections of social robots and create more believable robot characters, we propose to specifically explore faulty robot behavior and the social signals humans show when a robot commits a mistake. The term social signal is used to describe verbal and non-verbal signals that humans use in a conversation to communicate their intentions. Vinciarelli et al. (2009) argued that the ability to recognize social signals is crucial to mastering social intelligence. It is our long-term goal to enable robots to communicate about their errors and deploy recovery strategies. To achieve this ambitious goal, more general knowledge about robot errors is required. We report on a user study where we purposefully elicited faulty robot behavior.
Our user study is based on our previous research where we analyzed an extensive pool of video data showing social HRI instances where the robot made an error. The videos covered a variety of scenarios in different contexts, different robots, and a multitude of social signals. The robot errors happened unintentionally and, thus, the data created a sound basis for studying the nature of error situations. We found that there are two different kinds of robot errors, i.e., social norm violations (SNV)and technical failures (TF) (Giuliani et al., 2015), for which human interaction partners respond with typical social signals (Mirnig et al., 2015). A social norm violation means that the robot’s actions deviate from the underlying social script, that is, the commonly known interaction steps a certain situation is expected to take. For example, a participant orders a drink from a bartender robot, the robot signals it has understood but then asks again for the participant’s order. A technical failure means that the robot experiences a technical disruption that is perceived as such by the user. For example, a robot picks up an object but then loses it while grasping. From an expert perspective all robot errors might be considered as technical failures. Since, we are interested in the human perception of robot errors, we distinguish error types from how a human most likely perceives error events.
With the user study presented in this paper, we expand our previous research in purposefully eliciting robot errors and researching the resulting social signals of the human interaction partners. We measured how users perceive a robot that makes errors during interaction (social norm violations and technical failures) as compared to a robot operating free from errors.
The directed exploration of robot errors in social interaction is a new and upcoming topic. The HRI research community has reported first results on exploratory user studies. For example, Salem et al. (2015) conducted an experiment with an erroneous robot. The researchers measured how the robot’s behavior influenced how the participants rated its trustworthiness and reliability. They also measured if robot errors affect the task performance. The researchers found that while participants rated the correctly behaving robot as significantly more trustworthy and reliable, the fact that a robot performs correctly or faulty did not influence the objective task performance.
In an earlier work, Salem et al. (2013) researched the effect of speech and gesture congruence on perceived anthropomorphism, likability, and task performance. In their experiment, a robot either spoke only, spoke while making congruent coverbal gestures, or spoke while making incongruent coverbal gestures. The researchers found that congruent coverbal gesturing makes a robot appear more anthropomorphic and more likeable. This effect was even stronger for incongruent coverbal gesturing. However, incongruent coverbal gesturing resulted in a lower task performance. Following our line of argumentation, such incongruent behavior violates the human social script, as humans do not expect incongruent messages from different modalities in everyday interactions (Schank and Abelson, 1977). Therefore, incongruent multimodal robot behavior results in a social norm violation. Ragni et al. (2016) reported similar effects. The researchers performed a study in which a human and a robot competed against each other in a reasoning task and a memory task. During the interaction, the robot either performed with or without errors. While participants rated the faulty robot as less competent, less reliable, less intelligent, and less superior than the error-free robot, participants reported having enjoyed the interaction more when the robot made errors. However, the task performance was significantly lower in the faulty robot condition.
Gompei and Umemuro (2015) investigated how a robot’s speech errors influenced how familiar and sincere it was rated. The researchers found that speech errors made early in an interaction might lower the robot’s sincerity rating. However, speech errors that are introduced later in the interaction might increase the robot’s familiarity. Short et al. (2010) investigated people’s perception when playing rock–paper–scissors with a robot that either played fair, cheated verbally by announcing a different hand gesture, or cheated with its actions by changing the hand gesture. The researchers found that a cheating robot resulted in a bigger social engagement, in comparison to one which plays fair. They stated that the results suggest that participants showed more verbal social signals to the robot that cheated. Participants were surprised by the cheating behavior of the robot, although verbal cheating was perceived as malfunction, while cheating through action was perceived as deliberate cheating behavior. These findings support our assumption that through unexpected behavior, people see a robot as a more social actor and that unexpected behavior might be interpreted as erroneous behavior.
In an online survey, Lee et al. (2010) found that when a service robot made a mistake, this has a strong negative impact on people’s rating of the service quality and the robot itself. However, when the robot deployed a recovery strategy, both the rating of the service and the rating of the robot improved. The researchers deployed different recovery strategies and found that all of them increased the ratings of the robot’s politeness. A robot which apologized for its mistake was seen more competent, people liked it more and felt closer to it, and a robot offering compensation for its mistake (such as a refund) was rated to be of more satisfying service quality but participants were hesitant to use the robot again. Whereas, an apology and a recovery strategy of offering options was perceived to foster reuse likelihood. In a related online survey, Brooks et al. (2016) explored people’s reactions to the failure of an autonomous robot. In the survey, participants were asked to assess situations where an autonomous robot experienced different kinds of failures that affected a human interacting with it. They found that people who saw an erroneous robot rated it rather negatively on a series of items (i.e., How satisfying, pleasing, disappointing, reliably, dependable, competent, responsible, trustworthy, risky to use is the robot?), while people who experienced a robot without failure rated it positively. When the erroneous robot deployed mitigation strategies to overcome the error either by prompting human intervention or by deploying a different approach, people’s ratings toward the erroneous robot became less negative. However, the amount the strategy influenced peoples reaction depended on the kind of task, the severity of the failure, and the risk of the failure.
To enable a robot to generate help requests in case of an error situation, Knepper et al. (2015) developed their inverse semantics algorithm. It allows the robot to phrase precise requests that specify the kind of help that is needed. The researchers evaluated their algorithm in a user study and found that participants preferred the precise request over high level, general phrasings. While in their approach errors are recognized through the robot’s internal state and the environment (e.g., the robot is supposed to pick up an object which it can visually detect, but the object is out of its reach), we envision an approach where the robot can additionally detect an error through its human interaction partner’s social signals. For example, Gehle et al. (2015) explored gaze patterns of human groups upon unexpected robot behavior in a museum guide scenario. They found that groups of visitors responded to unexpected robot behavior with stepwise gaze coordination, applying different modes of gaze constellation. Unexpected robot behavior is likely to conflict with the user expectations about the adequate social script in a certain situation. Therefore, unexpected robot behavior can lead to a social norm violation. A deviation from the social script resulted in a different strategy in the human gaze coordination (social signals). Hayes et al. (2016) performed a user study in which participants were instructed to teach a dance to a robot. They explored how humans implicitly responded when the robot made a mistake. The authors used a very small sample in their explorative study and did not provide a statistical analysis of their descriptive results.
Our approach extends the existing findings in several dimensions. While the errors in the study of Ragni et al. (2016) were based on errors from HHI, the errors we used were modeled based on data from HRI. Our work and that of Ragni et al. (2016) further cover different aspects: (a) their errors were task-related, ours non-task-related; (b) they covered the cognitive ability of the robot and we dealt with socially (in)appropriate robot behavior and more general soft- and hardware problems; and (c) they assessed the overall enjoyment of the interaction and users’ task performance, while we looked into the interconnectedness of likability, anthropomorphism, and intelligence. We chose to examine these factors since they are commonly used and accepted measures in the HRI domain. We were especially interested in likability as it contributes to the overall user experience and it may foster technology acceptance. Since erroneous behavior potentially compromises intelligence ratings, we were also interested in exploring if our robot’s mistakes make it seem less intelligent. In the light of the Pratfall Effect, we wanted to see if the robot’s anthropomorphism level is influenced by the fact that it makes or does not make mistakes.
The related literature shows that the importance of exploring robot errors has been recognized. We extend the state of the art with our data-driven approach by systematically analyzing specific kinds of errors and their effects on the interaction experience, as well as the users’ reactions to those errors (i.e., social signals).
We set up a Wizard of Oz (WOz) user study to specifically explore robot errors. A human and a robot interacted with each other in two verbal sessions. The first session was a verbal interview where the robot asked a few questions to the participant. The second session was a LEGO task, where the robot invited the participant to build a few simple objects. We chose this setup in order to reenact the verbal context of the related work (Giuliani et al., 2015; Mirnig et al., 2015). In addition, the interview session enabled us to collect qualitative data on the participants’ opinions, which we included in our data analysis.
The user study was performed between subjects, with each participant taking part in one of the following two conditions: (a) no error (baseline—the robot performs error-free) and (b) error (experimental condition—the robot commits eight errors over the entire interaction). To base the user study on the previous findings from Giuliani et al. (2015) and Mirnig et al. (2015), we programmed the robot to commit two social norm violations and two technical failures in each session. Based on our previous research, we defined these two types of error as the typical mistakes robots make in HRI. Therefore, we suppose that an interaction including these error types would be perceived as plausible. The complexity, severity, and risk level of the induced errors were chosen in alignment with our scenario. Naturally, different scenarios will entail other errors, different severity and risk levels. For example, Robinette et al. (2014) investigated faulty behavior of robots in safety critical situations. They simulated erroneous behavior of an emergency guiding robot that helps people to escape from a dangerous zone. They found that after the first error of the robot, people’s attitude toward the robot decreased significantly. However, the decision to follow the robot in a follow-up interaction was not affected by their decreased attitude.
As discussed in the previous sections, it is known that humans often base their expectations about robots on how robots are portrayed in the media. Since the media present robots frequently as perfect entities, we assume that social robots making errors negatively influence how their human interaction partners perceive them. Based on the findings on faulty robot actions in HRI as discussed so far and in light of the Pratfall Effect, we have postulated the following hypotheses for our user study:
H1:A robot that commits errors during its interaction with humans is perceived as more likeable than a robot that performs flawlessly.
H2:A robot that commits errors during its interaction with humans is perceived as more anthropomorphic than a robot that performs flawlessly.
H3:A robot that commits errors during its interaction with humans is perceived as less intelligent than a robot that performs flawlessly.
For the WOz user study, the participants were asked to interact with a NAO robot.1 We set the interaction up in two sessions. During the first session, the robot asked a set of predefined questions to the participant in order to restrict the thematic dimension of the conversation. During the second session, the robot invited the participant to perform a couple of tasks using LEGO bricks.
In the interview session, the robot asked ten questions to the participant. The first three questions were meant to make the participant familiar with the situation and to create a comfortable atmosphere. For this reason, they were always presented in the same order and they never contained an error. The subsequent seven questions were asked in random order and four out of seven questions contained errors in the error condition.
In the LEGO session, the participant had to (dis-)assemble LEGO bricks according to the robot’s instructions. The first two tasks were assigned in the same order for all participants and they did not contain errors. The subsequent eight tasks were assigned in random order and four out of eight tasks contained errors in the error condition.
The interview session lasted for an average of 3 min and 37 s (SD = 59 s) and the LEGO session 8 min and 14 s (SD = 1 min and 54 s). We decided for this two-part setup to keep the participants entertained with a diversified scenario. The two-part setup provided us also with the possibility to introduce a greater variety of errors and to achieve a higher number of errors in total.
The user study was performed in the User Experience and Interaction Experimentation Lab at the Center for Human-Computer Interaction at the University of Salzburg. The robot was wizarded from a researcher seated behind a bookshelf so that the wizarding was not obvious to the participant. A second researcher, likewise seated behind the bookshelf, controlled the video recording. During the entire interaction the participants stood adverse to the NAO robot at a distance of approximately 1.5 m. NAO was standing on a desk (see Figure 1 for the setup). The transition between the two sessions was immediate with no break in between. Both sessions happened in the same setting. The only change was that the researcher placed a wooden box (80 cm × 50 cm × 50 cm) on the table in front of the robot right before the LEGO session started. The box was used to provide the participants with a comfortable height to complete the building tasks. Together with the box, the participants were given a set of LEGO blocks (prebuilt shapes) with which they were to perform the tasks (see Figure 2).
Figure 1. Study setup with the participant interacting with the robot and two researchers seated behind a bookshelf who supervised the technology.
The between-subjects design required each person participating in either one of the two conditions. In the baseline condition, the robot performed free from errors. In the experimental condition, the robot committed two social norm violations and two technical failures each in both sessions. After each robot error, the researchers waited for the situation to unravel without them interfering. In many cases, the participants showed a reaction that confirmed that they had noticed the error (e.g., some participants laughed or frowned) and then moved on. The researchers only intervened in the rare cases where the interaction was severely interrupted, for example, when the participant directly addressed the researchers and commented on the error. In this case, the researcher simply asked the participant to continue interacting with the robot, in order to limit the interference as much as possible.
The three starting questions in the interview session and the first two building tasks were meant as an introduction and were not varied in order. Therefore, the robot errors occurred in the randomized questions/tasks only. Tables 1 and 2 give an overview on the questions and tasks and which errors occurred together with which question or task. The questions were similar in both conditions. The difference between the baseline and the experimental condition was achieved by the presence or absence of the robot errors.
The induced errors were mainly modeled based on our previous findings on typical robot errors as reported in the studies of Giuliani et al. (2015) and Mirnig et al. (2015). Only LEGO task number 7 in the error condition was inspired by unusual requests as reported in the study of Salem et al. (2015).
The setup of our user study is based on real-life HRI. It is data-driven in representing actual error situations and corresponding robot errors that occur when humans interact with state-of-the-art social robots, which makes our setup ecologically valid.
The participants were welcomed to the laboratory. After a short briefing, they were asked to sign an informed consent. Next, the participants were asked to complete questionnaires to assess their demographics, personality traits, and attitude toward robots. The participants were introduced to the robot and they were given an overview on the process of the user study. As soon as the participants took their position opposite the robot, the user study began. First, the participants answered a set of questions the robot asked them (Session 1). Second, the robot instructed the participants to complete a set of building tasks with LEGO blocks (Session 2). After the interaction with the robot, the participants were again asked to complete the questionnaire assessing their attitude toward robots. They were further asked to complete a questionnaire rating the robot’s likability, anthropomorphism, and perceived intelligence. The study was finalized with a closing interview where the researcher asked the participants four open-ended questions, which were followed by a short debriefing in which the purpose of the study was explained to the participants. The study procedure is depicted in Figure 3.
Before the interaction, we asked our participants to fill in the Big Five Inventory (BFI) questionnaire by John et al. (2008). We used this questionnaire to analyze if people’s personality influences how they perceive the robot. The BFI consists of 44 items (5-point Likert-scaled), constructing five subscales (extraversion, agreeableness, conscientiousness, neuroticism, and openness). This questionnaire is a well-accepted instrument among psychologists to assess the personality of humans. Therefore, we chose to use it for exploring potential connections between personality and how a social robot is perceived.
We used the Negative Attitude Toward Robots Scale (NARS) (Nomura et al., 2004) to assess participants’ general attitude toward robots. The NARS consists of 14 items (5-point Likert-scaled) that account for three scales: people’s negative attitude toward (S1) interaction with robots, (S2) social influence of robots, and (S3) emotions in interaction with robots. We asked the participants to complete the questionnaire before and after their interaction with the robot in order to measure if the interaction changed people’s attitude. The NARS is a widely used questionnaire in the HRI community and it provides researchers with a comprehensive understanding of human fears around social robots.
To explore how our participants rate the robot, we used three subscales from the Godspeed Questionnaire Series by Bartneck et al. (2009), i.e., anthropomorphism, likability, and perceived intelligence. Each of the scales consists of five 5-point Likert-scaled items. The scales were developed in the HRI community to specifically assess users’ perception of social robots. We chose the questionnaires since they are frequently used and widely accepted among the HRI community. The concepts the questionnaires cover are very relevant to social HRI and they represent the concepts we explore with our research. This questionnaire was administered once, after our participants’ interaction with the robot.
We used two sources to gain qualitative data from the participants regarding their attitude toward robots. First, the robot asked the participants about their opinion on robots in the interview session (see Table 1). Second, in the concluding interview after the interaction and after all the other questionnaires were filled in, we asked the following questions:
1. Did you notice anything special during your interaction with the robot that you would like to tell us?
2. Did your attitude toward robots change during the interaction?
3. What would you change about the interaction with the robot?
4. What did you think when the robot made a mistake? (This question was only asked for participants who took part in the error condition.)
A total of 45 participants took part in our user study (25 males and 20 females). The participants were recruited over a university mailing list and social media. They were primarily university students and they had no previous experience with robots. Their age ranged from 16 to 76 years, with a mean age of 25.91 years (SD = 10.82). As regards conditions, 21 participants completed the error condition and 24 the no error condition. The participants’ technology affinity was rated on average with a mean of 3.09 (SD = 1.49; 5-point Likert-scaled ranging from 1—“not technical” to 5—“technical”) and their preexperience with robots was below average with a mean of 1.96 (SD = 0.82; 5-point Likert-scaled ranging from 1—“never seen” to 5—“frequent usage”).
In order to verify that the manipulation programmed into the robot’s behavior was effective, we analyzed the videos of the interactions. Out of the 21 participants of the error condition, 18 exhibited clearly noticeable reactions upon the robot’s faults (e.g., laughing, looking up from the LEGO at the robot, annoyed facial expression). During the closing interview with the researcher, 15 of the 21 participants stated that they noticed the robot making errors. All three persons who had not shown reactions upon the robot’s errors in the video mentioned them in the interview. We, therefore, conclude that our manipulation was effective.
We used non-parametric statistical test procedures for data analysis, since our data were mostly not normally distributed (Kolmogorov–Smirnov test). Mann–Whitney-U tests were used to compare between two independent samples (between the two conditions and between the genders). Wilcoxon rank-sum tests were used to compare paired samples (ratings of the same scales before and after the interaction).
We coded the qualitative data from both interviews thematically (the one the robot conducted and the concluding interview after the interaction). We further annotated the video recordings from the participants’ interaction to investigate their social signals when experiencing an error situation with the robot. Figure 4 shows a participant interacting with the robot during the LEGO building session. The coding was performed from one of the authors since we coded objectively visible events only.
The gender distribution across conditions was roughly balanced. While 24 participants (15 males and 9 females) interacted with a flawless robot in the no error baseline condition, 21 participants (10 males and 11 females) were interviewed by an error-prone robot in the error experimental condition.
We explored if our participants’ personality influenced their rating of the robot by measuring five major personality traits. The scales of the BFI are constructed with semantic differential items that measure the participants’ position between two poles (e.g., 1—introvert to 5—extravert). The arithmetic mean of these items with no emphasis on either one of the poles is 3.
3.1.1.1. Scale Reliability
The subscales extraversion, neuroticism, and openness resulted in high reliability (Cronbach’s α = 0.82, 0.81, and 0.85). The reliability for the conscientiousness scale was acceptable (α = 0.71) and the one for agreeableness borderline acceptable (α = 0.61).
3.1.1.2. Participants’ Overall Personality
The results showed that the participants were slightly more extroverted (mean = 3.34, SD = 0.72), conscientious (mean = 3.42, SD = 0.57), and open (mean = 3.38, SD = 0.79) than the arithmetic mean. They were rather agreeable (mean = 3.79, SD = 0.47) and slightly less neurotic than average (mean = 2.91, SD = 0.73).
3.1.1.3. Participants’ Personality Compared between Conditions
We performed Mann–Whitney-U tests to explore if participants’ personality profile differed between conditions. The tests for all three subscales were non-significant, showing that participants’ personality profile did not differ between people who completed the error condition and people who completed the no error condition (U ≥ 235, z ≥ −0.388, p ≥ 0.553, r ≥ 0.03).
We measured people’s negative attitude toward robots for two reasons. First, we wanted to assess our participants’ general attitude. Therefore, we administered the NARS questionnaire before the participants’ interaction with the robot. Second, we assumed that participants’ attitude would be affected through the high number of errors. Therefore, we administered the questionnaire a second time, following the interaction. The individual NARS items range from 1—“I strongly disagree” to 5—“I strongly agree.”2 This means that low-scale values indicate that people have a more positive attitude toward robots and high-scale values denote a rather negative attitude.
3.1.2.1. Scale Reliability
We checked the reliability for all three subscales, before and after the interaction. The reliability for S1 before interaction resulted in borderline acceptable reliability (Cronbach’s α = 0.64), S1 after interaction in acceptable reliability (α = 0.74). The reliability for S2 before interaction was too low (α = 0.51). To increase reliability, we excluded item 2 (I feel that in the future society will be dominated by robots), and we recalculated the scale which resulted in borderline acceptable reliability (α = 62). S2 after interaction was recalculated accordingly after excluding item 2 (α = 0.77). S3 resulted in borderline acceptable reliability both before and after interaction (α before interaction = 0.62, after interaction = 0.67).
3.1.2.2. Participants’ Overall Negative Attitude toward Robots
While our participants’ rating for S2 and S3 resulted in a neutral standpoint, the rating for S1 showed that participants have a rather positive to neutral attitude toward interacting with robots (mean values before interaction are presented in Table 3).
Table 3. Mean values (SD) of the NARS questionnaire before and after the interaction (error and no error combined).
3.1.2.3. Participants’ Negative Attitude toward Robots Compared between Before and After Interaction
We were interested in investigating if our participants’ negative attitude toward robots was influenced by their interaction with the robot. We conducted Wilcoxon rank-sum tests to evaluate if the ratings differed significantly before and after the interaction. The results showed that there was no significant difference in NARS ratings before and after the interaction with the robot (S1: W = 248.00, z = −0.59, p = 0.558, r = −0.06; S2: W = 460.00, z = 1.66, p = 0.097, r = −0.18; and S3: W = 234.50, z = −1.81, p = 0.071, r = −0.19). The mean values for the three scales before and after the participants’ interaction with the robot are provided in Table 3.
3.1.2.4. Participants’ Negative Attitude toward Robots Compared between Conditions
We explored if participants’ rating after their interaction with the robot differed between the error and no error condition. We conducted Mann–Whitney-U tests for the scales completed after interaction. However, none of the scales resulted in significant differences between the conditions (S1: U = 277.50, z = 0.85, p = 0.395, r = 0.13; S2: U = 324.50, z = 1.66, p = 0.098, r = 0.25; and S3: U = 277.00, z = 0.58, p = 0.564, r = 0.09).
3.1.2.5. Participants’ Negative Attitude toward Robots Compared between the Genders
We performed Mann–Whitney-U tests to assess if the NARS ratings differed between male and female participants. The ratings for S2 and S3 (both before and after interaction) did not differ significantly. However, both ratings for S1 differed significantly between men and women (S1 before interaction: U = 419.50, z = 3.89, p = 0.000, r = 0.58 and S1 after interaction: U = 341.50, z = 2.41, p = 0.016, r = 0.36). This result yielded in a large (before) and medium (after) effect size. For an overview on the mean values refer to Table 4. Even though males and females rated their potential interaction with a robot as rather positive, male ratings are significantly more positive than those of the female participants.
We measured how people rated the likability, anthropomorphism, and perceived intelligence of the robot after interacting with it. To do so, we used the three corresponding subscales of the Godspeed questionnaire, each of which consists of five semantic differential items. These items measure the participants’ position between two poles. Therefore, the arithmetic mean of these items with no emphasis on either one of the poles is 3. The calculated likability score ranges from 1—“dislike” to 5—“like,” anthropomorphism from 1—“fake” to 5—“natural,” and perceived intelligence from 1—“incompetent” to 5—“competent”.
3.1.3.1. Scale Reliability
The anthropomorphism and perceived intelligence scales resulted in acceptable reliability (Cronbach’s α = 0.78 and 0.79) and likability in high reliability (α = 0.83).
3.1.3.2. Participants’ Overall Rating of the Robot
Our participants rated the robot less anthropomorphic than the arithmetic mean (mean = 2.16, SD = 0.74), slightly more intelligent (mean = 3.28, SD = 0.69), and considerably more likeable (mean = 4.10, SD = 0.63).
3.1.3.3. Participants’ Rating of the Robot Compared between Conditions
In order to explore if people who experienced erroneous robot behavior rated the robot differently from those participants who had interacted with a flawless robot, we conducted Mann–Whitney-U tests (see Table 5). While the mean ratings for anthropomorphism and perceived intelligence did not differ significantly between conditions, participants’ rating of the robot’s likability differed significantly between conditions. People who interacted with an erroneous robot liked the robot significantly more than people who interacted with a flawless robot. This difference yielded in a medium effect size.
3.1.3.4. Participants’ Rating of the Robot Compared between the Genders
We conducted further Mann–Whitney-U tests to detect potential differences in robot ratings between the genders. The tests showed that none of the three scales resulted in different ratings for male and female participants (anthropomorphism: U = 290.50, z = 0.93, p = 0.352, r = 0.14; likability: U = 317.50, z = 1.55, p = 0.121, r = 0.23; perceived intelligence: U = 323.00, z = 1.68, p = 0.094, r = 0.25). We further checked if our participants’ age, their preexperience with robots, and their technological affinity influenced how the robot was rated. None of these attributes resulted in significant differences.
Given our results, we can infer the following for our previously postulated hypotheses. Our participants liked the robot that made errors significantly more than the flawless robot which confirms our hypothesis 1. The hypotheses 2 and 3 have to be rejected since the robot committing errors did neither result in significantly higher anthropomorphism nor in significantly lower perceived intelligence ratings.
For the qualitative data analysis, we annotated the video recordings of the interview and LEGO sessions from the error condition. We hand coded the social signals the participants showed toward the robot, not toward the researcher, and which were objectively countable. Ambiguous events were discarded. For two of the participants, there was no video data due to technical problems from the recording equipment. The video data reported are based on the remaining 19 participants that completed the error condition. The data from the concluding interview were coded thematically in order to support our findings.
In this results section, we will report those findings from the qualitative data that are related to our research topic of robot errors.
3.2.1.1. Interview Session
NAO began the interview with asking the participants to state their definition of a robot. The majority of people provided a very technical definition: 17 people used the word machine, 10 the word device, and 10 referred to a robot as some other technical object. While 2 people directly referred to NAO as being a robot (“NAO, you are a robot.”), 4 participants used an “organic” noun (i.e., human, life form, and creature). However, they still used a technical adjective to further specify that noun (i.e., mechanical, artificial, electronic, and technical). Two participants provided unrelated answers.
We had the above question included in the robot’s questionnaire to gather people’s general standpoint on robots. Since most of the participants regarded a robot as a technical object, we assumed that they would want it to work reliably. In order to back our assumption up, the robot’s next question targeted the three most prominent qualities people attribute with a robot. Again, many participants listed technical terms (N = 24; e.g., mechanical, electronic, and programmed). While 11 participants attributed a practical quality to robots (e.g., helpful, efficient, and diligent), 3 people said robots were intelligent, and 6 people pointed out that robots are controlled by humans (e.g., there is human intelligence in the background, not very intelligent, no free will). As regards performance, 3 people referred to robots as precise/reliable, 1 participant said that robots would do what they are meant to, given they are programmed correctly, and only one person said that robots often make errors. This confirms our previous assumption that people assume robots to perform error-free.
The questions reported above were asked at the beginning of the interview. In order to make the participant familiar with the situation, no errors were included in here, irregardless of the condition (for a complete description of the user study procedure refer to Section 2.2). Therefore, the answers were not influenced by the fact that the robot made or did not make mistakes. The following questions, however, contained robot errors in the error condition.
Upon asking the participants which skills they would want a robot to have, 8 participants referred to robots as error-free (e.g., should do what people tell it to do, work reliably, and make no mistakes). Other skills included that the robot should be helpful and take on work that is too difficult/tedious/dangerous for humans (N = 13), it should be communicative and understand the human (N = 5), it should be easy to handle (N = 3), and it should be witty (N = 2).
3.2.1.2. LEGO Session
The robot asked the participants to express their current emotional state with LEGO bricks. The emotional state declarations were classified through lip and/or eyebrow shape (for an example see Figure 5). Most of the emotional state declarations were closely modeled to emoticons that are widely used in social media. Depictions that could not clearly be matched to an emotion were excluded (no data entries in Figure 6). No apparent difference in participants’ emotional state could be detected between the conditions. While the majority of participants was happy, only a few indicated a neutral expression. In the baseline condition, one participant reported a puzzled feeling and one felt silly. In the experimental condition, one participant indicated to be sad, one surprised. For an overview on all emotions refer to Figure 6.
Figure 5. An example of how the participants showed their current emotion to NAO during the LEGO session.
In the error condition, the robot failed to grasp the LEGO board that the participants were supposed to hand over. Since the participants were instructed to tell the robot to grasp, we wanted to know how often participants were willing to repeat their instructions. The number of expressed instructions (“grasp!”) ranged from 2 to 7 (mean = 4.16, SD = 1.21). This result lets us assume that people are to some extent patient with a faulty robot.
Upon placing an unusual request in the error condition, the participants’ willingness to comply was striking. A total of 17 participants threw LEGO blocks to the floor when asked to do so and 2 participants bent down and placed them on the floor, but no one refused to carry out the robot’s request. The fact that the participants complied with the robot’s unusual request links up with the research of Salem et al. (2015). The authors report that although people seemed to know that the robot’s request was not right (the researchers made the robot ask a number of unusual things of the participants, such as throwing someone’s personal mail in a garbage can), people complied as long as the action was not fatal and could be undone.
3.2.1.3. Social Signals
As we intended, the participants correctly interpreted the majority of social norm violations (SNV) and technical failures (TF) as error situations. The effectiveness manifests in the circumstance that most participants produced social signals when the robot made an error. Only the error where the robot waited for 15 s until it spoke was not recognized in 3 cases in the interview and in 7 cases in the LEGO session. The video footage showed that during the LEGO session, the participants were simply preoccupied with the previous task. This means that they were still dealing with the LEGO bricks (e.g., disassembling, counting, assembling, etc.) and, thus, did not pay attention to the robot’s long silence. During the interview session, three participants were more patient than the rest of our sample and just waited for the robot to continue. The SNV in the interview session where the robot cut the participant off did not work in one case. This participant provided such a short but coherent answer that he was finished by the time the robot started speaking.
Each of the 19 participants experienced 8 error situations, which results in 152 error situations. From those, 11 were not recognized as error (see above) and in 19 cases, the participants did not show a reaction toward the robot. This leaves us with 122 error situations in which the participants showed 1 or more social signals (maximum 5). See Table 6 for an overview on the mean number of social signals per error situation.
The mean number of social signals expressed during a SNV is 1.36 (SD = 0.56) and during a TF 1.53 (SD = 0.72). A Kolmogorov–Smirnov test for normality over the differences of the variable scores indicated that the data are normally distributed (D(19) = 0.131, p = 0.200). We performed a paired-samples t-test and found that the amount of social signals the participants produced did not differ significantly between SNV and TF (t(18) = −1.112, p = 0.281, d = 0.27). Table 7 gives an overview on how many social signals were made for each category in each type of error situation. The table also shows which kinds of social signals were grouped in the categories. Our analysis contains only social signals that were made toward the robot. Signals toward the present experimenters were not included in our analysis (e.g., verbal statements to the experimenter, head turns in the direction of the experimenter). We hand coded the data by counting the objectively perceivable events. Thereby, we distinguished a head tilt (head moves sideways with gaze staying in place) from a shift in gaze (the participant’s gaze shifts visibly from, e.g., the robot to the LEGO parts). Head turns (head movements with the gaze leaving the scene) were all directed toward the present experiment and, thus, disregarded.
A Kolmogorov–Smirnov test for normality over the frequency differences of the variable scores for the speech category indicated that the data deviate from normal distribution (D(19) = 0.250, p = 0.003). Therefore, we performed Wilcoxon signed-rank tests to assess the differences in frequencies for each category. Table 8 provides an overview on the mean number of social signal of each category per error situation type. The results show that during technical failures people made significantly more facial expressions, head movements, body movements, and gaze shifts.
After the participants finished interacting with the robot and after they completed the postinteraction questionnaires (NARS after interaction and Godspeed), they were asked four open-ended questions in the final interview. While the questions 1–3 asked about some general aspects of the participants’ impression of the interaction and the robot, question 4 specifically targeted the robot’s errors (see Section 2.5 for the specific questions). Therefore, question 4 was only asked for participants in the error condition. The resulting data were analyzed through an affinity diagram (Holtzblatt et al., 2004). An affinity diagram is a method for organizing ideas, challenges, and solutions into a wall-sized hierarchical diagram.
In question 1, participants were asked to report anything particular they had noticed during their interaction with the robot. Here, 12 participants reported that the robot had made some mistakes (e.g., it went in a loop; it cut my word). The participants’ answers to question 2 did not include any mentions about the robot’s mistakes. In question 3, 7 participants reported that they would like to change the faulty robot behavior (e.g., fix the technical bugs; it does not leave time for you to respond; loops).
With the final question in the interview, we specifically targeted the robot’s errors, in asking what the participants thought of the robot making mistakes. While 7 participants uttered specifically negative aspects (e.g., unpleasant; confusing; that’s just what one would expect from technology; I was unsure if the interaction had stopped; I thought I had made a mistake), 10 participants uttered positive feelings when asked about the fact that the robot made mistakes (e.g., funny; friendly; it was great that the robot did not make it look like I made a mistake; I do not like it less because of the mistakes; it would be scary if all went smooth because that would be too human-like).
Our results showed that the participants liked the faulty robot significantly more than the flawless one. This finding confirms the Pratfall Effect, which states that people’s attractiveness increases when they make a mistake as shown by Aronson et al. (1966). Therefore, the psychological concept can successfully be transferred from interpersonal interaction to HRI. Upon the attempt of including socially acting robots into this concept, we can extend it to: “Imperfections and mistakes carry the potential of increasing the likability of any social actor (human or robotic).” The same effect was previously researched by Salem et al. (2013), where incongruent behavior of a robot can be seen as a social norm violation as such behavior violates participants’ expectations from a social script. To overcome this error situation, participants changed their social signals, but on the other hand they rated the likability of the robot higher. Similarly, Ragni et al. (2016) showed that the participants in their study enjoyed the interaction with the faulty robot significantly more, than the participants who had interacted with a flawless robot. On the other hand, their participants who had interacted with the faulty robot, rated it less intelligent, less competent, and less superior, which again confirms the Pratfall Effect.
The repeated evidence of this phenomenon existing in HRI strengthens our argument to create robots that do not lead to believe they perform free from errors. We recommend that robot creators design social robots with their potential imperfections in mind. We see two sources for these imperfections that link back to the two error types found in HRI. On one hand, creators of social robots should follow the notions of interpersonal interaction to meet the expectations humans have about social actors and with it socially interacting robots. On the other hand, it is advisable to embrace the imperfections of robot technology. Technology that is created with potential shortcomings in mind can be designed to include methods for error recovery. Therefore, one way to go here would be to make robots understand they made an error by correctly interpreting the human’s social signals and indicate their understanding to the human user. Both of these sources of imperfections will lead to more believable robot characters and more natural interaction. Of course, this applies to social robots operating in non-critical environments. Safety-relevant applications and scenarios must under all circumstances operate at zero-defect level.
Interestingly, we could not find a comparable effect for anthropomorphism in our data. The robot’s anthropomorphism level was rated similar, irregardless of the fact if the robot made errors or not. Our result is different from the findings of Salem et al. (2013), who also used a human-like robot, and where the participants rated the faulty robot more anthropomorphic as the flawless one. The researchers used coverbal gestures, while we programmed the robot to provide mostly random gestures to make it appear more life-like. This might have in general diminished the effect of anthropomorphism in our setup (which is indicated by the low overall anthropomorphism level). However, more research is required to further explore the role of anthropomorphism in faulty robot behavior.
Contrary to our assumption, the faulty robot was not rated as less intelligent than the flawless one. This seems striking since the robot made several errors over a relatively short interaction time. Furthermore, most participants had noticed the robot making errors, while, at the same time, they had indicated to regard a robot as something very technical that should perform reliably. One potential explanation could be the fact that the induced errors were non-task-related. Follow-up research is required to further explore the perceived intelligence of erroneous robot behavior.
Upon asking the participants about their current emotional state, the majority of participants showed the robot that they were happy. The participants were also quite patient and tried handing the object several times, when the robot failed grasping it. All of these observations point toward the notion that a faulty social robot is a more natural social robot. In our future research on this topic, we will extend our approach to include more user experience measures to get a more profound understanding on the users’ perception of the robot. For example, it will be interesting to further investigate possible impacts on subjective performance and acceptance.
Our data showed that when people interacted with a social robot that made an error, they were likely to show social signals in response to that error. In our previous research, we performed an analysis of video material in which robot errors occurred unintentionally and we found that users showed social signals in about half the interactions (Mirnig et al., 2015). In the herein reported study, however, most participants showed at least one social signal per error situation. We explain this difference in part with the high error rate (8 errors in an average total interaction time of about 12 min). Users seem to anticipate the robot making more errors once they experienced it is not flawless and responded more frequently with social signals. The reason for the increased number of social signals could also be based on the size of the robot. While the majority of interactions from the previous study were with a human-sized robot at eye level, the robot in our case was small and placed slightly below participants’ eye level. This aspect remains to be studied further.
With our results we show again that humans respond to a robot’s error with social signals. Therefore, recognizing social signals might help a robot to understand that an error happened. According to the frequencies of occurrence, gaze shifts and smile/laughter carry most potential for error detection, which is in line with our previous findings in the study of Giuliani et al. (2015). Upon a detailed analysis on the categories of social signals we found that people make significantly more gaze shifts during technical failures. This result is in contrast to our previous findings where significantly more gaze shifts were made during social norm violations. We take from this that gaze shifts are a potential indicator for robot errors, but it remains to be studied if they can be used to distinguish between the two error types.
We also found that people made significantly more facial expressions, head-, and body movements during technical failures. The increase in social signals during technical failures may be rooted in the circumstance that the technical failures were more obvious in the present user study. For example, in the video material from the previous study the robot failed to grasp an object that was placed in front of it. In our setup, the robot failed to grasp an object that the participant handed to it, which made the participant more actively perceive the robot’s error.
Contrary to our previous findings, we did not detect significant differences in spoken social signals. This could be grounded in the fact that due to the setup, the robot had in general a much larger share in spoken utterances.
In response to the robot’s unusual request, most users showed social signals. The kind of signals (gaze shifts and laughter) displayed the users’ slight discomfort and provided evidence that they knew the robot’s request implied a deviation from the social script of the situation. However, most users nevertheless followed the robot’s order and threw the LEGO blocks to the floor. In addition to the previous results as reported in the study of Mirnig et al. (2015), this result provides further evidence that users show specific social signals in response to robot errors. Future research should be targeted at making a robot understand the signals and make sense of them. A robot that can understand its human interaction partner’s social signals will be a better interaction partner itself and the overall user experience will improve.
Since most of our participants had not interacted with a robot before, a potential novelty denotes a certain limitation to our results. Some participants were probably captivated with the technology, which made them remain patient. It remains to be studied how such novelty wears off over time and how this influences people’s willingness to interact. It will, furthermore, be interesting to assess the dimensions of faults. That is, how extensive can an error become until it becomes a deal-breaker. Ragni et al. (2016) already provided evidence that erroneous robot behavior decreases performance of a human interacting with the robot. It could also be interesting to explore how users react in case of the robot giving ambiguous information. Further aspects of robot errors that are worthwhile exploring are, for example, the following. What kinds of errors are forgivable and which ones are not? What is the threshold for error rate or number of errors until the participants’ patience is over or performance drops considerably? A lot more specific research is required to understand and make use of the effects of errors in social HRI.
With our user study we explored how people rated a robot making errors in comparison to a perfectly performing robot. We measured the robot’s likability, anthropomorphism, and perceived intelligence. We found that the faulty robot was rated as more likeable, but neither more anthropomorphic nor less intelligent. We recommend robots to be designed with their possible shortcomings in mind as we believe that this will result in more likeable social robots. Similar to interpersonal interaction, imperfections might even have a positive influence in terms of likability. We expect social HRI that embraces the imperfectness of today’s robots to result in more natural interaction and more believable robot characters.
Our results confirm existing HRI research on robot likability such as the studies of Salem et al. (2013) and Ragni et al. (2016), hinting at error-prone robots supposedly resulting in more believable robots. Our work successfully proves the existence of the psychological concept Pratfall Effect in HRI and suggests that it should be our community’s aim to bear potential shortcomings of social robots in mind when creating them. The nature and extent of errors that can be handled through the interactional design remain yet to be studied.
With our results we could again show that humans respond to faulty robot behavior with social signals. A robot that can recognize these social signals can, in subsequence, understand that an error happened. We detected gaze shifts and laughter/smiling as the most frequently shown social signals, which is in line with our previous research.
We see the following next steps to the ambitious goal of creating social robots that are able to overcome an error situation. First, it needs to be studied how we can let robots understand that an error occurred. Second, robots must be enabled to communicate about such errors. Third, robots need to know how to behave in an error situation in order to effectively apply error recovery strategies.
This study was carried out in accordance with the ethical regulations of conducting user studies at the University of Salzburg. The entire process was supervised, and the protocol was approved by the department director, Prof. Manfred Tscheligi. Each of our participants was given information about the study process beforehand, including the information that it was possible to quit participating at every point in time. Every participant gave their written informed consent in accordance with the Declaration of Helsinki.
NM is the main author of this article who provided the storyline and most of the text is written by her. She was the responsible supervisor of the user study and she performed the data analysis and statistics. GS contributed to the setup of the user study, he assisted with writing and the storyline, and he contributed to data analysis. MM recruited participants and performed the user study. SS provided input on the related work. She provided Figure 3 and she helped with formatting the tables. MG provided related work and he contributed to the overall storyline. MT was supervising the user study and writing processes.
The authors declare that the research was conducted in the absence of any commercial or financial relationships that could be construed as a potential conflict of interest.
The authors of this paper would like to thank Michael Miksch for his contribution in performing the user study.
We gratefully acknowledge the financial support by the Austrian Federal Ministry of Economy, Family and Youth and the National Foundation for Research, Technology and Development (Christian Doppler Laboratory for “Contextual Interfaces”). This work was additionally funded in part by the European Commission in the project ReMeDi (Grant No. 610902). We acknowledge financial support by the Open Access Publication Fund of the University of Salzburg.
Aronson, E., Willerman, B., and Floyd, J. (1966). The effect of a pratfall on increasing interpersonal attractiveness. Psychon. Sci. 4, 227–228. doi: 10.3758/BF03342263
Bartneck, C., Kulić, D., Croft, E., and Zoghbi, S. (2009). Measurement instruments for the anthropomorphism, animacy, likeability, perceived intelligence, and perceived safety of robots. Int. J. Soc. Robot. 1, 71–81. doi:10.1007/s12369-008-0001-3
Brooks, D. J., Begum, M., and Yanco, H. A. (2016). “Analysis of reactions towards failures and recovery strategies for autonomous robots,” in Proceedings of the IEEE International Symposium on Robot and Human Interactive Communication (RO-MAN 2016) (New York, NY: IEEE), 487–492.
Bruckenberger, U., Weiss, A., Mirnig, N., Strasser, E., Stadler, S., and Tscheligi, M. (2013). “The good, the bad, the weird: audience evaluation of a “real” robot in relation to science fiction and mass media,” in Proceedings of the International Conference on Social Robotics (Bristol, UK: Springer), 301–310.
Gehle, R., Pitsch, K., Dankert, T., and Wrede, S. (2015). “Trouble-based group dynamics in real-world HRI—reactions on unexpected next moves of a museum guide robot,” in Proceedings of the International Symposium on Robot and Human Interactive Communication (Kobe: IEEE), 407–412.
Giuliani, M., Mirnig, N., Stollnberger, G., Stadler, S., Buchner, R., and Tscheligi, M. (2015). Systematic analysis of video data from different human-robot interaction studies: a categorisation of social signals during error situations. Front. Psychol. 6:931. doi:10.3389/fpsyg.2015.00931
Gompei, T., and Umemuro, H. (2015). “A robot’s slip of the tongue: effect of speech error on the familiarity of a humanoid robot,” in Proceedings of the International Symposium on Robot and Human Interactive Communication (Kobe: IEEE), 331–336.
Hayes, C. J., Maryam, M., and Riek, L. D. (2016). “Exploring implicit human responses to robot mistakes in a learning from demonstration task,” in Proceedings of the International Symposium on Robot and Human Interactive Communication (New York, NY: IEEE), 246–252.
Holtzblatt, K., Wendell, J. B., and Wood, S. (2004). Rapid Contextual Design: A How-to Guide to Key Techniques for User-Centered Design. San Francisco, CA: Elsevier.
John, O. P., Naumann, L. P., and Soto, C. J. (2008). “Paradigm shift to the integrative big-five trait taxonomy: history, measurement, and conceptual issues,” in Handbook of Personality: Theory and Research, eds O. P. John, R. W. Robins, and L. A. Pervin (New York, NY: Guilford Press), 114–158.
Knepper, R. A., Tellex, S., Li, A., Roy, N., and Rus, D. (2015). Recovering from failure by asking for help. Auton. Robots 39, 347–362. doi:10.1007/s10514-015-9460-1
Lee, M. K., Kielser, S., Forlizzi, J., Srinivasa, S., and Rybski, P. (2010). “Gracefully mitigating breakdowns in robotic services,” in Proceedings of the 5th ACM/IEEE International Conference on Human-Robot Interaction (Osaka: IEEE Press), 203–210.
Lohse, M. (2011). The role of expectations and situations in human-robot interaction. New Front. Hum. Robot Interact. 2, 35–56. doi:10.1075/ais.2.04loh
Mirnig, N., Giuliani, M., Stollnberger, G., Stadler, S., Buchner, R., and Tscheligi, M. (2015). “Impact of robot actions on social signals and reaction times in HRI error situations,” in Proceedings of the International Conference on Social Robotics (Paris: Springer), 461–471.
Nomura, T., Kanda, T., Suzuki, T., and Kato, K. (2004). “Psychology in human-robot communication: an attempt through investigation of negative attitudes and anxiety toward robots,” in Proceedings of the International Symposium on Robot and Human Interactive Communication (Kurashiki: IEEE), 35–40.
Ragni, M., Rudenko, A., Kuhnert, B., and Arras, K. O. (2016). “Errare humanum est: erroneous robots in human-robot interaction,” in Proceedings of the IEEE International Symposium on Robot and Human Interactive Communication (RO-MAN 2016) (New York, NY: IEEE), 501–506.
Robinette, P., Wagner, A. R., and Howard, A. M. (2014). “Assessment of robot guidance modalities conveying instructions to humans in emergency situations,” in Robot and Human Interactive Communication, 2014 RO-MAN: The 23rd IEEE International Symposium on, (Edinburgh, UK: IEEE), 1043–1049.
Salem, M., Eyssel, F., Rohlfing, K., Kopp, S., and Joublin, F. (2013). To err is human (-like): effects of robot gesture on perceived anthropomorphism and likability. Int. J. Soc. Robot. 5, 313–323. doi:10.1007/s12369-013-0196-9
Salem, M., Lakatos, G., Amirabdollahian, F., and Dautenhahn, K. (2015). “Would you trust a (faulty) robot? Effects of error, task type and personality on human-robot cooperation and trust,” in Proceedings of the Tenth Annual ACM/IEEE International Conference on Human-Robot Interaction (Portland, OR: ACM), 141–148.
Schank, R., and Abelson, R. (1977). Scripts, Plans, Goals and Understanding: An Inquiry into Human Knowledge Structures, Vol. 2. Hillsdale, NJ: Lawrence Erlbaum Associates.
Short, E., Hart, J., Vu, M., and Scassellati, B. (2010). “No fair!!: an interaction with a cheating robot,” in Proceedings of the 5th ACM/IEEE International Conference on Human-robot Interaction, HRI’10 (Piscataway, NJ: IEEE Press), 219–226.
Keywords: social human–robot interaction, robot errors, user experience, social signals, likeability, faulty robots, error situations, Pratfall Effect
Citation: Mirnig N, Stollnberger G, Miksch M, Stadler S, Giuliani M and Tscheligi M (2017) To Err Is Robot: How Humans Assess and Act toward an Erroneous Social Robot. Front. Robot. AI 4:21. doi: 10.3389/frobt.2017.00021
Received: 30 December 2016; Accepted: 10 May 2017;
Published: 31 May 2017
Edited by:
Ginevra Castellano, Uppsala University, SwedenReviewed by:
Rodolphe Gelin, Aldebaran Robotics, FranceCopyright: © 2017 Mirnig, Stollnberger, Miksch, Stadler, Giuliani and Tscheligi. This is an open-access article distributed under the terms of the Creative Commons Attribution License (CC BY). The use, distribution or reproduction in other forums is permitted, provided the original author(s) or licensor are credited and that the original publication in this journal is cited, in accordance with accepted academic practice. No use, distribution or reproduction is permitted which does not comply with these terms.
*Correspondence: Nicole Mirnig, bmljb2xlLm1pcm5pZ0BzYmcuYWMuYXQ=
Disclaimer: All claims expressed in this article are solely those of the authors and do not necessarily represent those of their affiliated organizations, or those of the publisher, the editors and the reviewers. Any product that may be evaluated in this article or claim that may be made by its manufacturer is not guaranteed or endorsed by the publisher.
Research integrity at Frontiers
Learn more about the work of our research integrity team to safeguard the quality of each article we publish.