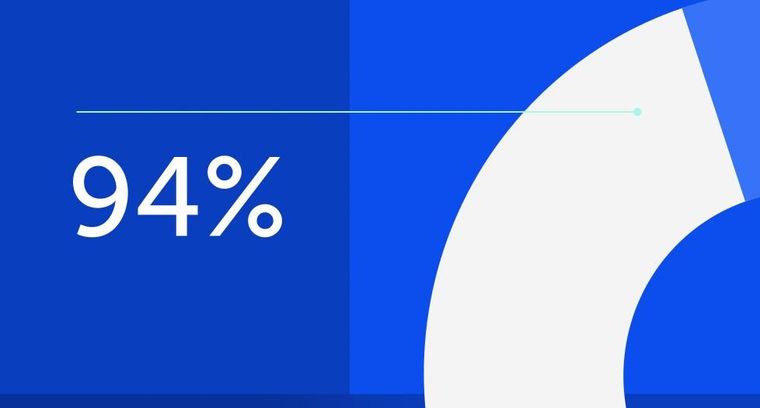
94% of researchers rate our articles as excellent or good
Learn more about the work of our research integrity team to safeguard the quality of each article we publish.
Find out more
ORIGINAL RESEARCH article
Front. Robot. AI, 23 May 2017
Sec. Humanoid Robotics
Volume 4 - 2017 | https://doi.org/10.3389/frobt.2017.00016
This article is part of the Research TopicAffective and Social Signals for HRIView all 14 articles
Humanoid robot avatars are a potential new telecommunication tool, whereby a user is remotely represented by a robot that replicates their arm, head, and possible face movements. They have been shown to have a number of benefits over more traditional media such as phones or video calls. However, using a teleoperated humanoid as a communication medium inherently changes the appearance of the operator, and appearance-based stereotypes are used in interpersonal judgments (whether consciously or unconsciously). One such judgment that plays a key role in how people interact is personality. Hence, we have been motivated to investigate if and how using a robot avatar alters the perceived personality of teleoperators. To do so, we carried out two studies where participants performed 3 communication tasks, solo in study one and dyadic in study two, and were recorded on video both with and without robot mediation. Judges recruited using online crowdsourcing services then made personality judgments of the participants in the video clips. We observed that judges were able to make internally consistent trait judgments in both communication conditions. However, judge agreement was affected by robot mediation, although which traits were affected was highly task dependent. Our most important finding was that in dyadic tasks personality trait perception was shifted to incorporate cues relating to the robot’s appearance when it was used to communicate. Our findings have important implications for telepresence robot design and personality expression in autonomous robots.
Telecommunication is omnipresent in today’s society, with people desiring to be able to communicate with one another, regardless of distance, for a variety of social and practical reasons. While video-enabled communication offers a number of benefits over voice-only communication, it is still lacking compared to face-to-face interactions (Daly-Jones et al., 1998). For example, remotely located team members are less included in cooperative activities than colocated team members (Daly-Jones et al., 1998) and have fewer conversational turns and speaking time in group conversations (O’Conaill et al., 1993). Suggested reasons for these disparities are a lack of social presence of these remote group members, reduced engagement, and reduced awareness of actions (Tang et al., 2004). A suggested underlying cause for the disparities found in traditional telecommunication is a lack of physical presence. An alternative is the use of teleoperated robots as communication media. A common approach to such embodied telecommunication is the use of mobile remote presence (MRP) devices: a screen displaying the operators face mounted on a stalk attached to a wheeled base (Kristoffersson et al., 2013). Though studies examining the utility of MRPs have found that there are some improvements in social presence, different social norms are observed when people use them to interact, and there are impacts on trust and rapport (Lee and Takayama, 2011; Rae et al., 2013). Further, such systems are not able to effectively transmit non-verbal communication cues, a key element of human communication not only for information conveyance but also in maintaining engagement and building rapport (Salam et al., 2016).
A proposed method for further improving social presence and effectively transmitting body language is to use a humanoid robot as a communication medium. In such a system, the operator’s body language is duplicated on a humanoid robot such that it is comprehensible and highly salient (Bremner and Leonards, 2016; Bremner et al., 2016b). Using a humanoid robot as a communications avatar has benefits with regard to engagement of conversational partners (Hossen Mamode et al., 2013), social presence (Adalgeirsson and Breazeal, 2010), group interaction (Hossen Mamode et al., 2013), and trust (Bevan and Stanton Fraser, 2015).
However, when using a robot as a remote proxy for communication, the operator is represented with a different physical appearance, much as computer generated avatars do in virtual environments. Appearance has been observed to be utilized in making interpersonal judgments (Naumann et al., 2009), and this can extend to virtual avatars (Wang et al., 2013; Fong and Mar, 2015). It was observed that judges made relatively consistent inferences based on avatar appearance alone (Wang et al., 2013; Fong and Mar, 2015), and more attractive avatars were rated more highly in an interview scenario (Behrend et al., 2012). How this might manifest with robot avatars, in particular in the interaction between a robot appearance and human voice communication, remains unclear and is yet to be explored.
Here, the particular judgment we are concerned with is that of personality perception, an important facet of communication. Researchers in psychology have shown that personality plays a key role in forming interpersonal relationships, and predicting future behaviors (Borkenau et al., 2004). These findings have motivated a significant body of work for how people judge others’ personalities based on their observable behaviors. A key component of these social cues for personality are non-verbal behaviors. We aim to investigate if such non-verbal personality cues transmitted by a teleoperated humanoid robot continue to be utilized in personality judgments, and how they interact with verbal cues. Non-verbal cues can be transmitted as our robot teleoperation system utilizes a motion capture-based approach so that arm and head movements the operator performs while talking are recreated with minimal delay on a NAO humanoid robot (Bremner and Leonards, 2016). The control system is intuitive and immersive, and we observe people behaving similarly to how they do face-to-face (Bremner et al., 2016b).
We designed two experiments which follow an experimental methodology common in the personality analysis literature, i.e., videos of participants performing different communication tasks are shown to external observers (judges) for personality assessment (e.g., Borkenau et al. (2004)). Personality judgments are made on the so-called big five traits, extroversion, conscientiousness, agreeableness, neuroticism, and openness (multiple questions relate to each trait). We varied communication media between judges, either video only or robot mediated (also recorded on video). Two main measures are used to see whether there was an effect of communication condition on personality judgments: (1) judge consistency in how they evaluate a given trait, both within and between judge (low consistency indicates lack of cues or conflicting cues); and (2) personality shifts between high and low classification for each trait between the video and robot conditions.
Hence we address the following research questions:
• RQ1: Are there differences in judges’ consistency in assessing personality traits (within-judge consistency)?
• RQ2: Are there differences in how much judges agree with one another on personality judgments (between-judge consistency)?
• RQ3: Are personality judgments less accurate compared to self-ratings (self-other agreement)?
• RQ4: Are perceived personalities systematically shifted to incorporate characteristics associated with the robot’s appearance (personality shifts)?
This paper is an extended version of our work published by Bremner et al. (2016a). We extended our previous work by adding a second experiment that refined our experimental procedure and used dyadic rather than solo tasks. Our discussions and conclusions are extended to include both experiments, evaluating all our results to give a clearer picture.
In the first experiment, three tasks are performed direct to camera, i.e., solo tasks. In the second experiment, participants performed three tasks that involved interaction with a confederate, i.e., dyadic. The first experiment provided some limited evidence for shifts in personality perception. Further, by adding an audio-only communication condition, we were able to show that the robot was not simply ignored, and gesture cues performed on the robot were utilized. An important finding from the first experiment was that effects were very task dependent, as the literature suggested. Borkenau et al. (2004) found that openness is better inferred in more ability-demanding tasks such as pantomime task. Hence, the second experiment used additional tasks, which by being dyadic will engender personality cues differently; it is also a refinement of our experimental procedure, improving the reliability of our results. It produced compelling evidence that cues related to the robot’s appearance were incorporated in personality judgments, causing consistent shifts in perceived personality.
A common approach to investigating personality judgments is first impression or thin slice personality analysis. It is a body of research that studies the accuracy with which people are able to make personality judgments of others based only on short behavioral episodes (termed thin slices). This approach is taken as it is believed that these judgments provide insight into the assessments people make in everyday interactions (Funder and Sneed, 1993; Borkenau et al., 2004). In such studies, targets are typically asked to perform a range of communication tasks, either solo performances to camera or dyadic with confederates, and are filmed while doing so. Judges then observe the video clips and complete personality assessment questionnaires. Ratings of judges are compared with target self-ratings, acquaintance ratings, and for inter-judge agreement. For many traits, there is sufficient inter-judge agreement for the method to be useful in assessing the impressions a person creates on those they interact with (Borkenau et al., 2004); however, the accuracy of judge ratings to self/acquaintance ratings is typically a lot lower, as self/acquaintance ratings are error prone, and use different sources to make their judgments (Vinciarelli and Mohammadi, 2014).
Often analyzed in thin slice personality studies are the cues that appear to be utilized in people making their judgments. Appearance, speaking style, gaze, head movements, and hand gestures have been frequently reported to be significant predictors of personality (Riggio and Friedman, 1986; Borkenau and Liebler, 1992; Borkenau et al., 2004). Indeed, this sort of analysis forms the basis for automated personality analysis systems. Aran and Gatica-Perez (2013) focused on personality perception in a small group meeting scenario. They extracted a set of multimodal features including speaking turn, pitch, energy, head and body activity, and social attention features. Thin slice analysis yielded the highest accuracy for extroversion, while openness was better modeled by longer time scales. With regard to the related work in personality computing, the closest approach was presented in the study by Batrinca et al. (2016). In order to analyze the Big Five personality traits, Batrinca et al. conducted a study where a set of participants were asked to interact with a computer, which was controlled by an experimenter, and then a different set of participants were asked to interact with the experimenter face-to-face to collaborate on completing a map task. In order to elicit the participants’ personality traits, the experimenter exhibited four different levels of collaborative behaviors from fully collaborative to fully non-collaborative. Self-reported personality traits were used to study the manifestation of traits from audiovisual cues. In the human-machine interaction setting, their results showed that (1) extroversion and neuroticism can be predicted with a high level of accuracy, regardless of the collaboration modality; (2) prediction of the agreeableness and conscientiousness traits depends on the collaboration modality; and (3) openness was the only trait that cannot be modeled. In contrast to their findings in the human–machine interaction setting, they showed that openness was the trait that can be predicted with highest accuracy in the human–human interaction setting.
Applying such personality perception analysis to robot teleoperators has so far been limited. Perception of teleoperator’s personality is important not only in social interactions but is also crucial where teleoperated robots are used in a service capacity such as for elderly care (Yamazaki et al., 2012), and search and rescue (Martins and Ventura, 2009). In these settings, perception of the operator will effect system utility for carrying out the desired service and achieving the desired outcome. In the study by Celiktutan et al. (2016), we showed that many of the aforementioned personality cues can be transmitted by a telepresence robot. We trained support vector machine classifiers with a set of features extracted from participants’ voice and body movements. We found that the use of a robot avatar helps to discriminate between different personality types (e.g., extroverted vs.introverted) better than audio-only mediated communication for extroversion (65%) and conscientiousness (60%).
Studies with Mobile Remote Presence devices (MRPs) have briefly mentioned perceiving the operator’s personality (Lee and Takayama, 2011), but it has not been deliberately studied as we do here. There are two studies that look directly at personality perception of teleoperators. Kuwamura et al. (2012) examined an effect that they term personality distortion, demonstrated by reduction in internal consistency of the personality questionnaire they used, for two different robot platforms and communication using video. They use 3 tasks: (1) an experimenter talks freely with the participant, (2) a different experimenter introduces and talks about themselves, and (3) a third experimenter interviews the participant. They only observed personality distortion for one of the robot platforms, for extroversion in the interview task, and for agreeableness in the introduction task. Using a single fixed person for each task, particularly members of the experimental team who are aware of the goals of the study, greatly reduces the ecological validity of their results. In contrast, here we use a large number of naïve targets performing naturalistic communication, and conduct far more in-depth data analysis.
In a study with a teleoperated, highly humanlike robot, Straub et al. (2010) examined both how participant teleoperators incorporate the fact that they are operating a robot into their presented identity, and how interlocutors at the robot’s location blend operator and robot identities. They used language analysis to make their assessments. They observed that many operators pretended they themselves were a robot, and interlocutors often referred to the operator as a robot. These behaviors are different from what we typically observe with our teleoperation system, where most operators appeared to act naturally as themselves (Bremner et al., 2016b).
We designed a two-stage experimental method for assessing changes in perceived personality that we used in two studies. First, a set of participants (targets) were recorded performing three communication tasks in two conditions, directly visible on video camera (audiovisual condition) and communicating using the teleoperated robot (teleoperated robot condition, also recorded on camera). This ensures that we have a large set of natural communication behaviors, and hence personality cues, for a range of personality types, that can be viewed directly or when mediated by a robot.
In the second stage of the study, the recorded data were used to create a set of video clips for each target in each communication condition. The video clips were pseudorandomly assigned to a set of surveys in such a way as to have one of each task and communication condition combinations present, with a given target only appearing once in a given survey (i.e., communication condition was varied between surveys). Each survey was viewed by a set of 10 judges, who after watching each clip assessed the personality of that target. We used an online crowdsourcing service to have the clips assessed. Employing judges via online crowdsourcing services has recently gained popularity due to its efficiency and practicality as it enables collecting responses from a large group of people within a short period of time (Biel and Gatica-Perez, 2013; Salam et al., 2016).
Personality was assessed by a questionnaire that aims to gather an assessment along the widely known Big Five personality traits (Vinciarelli and Mohammadi, 2014). These five personality traits are extroversion (EX—assertive, outgoing, energetic, friendly, socially active), agreeableness (AG—cooperative, compliant, trustworthy), conscientiousness (CO—self-disciplined, organized, reliable, consistent), neuroticism (NE—having tendency to negative emotions such as anxiety, depression, or anger), and openness (OP—having tendency to changing experience, adventure, new ideas). Each trait is measured using a set of items (the BFI-10 (Rammstedt and John, 2007) with 2 per trait in the Solo Tasks Study, and the IPIP-BFM-20 (Topolewska et al., 2014) with 4 per trait in the Dyadic Tasks Study) scored on 10-point Likert scales. As well as being assessed by external observers, each target completed the personality questionnaire for self-assessment.
In order to reproduce the gestures of targets on the NAO humanoid robot platform from Softbank Robotics (Gouaillier et al., 2009), we used a motion capture-based teleoperation system. Previously we have demonstrated the system to be capable of producing comprehensible gestures (Bremner and Leonards, 2015, 2016). The arm motion of the targets is recorded using a Microsoft Kinect and Polhemus Patriot,1 and used to produce equivalent motion on the robot. Arm link end points at the wrist, elbow, and shoulder are tracked and were used to calculate joint angles for the robot so that its upper and lower arm links reproduce human arm link positions and motion. This method ensures that joint coordination, and hand trajectories are as similar as possible between the human and the robot within the constraints of the NAO robot platform. Figure 1 shows a gesture produced by one of the targets, and the equivalent gesture on the NAO.
Figure 1. Snapshots from the Solo Tasks Study. Left hand side: a target perceived to be extroverted by judges. Right hand side: a target perceived to be introverted by judges.
In the first study, the three tasks performed by participants involved them performing directly to the camera, i.e., solo, and were based upon a subset of tasks used by Borkenau et al. (2004). Each of the tasks was framed as an interaction with the experimenter who stood beside the video camera used in the recordings, and provided non-verbal feedback and prompt questions to ensure as natural communicative behaviors as possible. Targets were instructed to speak for as long as they felt able, with a maximum time of 2 min for each task. The majority of the targets talked for 30–60 s on each task, with occasional prompts for missing information. Prior to performing tasks, we asked the targets to introduce themselves and give some information about themselves, e.g., where they work, what they do, their family, etc. This stage was purely to help naturalize the target to the experimental setting. It was not used to produce clips for judge rating.
3.2.1.1. Task 1 (Hobby)
This task asked targets to describe one of their hobbies, providing as much detail as possible. Suggested detail included what their hobby involves, why they like it, how long have they been doing it for, etc. Example personality cues we anticipated from this task include what targets have as their hobby, and what detail and the depth of detail they provide while describing their hobby.
3.2.1.2. Task 2 (Story)
This task is based on Murray’s thematic apperception test (TAT), where the target is shown a picture and is asked to tell a dramatic story based on a picture (Murray, 1943). They are asked what is happening in the picture,2 what are the characters thinking and feeling, what happens before the events in the picture and what happens after. The picture is purposely designed to be ambiguous so that the target has the scope to interpret the picture as they see fit, and has to be creative in their story telling. It is a projective test, where the details given by the target, and how they relate the actions of the characters, provide cues about their personality.
3.2.1.3. Task 3 (Mime)
This task required the targets to mime preparing and cooking a meal of their choice. This was different from the mime task used by Borkenau et al. (2004), where targets had to mime alternative uses for a brick. Our pretests showed little variability between targets for that task. Instead, the chosen task gave the desired variability, and the gestures were better suited to performance on the NAO robot. Which meal was selected, and the complexity of the mime, are example personality cues we anticipated from this task.
Twenty-six participants were recorded as targets (16 female, mean age = 30.85, SD = 7.58) and gave written informed consent for their participation, they were reimbursed with a £5 gift voucher for their time. Recordings for 20 of the targets were used to create the clips used for judgments (6 targets were omitted due to recording problems). The study was approved by the ethics committee of the Faculty of Environment and Technology of The University of the West of England.
Clip ratings were undertaken by 143 judges recruited through the CrowdFlower online crowdsourcing platform.3 Judges were compensated 50 cents for annotating a total of four clips.
All tasks were recorded by one RGB video camera and the motion capture system used for teleoperation. The recorded motion capture data were then used to produce robot-mediated versions of the targets’ performances on the NAO robot using the aforementioned teleoperation system, which were also recorded on video.
In addition to the audiovisual and teleoperated robot conditions, an audio-only condition was created using the audio from hobby and story tasks. Hence, each target had a total of 8 clips split over 3 communication conditions: 3 clips for the audiovisual condition, 2 clips for the audio-only condition, and 3 clips for the teleoperated robot condition. This resulted in a total of 158 clips (two clips became corrupted).
To avoid confusion, prompt questions were edited out of the clips. Further, for the few tasks where performance exceeded 60 s, clips were edited to be close to this length as pretests showed a decrease in the reliability of judgments with overly long clips. Mean clip duration was 50 s (SD = 20 s).
The clips were split up into surveys each containing four clips: one of each task and one of the audio-only clips, each of a unique target. Communication condition was pseudo-randomized across the three tasks in each survey, but always contained at least one of each communication condition.
The teleoperation system was extended to enable interactive multimodal communication. The first addition made was a stereo camera helmet on the NAO robot, the images from which are displayed in an Oculus Rift head-mounted display (HMD). Coupled with using the Rift’s inertial measurement unit to drive the robot’s head, meant the operator could see from the robots point of view, and their gaze direction and head motion could be observed on the robot. Secondly we used a voice over IP communication system to allow full duplex audio communication. Finally, due to feedback from participants in the Solo Tasks Study, we did not use the Polhemus Patriot in the Dyadic Tasks Study to make behaviors more natural; importantly, wrist rotation was only really needed for the mime task in the Solo Tasks Study, and is less important for normal gesturing. Figure 2 shows the teleoperation system and the setup during performance of dyadic tasks in the teleoperation (TO) condition.
Figure 2. Snapshots from the Dyadic Tasks Study. Upper row: illustration of teleoperation (TO) room and interaction room. Lower row: snapshots from the dyadic interaction sequences.
In the second study, the three tasks performed by participants involved interacting with a confederate, i.e., dyadic. A confederate was used to ensure that each participant had the same interactive partner, giving us a measure of control over the interactions, while still seeming natural to the participants. The three selected tasks were based on the suggestions by Funder et al. (2000) of having an informative task, a competitive task, and a cooperative task. The intention of these task types is that they each engender personality cues in different ways.
The three tasks were briefly explained to the participant and the confederate together, and more detailed written instructions were provided to be used during the experimental session. This was done to ensure that the experimenters could leave the room for the participant and confederate to converse alone. The two communication conditions (audiovisual and teleoperated robot) were performed sequentially, in a pseudorandomized order, in the same room. The audiovisual condition was recorded face-to-face, i.e., with both participant and confederate seated across a table from one another. In the teleoperated robot condition, the participant moved to an adjoining room where the teleoperation controls were located, while the confederate sat at a table across from the robot.
3.3.2.1. Task 1 (Informative)
Participants watched a clip from a Sylvester and Tweety cartoon, which they then had to describe to the confederate. This is a task commonly used to examine gesturing (Alibali, 2001), as describing the action filled cartoon often engenders gestures, which may be useful personality cues that can be produced by the robot. Another key reason for this task choice was that all participants have the same things to talk about: in the previously used hobby task several participants struggled to find much to say without significant prompting. Two different Sylvester and Tweety cartoons were used, one for each communication condition; cartoon assignment was randomized between conditions. We expected there to be an abundance of gestural cues, as well as cues related to the participants’ verbal behavior (such as how detailed the description was).
3.3.2.2. Task 2 (Competitive)
The participants and the confederate played a memory-based word game adapted from the traditional Grandmothers Trunk game. The first player says “My Grandmother went on holiday and she…” and adds something she did, accompanied by a gesture, the other player then repeats what the first said and their gesture, and adds something else she did. Play continues alternating between players who repeat the whole list of things and perform the gestures, adding a new thing each time, until one player forgets something and that player loses. How they approach the competitive nature of the task, and the actions they select are personality cues we expected from this task.
3.3.2.3. Task 3 (Cooperative)
The participants and the confederate cooperated to put a set of 5 items into utility order for surviving in a given scenario. There were two scenarios each with its own set of items, surviving a ship wreck, and surviving a crash landing on the moon. One scenario was presented per communication condition and was randomly assigned. How agreement is reached, and how the task is approached are the main cues we expect from this task.
Thirty participants were recorded as targets (13 female, mean age = 25.01, SD = 4.2), and gave written informed consent for their participation, they were reimbursed with a £5 gift voucher for their time. Recordings for 25 of the targets were used to create the clips used for judgments (5 targets were omitted due to recording problems). The study was approved by the ethics committee of the University of Cambridge.
Clip ratings were undertaken by 250 judges recruited through the Prolific Academic online crowdsourcing platform.4 Each judge rated 6 clips and was compensated £2 for their time.
In all tasks, both the confederate and the participant were recorded by separate RGB video cameras. The confederate was only recorded to obscure the fact that she was a confederate. In the teleoperated robot condition, a video camera recorded the robot instead of the participant. In order to produce videos of identical length for all targets and tasks, the video clips were further edited to select a 60 s segment from the beginning of the Informative task and from the end of Competitive and Cooperative tasks. This is in line with suggestions by Carney et al. (2007b) for using clips of this length of a task to maximize consistent judgment conditions for each target. Thus, each target had a set of three 60 s clips for each of the two communication conditions. One survey consisted of a pseudo-randomized set of 6 clips, 1 example of each task in each communication condition, with unique targets in each clip. Additionally a practice clip of the confederate was added to the start of all surveys to use as a measure of judge reliability, it also served to demonstrate her voice such that it could be ignored when she spoke during the target clips.
In Table 1, we summarized both studies in terms of number of participants, tasks, communication conditions, and communicated cues.
To address the research questions introduced in Section 1, we analyzed the level of agreement and the extent of shifts with respect to different communication conditions (e.g., audiovisual/AV, audio-only/AO, teleoperation/TO) and different tasks for each personality trait. We evaluated personality judgments to measure intra-/inter-agreement, self-other agreement, and personality shifts as below.
• Intra-judge Agreement: Intra-judge agreement (also known as internal consistency) evaluates the quality of personality judgments based on correlations between different questionnaire items that contribute to measuring the same personality trait by each judge. We measured intra-judge agreement in terms of standardized Cronbach’s α: where K is the number of the items (K = 2 in the Solo Tasks Study, and K = 4 in the Dyadic Tasks Study) and is the mean of pairwise correlations between values assigned. The resulting α coefficient ranges from 0 to 1; higher values are associated with higher internal consistency and values less than 0.5 are usually unacceptable (McKeown et al., 2012).
• Inter-judge Agreement: Inter-judge agreement refers to the level of consensus among judges. We computed the inter-judge agreement in terms of intraclass correlation (ICC) (Shrout and Fleiss, 1979). ICC assesses the reliability of the judges by comparing the variability of different ratings of the same target to the total variation across all ratings and all targets. We used ICC(1,k) as in our experiments each target subject was rated by a different set of k judges, randomly sampled from a larger population of judges. ICC(1,k) measures the degree of agreement for ratings that are averages of k independent ratings on the target subjects.
• Self-other Agreement: Self-other agreement measures the similarity between the personality judgments made by self and others. We computed self-other agreement in terms of Pearson correlation and tested the significance of correlations using Student’s t distribution. Pearson correlation was computed between the target’s self-reported responses and the mean of the others’ scores per trait.
• Personality Shifts: Personality shift refers to the extent to which people shifted from one personality class to another, in judges’ perception, between AV and TO conditions. In order to measure shifts, we first classified each target into low or high (e.g., introverted or extroverted) for each trait according to if their average judge rating for each task was above or below the mean for all targets in AV. For each trait, each target was grouped according to their classification in both conditions, creating 4 groups (i.e., AV: high and TO: high, AV: high and TO: low, etc.). We presented these results in terms of contingency tables and tested the significance using McNemar’s test with Edwards’s correction (Edwards, 1948).
In the following subsections, we present these results for each study (solo and dyadic) separately.
Although crowdsourcing techniques have many advantages, identifying annotators who assign labels without looking at the content (low-quality judges or spammers) is necessary to get informative results. As a first measure, we eliminated judges who incorrectly answered a test question about the content of the clips. After this elimination mean-judges-per-clip was 7.9 (SD = 1.5), with minimum judges-per-clip being 5.
To assess whether there remained further low-quality judges we calculated within-judge consistency for the AV clips using Cronbach’s α, which measures whether the values assigned to the items that contribute to the same trait are correlated. The average value across all tasks was lower than we expected (less than 0.5), indicating some judges answer randomly. With no low-quality judges, we would expect values for the AV clips greater than 0.5, i.e., in line with values reported in the literature for the BFI-10 with video clips assessed by online judges (Credé et al., 2012). We therefore used a judge selection method to remove these additional low-quality judges. We used a ranking-based method based on pairwise correlations instead of standard methods for outlier detection. For each clip, we calculated an average correlation score for each judge from pairwise correlations (using all 10 questions in the BFI-10) with the remaining judges. Judges with low correlation scores are deemed to be spammers. The judges were then ranked in order of correlation score and the k highest ranked selected.
To evaluate the efficacy of this ranking procedure we calculated within-judge consistency results for the AV clips for different judge numbers ranging from k = 10 (without elimination) to k = 3. These values averaged over all tasks are presented in Figure 3A. We further validated this by computing ICC with varying number of judges, Figure 3C. Selecting 5 judges per clip (based on pairwise comparisons) was found to be sufficient to increase reliability to acceptable levels for the AV clips (greater than 0.5) for all traits except for openness. We use 5 judges as it allows us to exclude all judges who failed the test question while having the same number of judges for all clips [5 judges is common in this type of study, e.g., Borkenau and Liebler (1992)].
Figure 3. Changes in Cronbach’s α values (A,B) and ICC values (C,D) as a function of number selected judges (k) for different traits in the AV communication condition for Solo Tasks Study (A–C) and Dyadic Tasks Study (B–D).
Within-judge consistency was measured in terms of Cronbach’s α. For the selected 5 judges per clip, the detailed results with respect to different communication conditions and tasks are presented in Table 2(a), where α values that indicate sufficient reliability for the BFI-10 (greater than 0.5, in line with values reported in the literature (Credé et al., 2012)) are highlighted in bold. To compare α values between communication conditions we follow the method suggested by Feldt et al. (1987): 95% confidence intervals are calculated for each α value, and if the value from one condition falls outside the confidence intervals from a condition it is being compared to, this suggests it is significantly less consistent. Comparing AO with AV for the hobby task, values for all traits, except for agreeableness, fall outside the 95% confidence intervals of the AV values. Comparing TO with AV for the mime task, values for all traits, except for conscientiousness, fall outside the 95% confidence intervals of the AV values. This indicates AV is found to be more consistent as compared to AO for the hobby task (except for agreeableness) and TO for the mime task (except for conscientiousness). No other comparisons indicate significant differences.
We computed between-judge consistency in terms of intraclass correlation, ICC(1,k) proposed by Shrout and Fleiss (1979), where k = 5. Our judge selection method uses the k most correlated judges so might bias the ICC results (see Section 4.1.1). To evaluate this, we calculated ICC for k = (10, …3) for the AV condition. Figure 3B shows that, for extroversion, conscientiousness, and neuroticism, ICC does not change meaningfully as the number of judges varies, while selecting the 5 most correlated judges slightly biases the results for agreeableness and openness.
The detailed results for the selected 5 judges per clip are presented in Table 2(b). We obtained significant correlations for most traits in the AV condition, with values in the same range (0.40 < ICC(1, k) < 0.81) as reported in the literature for online judges using a 10-item test (0.42 < ICC(1, k) < 0.76) (Biel and Gatica-Perez, 2013). Fewer significant correlations were observed in the other communication conditions, particularly in the story task for AO and the mime task for TO. Extroversion was the only trait that consistently maintained correlation across conditions.
We examined the extent to which judges agree with the target’s self-assessment. Pearson correlations between the self-ratings and the judge’s ratings of conditions and tasks are reported in Table 2(c) for the selected 5 judges per clip. We observed that the judge’s ratings bear a significant relation to the target’s self-ratings for extroversion only (r = 0.24 − 0.44 and p < 0.05). However, we did not obtain any significant correlations in the TO condition (all r < 0.2 and p > 0.05).
We examined the extent to which people shifted from one personality class to another, in judges’ perception, between AV and TO conditions, in the hobby and story tasks for the selected 5 judges per clip. We did not examine shifts involving AO or Mime task as the ICC scores indicated that personality ratings in this condition would be too unreliable. These results are presented in Table 3 as 2 × 2 contingency tables. To aid analysis we have also illustrated each shift as a proportional change (%) both from high to low (HIGH2LOW) and from low to high (LOW2HIGH) in Figure 4 (see the figure on the left hand side).
Figure 4. Amount of shifts (%) from high to low (HIGH2LOW) and from low to high (LOW2HIGH) (*p < 0.05, ***p < 0.001) between AV and TO: solo tasks (left hand side) versus dyadic tasks (right hand side).
We found a significant shift from high to low for neuroticism (70%). Note that the corrected McNemar’s test is very conservative in estimating significance, particularly for small sample sizes. Although not statistically significant, we observed large shifts from low to high for extroversion (56%), conscientiousness (67%), and openness (57%).
As in the Solo Tasks Study, we assessed whether there existed low-quality judges (spammers) in the judge pool used for the Dyadic Tasks Study. To do so, we repeated the same method that we used for the Solo Tasks Study, where we evaluated ICC values, and used judge rating techniques to selectively remove judges. These results are presented in Figures 3B,D. As we observed ICC values for the AV condition in line with expectation with all judges included, and cannot observe large changes in the Cronbach’s α values and the ICC values, by excluding judges, we concluded that the judges were reliable. Hence, we present the results for the Dyadic Tasks Study without eliminating any judges.
Within-judge consistency was measured in terms of Cronbach’s α. The detailed results with respect to different communication conditions and tasks are presented in Table 4(a), where α values that indicate sufficient reliability for the IPIP-BFM-20 (greater than 0.75, in line with values reported in the literature (Credé et al., 2012)) are highlighted in bold. Values are above or close to good reliability (>0.7) for all traits except for neuroticism. Comparing values across communication conditions, we observe little difference, hence judges were able to make consistent trait evaluations when the robot is used for communication.
We computed between-judge consistency in terms of intraclass correlation, ICC(1,k), where k = 10 (Shrout and Fleiss, 1979). The detailed results for the 10 judges per clip are presented in Table 4(b). Extroversion and openness are the only traits with significant agreement across most tasks and both conditions (0.47 ≤ ICC(1, k) ≤ 0.85 at a significance level of p < 0.01). Other traits vary between tasks and conditions as to where significant agreement is achieved. A clearer picture can be gained from the all task results, where it can be seen that agreement on conscientiousness deteriorates in the TO condition relative to AV (a drastic drop from 0.61 to −0.26 over all tasks).
We examined the extent to which judges agree with the target’s self-assessment. Pearson correlations between the self-ratings and the judge’s ratings of conditions and tasks are reported in Table 4(c). Significant agreement was found for agreeableness and openness across most tasks and both conditions (rag = 0.75 and rop = 0.71 over all tasks), although agreement is much lower in the TO condition (rag = 0.63 and rop = 0.46 over all tasks). For extroversion and neuroticism, agreement is much lower than for other traits, and this is fairly consistent across conditions. Again we observe the larger difference across conditions for conscientiousness (rco = 0.17), with almost no significant agreement in the TO condition compared to significant agreement across all tasks in the AV condition (rco = 0.31).
We examined the extent to which people shifted from one personality trait classification to another, in judges’ perception, between AV and TO conditions for each task. These results are presented in Tables 3 and 5 as 2 × 2 contingency tables. To aid analysis, we have also illustrated each shift as a proportional change (%) both from high to low (HIGH2LOW) and from low to high (LOW2HIGH) in Figure 4 (see the figure on the right hand side). We found a significant shift from high to low for agreeableness (65%), conscientiousness (67%) and openness (56%). Although not statistically significant, we observed a large shift from high to low for neuroticism (57%).
In this section, we discuss our results, including comparisons with related work introduced in Section 2. We present in-depth discussion of meta-data (i.e., judge ratings, self-ratings) in terms of intra/inter-judge agreement, accuracy of judgments and personality shifts, with regard to different communication conditions (i.e., AO: audio-only, AV: audiovisual, and TO: teleoperation) and different tasks (i.e., solo and dyadic tasks). Note that in the majority of related works results were not directly comparable as personality recognition accuracy is typically the reported metric, as opposed to agreement as used here; accuracy as measured by comparing human responses with machine learning systems (e.g., Aran and Gatica-Perez (2013), Batrinca et al. (2016)), or between self-ratings and judge ratings (e.g., Funder (1995), Borkenau et al. (2004)). Nevertheless, for which traits this reported accuracy is high or low helps provide some explanation for our findings.
Consistency within judges for how each trait is judged (Table 2(a) and Table 4(a)) is used to address RQ1. In both studies, judges were sufficiently consistent in their trait ratings in the audiovisual condition (AV), with the exception of openness in the Solo Tasks Study, and to a lesser extent neuroticism in the Dyadic Tasks Study for us to conclude that the tasks and judges’ behaviors were reliable. Batrinca et al. (2016) also reported a similar finding that openness was not modeled successfully in the human-machine interaction, whereas, in the human–human interaction setting, it was the only trait that could be predicted with a high accuracy over all collaboration tasks. In our case, the difference between the two studies with regard to consistent judgment of the openness trait indicates that cues for this trait may be more evident in dyadic tasks. Some researchers have suggested that one aspect of openness is intellect, where intellect incorporates the facets of intelligence, intellectual engagement, and creativity (DeYoung, 2011), and the tasks in the Dyadic Tasks Study are more conducive to displaying these facets.
In the Solo Tasks Study, there were some notable differences between the audio-only (AO) and the teleoperated robot (TO) conditions. For the hobby task, judges remained consistent in both the AO and TO conditions, indicating they were able to use audio cues to make judgments for this task, and robot appearance had no effect on consistency. However, for the story task, judges were much less consistent in the AO than in the AV condition, for all traits except for agreeableness. This is in contrast to the teleoperated robot condition (TO), where they remained as consistent as in the AV condition. The only additional cues available with the robot compared to audio only are gestures and appearance. The results indicate that such cues are used to aid judgments in the same way that they do in the AV condition, though their utility appears to be task dependent (only of apparent benefit in the story task). Importantly, the fact that they are utilized provides good evidence that the robot is not simply ignored when making judgments. Hence, the findings of high levels of agreement across both conditions in all tasks in the Dyadic Tasks Study indicate that in dyadic tasks the robot transmits sufficient cues to make judgments as consistently as observing the target directly.
The use of gesture to aid personality judgments appears to be dependent on it accompanying speech, as in the Solo Tasks Study ratings in the TO condition are far less consistent than in the AV condition for the mime task. That is to say, gestures alone do not provide sufficient information for judging personality. This was in contrast to what was reported by Aran and Gatica-Perez (2013), where the best results were achieved when they used visual cues only for predicting personality traits, and using audio cues or combining them with visual cues resulted in lower accuracy. This showed that either other behavior cues not transmitted by the robot are needed, or appearance cues are used which conflict with gesture cues in the TO condition.
Taking the results from both studies together, it is apparent that judges are able to remain consistent in their judgments of a given trait whether they are observing someone directly or their communication relayed through a teleoperated robot. Indeed, where there are slight shifts in consistency between AV and TO conditions, they are not large; the one exception being for the mime task in the Solo Tasks Study. Hence, each judge appears to formulate a relatively consistent evaluation of a given targets’ personality traits based on speech, gesture, and appearance, combining them to assess each trait facet. This finding is in contrast to the study by Kuwamura et al. (2012) where they suggested small shifts in intra-judge consistency provided evidence of robot appearance effects on personality perception. While in subsequent sections we do observe evidence for effects of robot mediation on perception, we do not find such small shifts in intra-judge consistency convincing in this regard.
Looking at inter-judge agreement results to address RQ2 (Table 2(b) and Table 4(b)), extroversion was the only trait on which judges reached consensus in both studies, regardless of the communication condition, and task (the mime task in the Solo Tasks Study being the one exception). This result is in line with the widely accepted idea that extroversion is the easiest trait to infer upon others (Barrick et al., 2000). Hence, the strength of the available cues was sufficient to overcome any conflict between appearance, vocal, and gesture based cues. Indeed it indicates that judges had a common set of interpretations for the available cues.
On the other hand, where agreement was reached on agreeableness, conscientiousness, and neuroticism for some tasks in the AV condition in each study, it had mostly deteriorated in the TO condition, and the AO condition in the Solo Tasks Study. The clearest example of this is for conscientiousness taking all three tasks together in the Dyadic Tasks Study (and to some extent in the Solo Tasks Study as well), where agreement drastically deteriorated in the TO condition as compared to the AV condition. As explained in the study by Macrae et al. (1996), physical appearance based impressions (facial and vocal features) are often used in the judgment of conscientiousness. In particular, low conscientiousness is conveyed by a childlike face (Macrae et al., 1996), which the face of the NAO robot can be considered to have, and this may conflict with the vocal cues of the operator. Neuroticism is mainly related to emotions, and agreeableness is related to trust, cooperation and sympathy (Zillig et al., 2002), both of which it seems reasonable to suggest judges might perceive as being low for a robot (particularly NAO with its lack of facial expressions), again creating conflicts. It would appear that judges do not have a consistent manner with which to resolve such conflicts.
Task-based analyzes in the Solo Tasks Study show that for agreeableness and conscientiousness the story task provides sufficient cues for agreement to be maintained in the TO condition, whereas the hobby task does so for neuroticism. As agreement being maintained in the TO condition indicates sufficient cues to overcome appearance/behavior conflicts, it is instructive to consider how those tasks might relate to the traits. In telling the story, targets might demonstrate their morality, and relation to others, components of agreeableness (Zillig et al., 2002). How well structured and clear the story is could relate to facets of the conscientiousness trait. The hobby task on the other hand might demonstrate how self-conscious a person is about their hobby, a facet of neuroticism (Zillig et al., 2002). While these two tasks might provide some cues for facets of the traits for which consistency was not maintained, they appear to do so in a way that conflicts with cues related to the robot.
We also compared differences in agreement between the TO and AO conditions in the Solo Tasks Study. Where there is agreement in TO for agreeableness, conscientiousness, and neuroticism, we found it was greatly reduced for agreeableness and conscientiousness, and to a lesser extent for neuroticism. This provides further evidence that physical cues, be they behavioral or appearance based, are utilized in the TO condition. Again, this appears to be dependent on the presence of speech: in the mime task for the Solo Tasks Study, judges were unable to provide a consistent rating for any trait in the TO condition, in contrast to the consistent ratings for extroversion, conscientiousness, and neuroticism in the AV condition. A likely reason for this observation is that without vocal cues there is an increased reliance on appearance based cues, often based on stereotypes (Kenny et al., 1994), and judges do not have consistent stereotypes relating to robot appearance.
Batrinca et al. (2016) showed that the prediction of agreeableness and conscientiousness in the human-machine interaction setting and the prediction of conscientiousness and neuroticism were highly dependent on the collaboration task, where the extroversion trait was the only trait yielding consistent results over all tasks in both settings. Similarly, our task-based analyses in the Dyadic Tasks Study show that in the AV condition, while the cooperative task provided a higher level of agreement for agreeableness and conscientiousness, the competitive task yielded better results for neuroticism and openness. Indeed, the results are somewhat expected given the nature of the tasks: the cooperative task was to agree upon how to order five items in a survival scenario, in which participants were expected to exhibit the agreeableness facet of personality; the competitive task was more related to creativity and intelligence, that are strongly associated with openness (Zillig et al., 2002). Though agreement is lower, it is still maintained for agreeableness in the cooperative task and openness in the competitive task in the TO condition. This indicates that in these cases, for at least some of the judges, either the vocal cues override the visual cues, or movement cues are utilized (with the vocal cues).
Taken together, the findings from both studies indicate that the ability of judges to make judgments based on a common interpretation of cues is affected not only by communication condition but is also dependent on the task. While in some cases it is apparent that a particular task is conducive to providing more verbal cues than another for a particular trait (as indicated by higher agreement, and inferred from the literature), whether these override the physical cues in the TO condition is hard to predict. Indeed, whether clear cues in the AV condition translate into agreement in the TO condition vary a great deal between all tasks. Hence, it seems reasonable to suggest that whether inter-judge consistency is observed also depends on how much appearance cues are utilized for a given task and trait, and thus how all the cues interact. This complex interaction effect provides strong evidence that personality perception is likely to be altered when communicating via a robot, and this depends on what cues are produced.
In order to assess RQ3, we analyzed the extent to which judge ratings correlated with self-ratings provided by target participants (Table 2(c) and Table 4(c)). In general in the Solo Tasks Study, there was very little correlation between self and other ratings. This is in contrast to previous findings where they found low, but significant, self-other correlation (0.11 − 0.42) (Carney et al., 2007a). The one exception to this was self-other correlation for extroversion in the AV condition. This suggests that participant targets did not present cues relating to their self-perception in the tasks we used, other than for extroversion which is commonly reported as the trait with the most available cues. Audio cues were sufficient for this correlation to be maintained in the hobby task in the AO condition, but not in the story task, or in either task in the TO condition.
In contrast to the tasks used in the Solo Tasks Study, the tasks of the Dyadic Tasks Study resulted in self-other agreement for extroversion, agreeableness, conscientiousness, and openness in the majority of tasks for the AV condition. This indicates that the tasks we used in the Dyadic Tasks Study were better at engendering more naturalistic behavior, and hence personality cues than the tasks in the Solo Tasks Study. Indeed, an important factor in thin slice personality analysis is how easy a person is to judge (Funder, 1995), and people behaving more naturally produce better cues. However, despite these apparently better cues, there was a large reduction in agreement for conscientiousness, neuroticism, and openness (and to a lesser extent agreeableness) in the TO condition relative to the AV condition. This finding combined with those of the Solo Tasks Study suggests that there is a shift in the way personality cues are interpreted caused by their interaction with the appearance of the robot, and the way non-verbal communication cues are reproduced on it.
In order to address RQ4, we analyzed the difference in perceived personality in terms of the occurrences of personality shifts. We principally consider the results from the Dyadic Tasks Study as it provides the more compelling evidence. The main reason for this assertion is that more naturalistic cues appeared to be produced in the Dyadic Tasks Study (see previous section), and we consider such cues and their interaction with the TO condition more ecologically valid. In addition, by being able to consider three tasks rather than the two considered in the Solo Tasks Study we have increased statistical power. The shifts we observed (Figure 4) provide evidence that cues related to the robots appearance are incorporated into, or even override personality judgments based on speech. Indeed, this is somewhat to be expected given that (Behrend et al., 2012) observed that, in judgments of suitability, attractiveness of a graphical avatar superseded qualities perceived in an interviewees words.
There are two likely causal factors in the perceived personalities being shifted, first human-based physical appearance stereotypes (inferred from humanlike characteristics of the robot) might be applied, second characteristics related to robots might be applied. Here, we will discuss possible underlying causes for the shifts observed in the Dyadic Tasks Study. In the case of conscientiousness and neuroticism a childlike face, as the NAO might be considered to have, conveys low ratings for both traits (Borkenau and Liebler, 1992; Macrae et al., 1996). Further, conscientiousness and neuroticism were also observed to be influenced by face shape in graphical avatars (Fong and Mar, 2015), and as the NAO has a face shape that differs from a human, hence this could lead to distortions in perceptions of these traits. Additionally, neuroticism is mainly related to emotions (Zillig et al., 2002), something which robots are rarely considered to have. Also linked to emotions is openness, which combined with its other facets of imagination and creativity, might also be reasonably expected to be low for a robot, which could also be considered to have hard facial linaments, also linked to low openness (Borkenau and Liebler, 1992). The NAO robot could also be considered male in appearance, and male avatars have been found to cue for lower conscientiousness and openness (Fong and Mar, 2015). Low agreeableness is more difficult to rationalize, but one facet is trustworthiness (Zillig et al., 2002), and judges may have perceived using a robot to communicate as less trustworthy. The vocal cues for extroversion appeared to be very strong, and this might explain why little influence on this trait was observed.
An important thing to note from these findings is that people appear to be attributing personality stereotypes to NAO for characteristics other than the extroversion trait, which has been previously examined (Park et al., 2012; Aly and Tapus, 2013; Celiktutan and Gunes, 2015). Hence, in future work in which a desired personality is to be expressed by an autonomous robot, its appearance based cues must be considered alongside any behavioral cues expressed. We suggest that strong behavioral cues may be required to overcome such stereotypes.
In this paper, we have shown that judges are able to make personality trait judgments that are as consistent with a robot avatar as when the same people are viewed on video in contrast to past work (Kuwamura et al., 2012). One possible reason for this difference in findings is that our teleoperation system allows reproduction of some non-verbal communication cues on the robot which might improve the ease with which judges can assess personality. Hence, we suggest that it is important for telepresence systems to be able to transmit non-verbal communication cues, whether this be actuation of physical systems, or large enough screens on remote presence devices.
We have shown that the appearance of a teleoperated robot avatar influences how the personality of its controller is perceived, i.e., robot appearance based personality cues are utilized along with cues in the speech of the operators. Hence, the perceived personality of a teleoperator is shifted toward that related to the robot’s appearance. In light of these findings, we suggest that robot avatar appearance and behavior be carefully considered relative to the person who will be controlling it, and this needs to be done on an individual basis. Training of operators to produce clear cues, or having some cues appropriate to the operator’s personality autonomously generated, might allow some control of appearance effects.
Having the correct robot personality has been found to have a positive effect on interactions with people (Park et al., 2012; Aly and Tapus, 2013; Celiktutan and Gunes, 2015), and our findings also have implications for such autonomous robot personality expression. It is important to consider what appearance cues for personality a robot has, as we have observed humanlike personality inferences, and whether the planned behavioral cues might conflict with them. Cues that work on one platform may not be transferable to another. Additionally, we suggest that future experiments on robots expressing personality need to carefully consider tasks undertaken, as we observed that intra-judge agreement on personality perception was highly task dependent.
While this paper provides evidence for how personality perception is affected for people teleoperating a humanoid robot avatar, it has a number of limitations we hope to address in future work.
One area of limitation in our work relates to the movement capabilities of the NAO robot, and the inherent differences with human movement capabilities. Although our previous work showed reproduced gestures are comprehensible (Bremner and Leonards, 2015, 2016), there are clearly appreciable differences in the way some movements are reproduced. Indeed, while these differences have limited affect on perceived meaning, they likely contribute to the observed distortions in personality. The main limitations in this regard are in elbow flexion, movement speed, and wrist and hand motion: the NAO elbow can only bend to ~90°, the main effect of which being a reduction in vertical travel of the hand for some gestures; humans are capable of extremely rapid motions that the robot cannot match, consequently it will catch up as best it can, but the usual response will be to not express some motions due to the method of motion processing; wrist flexion and hand shape are clearly of utility in many gestures, and their absence (as well as wrist rotation in study 2) restricts the expression of components of some gestures. These movement restrictions are added to by limitations in the Kinect sensor and software processing: movements that result in hand occlusions can lead to imprecision, as well as noise in the sensor data can lead to some added jitter on the robot (though this is filtered as much as possible).
It is also important to note that robot operators had little to no awareness of the limitations of the robot as none of them had prior experience with NAO, and when in control of it they could not observe its motion. The only instruction given pertaining to system capabilities was to not to rest with the arms flat against the body or behind the back as tracking would be lost. While this resulted in some initial poses that were a bit unnatural (video of which was not used in the studies), participants soon reverted to “normal” behavior. Indeed, qualitative comparison of participants in the dyadic study in each condition (video of participants recorded while they were operating the robot allowed this) reveals little difference in gesturing behavior for the majority of participants. Exceptions were the two participants with prior experience working with robots who moved more than they did face-to-face. In further work, we aim to more closely examine the data for any differences (which may be subtle), and if present test how they contribute to the observed personality distortion effects.
In the study by Celiktutan et al. (2016), our AV condition results showed that face gestures and head activity play an important role in the recognition of the extroversion, agreeableness and conscientiousness traits. This implies another limitation of the robotic platform used in this study. To convey the teleoperators personality traits more accurately, the robot should portray head pose or facial activity together with audio and arm gestures.
A further limitation is that there are some differences between our two studies, the Dyadic Tasks Study has a slightly different design due to correcting issues we encountered in the Solo Tasks Study, making the study comparison slightly less fair. In particular, we addressed the issue with low-quality judges, by utilizing a different recruiting platform which allowed us to recruit better quality judges, and thus did not require a judge removal process. In the Solo Tasks Study, the issues with low-quality judges meant we used a judge selection method based on the gathered responses. The procedure we used had a slight biasing effect on the between-judge consistency (ICC) result for agreeableness and openness. This bias means that where ICC values are not significant it is strong evidence that there is either a lack of cues or conflicting cues, as even amongst the most agreeing judges consensus of opinion was not possible. Where there is significant agreement, it indicates there are cues for that trait in the particular task and condition and some judges are able to pick up on these cues. Indeed, Funder points out that there exists good and bad judges of personality (Funder, 1995), and we suggest our selection method allowed us to bias toward good judges. This limits the generalizability of our results to judges more adept at picking up on personality cues. By changing crowdsourcing platforms we were able to remove the need for this selection process in the Dyadic Tasks Study.
In addition to recruiting better quality judges, we also utilized a larger personality questionnaire, making our results more accurate, especially with regard to measuring intra-judge and inter-judge consistency.
In the work reported here, it is not clear how different cues are utilized in the aforementioned personality perception. Given that there was such high variability in affects of robot appearance dependent on the task, it seems likely this is due to differences in use of audio and visual cues. Hence, we intend to analyze in-depth the behaviors of targets relative to their judged personality for different tasks. To facilitate this, we aim to extend our work on automatic personality classification, which can extract and identify useful cues automatically (Celiktutan et al., 2016), and apply it to the recordings from the Dyadic Tasks Study. A comparative cue analysis could not only allow us to gain a better understanding of the causes of personality shifts, but could also be useful in synthesizing robot personality behavioral cues.
This study was carried out in accordance with the recommendations of the ethics committees of the University of the West of England and the University of Cambridge with written informed consent from all subjects. All subjects gave written informed consent in accordance with the Declaration of Helsinki. The protocol was approved by the ethics committees of the University of the West of England and the University of Cambridge.
PB: substantial contributions to the conception and design of the work, the acquisition, analysis, and interpretation of data; drafting the work; final approval of the version to be published; and agreement to be accountable. OC: substantial contributions to the conception and design of the work, the acquisition, analysis, and interpretation of data; drafting the work; final approval of the version to be published; and agreement to be accountable. HG: substantial contributions to the design of the work, analysis, and interpretation of data; revising the work critically for important intellectual content; final approval of the version to be published; and agreement to be accountable.
The authors declare that the research was conducted in the absence of any commercial or financial relationships that could be construed as a potential conflict of interest.
This work was funded by the EPSRC under its IDEAS Factory Sandpits call on Digital Personhood (Grant Ref: EP/L00416X/1).
Adalgeirsson, S. O., and Breazeal, C. (2010). “MeBot: a robotic platform for socially embodied telepresence,” in Proc. of Int. Conf. Human Robot Interaction (Osaka: ACM/IEEE), 15–22.
Alibali, M. (2001). Effects of visibility between speaker and listener on gesture production: some gestures are meant to be seen. J. Mem. Lang. 44, 169–188. doi: 10.1006/jmla.2000.2752
Aly, A., and Tapus, A. (2013). “A model for synthesizing a combined verbal and nonverbal behavior based on personality traits in human-robot interaction,” in Proc. of ACM/IEEE Int. Conf. on Human-Robot Interaction, Tokyo.
Aran, O., and Gatica-Perez, D. (2013). “One of a kind: inferring personality impressions in meetings,” in Proc. of ACM Int. Conf. on Multimodal Interaction, Sydney.
Barrick, M. R., Patton, G. K., and Haugland, S. N. (2000). Accuracy of interviewer judgments of job applicant personality traits. Personnel Psychol. 53, 925–951. doi:10.1111/j.1744-6570.2000.tb02424.x
Batrinca, L., Mana, N., Lepri, B., Sebe, N., and Pianesi, F. (2016). Multimodal personality recognition in collaborative goal-oriented tasks. IEEE Trans. Multimedia 18, 659–673. doi:10.1109/TMM.2016.2522763
Behrend, T., Toaddy, S., Thompson, L. F., and Sharek, D. J. (2012). The effects of avatar appearance on interviewer ratings in virtual employment interviews. Comput. Human Behav. 28, 2128–2133. doi:10.1016/j.chb.2012.06.017
Bevan, C., and Stanton Fraser, D. (2015). “Shaking hands and cooperation in tele-present human-robot negotiation,” in Proc. of Int. Conf. Human Robot Interaction (Portland: ACM/IEEE), 247–254.
Biel, J., and Gatica-Perez, D. (2013). The YouTube lens: crowdsourced personality impressions and audiovisual analysis of Vlogs. IEEE Trans. Multimedia 15, 41–55. doi:10.1109/TMM.2012.2225032
Borkenau, P., and Liebler, A. (1992). Trait inferences: sources of validity at zero acquaintance. J. Pers. Soc. Psychol. 62, 645–657. doi:10.1037/0022-3514.62.4.645
Borkenau, P., Mauer, N., Riemann, R., Spinath, F. M., and Angleitner, A. (2004). Thin slices of behavior as cues of personality and intelligence. J. Pers. Soc. Psychol. 86, 599–614. doi:10.1037/0022-3514.86.4.599
Bremner, P., Celiktutan, O., and Gunes, H. (2016a). “Personality perception of robot avatar tele-operators,” in The Eleventh ACM/IEEE International Conference on Human Robot Interaction, HRI ’16 (Christchurch: IEEE), 141–148.
Bremner, P., Koschate, M., and Levine, M. (2016b). “Humanoid robot avatars: an ‘in the wild’ usability study,” in RO-MAN (New Zealand: IEEE).
Bremner, P., and Leonards, U. (2015). “Efficiency of speech and iconic gesture integration for robotic and human communicators – a direct comparison,” in Proc. of IEEE Int. Conf. on Robotics and Automation (Seattle: IEEE), 1999–2006.
Bremner, P., and Leonards, U. (2016). Iconic gestures for robot avatars, recognition and integration with speech. Front. Psychol. 7:183. doi:10.3389/fpsyg.2016.00183
Carney, D. R., Colvin, C. R., and Hall, J. A. (2007a). A thin slice perspective on the accuracy of first impressions. J. Res. Pers. 41, 1054–1072. doi:10.1016/j.jrp.2007.01.004
Celiktutan, O., Bremner, P., and Gunes, H. (2016). “Personality classification from robot-mediated communication cues,” in 25th IEEE International Symposium on Robot and Human Interactive Communication (RO-MAN), New York.
Celiktutan, O., and Gunes, H. (2015). “Computational analysis of human-robot interactions through first-person vision: personality and interaction experience,” in 24th IEEE International Symposium on Robot and Human Interactive Communication (RO-MAN) (Kobe: IEEE), 815–820.
Credé, M., Harms, P., Niehorster, S., and Gaye-Valentine, A. (2012). An evaluation of the consequences of using short measures of the big five personality traits. J. Pers. Soc. Psychol. 102, 874–888. doi:10.1037/a0027403
Daly-Jones, O., Monk, A., and Watts, L. (1998). Some advantages of video conferencing over high-quality audio conferencing: fluency and awareness of attentional focus. Int. J. Human Comput. Stud. 49, 21–58. doi:10.1006/ijhc.1998.0195
DeYoung, C. D. (2011). “Intelligence and personality,” in The Cambridge Handbook of Intelligence, eds R. J. Sternberg and S. B. Kaufman (New York, NY: Cambridge University Press), 711–737.
Edwards, A. L. (1948). Note on the correction for continuity in testing the significance of the difference between correlated proportions. Psychometrika 13, 185–187. doi:10.1007/BF02289261
Feldt, L. S., Woodruff, D. J., and Salih, F. A. (1987). Statistical inference for coefficient alpha. Appl. Psychol. Measure. 11, 93–103. doi:10.1177/014662168701100107
Fong, K., and Mar, R. A. (2015). What does my avatar say about me? Inferring personality from avatars. Pers. Soc. Psychol. Bull. 41, 237–249. doi:10.1177/0146167214562761
Funder, D. C. (1995). On the accuracy of personality judgment: a realistic approach. Psychol. Rev. 102, 652–670. doi:10.1037/0033-295X.102.4.652
Funder, D. C., Furr, R. M., and Colvin, C. R. (2000). The riverside behavioral q-sort: a tool for the description of social behavior. J. Pers. 68, 451–489. doi:10.1111/1467-6494.00103
Funder, D. C., and Sneed, C. D. (1993). Behavioral manifestations of personality: an ecological approach to judgmental accuracy. J. Pers. Soc. Psychol. 64, 479–490. doi:10.1037/0022-3514.64.3.479
Gouaillier, D., Hugel, V., Blazevic, P., Kilner, C., Monceaux, J., Lafourcade, P., et al. (2009). “Mechatronic design of NAO humanoid,” in Proc of IEEE Int. Conf. on Robotics and Automation (Kobe: IEEE), 769–774.
Hossen Mamode, H. Z., Bremner, P., Pipe, A. G., and Carse, B. (2013). “Cooperative tabletop working for humans and humanoid robots: group interaction with an avatar,” in IEEE Int. Conf. on Robotics and Automation (Karlsruhe: IEEE), 184–190.
Kenny, D. A., Albright, L., Malloy, T. E., and Kashy, D. A. (1994). Consensus in interpersonal perception: acquaintance and the big five. Psychol. Bull. 116, 245–258. doi:10.1037/0033-2909.116.2.245
Kristoffersson, A., Coradeschi, S., and Loutfi, A. (2013). A review of mobile robotic telepresence. Adv. Human-Comput. Interact. 2013, 17. doi:10.1155/2013/902316
Kuwamura, K., Minato, T., Nishio, S., and Ishiguro, H. (2012). “Personality distortion in communication through teleoperated robots,” in Proc of IEEE Int. Symp. on Robot and Human Interactive Communication (Paris: IEEE), 49–54.
Lee, M. K., and Takayama, L. (2011). “Now, I have a body,” in Proc. of the Conf. on Human Factors in Computing Systems (Vancouver, BC: ACM Press), 33.
Macrae, C. N., Stangor, C., and Hewstone, M. (1996). Stereotypes and Stereotyping (New York, NY: The Guilford Press).
Martins, H., and Ventura, R. (2009). “Immersive 3-d teleoperation of a search and rescue robot using a head-mounted display,” in IEEE Conf. on Emerging Technologies Factory Automation (ETFA) (Mallorca: IEEE), 1–8.
McKeown, G., Valstar, M., Cowie, R., Pantic, M., and Schroder, M. (2012). The semaine database: annotated multimodal records of emotionally colored conversations between a person and a limited agent. IEEE Trans. Affect. Comput. 3, 5–17. doi:10.1109/T-AFFC.2011.20
Naumann, L. P., Vazire, S., Rentfrow, P. J., and Gosling, S. D. (2009). Personality judgments based on physical appearance. Pers. Soc. Psychol. Bull. 35, 1661–1671. doi:10.1177/0146167209346309
O’Conaill, B., Whittaker, S., and Wilbur, S. (1993). Conversations over video conferences: an evaluation of the spoken aspects of video-mediated communication. Human Comput. Interact. 8, 389–428. doi:10.1207/s15327051hci0804_4
Park, E., Jin, D., and del Pobil, A. P. (2012). The law of attraction in human-robot interaction. Int. J. Adv. Rob. Syst. 9, 1–7. doi:10.5772/50228
Rae, I., Takayama, L., and Mutlu, B. (2013). “In-body experiences,” in Proceedings of the SIGCHI Conference on Human Factors in Computing Systems – CHI ’13 (New York, NY: ACM Press), 1921–1930.
Rammstedt, B., and John, O. P. (2007). Measuring personality in one minute or less: a 10-item short version of the big five inventory in English and German. J. Res. Pers. 41, 203–212. doi:10.1016/j.jrp.2006.02.001
Riggio, R. E., and Friedman, H. S. (1986). Impression formation: the role of expressive behavior. J. Pers. Soc. Psychol. 50, 421–427. doi:10.1037/0022-3514.50.2.421
Salam, H., Celiktutan, O., Hupont, I., Gunes, H., and Chetouani, M. (2016). Fully automatic analysis of engagement and its relationship to personality in human-robot interactions. IEEE Access 5, 705–721. doi:10.1109/ACCESS.2016.2614525
Shrout, P., and Fleiss, J. (1979). Intraclass correlations: uses in assessing rater reliability. Psychol. Bull. 86, 420–428. doi:10.1037/0033-2909.86.2.420
Straub, I., Nishio, S., and Ishiguro, H. (2010). “Incorporated identity in interaction with a teleoperated android robot: a case study,” in Proc of Int. Symp. in Robot and Human Interactive Communication (Viareggio: IEEE), 119–124.
Tang, A., Boyle, M., and Greenberg, S. (2004). “Display and presence disparity in mixed presence groupware,” in Proc. of Australasian User Interface Conf (Dunedin: Australian Computer Society, Inc.), 73–82.
Topolewska, E., Skiminia, E., Strus, W., Cieciuch, J., and Rowinski, T. (2014). The short ipip-bfm-20 questionnaire for measuring the big five. Ann. Psychol. 2, 385–402.
Vinciarelli, A., and Mohammadi, G. (2014). A survey of personality computing. IEEE Trans. Affect. Comput. 5, 273–291. doi:10.1109/TAFFC.2014.2330816
Wang, Y., Geigel, J., and Herbert, A. (2013). “Reading personality: avatar vs. human faces,” in Proc. of HAC Conf. on Affective Computing and Intelligent Interaction (Geneva: IEEE), 479–484.
Yamazaki, R., Nishio, S., Ogawa, K., and Ishigur, H. (2012). “Teleoperated android as an embodied communication medium: a case study with demented elderlies in a care facility,” in RO-MAN (Paris: IEEE), 1066–1071.
Zillig, L. M. P., Hemenover, S. H., and Dienstbier, R. A. (2002). What do we assess when we assess a big 5 trait? A content analysis of the affective, behavioral, and cognitive processes represented in big 5 personality inventories. Pers. Soc. Psychol. Bull. 28, 847–858. doi:10.1177/0146167202289013
Keywords: telepresence, Big Five personality traits, personality perception
Citation: Bremner PA, Celiktutan O and Gunes H (2017) Personality Perception of Robot Avatar Teleoperators in Solo and Dyadic Tasks. Front. Robot. AI 4:16. doi: 10.3389/frobt.2017.00016
Received: 20 December 2016; Accepted: 27 April 2017;
Published: 23 May 2017
Edited by:
Giuseppe Carbone, University of Cassino, ItalyReviewed by:
Fulvio Mastrogiovanni, University of Genoa, ItalyCopyright: © 2017 Bremner, Celiktutan and Gunes. This is an open-access article distributed under the terms of the Creative Commons Attribution License (CC BY). The use, distribution or reproduction in other forums is permitted, provided the original author(s) or licensor are credited and that the original publication in this journal is cited, in accordance with accepted academic practice. No use, distribution or reproduction is permitted which does not comply with these terms.
*Correspondence: Paul Adam Bremner, cGF1bC5icmVtbmVyQGJybC5hYy51aw==
†These authors have contributed equally to this work.
Disclaimer: All claims expressed in this article are solely those of the authors and do not necessarily represent those of their affiliated organizations, or those of the publisher, the editors and the reviewers. Any product that may be evaluated in this article or claim that may be made by its manufacturer is not guaranteed or endorsed by the publisher.
Research integrity at Frontiers
Learn more about the work of our research integrity team to safeguard the quality of each article we publish.