- 1Tel-Aviv Center for Brain Functions, Wohl Institute for Advanced Imaging, Tel-Aviv Sourasky Medical Center, Tel-Aviv, Israel
- 2The School of Psychological Sciences, Tel-Aviv University, Tel-Aviv, Israel
- 3The Linguistics Department, The Lester and Sally Entin Faculty of Humanities, Tel-Aviv University, Tel-Aviv, Israel
- 4Faculty of Science and Technology, Bournemouth University, Poole, UK
- 5School of Engineering and Digital Arts, University of Kent, Canterbury, UK
- 6Sagol School of Neuroscience, Tel-Aviv University, Tel-Aviv, Israel
- 7Sackler Faculty of Medicine, Tel-Aviv University, Tel-Aviv, Israel
- 8The Steve Tisch School of Film and Television, Tel-Aviv University, Tel-Aviv, Israel
In the past decade neurofeedback (NF) has become the focus of a growing body of research. With real-time functional magnetic resonance imaging (fMRI) enabling online monitoring of emotion-related areas, such as the amygdala, many have begun testing its therapeutic benefits. However, most existing NF procedures still use monotonic uni-modal interfaces, thus possibly limiting user engagement and weakening learning efficiency. The current study tested a novel multi-sensory NF animated scenario (AS) aimed at enhancing user experience and improving learning. We examined whether relative to a simple uni-modal 2D interface, learning via an interface of complex multi-modal 3D scenario will result in improved NF learning. As a neural-probe, we used the recently developed fMRI-inspired EEG model of amygdala activity (“amygdala-EEG finger print”; amygdala-EFP), enabling low-cost and mobile limbic NF training. Amygdala-EFP was reflected in the AS by the unrest level of a hospital waiting room in which virtual characters become impatient, approach the admission desk and complain loudly. Successful downregulation was reflected as an ease in the room unrest level. We tested whether relative to a standard uni-modal 2D graphic thermometer (TM) interface, this AS could facilitate more effective learning and improve the training experience. Thirty participants underwent two separated NF sessions (1 week apart) practicing downregulation of the amygdala-EFP signal. In the first session, half trained via the AS and half via a TM interface. Learning efficiency was tested by three parameters: (a) effect size of the change in amygdala-EFP following training, (b) sustainability of the learned downregulation in the absence of online feedback, and (c) transferability to an unfamiliar context. Comparing amygdala-EFP signal amplitude between the last and the first NF trials revealed that the AS produced a higher effect size. In addition, NF via the AS showed better sustainability, as indicated by a no-feedback trial conducted in session 2 and better transferability to a new unfamiliar interface. Lastly, participants reported that the AS was more engaging and more motivating than the TM. Together, these results demonstrate the promising potential of integrating realistic virtual environments in NF to enhance learning and improve user’s experience.
Introduction
Neurofeedback (NF) training is defined as learned volitional regulation of one’s own brain activity via a closed-loop reinforcement protocol, usually interfaced by visual or auditory stimuli. The introduction of real-time functional magnetic resonance imaging (rt-fMRI) has generated a growing interest in the clinical and behavioral benefits of the procedure, as it made it possible to target specific anatomical areas, including subcortical regions, such as the amygdala (Johnston et al., 2010). The majority of the research done so far, particularly in fMRI-NF, focused in proving the feasibility of learning to volitionally regulate localized brain activity in different areas (Hamilton et al., 2011; McCaig et al., 2011; Zotev et al., 2011; Lawrence et al., 2014; MacInnes et al., 2016) with some also showing behavioral changes following the NF procedure (Scheinost et al., 2013; Young et al., 2014; Keynan et al., 2016). While importantly proving that individuals can obtain volitional regulation of local blood oxygen level-dependent (BOLD) activity, the research so far has paid little attention to substantial theoretical aspects regarding the learning mechanisms underlying NF training (Sulzer et al., 2013a,b; Thibault et al., 2016).
Neurofeedback learning is commonly considered as a type of associative learning with the online neural feedback providing reinforcement (Birbaumer et al., 2013). Accordingly, the learner explores different mental and cognitive strategies while some are reinforced by the feedback showing successful regulation. Strategies that are repeatedly reinforced are, thus, gradually adopted by the trainee. Viewed as a learning challenge, the design of the NF interface may critically influence its efficiency. In a systematic inquiry of the effects of teaching methods on memorizing and comprehension, Mayer (2009) showed a considerable advantage of multi- over uni-modal techniques in terms of memory and comprehension, which was replicated over about 50 tests. The advantage of multi-modal stimuli has been demonstrated also in various contexts of perceptual learning, including various detection tasks (Gibson and Maunsell, 1997; von Kriegstein and Giraud, 2006; Shams and Seitz, 2008). A recent theoretical model further explains the effect of multi-modal stimuli on learning in terms of integrative processes at the neural population level (van Atteveldt et al., 2014). However, most existing NF paradigms use simple uni-modal 2D graphic simplified representations of brain activity, such as bars, meters, or single tones (Sulzer et al., 2013a,b), composing only one dimension (size/height, volume/tone) in one modality (visual/auditory). This trend does not exploit current developments and increasing availability of virtual environments encouraging the use of multi-modal information presentation, also in the context of NF (Mueller et al., 2012). While simple interfaces may provide the individual with the necessary information in order to learn volitional regulation (Thibault et al., 2016), complex multi-modal interfaces may produce a more effective learning and a more favorable experience (Mishra et al., 2016).
A multi-modal animated interface may be of specific value in the context of NF training. Based on Gallese and Lakoff’s (2005) theory of embodied learning, it may be predicted that an anthropomorphic feedback (i.e., the use of human-like animation) will facilitate better NF performance. These theorists maintain that knowledge is “mapped with our sensory-motor system” so that successful learning relies on embodied simulation of the learned content. Thus, an anthropomorphic feedback that encodes the target process (e.g., amygdala downregulation) as a sensory-motor human-like stimulus may allow for better learning in comparison with a more abstract interface, such as a dynamic thermometer (TM). Such anthropomorphic metaphorical encoding may be specifically beneficial in case of long-term learning in which it can be more easily invoked by mental imagery in the absence of an actual feedback. Furthermore, multi-modal animated scenarios (ASs) may enhance user engagement and motivation, thus, not only preventing dropout but also providing a challenging environment, allowing better realization of individual learning potential (Mishra et al., 2016). Previous work has demonstrated the feasibility of using 3D multi-modal scenarios with rt-fMRI (Mueller et al., 2012; Cavazza et al., 2014). However, prior studies have yet to investigate whether such ASs could facilitate a quantitatively and qualitatively different NF experience with respect to the underlying learning mechanisms.
The current study tested a newly developed NF interface composed of a multi-modal AS designed to enhance learning efficiency (Cavazza et al., 2014). Brain activity in the AS is represented by the unrest level of a group of virtual characters in a virtual hospital waiting room. Unrest manifests itself so that during baseline, the AS is noisy (people shouting and crying) and the characters behave impatiently, trying to reach the admission desk and complain to the helpless secretary (Figure 1B; Video S1 in Supplementary Material). Thus, our AS incorporates both visual (ratio between sitting and standing characters and their bodily gestures) and auditory (soundtrack agitation level) anthropomorphized features to facilitate enhanced embodied cognition. The NF trainees are instructed to find the mental state that corresponds to an ease in the unrest level of the AS (i.e., causes people to seat down calmly). It is explained to the trainees that the unrest level is determined by modulations in their brain activity as recorded online. NF training in the current work targeted downregulation of limbic activity using a novel fMRI-inspired EEG model termed the “amygdala electrical finger print” (amyg-EFP). The amyg-EFP was recently developed in our laboratory to enable accessible limbic targeted NF by applying advanced machine learning algorithms on EEG data acquired simultaneously with fMRI (Meir-Hasson et al., 2014, 2016; Keynan et al., 2016). We examined whether relative to a standard 2D TM visualization, learning via an interface of complex multi-modal 3D scenario will result in a more effective NF learning as measured by three parameters: (a) learning effect size (b) sustainability, and (c) transferability. The learning effect size was used to evaluate the overall effect of the training on the targeted brain activity and was measured by comparing the mean amyg-EFP signal amplitude in the last compared to the first NF trial. Sustainability commonly refers to the time period that participants can retain the learned skill of volitional regulation after the initial training is over, in the absence of online feedback (Thibault et al., 2016). In the current study, we tested sustainability by conducting a no-feedback trial 1 week following the initial training and, additionally, by testing whether the effect exhibited in the first NF training trial could be replicated in a second training trial. Lastly, we tested whether learning in one context could be transferred to a different unfamiliar context. Together these three aspects may be critical in evaluating the possible befits of NF training in daily life outside the laboratory.
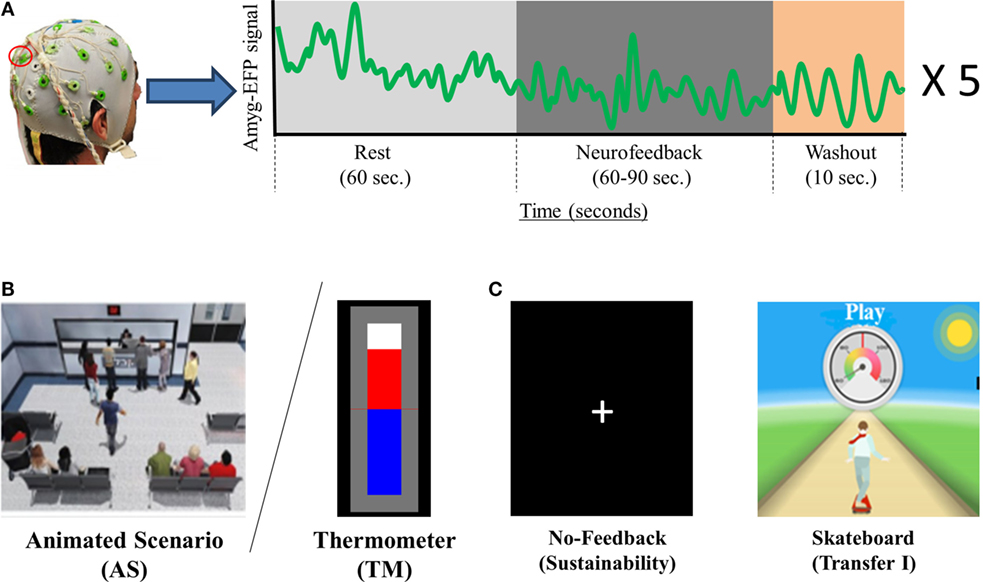
Figure 1. NF training procedure and conditions. (A) Participant’s EEG is continuously recorded via one Pz electrode (marked in red) and momentary amyg-EFP activity is calculated online through rest. NF and washout periods (a time course for one training cycle is shown in the box on the right) (B) The training interfaces: either AS (AS. left) or thermometer (TM. Right). (C) Interfaces presented on the second session. The no-feedback consisted of a dark screen with a fixation cross (left) and the first transfer trial consisted of a 2D moving skateboard interface.
Participants in the current study underwent two separate NF sessions practicing downregulation of the amyg-EFP (see Table 1 for the experimental time line). In the first session, half of the participants trained via the multi-modal AS and half via a standard TM interface. A week later, we tested learning sustainability using a no-feedback trial, followed by a second trial using the same interface as in the first session. Subsequently, we tested participant’s ability to transfer the NF learning to a new context using a third unfamiliar interface (“transfer trial I”). Lastly, each participant practiced NF with the interface initially assigned to the other group (“transfer trial II”). Improvement in the learning effect size was done by comparing the overall mean amyg-EFP signal amplitude during the last relative to the first NF trial in each group. To evaluate differences in the engagement and motivation induced by the different interfaces, participants rated their learning experience in each of the interfaces using the Intrinsic Motivation Inventory (IMI) (Ryan, 1982). We hypothesized that, relative to the TM interface, training via the AS will result in a more effective learning as indicated by effect size, sustainability, and transfer measures. We further hypothesized that the AS interface will be rated as more engaging and more motivating.
Materials and Methods
All experiments and data analysis were conducted at the Tel-Aviv Center for Brain Functions, Wohl Institute for Advanced Imaging, Tel-Aviv Sourasky Medical Center and were approved by the Sourasky ethics review board.
Participants
Thirty-two participants were randomly assigned either to the TM group [n = 16; 8 females, aged (mean ± SD) 27 ± 5] or to the AS group (n = 16; 7 females, aged 26 ± 4). All participants gave written informed consent were healthy, and had normal hearing and normal or corrected-to-normal vision.
Procedure
The experiment consisted in two separate sessions (Table 1). During session 1, the TM group underwent NF training guided by the TM interface and the AS group underwent NF guided by the AS interface. During session 2 (1 week following session 1), in order to test the sustainability of the NF learning obtained in session 1, participants underwent a “no-feedback” trial practicing downregulation of the amyg-EFP without online feedback. Subsequently, participants underwent a second training trial (“training trial II”) practicing NF guided by the same interface as in session 1 (TM for TM group and AS for AS group). Next, participants underwent a transfer trial testing their ability to transfer the NF learning to a different context using an unfamiliar interface (“transfer trial I”), followed by “transfer trial II” in which participants practiced NF guided by the interface originally assigned to the other group (TM for AS group, AS for TM group). Lastly, participants answered the IMI regarding both the AS and the TM interfaces in a counter balanced order. Two participants (1 AS, 1 TM) were unable to meet the study time line and were, thus, excluded from results analysis. The final analysis included 30 subjects (15 AS; 15 TM).
NF Training
The NF trials in both sessions followed a similar block design (see Figure 1A) differing only in the number of cycles. Each cycle comprised one rest block (60 s), one NF block (60–90 s), and one wash-out block (30 s). During rest blocks participants were instructed to passively view the interface animation and were explained that at this time the animation is not influenced by their brain activity. During NF blocks participants were instructed to downregulate amyg-EFP signal amplitude by practicing mental strategies. Instructions were intentionally unspecific, allowing individuals to adopt the mental strategy that they subjectively found most efficient (Shibata et al., 2011). During washout blocks, participants were instructed to tap their thumb to their fingers according to a 3 digit number that appeared on their screen.
During session 1, participants underwent one baseline trial consisting of two cycles with NF block duration of 90 s, followed by a training trial consisting of five cycles with NF block duration of 60 s (“training trial I”). The no-feedback trial at session 2 consisted one 3 min rest block followed by two 60 s long NF blocks. The subsequent second training trial guided by the familiar interface consisted of three cycles with NF block duration of 60 s. Both transfer trial I (skateboard interface) and transfer trial II (TM or AS) consisted of five cycles with NF block duration of 60 s.
The Intrinsic Motivation Inventory
To test for differences in the learning experience between the TM and AS interfaces, we used the IMI questionnaire (Ryan, 1982), a multidimensional inventory, including seven subscales aimed to evaluate the subjective experience induced by an activity in laboratory experiments. Each subscale is scored in a scale of 1–7. In the current research, we used the following subscales:
1. Effort/Importance – How motivated was the participant to succeed in the task and to what extent were they willing to make an effort toward the task?
2. Interest/Enjoyment – How interesting and enjoyable was the task?
3. Value/Usefulness – Did the participant find the learned skill useful to their daily life?
4. Relatedness – To what extent was the participant able to relate to figures/objects presented in the task?
5. Perceived competence – To what extent did the training make the participant feel competent in the skill at hand?
Two of the IMI subscales (perceived choice and felt tension) were not included in the current study as they were not relevant to both interfaces. The questionnaire was translated to Hebrew and adjusted to be compatible to the NF task.
Participants answered the IMI only once, at the end of session 2. Each participant rated both the TM and the AS interfaces in a counter balanced order.
The amyg-EFP Model
The amyg-EFP model was previously developed by our lab in order to enable the prediction of localized activity in the amygdala using EEG only (Meir-Hasson et al., 2014, 2016). This was done by applying machine learning algorithms on EEG data acquired simultaneously with fMRI. This procedure resulted in a Time-Delay × Frequency × weight coefficient matrix. EEG data recorded from electrode Pz at a given time-point is multiplied by the coefficient matrix to produce the predicted amygdala fMRI-BOLD activity at this point. Keynan et al. (2016) validated the reliability of the amyg-EFP in predicting amygdala BOLD activity by conducting further simultaneous EEG–fMRI recordings using a new sample not originally used to develop the model. For further specification, see Meir-Hasson et al. (2014, 2016) and Keynan et al. (2016).
EEG Raw-Data Acquisition
EEG data were acquired using the V-Amp™ EEG amplifier (Brain Products™, Munich Germany) and the BrainCap™ electrode cap with sintered Ag/AgCI ring electrodes providing 16 EEG channels, 1 ECG channel, and 1 EOG channel (Falk Minow Services™, Herrsching-Breitburnn, Germany). The electrodes were positioned according to the standard 10/20 system. The reference electrode was between Fz and Cz. Raw EEG was sampled at 250 Hz and recorded using the Brain Vision Recorder™ software (Brain Products).
Online Calculation of amyg-EFP Amplitude
Raw EEG data were collected online by Brain Vision RecView™ (Brain Products) at a sampling rate of 250 Hz. The RecView software makes it possible to remove cardio-ballistic artifacts from the EEG data in real time using a built-in automated implementation of the average artifact subtraction method (Allen et al., 1998, 2000). RecView™ was custom modified to enable export of the corrected EEG data in real time through a TCP/IP socket. Preprocessing algorithm and amyg-EFP calculation models were compiled from Matlab R2009b™ to Microsoft.NET™ in order to be executed within the Brain Vision RecView™ EEG Recorder system. Data were then transferred to a MATLAB.NET compiled DLL that calculated the value of the EFP amplitude every 3 s.
Feedback Generation
Thermometer
Online amyg-EFP values were transferred from Brain Vision RecView™ to MATLAB™ which in turn set the TM’s height ranging across 30 different levels (Figure 1B). During rest blocks the TM was pre-set to level 15. During NF blocks, the TM’s height was set in accordance to the momentary amyg-EFP value. Mathematically, the height at time point t of the NF block is determined by the probability (p-value) of the amyg-EFP value received at time point t, under the amyg-EFP distribution of the rest block.
EFP(t) is the amyg-EFP value at time point t, and μ(EFPRest) is the mean amyg-EFP value during the previous rest block. σ(EFPRest) is the SD of the amyg-EFP distribution during rest.
Animated Scenario
This interface includes a virtual hospital waiting room that may be either agitated or relaxed (Figure 1B; Video S1 in Supplementary Material). The ratio between characters sitting down and protesting at the counter is considered as a two-state Boltzmann distribution (Atkins and De Paula, 2006), whose evolution is driven by a “virtual temperature” whose value is derived from the momentary value of the amyg-EFP. In equivalence to the TM interface, the AS uses the probability (p-value) of the momentary amyg-EFP value to be sampled under the rest distribution. This p-value is used to determine the probability of virtual characters to be moving in the virtual room (Cavazza et al., 2014). A matching soundtrack, recorded inside a real hospital complements the system output. Three alternative soundtracks with different agitation levels were produced and switched according to the amyg-EFP index. In the rest block, only half of the characters congregate at the front desk also expressing their frustration through body and verbal language. The system is implemented using the Unreal Development Kit (UDK™) game engine, which controls walking animations for individual characters.
Unfamiliar Interface for Transfer Trial I (Skateboard)
This visual interface consisted of a 2D uni-modal flash-based graphic interface of an animated figure standing on a skateboard, skating down a rural road (Figure 1C). During training the participant is asked to control the speed of the skateboard. The feedback is generated in a technically and mathematically similar manner to the TM interface except that the online amyg-EFP value determines speed of the skating figure instead of height of a bar, which is represented through a speedometer on the top and the scrolling speed of the landscape (in one fixed, forward direction). The speed of the skateboard ranged between 50 and 130 km/h. During rest blocks, the skateboard moved at a constant pre-set speed of 90 km/h. During NF blocks the skateboard’s speed was set in accordance to the momentary amyg-EFP value.
Statistical Analysis
For each NF trial, we conducted a two-way repeated measured analysis of variance (ANOVA) with the amyg-EFP as a dependent variable and group (AS vs. TM) and condition (NF vs. rest) as factors. Effect size (Cohen’s d) was measured for the first session by comparing the mean amyg-EFP signal amplitude during training trial I relative to the baseline trial and by conducting a repeated measures ANOVA with the amyg-EFP as a dependent variable and group (AS vs. TM) and trial (baseline vs. training trial I) as factors. The overall improvement in the learning effect size was tested by comparing the mean amyg-EFP signal amplitude during the last NF trial relative to the first NF trial and by conducting a repeated measures ANOVA with the amyg-EFP as a dependent variable and group (AS vs. TM) and trial (first vs. last) as factors. Results of the IMI were analyzed in a similar manner with the self-report scoring as a dependent variable and group (AS vs. TM) and subscale as factors. Post hoc analysis of simple effects was Bonferroni corrected with respect to the number of comparisons in each analysis. All reported p-values are two tailed. Analysis was executed within MATLAB 2013R™ and Statistica 7™. Disrupted EEG recordings (one AS subject in training trial I, one TM subject in training trial II, and one TM subject in transfer trial II) were excluded from analysis of the relevant trial.
Results
Session 1
Baseline Trial
As expected, during the first baseline trial participants of both groups (TM and AS) were yet unable to lower amyg-EFP signal amplitude during NF relative to rest (Figure 2A). A two-way repeated measures ANOVA revealed no effect of condition (NF vs. rest) [F(1,28) = 0.01, p > 0.99] nor group (AS vs. TM) [F(1,28) = 0.24, p > 0.62; AS: rest = −0.41 ± 0.76, NF = −0.39 ± 0.71; TM: rest = −0.28 ± 0.6, NF = −0.3 ± 0.5].
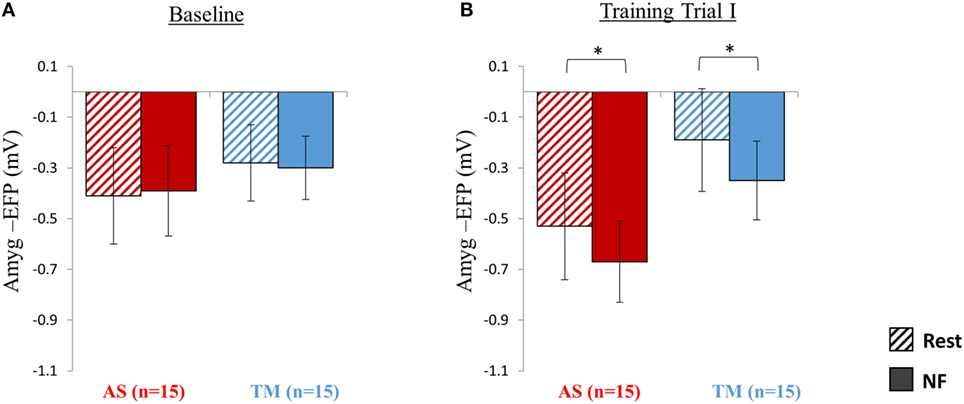
Figure 2. Session 1 NF Training Results. (A) As expected at the first baseline trial (5 min) participants were yet unable to down regulate the amyg-EFP (Y-axis) during NF (solid bars) relative to rest (dashed bars), and no differences were observed between the groups (red bars for AS; blue for TM) (All p > 0.8). (B) By the end of the first session, during the first training trial (15 min), both groups decreased the amyg-EFP signal amplitude during NF relative to rest with no group differences. *p < 0.05.
NF Training Trial I
Analysis of amyg-EFP signal modulations during NF relative to rest indicated that, as hypothesized, both groups learned to downregulate the amyg-EFP during NF relative to rest (Figure 2B). A two-way repeated measures ANOVA revealed a main effect of condition (NF < rest) [F(1,27) = 20.41, p < 0.001; rest = −0.36 ± 0.83, NF = −0.51 ± 0.63] with no interaction between the groups [F(1,27) = 0.05, p > 0.8]. Post hoc analysis (Bonferroni) further indicated that this effect (NF < rest) was significant within both groups (AS: p < 0.02, rest = −0.53 ± 0.84, NF = −0.67 ± 0.64; TM: p < 0.02, rest = −0.19 ± 0.81, NF = −0.35 ± 0.62).
First Session Learning Effect Size
Comparing the mean amyg-EFP signal amplitude during the baseline trial relative to the first training trial at session 1, revealed no difference between the groups in learning effect size [F(1,27) = 1.63, p > 0.2; AS: training I = −0.60 ± 0.82, baseline = −0.40 ± 0.70, Cohen’s d = −0.07; TM: training I = −0.27 ± 0.62, baseline = −0.29 ± 0.53, Cohen’s d = 0.24].
Session 2
Effect Size: First vs. Last NF Trial
As hypothesized, the AS group showed a robustly stronger effect size compared to the TM group (Figure 3). Analysis of mean amyg-EFP signal amplitude for both groups during the last NF trial (transfer trial II) compared to the first NF trial (baseline trial) revealed a significant group by trial interaction [F(1,28) = 4.52, p < 0.05], indicating that only the AS group showed lower amyg-EFP activity during the last relative to the first NF trial [AS: p(Bonferroni) < 0.001; transfer II = −1.56 ± 0.79, baseline = −0.40 ± 0.70, Cohen’s d = −1.56; TM: p(Bonferroni) > 0.90; transfer II = −0.53 ± 1.32, baseline = −0.29 ± 0.53, Cohen’s d = −0.27].
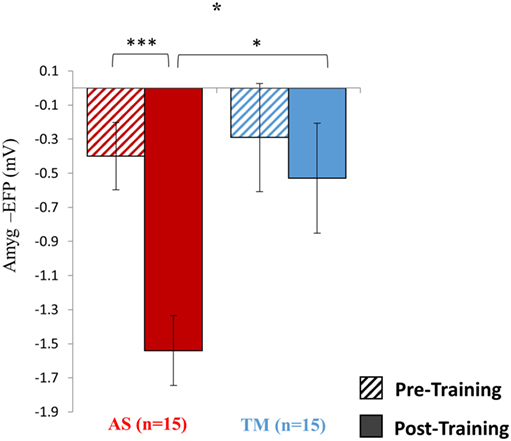
Figure 3. Pre vs. post training results. Training with the AS interface resulted in an overall stronger effect on the targeted brain activity. The figure demonstrates that only the AS group showed a reduction in the mean amyg-EFP signal amplitude (y-axis) during the last (solid fill) compared to the first (dashed fill) NF trial. Furthermore, while pre-training no differences were observed between the groups, during the last NF trial the AS group showed lower amyg-EFP signal amplitude. *p < 0.05, ***p < 0.001.
No-Feedback Trial
As hypothesized, during the no-feedback trial the AS group showed better downregulation relative to the TM group (Figure 4A). A group (AS vs. TM) by condition (NF vs. TM) interaction [F(1,28) = 9.16, p < 0.01] indicated that compared to the TM group, amyg-EFP signal amplitude of the AS group was lower during NF relative to rest. While the AS group showed a trend toward lower amyg-EFP signal amplitude during NF relative to rest [p(Bonferroni) < 0.15, rest = −1.25 ± 0.80, NF = −1.51 ± 0.61], the TM group showed the opposite pattern [p(Bonferroni) < 0.03, rest = −1.13 ± 0.60, NF = −0.93 ± 0.63].
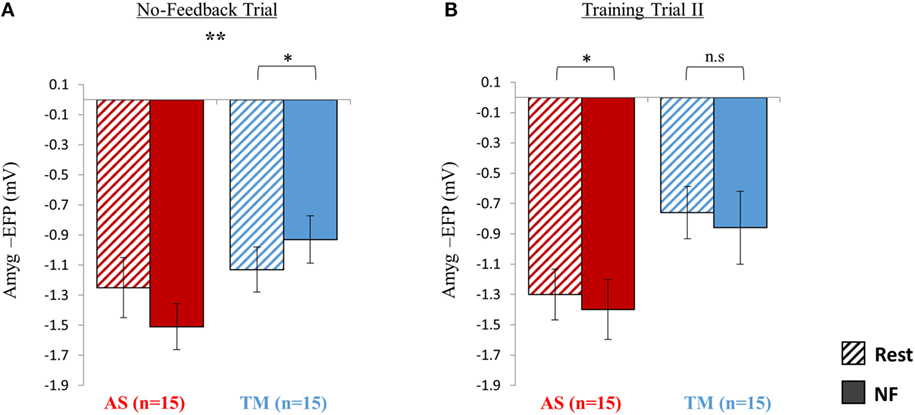
Figure 4. Sustainability testing at session 2. (A) At the no-feedback trial conducted at the beginning of session 2, the AS group (red) exhibited better down regulation of the amyg-EFP (NF relative to rest) compared to the TM group (blue) as indicated by a significant group by condition interaction [F(1,28) = 9.16. p < 0.01] (B) When practicing for the second time with the same interface assigned initially, only the AS group was able to replicate the success of the first training trial. *p < 0.05, **p < 0.01.
Training Trial II
When practicing NF with the familiar interface for the second time, the mean EFP signal amplitude across both groups was lowered during NF relative to rest (Figure 4B); however, this effect was significant only within the AS group. Similarly to training trial I, repeated measures ANOVA revealed a main effect of condition (NF < rest) [F(1,27) = 4.35, p < 0.05; rest = −1.03 ± 0.68, NF = −1.13 ± 0.88] with no interaction between the groups [F(1,27) = 0.001, p > 0.9]. Post hoc analysis (Bonferroni) showed that this effect (NF < rest) was significant only within the AS group (AS: p < 0.05, rest = −1.30 ± 0.67, NF = −1.40 ± 0.79; TM: p > 0.3, rest = −0.76 ± 0.69, NF = −0.86 ± 0.96).
Transfer Trial I
Analysis of the NF trial guided by the skateboard interface interestingly revealed that although both groups successfully downregulated amyg-EFP signal amplitude during NF relative to rest. The AS group exhibited an overall lower amyg-EFP signal amplitude across both conditions (Figure 5A). Repeated measures ANOVA revealed a main effect of group [F(1,28) = 8.66, p < 0.02] indicating that the mean amyg-EFP signal amplitude of the AS group (−1.43 ± 0.76) was lower relative to the TM group (−0.67 ± 0.86). The effect of condition (NF < rest) was also significant [F(1,28) = 120.70, p < 0.001] with post hoc analysis (Bonferroni) indicating that both groups showed reduced amyg-EFP signal amplitude during NF relative to rest (AS: p < 0.001, rest = −1.32 ± 0.76, NF = −1.54 ± 0.82; TM: p < 0.001, rest = −0.52 ± 0.85, NF = −0.82 ± 0.86).
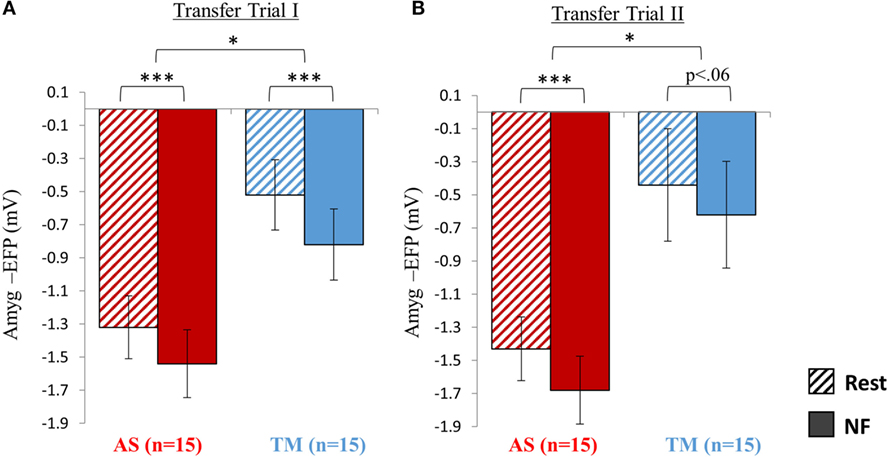
Figure 5. Transfer testing at session 2. (A) When introduced to an unfamiliar context (skateboard interface) although both groups were able to downregulate the amyg-EFP during NF relative to rest, the AS group showed an overall lower amyg-EFP signal amplitude. (B) Similar to the first transfer trial, the AS group showed an overall lower amyg-EFP activity relative to the TM group. The condition effect (NF < rest) was significant only for the AS group. *p < 0.05, **p < 0 01, ***p < 0.001.
Transfer Trial II
Similar to transfer trial I, the AS group showed an overall lower amyg-EFP signal amplitude compared to the TM group [F(1,27) = 6.53, p < 0.02; AS = −1.55 ± 0.77; TM = −0.53 ± 1.325] (Figure 5B). A main effect for condition (NF vs. rest): [F(1,27) = 25.79, p < 0.001] was also found with no group interaction. However, this condition effect (NF < rest) was significant only for the AS group, and marginally significant for the TM group [AS: p(Bonferroni) < 0.001, rest = −1.43 ± 077, NF = −1.68 ± 0.82; TM: p(Bonferroni) < 0.06, rest = −0.44 ± 1.36, NF = −0.62 ± 1.23].
IMI Questionnaire
In line with our hypothesis, a repeated measures ANOVA revealed a main effect of interface [F(1,27) = 13.63, p < 0.001] showing higher ratings for the AS interface (5.37 ± 1.14) relative to the TM interface (4.67 ± 1.25). Post hoc (Bonferroni) analysis further indicated that while participants reported that it was equally important for them to succeed with both interfaces (p > 0.78, AS = 6.13 ± 0.81, TM = 6.18 ± 0.99) the AS interface was rated higher on all of the other subscales (Figure 6): interest/enjoyment (p < 0.03, AS = 5.23 ± 1.29, TM = 4.25 ± 1.29); value/usefulness (p < 0.057, AS = 5.20 ± 1.21, TM = 4.79 ± 1.38); Relatedness (p < 0.05, AS = 5.31 ± 0.75, TM = 4.83 ± 1.10); Perceived competence (p < 0.03, AS = 5.00 ± 1.63, TM = 3.41 ± 1.53). Group assignment (TM or AS) had no effect on individual IMI ratings [F(1,27) = 1.48, p > 0.23].
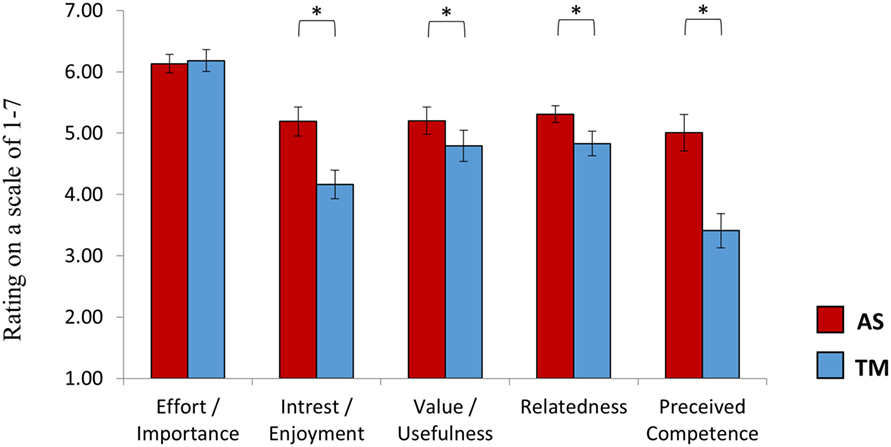
Figure 6. Intrinsic motivation inventory (EMI). At the end of session 2, after practicing NF both with the TM and the AS, participants rated the learning experience induced by each interface. While participants reported that it was equally important for them to succeed with both interfaces, the AS interface was reported more enjoyable and as providing a feedback to which participants could more easily relate. Furthermore, participants reported that they felt more competent following training with the AS interface relative to the TM interface. The interface assigned at the first session had no influence on individual ratings. *p(Bonferroni) < 0.05.
Discussion
The current work introduced a novel multi-modal 3D AS for NF training and demonstrated its advantages over a commonly used uni-modal 2D TM interface. Our results indicate that the NF experience induced by such a virtual scenario may facilitate a stronger and more sustainable learning effect that could be easily transferred to different learning contexts. While participants reported that it was equally important for them to succeed with both interfaces, the AS interface was reported by participants as more engaging and more motivating, and as providing a feedback to which participants could relate to more easily. Moreover, participants reported that they had felt more competent following training with the AS interface compared with the TM interface.
As shown in previous fMRI-NF studies (Sulzer et al., 2013a,b; Bruhl et al., 2014; Lawrence et al., 2014; Paret et al., 2014), we found that volitional regulation of localized limbic activity could be obtained even after one session. Interestingly, comparing the two NF trials conducted in session 1 (baseline vs. training I) revealed that, at this stage, no significant difference existed in the effect size facilitated by the AS relative to the TM (Figure 2). However, the results of the second session clearly demonstrated that relative to the TM interface, NF learning via the AS had a substantially larger effect on the targeted brain activity. Compared to the TM group, the AS group showed a larger reduction in mean amyg-EFP activity during the last relative to the first NF trial (Figure 3). The results further showed that during the last NF trial the AS group showed lower amyg-EFP activity relative to the TM group. This group difference in amyg-EFP activity was not observed pre-training (Figure 2A), thus indicating an overall stronger learning effect of the AS interface. These results are consistent with prior work in other learning domains showing that multi-modal techniques may facilitate better memory and comprehension compared to uni-modal techniques (Gibson and Maunsell, 1997; von Kriegstein and Giraud, 2006; Shams and Seitz, 2008; Mayer, 2009).
In addition to effect size, we tested learning sustainability by conducting a no-feedback trial at the beginning of session 2. The results showed that participants who initially trained via the AS interface were better able to regulate the amyg-EFP in the absence of online feedback (open-loop Brain–Computer Interface), indicating better learning sustainability (Figure 4A). This “sustainability effect” in a no-feedback trial was demonstrated in previous studies, although mostly conducted immediately after one NF session (i.e., at the same day) (deCharms et al., 2004, 2005; Ruiz et al., 2013; Sulzer et al., 2013a,b). In the current study, we further challenged our trainees by conducting the no-feedback trial 1 week following the initial NF session, with no refresher training in between. This better sustainability of the AS group was further demonstrated by the fact that only the AS group were successful in the subsequent “training trial II” in which participants trained NF for the second time via the interface initially assigned at session 1 (Figure 4B). The observed difference in sustainability might be explained by the anthropomorphic metaphorical encoding induced by the AS, that is more easily invoked by mental imagery in the absence of online feedback. The finding that only the AS group maintained the successful performance with the same interface for a second time (“training trial II”) may also be related to the higher potential of multi-modal content to be readily learned, as well as to the more motivating nature of the AS interface, keeping participants engaged on the task at hand. The latter explanation is also consistent with the results of the IMI questionnaire, indicating that the learning experience via the AS interface was rated as more interesting and more enjoyable relative to the TM interface (Figure 6). When aiming toward multiple NF sessions, this engaging factor could be crucial.
To further assess learning efficiency, we tested whether participants could transfer NF learning to a different context using a third unfamiliar interface followed by a second transfer trial at which participants trained with the interface initially assigned to the other group. In a previous study, we have shown that training in one sensory modality (auditory or visual) and context (different interfaces) can be transferred to a completely different context and even a different modality, as long as the same localized brain activity is targeted (Keynan et al., 2016). In the current study, we used an unfamiliar 2D graphic, flash-based interface (skateboard visualization; Figure 1C) to test for differences in transferability of learning between the AS compared to the TM. Comparing the success of the groups in down-regulating amyg-EFP signal amplitude during NF relative to rest baseline revealed no difference between the groups. However, a closer look at the results indicated an overall lower amyg-EFP signal amplitude for the AS group relative to the TM group already at the baseline rest condition (Figure 5A). Not only did the AS group started the first transfer trial with lower amyg-EFP activity at rest baseline but they were able to further lower this activity during NF. In the following second transfer trial, additionally to showing a similar overall lower amyg-EFP amplitude relative to the TM group, only the AS group was able to further lower amyg-EFP amplitude during NF relative to rest (Figure 5B).
One might argue that without a sham control group we cannot infer that the observed modulation in amyg-EFP signal amplitude is due to the feedback and not a result of other intervening factors in the experimental setup (Thibault et al., 2016). It is, therefore, important to note that our previous studies (Keynan et al., 2016; Meir-Hasson et al., 2016) clearly showed that volitional regulation of the amyg-EFP could be learned only when veritable feedback and not sham-feedback is provided. Another possible limitation regards the interface chosen for the transferability testing (skateboard visualization; Figure 1C). As both groups originally trained via visual feedback, testing transfer using another visual feedback may not be strong enough in order to fully reflect the differences between the groups. Using a different sensory modality (auditory), as done in Keynan et al. (2016), could provide a more robust comparison for testing differences in transferability. However, showing that participants of the AS group started the NF transfer trial with a lower amyg-EFP activity at rest baseline and were still able to further downregulate this activity during NF (Figure 5A) is nonetheless intriguing. Future studies should also test differences in behavioral transfer effects following multiple training sessions. It should also be noted that the aim of the present study was to compare the efficiency of an animated NF interface, which is currently successfully used by our lab (Cavazza et al., 2014), with a standard and commonly used feedback format. In light of the evidence that the animated format is preferable to the standard bar feedback, a follow-up research should look into the specific parameters that contribute to this effect. In specific, the multi-modality of the animated interface, its anthropomorphism, and realism (including 3D illusion) could be controlled for and tested for independent effects on the NF efficiency.
To examine the impact of the interface on the training experience, we used the IMI developed by Ryan (1982). This allowed us to reveal several important aspects facilitated by the enhancement of the NF experience (Figure 6). As expected, the AS interface was rated as more interesting and more enjoyable, indicating higher engagement and possibly explaining some of the learning differences discussed earlier. Having a feedback to which participants could relate to, as also indicated by the IMI, probably made the feedback more rewarding, thus, further contributing to the learning process. The most prominent difference was observed in the perceived competence subscale, showing that participants felt more competent in volitional regulation following training via the AS. This stronger relatedness and a feeling of better competence provide empirical support to the notion that a sensory-motor human-like stimulus may allow for better learning in comparison with a more abstract interface, such as a dynamic TM (Gallese and Lakoff, 2005). Considering that the same skill is learned in both interfaces, it was somewhat surprising to find that participants rated learning via the AS as more valuable to daily life. Once again, this could be a result of the AS’s realistic and anthropomorphic nature making it easier for participants to consider how to transfer the learning to real-life situations. Taken together, the results of the IMI questionnaire robustly show that the AS interface facilitated a favorable learning experience that is qualitatively different relative to the TM interface. This enhanced learning experience might induce higher engagement and a stronger feeling of self-competence; both critical for adherence in neuropsychiatric treatment.
Conclusion
Taking advantage of the interactive and engaging qualities of virtual reality media may greatly assist in keeping individuals engaged in the learning effort over a prolonged time period. As the current work indicates, this enhanced NF experience may also yield stronger and more sustainable learning. When applying multiple NF sessions with clinical populations, these factors may critically contribute to the desirable behavioral changes.
Author Contributions
TH, JK, and AC conceived and designed the study. AC conducted the experiments and collected the data. GJ programed the online data analysis methods. NG, IR, and OS assisted in collecting the data. MC, FC, and GR designed the animated scenario. MC and FC programed the animated scenario supporting software. JK analyzed the data. JK, GR, and TH wrote the paper.
Conflict of Interest Statement
The authors declare that the research was conducted in the absence of any commercial or financial relationships that could be construed as a potential conflict of interest.
Funding
The European Union’s Seventh Framework Programmed for research, technological development and demonstration under grant agreement no. 602186. The Ministry of Science, Technology and Space, Israel. The Chief Scientist at the Ministry of Economy, Israel.
Supplementary Material
The Supplementary Material for this article can be found online at http://journal.frontiersin.org/article/10.3389/frobt.2016.00052
References
Allen, P. J., Josephs, O., and Turner, R. A. (2000). Method for removing imaging artifact from continuous EEG recorded during functional MRI. Neuroimage 12, 230–239. doi:10.1006/nimg.2000.0599
Allen, P. J., Pollizi, G., Karkow, K., Fish, D. R., and Lemieux, L. (1998). Identification of EEG events in the MR scanner: the problem of pulse artifact and a method for its subtraction. Neuroimage 8, 229–239. doi:10.1006/nimg.1998.0361
Birbaumer, N., Ruiz, S., and Sitaram, R. (2013). Learned regulation of brain metabolism. Trends Cogn. Sci. 17, 295–302. doi:10.1016/j.tics.2013.04.009
Bruhl, A. B., Scherpiet, S., Sulzer, J., Steampfli, P., Seifritz, E., and Herwig, U. (2014). Real-time neurofeedback using functional MRI could improve down-regulation of amygdala activity during emotional stimulation: a proof-of-concept study. Brain Topogr. 27, 138e148. doi:10.1007/s10548-013-0331-9
Cavazza, M., Charles, F., Aranyi, G., Porteous, J., Gilroy, S. W., and Raz, G. (2014). “Towards emotional regulation through neurofeedback,” in Proceedings of the 5th Augmented Human International Conference (New York: ACM), 42.
deCharms, R. C., Christoff, K., Glover, G. H., Pauly, J. M., Whitfield, S., and Gabrieli, J. D. (2004). Learned regulation of spatially localized brain activation using real-time fMRI. Neuroimage 21, 436–443. doi:10.1016/j.neuroimage.2003.08.041
deCharms, R. C., Maeda, F., Glover, G. H., Ludlow, D., Pauly, J. M., Soneji, D., et al. (2005). Control over brain activation and pain learned by using real-time functional MRI. Proc. Natl. Acad. Sci. U.S.A. 102, 18626. doi:10.1073/pnas.0505210102
Gallese, V., and Lakoff, G. (2005). The brain’s concepts: the role of sensory-motor system in reason and language. Cogn. Neuropsychol. 22, 455–479. doi:10.1080/02643290442000310
Gibson, J. R., and Maunsell, J. H. R. (1997). Sensory modality specificity of neural activity related to memory in visual cortex. J. Neurophysiol. 78, 1263–1275.
Hamilton, J. P., Glover, G. H., Hsu, J. J., Johnson, R. F., and Gotlib, I. H. (2011). Modulation of subgenual anterior cingulate cortex activity with real-time neurofeedback. Hum. Brain Mapp. 32, 22–31. doi:10.1002/hbm.20997
Johnston, S. J., Boehm, S. G., Healy, D., Goebel, R., and Linden, D. E. (2010). Neurofeedback: a promising tool for the self-regulation of emotion networks. Neuroimage 49, 1066–1072. doi:10.1016/j.neuroimage.2009.07.056
Keynan, J. N., Meir-Hasson, Y., Gilam, G., Cohen, A., Jackont, G., Kinreich, S., et al. (2016). Limbic activity modulation guided by functional magnetic resonance imaging-inspired electroencephalography improves implicit emotion regulation. Biol. Psychiatry 80, 490–496. doi:10.1016/j.biopsych.2015.12.024
Lawrence, E. J., Su, L., Barker, G. J., Medford, N., Dalton, J., Williams, S. C., et al. (2014). Self-regulation of the anterior insula: reinforcement learning using real-time fMRI neurofeedback. Neuroimage 88, 113–124. doi:10.1016/j.neuroimage.2013.10.069
MacInnes, J. J., Dickerson, K. C., Chen, N. K., and Adcock, R. A. (2016). Cognitive neurostimulation: learning to volitionally sustain ventral tegmental area activation. Neuron 89, 1331–1342. doi:10.1016/j.neuron.2016.02.002
McCaig, R. G., Dixon, M., Keramatian, K., Liu, I., and Christoff, K. (2011). Improved modulation of rostrolateral prefrontal cortex using real-time fMRI training and meta-cognitive awareness. Neuroimage 55, 1298–1305. doi:10.1016/j.neuroimage.2010.12.016
Meir-Hasson, Y., Keynan, J. N., Kinreich, S., Jackont, G., Cohen, A., Podlipsky-Klovatch, I., et al. (2016). One-class fMRI-inspired EEG model for self-regulation training. PLoS ONE 11:e0154968. doi:10.1371/journal.pone.0154968
Meir-Hasson, Y., Kinreich, S., Podlipsky, I., Hendler, T., and Intrator, N. (2014). An EEG finger-print of fMRI deep regional activation. Neuroimage 102, 128–141. doi:10.1016/j.neuroimage.2013.11.004
Mishra, J., Anguera, J. A., and Gazzaley, A. (2016). Video games for neuro-cognitive optimization. Neuron 90, 214–218. doi:10.1016/j.neuron.2016.04.010
Mueller, C., Luehrs, M., Baecke, S., Adolf, D., Luetzkendorf, R., Luchtmann, M., et al. (2012). Building virtual reality fMRI paradigms: a framework for presenting immersive virtual environments. J. Neurosci. Methods 209, 290–298. doi:10.1016/j.jneumeth.2012.06.025
Paret, C., Kluetsch, R., Ruf, M., Demirakca, T., Hoesterey, S., Ende, G., et al. (2014). Down-regulation of amygdala activation with real-time fMRI neurofeedback in a healthy female sample. Front. Behav. Neurosci. 8:299. doi:10.3389/fnbeh.2014.00299
Ruiz, S., Lee, S., Soekadar, S. R., Caria, A., Veit, R., Kircher, T., et al. (2013). Acquired self-control of insula cortex modulates emotion recognition and brain network connectivity in schizophrenia. Hum. Brain Mapp. 34, 200–212. doi:10.1002/hbm.21427
Ryan, R. M. (1982). Control and information in the intrapersonal sphere: an extension of cognitive evaluation theory. J. Pers. Soc. Psychol. 43, 450–461. doi:10.1037/0022-3514.43.3.450
Scheinost, D., Stoica, T., Saksa, J., Papademetris, X., Constable, R. T., Pittenger, C., et al. (2013). Orbitofrontal cortex neurofeedback produces lasting changes in contamination anxiety and resting-state connectivity. Transl. Psychiatry 3, e250. doi:10.1038/tp.2013.24
Shams, L., and Seitz, A. R. (2008). Benefits of multisensory learning. Trends Cogn. Sci. 12, 411–417. doi:10.1016/j.tics.2008.07.006
Shibata, K., Watanabe, T., Sasaki, Y., and Kawato, M. (2011). Perceptual learning incepted by decoded fMRI neurofeedback without stimulus presentation. Science 334, 1413–1415. doi:10.1126/science.1212003
Sulzer, J., Haller, S., Scharnowski, F., Weiskopf, N., Birbaumer, N., Blefari, M. L., et al. (2013a). Real-time fMRI neurofeedback: progress and challenges. Neuroimage 76, 386–399. doi:10.1016/j.neuroimage.2013.03.033
Sulzer, J., Sitaram, R., Blefari, M. L., Kollias, S., Birbaumer, N., Stephan, K. E., et al. (2013b). Neurofeedback-mediated self-regulation of the dopaminergic midbrain. Neuroimage 75C, 176–184. doi:10.1016/j.neuroimage.2013.02.041
Thibault, R. T., Lifshitz, M., and Raz, A. (2016). The self-regulating brain and neurofeedback: experimental science and clinical promise. Cortex 74, 247–261. doi:10.1016/j.cortex.2015.10.024
van Atteveldt, N., Murray, M. M., Thut, G., and Schroeder, C. E. (2014). Multisensory integration: flexible use of general operations. Neuron 81, 1240–1253. doi:10.1016/j.neuron.2014.02.044
von Kriegstein, K., and Giraud, A. L. (2006). Implicit multisensory associations influence voice recognition. PLoS Biol. 4:e326. doi:10.1371/journal.pbio.0040326
Young, K. D., Zotev, V., Phillips, R., Misaki, M., Yuan, H., Drevets, W. C., et al. (2014). Real-time FMRI neurofeedback training of amygdala activity in patients with major depressive disorder. PLoS ONE 9:e88785. doi:10.1371/journal.pone.0088785
Keywords: EEG–fMRI integration, EEG-neurofeedback, fMRI-neurofeedback, real-time fMRI, amygdala, emotion regulation, interface, virtual reality
Citation: Cohen A, Keynan JN, Jackont G, Green N, Rashap I, Shani O, Charles F, Cavazza M, Hendler T and Raz G (2016) Multi-modal Virtual Scenario Enhances Neurofeedback Learning. Front. Robot. AI 3:52. doi: 10.3389/frobt.2016.00052
Received: 01 May 2016; Accepted: 15 August 2016;
Published: 31 August 2016
Edited by:
Fabien Lotte, INRIA, French Institute for Research in Computer Science and Automation, FranceReviewed by:
Emanuele Ruffaldi, Sant’Anna School of Advanced Studies, ItalyGeorge Papagiannakis, Foundation for Research and Technology Hellas, Greece
Copyright: © 2016 Cohen, Keynan, Jackont, Green, Rashap, Shani, Charles, Cavazza, Hendler and Raz. This is an open-access article distributed under the terms of the Creative Commons Attribution License (CC BY). The use, distribution or reproduction in other forums is permitted, provided the original author(s) or licensor are credited and that the original publication in this journal is cited, in accordance with accepted academic practice. No use, distribution or reproduction is permitted which does not comply with these terms.
*Correspondence: Talma Hendler, hendlert@gmail.com
†Avihay Cohen and Jackob N. Keynan contributed equally to this work.