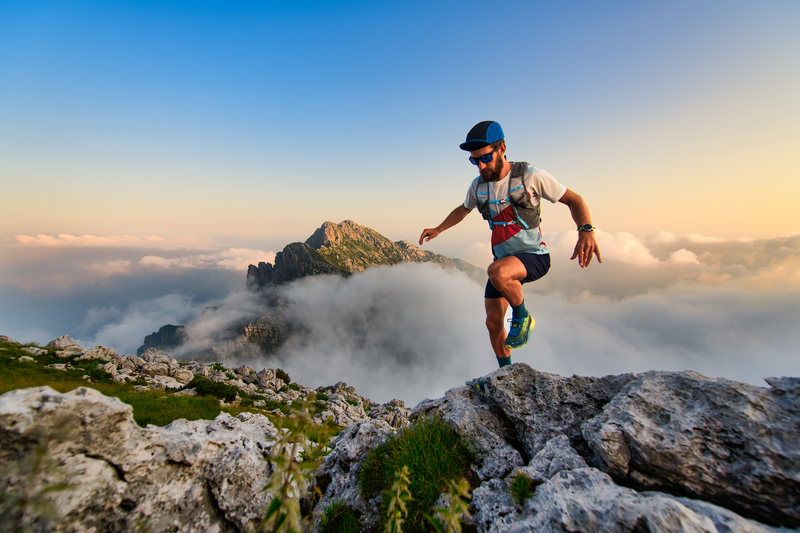
94% of researchers rate our articles as excellent or good
Learn more about the work of our research integrity team to safeguard the quality of each article we publish.
Find out more
BRIEF RESEARCH REPORT article
Front. RNA Res. , 01 October 2024
Sec. Non-coding RNA
Volume 2 - 2024 | https://doi.org/10.3389/frnar.2024.1473293
Long noncoding RNA (lncRNA) genes outnumber protein coding genes in the human genome and the majority remain uncharacterized. A major difficulty in generalizing understanding of lncRNA function is the dearth of gross sequence conservation, both for lncRNAs across species and for lncRNAs that perform similar functions within a species. Machine learning based methods which harness vast amounts of information on RNAs are increasingly used to impute certain biological characteristics. This includes interactions with proteins that are important mediators of RNA function, thus enabling the generation of knowledge in contexts for which experimental data are lacking. Here, we applied a natural language-based machine learning approach that enabled us to identify RNA binding protein interactions in lncRNA transcripts, using only RNA sequence as an input. We found that this predictive method is a powerful approach to infer conserved binding across species as distant as human and opossum, even in the absence of sequence conservation, thus informing on sequence-function relationships for these poorly understood RNAs.
Long noncoding RNAs (lncRNAs) have been associated with a plethora of molecular and cellular functions in both normal and disease processes (Delás and Hannon, 2017; Andergassen and Rinn, 2022; Rinn and Chang, 2012; 2020; Mattick et al., 2023). While many lncRNAs are conventionally transcribed by RNA Polymerase II and therefore bear commonalities with mRNAs including 5′ capping, similar splicing mechanistics, and a poly-A tail, they generally lack linear sequence conservation across species (Mattick et al., 2023; Rinn and Chang, 2020; Quinn and Chang, 2016). Therefore, unlike mRNAs, sequence contexts that have been used to impute conserved protein domains that inform on functionality, are absent for lncRNAs (Mattick et al., 2023). Based on these limitations, general lncRNA classification approaches are lacking, even for lncRNAs with striking functional conservation and impact on organismal biology (Furlan and Rougeulle, 2016). This has implications for classifying newly identified and/or under-characterized lncRNAs.
Given the difficulties in assigning lncRNA function from sequence information, there is a need for methodology to characterize the plethora of uncharacterized lncRNAs in humans and other species (Mattick et al., 2023). Emerging approaches are based on short sequence motifs (Kirk et al., 2018; Ross et al., 2021), which suggest that lncRNAs may be functionally classified based on related motifs/k-mers (Kirk et al., 2018; Ross et al., 2021). These motifs are often present in interaction sites for RNA-binding proteins (RBPs) (Lambert et al., 2014; Kuret et al., 2022), which are critical regulatory mediators and functional partners of lncRNAs (Briata and Gherzi, 2020; Huang et al., 2021; Ferrè et al., 2016; Noh et al., 2018). However, the simple presence or absence of a motif is not sufficient to determine whether an RNA region is a binding site for an RBP ((Van Nostrand et al., 2020b). Additional context determines whether an RBP can bind, and thus predicting RNA-RBP interactions requires methods that can accommodate more contextual information than a motif search alone.
High-throughput approaches have been used to experimentally map RBP-RNA interactions (Ule et al., 2018; Kuret et al., 2022; Van Nostrand et al., 2016; 2020b). These results have been used to provide input for machine learning to generate predictions in the absence of direct experimental data (Pan et al., 2019; Moore and 't Hoen, 2019; Horlacher et al., 2023). Recently self-attention dependent, deep learning methods have shown promise for complex language tasks using massive sequence-based datasets, including entire genomes (Iuchi et al., 2021; Ji et al., 2021). Bidirectional encoder representations from transformer (BERT) has been pretrained on massive corpora of DNA sequence to create DNABERT (Zhou et al., 2023; Ji et al., 2021; Devlin et al., 2018). While DNABERT predicts regulatory regions such as promoters and transcription factor binding sites (Ji et al., 2021; Iuchi et al., 2021), further fine-tuning on RBP-RNA binding data to create BERT-RBP (Yamada and Hamada, 2022), enables prediction of whether relatively short (approximately 100 nucleotide) RNA sequences bind specific RBPs.
We designed an approach to use BERT-RBP (Yamada and Hamada, 2022) to address the lncRNA-functional prediction problem, applying sliding-window segmentation to facilitate RBP-lncRNA interaction predictions in full transcripts. We found we were able to predict conserved lncRNA interactions in multiple species, thereby overcoming roadblocks in lncRNA characterization. Importantly, since it uses only sequence as input, this is an accessible approach that is applicable across a range of species.
The benchmark training dataset was downloaded from RBPSuite (Pan et al., 2020) and consisted of sequences around eCLIP peaks from K562 and HepG2 cell lines for 154 RBPs (Van Nostrand et al., 2016; Kagda et al., 2023). The positive dataset comprised of up to 60,000 sequences of 101 nucleotides (nt), derived from eCLIP peaks for each RBP. The negative dataset consisted of matched regions without any peaks from the same gene. For fine-tuning, we randomly selected 15,000 positive and negative sequences and split them into training, evaluation, and test sets, using scripts from BERT-RBP (Yamada and Hamada, 2022).
BERT-RBP models were fine-tuned from the pretrained 3-mer DNABERT model (Ji et al., 2021) following instructions from Yamada and Hamada 2022 (https://github.com/kkyamada/bert-rbp). We first used default hyperparameters of 4 GPUs with a per-gpu-batch-size of 32 for a total batch size of 128. However, this method gave unstable results as also found by Horlacher et al., 2023 (with 16/154 models lacking any classification ability). We therefore trained additional model sets with batch sizes of 192 and 256 (using 6 and 8 GPUs, respectively), which showed improved but not perfect stability. Based on training differences, we selected models trained with batch sizes of 192, except when their AUROC negatively deviated from previously reported values by more than 0.005, in which case we used the higher performing of the 128 or 256 batch size model (Supplementary Table S1).
BERT-RBP model performance (Yamada and Hamada, 2022) was evaluated using withheld test data, with outputs normalized to a range of 0–1 using Min-Max normalization. Performance metrics including accuracy, precision, recall, F1 score, Matthew’s correlation coefficient, average precision (area under precision-recall curve) and AUROC were calculated using the scikit-learn python package, version 1.3.0 (Supplementary Table S2).
BERT-RBP models predict binding to RNA sequences of 101 nucleotides (Pan et al., 2020; Yamada and Hamada, 2022). To predict binding to full-length RNA transcripts, we generated segments of overlapping 101 nt sequences, used a 10 nt sliding window for segments to overlap neighbors by 90 nt, and ran each segment through the given BERT-RBP model to obtain a binding prediction for that segment. We used Min-Max normalization for model outputs, with minimum and maximum values from model predictions on the test dataset. To facilitate comparisons, we used a 1-D gaussian filter (scipy.ndimage.gaussian_filter1d, scipy version 1.10.1) with a sigma of 20 nt and linearly interpolated results. Regions of the resulting curve above a threshold of 0.9 are classified as binding regions.
All sequences were downloaded with exons and introns demarcated (Kent et al., 2002) as detailed in Supplementary Table S4 using UCSC’s Table Browser tool (Karolchik et al., 2004).
Motif analysis from BERT-RBP models was done similar to prior work (Ji et al., 2021; Yamada and Hamada, 2022), with modifications to how motif candidates were merged. Briefly, attention vectors from the CLS token to the last layer of the model were extracted for each sequence in the test dataset and summed across attention heads. For positive sequences, we selected contiguous 5–10 nt regions where attention was either greater than the average attention for the sequence or greater than 10 times the minimum attention for the sequence. For these putative motifs, we performed a hypergeometric test to test if it occurs more often in positive sequences than in negative ones, kept motifs where the p-value from the hypergeometric test is <0.005 after correction for multiple tests, and merged similar motifs together using an Unweighted Pair Group Method with Arithmetic mean (UPGMA) algorithm. Similarities between each motif were measured using the alignment score from biopython’s Pairwise Aligner. The similarity matrix was then inverted to create a distance matrix, which was put into scipy’s UPGMA clustering algorithm (scipy.cluster.hierarchy.average), with motifs grouped until the distance between groups reached a threshold corresponding to the inversion of a minimum alignment score of 5. For alignment scoring, matching nucleotides scored 2, mismatches scored −1, open and extended gaps scored −0.75, and internal gaps were not allowed. Alignment scores were adjusted for length so that scores for longer motifs were similar to scores for shorter motifs. For each motif group, component sequences were collected and extended to 12 nt. Depictions of motifs were generated from these sequences using WebLogo version 3.7.12.
We fine-tuned DNABERT-derived (Ji et al., 2021), BERT-RBP models following procedures from Yamada and Hamada (2022), with customized hyperparameters (see Methods). Training data originated from ENCODE eCLIP data for 154 RBPs (Pan et al., 2020; Yamada and Hamada, 2022; Van Nostrand et al., 2016), for sequences around eCLIP peak sites in K562 and HepG2 cells as the positive class, and matched genic regions without peaks as the negative class.
We utilized Area Under the Receiver Operating Characteristic (AUROC) scores to evaluate model performance, and scores were comparable to original reports (Yamada and Hamada, 2022) (Yamada and Hamada: 0.786 ± 0.041; In-house: 0.791 ± 0.037, two-sided t-test p-value: 0.250) (Figure 1A).
Figure 1. (A) Violin plots showing distribution of AUROC scores of BERT-RBP models reported by Yamada and Hamada, 2022 (gray), and BERT-RBP models fine-tuned in-house (blue). Black dots indicate scores of individual RBP models. (B) Plots showing precision and recall across thresholds for in-house BERT-RBP models. Shaded area is mean ± standard deviation across RBPs. Yellow, orange, and red lines indicate thresholds of 0.5, 0.7, and 0.9, respectively. (C) Schematic illustrating analysis approach for running predictions on full-length RNA transcripts. Yellow, orange, and red lines are as in (B). Thicker yellow, orange, and red bars denote predicted binding regions using the corresponding threshold. (D) Example plot showing the effect of varying the sub-segment spacing. Points are model outputs of individual segments; lines are smoothed with a gaussian filter (sigma = 20 nt).
BERT-RBP prediction output for a 101 nt RNA sequence is a binding probability ranging from 0 to 1. We selected thresholds to generate binary binding/not-binding decisions from binding probabilities and used precision-recall curves to guide threshold selection decisions (Figure 1B). Lower threshold values prioritize recall while higher threshold values prioritize precision (Figures 1B, C). We used a high threshold of 0.9 to prioritize identification of most-likely binding sites and to minimize false positive predictions.
Given that RNA transcript lengths vary significantly (Quinn and Chang, 2016; Ransohoff et al., 2018; St Laurent et al., 2015; Mattick et al., 2023), we developed a segmentation approach to run predictions on longer sequences using overlapping 101 nt sequence segments and a 10 nt sliding window, based on a balance between output and computational efficiency (Figures 1C, D). Our primary goal was to develop a methodology to guide assessment of lncRNA function. To call predicted binding sites, we passed the model output through a gaussian filter (sigma: 20 nt) (Figures 1C, D), and defined regions above the 0.9 threshold as probable binding sites.
RBPs interact with RNAs to regulate cellular processes such as splicing and translation (Gerstberger et al., 2014; Hentze et al., 2018). We tested predictive ability using the established model of autoregulation of TARDBP via its 3′UTR (Ayala et al., 2011; Sun et al., 2014; Bhardwaj et al., 2013) and successfully predicted interactions over a region of approximately 500 nt in the 3′UTR of TARDBP, similar to a region of enrichment identified in prior work (Wolin et al., 2023; Van Nostrand et al., 2016) (Figure 2A). We found that model-derived TARDBP binding motifs were similar to those previously identified experimentally (Figure 2B). We queried motif locations within predicted interacting sites and found interactions within a 600 nt region enriched with GAAUG and (UG)n repeat motifs (Bhardwaj et al., 2013). Motif sites were within or proximal to predicted binding regions (Figure 2C) and included well-known TARDBP interaction elements including U rich sequences, GU repeats, and the GAAUG motif (Figure 2C), relative to a neighboring region with low probability of predicted interactions (Figure 2C).
Figure 2. (A) Genome browser shots of TARDBP showing eCLIP wiggle tracks and called peaks (top, black) relative to model predictions displayed as a BED track and plot of model output (plot, blue). Points represent the model output for the 101 nt sequence centered on the point. Lines are the result of passing model output through a 1-d gaussian filter with sigma of 20 nt. The lower plot shows predictions zoomed in on the region from Chr1:11,082,300–11,083,952 (region indicated by the gray bar). (B) Motifs identified by attention analysis of BERT-RBP model for TARDBP. (C) Sequence of zoomed-in region of TARDBP mRNA shown in (A). Predicted TARDBP binding sites are highlighted in blue. Red boxes indicate previously identified binding sites. Motifs identified in B are shown in bold. (D) Genome browser shots of VIM RNA and the RBP METAP2 showing eCLIP wiggle tracks and called peaks (top, black) relative to model predictions displayed as BED track and model output plot (blue). (E) Genome browser shots of NDEL1 RNA and the RBP RBFOX2 showing eCLIP wiggle tracks and called peaks (top, black) relative to model predictions displayed as BED track and model output plot (blue). For A, D and E, colors indicate, black: K562 eCLIP, grey: HepG2 eCLIP, blue: BERT-RBP model predictions.
We also examined RBPs with different binding characteristics and functional properties (Van Nostrand et al., 2020b; Briata and Gherzi, 2020) to test the capacity to predict interactions among different RBP/RNAs (Figures 2D, E). Relative to ENCODE eCLIP peaks, we successfully predicted interactions in similar patterns as ENCODE e-CLIP data for METAP2 and VIM (Figure 2D), and RBFOX2 and NDEL1 (Figure 2E) (Van Nostrand et al., 2016; Kagda et al., 2023).
A primary goal of our study was to determine if this predictive approach could inform on functional and/or regulatory aspects of lncRNA biology. To test this, we used highly studied candidate RNAs, beginning with the lncRNA XIST, which has well-defined roles in X-chromosome inactivation (Loda and Heard, 2019; Sahakyan et al., 2018; Furlan and Rougeulle, 2016). Examination of the top 20 predicted interactions revealed RBPs including MATR3, KHSRP, PTBP1, and HNRNPC (Figure 3A) that were previously shown to interact with XIST in various contexts (Minajigi et al., 2015; Chu et al., 2015; Teng et al., 2019; Li et al., 2014). Moreover, model predictions recapitulated enrichment patterns for XIST’s modular repeat domains (Brockdorff, 2002; Brockdorff et al., 2020), with HNRNPC binding within Repeat A, HNRNPK within mid-regions containing Repeats B, C, and D, and PTBP1 within Repeat E (Figure 3B). Interaction sites for another top interactor, MATR3, overlapped with PTBP1’s predicted interaction in Repeat E, similar to prior findings (Pandya-Jones et al., 2020; Jacobson et al., 2022).
Figure 3. (A) Table showing RBPs with most predicted interactions with the human lncRNA XIST as measured by Aggregated Binding, a count of the total number of nucleotides in predicted binding regions. Previously identified XIST-interacting proteins are marked with asterisks. (B) Genome browser shots of the XIST RNA showing eCLIP wiggle tracks and called peaks (top, black) relative to model predictions displayed as BED tracks for RBPs (blue, HNRNPC, HNRNPK, PTBP1, MATR3), established as binding to defined XIST repeat sequences. (C) Table showing RBPs with most predicted interactions with the human lncRNA MALAT1, as in (A). (D) Genome browser shots of the MALAT1 RNA showing eCLIP wiggle tracks and called peaks (top) relative to model predictions displayed as BED tracks for top RBPs in (C) and RBPs (TARDBP, TRA2A) shown to bind previously in eCLIP studies. (E) Table showing top predictions for the human lncRNA H19, as in (A). (F) Genome browser shots of the H19 RNA showing eCLIP wiggle tracks and called peaks (top) relative to model predictions displayed as BED tracks for the top four RBPs. For (B, D, F), colors indicate, black: K562 eCLIP, grey: HepG2 eCLIP, light blue: BERT-RBP model predictions.
MALAT1 differs from XIST in its sequence and structural characteristics, and has roles in splicing, transcription, and as a competing endogenous RNA, with numerous disease implications (Arun et al., 2020; Zhang et al., 2017; Wu et al., 2015). We predicted differentially localized interactions, consistent with different RBP functions (Figures 3C, D), as seen with WDR3 and HLTF and previously described interactors, TARDBP and TRA2A (Van Nostrand et al., 2016; Kagda et al., 2023). This included relatively more 5′-localized predictions for WDR3, with similar predictions for HLTF (Figure 3D). Predictions for HLTF were intriguing since they were similar to CLIP-seq peaks in K562 cells but different from more widespread binding in HepG2 cells. We predicted more discrete 3′ binding for TARDBP, relative to broader binding to the 5′ of the transcript for TRA2A (Figure 3D), similar to experimentally mapped interactions (Van Nostrand et al., 2016; Kagda et al., 2023).
We examined interactions for H19 (Figure 3E), which differs from XIST and MALAT1 based on its shorter length, cytoplasmic subcellular location, and associated roles including functioning as a miRNA precursor (Jonas et al., 2020; Noh et al., 2018; Liang et al., 2015). Among top predicted interactors, GRSF1 is known to bind to lncRNAs as it has been shown to interact with the lncRNA RMRP for its mitochondrial localization, while EWSR1 has been implicated in cancer biology like H19 (Liang et al., 2015; Matouk et al., 2015; Lee et al., 2019). GRSF1 and EWSR1 were predicted to interact at the 5′ end of the transcript, while LARP7 and EIF4G2 were predicted to show more dispersed binding (Figure 3F), similar to that observed for ENCODE e-CLIP.
Altogether, these data suggest that our approach is able to predict RBP interactions with lncRNAs of varying lengths, and different cellular and/or molecular regulatory and functional aspects.
A major gap for the lncRNA field is the ability to predict functional properties for lncRNAs a priori, particularly across species as can be done for mRNAs (Necsulea et al., 2014; Johnsson et al., 2014). One approach to deduce functional conservation could be through identifying conserved interactions with proteins. Since many RNA binding proteins bind through short motifs (Lambert et al., 2014; Kuret et al., 2022), we undertook k-mer content comparisons of human and mouse XIST, MALAT1, and H19 RNAs and found similar sequence composition, particularly for MALAT1 and XIST (Figure 4A), suggesting functional commonalities similar to prior observations (Kirk et al., 2018). However, the sequence content alone did not easily inform on possible functional and/or regulatory interactions, particularly between the species. We postulated that RBP interaction predictions could fill this gap (Huang et al., 2021; Briata and Gherzi, 2020), given that RBPs are functionally conserved across vertebrates (Gerstberger et al., 2014).
Figure 4. (A) K-mer analysis using the SEEKR algorithm on human and mouse XIST, Malat1, and H19 lncRNAs. SEEKR correlation of all human and mouse lncRNAs shown in black/gray (mean ± stdev). Correlation analysis between RBPs predicted to interact with human and mouse Xist (B), Malat1 (C) and H19 (D) lncRNAs. Predicted binding of HNRNPC with human Xist (E), mouse Xist (F), and opossum Rsx (G). Predicted binding of MATR3 with human Xist (H), mouse Xist (I), and opossum Rsx (J). Cartoon illustration of repeats (Xist) or repeat-like areas (Rsx) in yellow (Rep A), orange (Reps B/C/D), and red (Rep E). (K) Table showing the RBPs predicted to have the most binding with Opossum Rsx, as measured by Aggregated Binding, a count of the total number of nucleotides in predicted binding regions. Human Xist and mouse Xist columns indicate whether the RBP was predicted to bind with human and mouse Xist, respectively. Predicted binding of SFPQ (L) and SRSF9 (M) with opossum Rsx. For (E–J, L, M), plots include the predicted binding probability after being passed through a 1D Gaussian filter, and bars (top) indicate called regions where the filtered line passed a threshold of 0.9.
Even though our approach is based on human RBP CLIP-Seq-derived training data (Pan et al., 2020; Ji et al., 2021; Yamada and Hamada, 2022), we sought to determine whether sequence-based machine learning could be used beyond human sequence. We began by examining whether we could predict interactions with mouse sequence and found congruence in predictions of top RBP interactors between mouse and human Xist and Malat1, and less so for H19 (Figures 4B–D). This suggests models’ capacity to predict protein-lncRNA interactions for species beyond human, and to glean potential cross-species differences.
We next examined well-characterized binding characteristics using Xist. Despite profound roles in dosage compensation, there is sequence divergence across human and mouse Xist (see PhyloP conservation, Supplementary Figure S1A). Despite this, for mouse Xist, we found overlap with 15 of the top 20 interacting RBPs identified for human (Figure 4B). The exceptions for mouse were at positions 32 (HNRNPC), 82 (DDX59), 22 (HNRNPK), 72 (CPEB4), and 37 (NSUN2) (Supplementary Figure S2). Differences could be due to model limitations or could point to species-specific interactions and functional differences. Indeed, further examination revealed general similarities in binding as well as species-specific intricacies, including differential binding propensity (Supplementary Figure S2).
We also examined interactions for Malat1 and found common predictions between human and mouse for 17 of the top RBPs including for HLTF and WDR3 (Figure 4C). Interestingly, prediction patterns showed similarity between human and mouse even in 5′ regions of the RNA that lack sequence conservation between human and mouse (Supplementary Figure S1B, see PhyloP track). Binding predictions for WDR3 were enriched in the 5′ region of the transcript, which was also where most HLTF predicted binding was observed, similar to what was seen for K562 enrichment in human MALAT1, and suggesting that this region is a highly regulated MALAT1 segment (Supplementary Figure S1B). Predicted binding patterns for previously shown interactors, TARDBP and TRA2A (Van Nostrand et al., 2016), were also similar to human binding patterns. This confirms the ability of models to identify possible RBP-mediated functional conservation without linear sequence conservation.
Of the top 20 predicted interactions, we found 10 common between human and mouse H19 (Figure 4C). H19’s expression pattern and functions are conserved, with roles in early embryonic development and cancers (Matouk et al., 2015; Jonas et al., 2020; Noh et al., 2018). Even though there were fewer common predictions for H19, we found similar binding patterns between human and mouse for some RBPs, e.g., EWSR1 (Supplementary Figure S1C), suggesting this approach can be used to query cross-species similarities and differences in function.
Using this foundation of human training data facilitating predictions for mouse sequence, we further probed applicability across evolutionary distance. We compared model predictions for human and mouse Xist, particularly repeat region predictions, to Rsx, a lncRNA that mediates X chromosome inactivation in marsupials. Rsx uses mechanisms similar to Xist, including recruitment of silencing factors through repeat domains, despite having no overt linear sequence conservation with Xist (Sahakyan et al., 2018; Loda and Heard, 2019; Furlan and Rougeulle, 2016).
First, as seen with human (Figures 3B, 4E), we found that models successfully predicted commonalities for repeat regions in mouse Xist (Figure 4F), including HNRNPC being predicted to interact with Repeat A, albeit less so in mouse (Figure 4F; Supplementary Figure S2), and MATR3 predicted interactions with Repeat E (Figures 4H, I). Relative to the 5′ Repeat A enrichment of binding to Xist in human and mouse (Figures 4E, F), we also predicted interactions with HNRNPC for opossum Rsx in the 3′ end of the transcript (Figure 4G). Previous work has shown that the repeat domains in the 3′ half of Rsx have similar k-mer content to Repeats A and E of Xist (Grant et al., 2012; Sprague et al., 2019), aligning with our interaction predictions (Figure 4G). Similarly, predictions for interactions with MATR3 were also enriched in the 3′ repeat region of Rsx (Figure 4J), supporting previous findings of similarities between this region and Repeats A and E of Xist.
We found common predicted interactions with Rsx and human and mouse Xist, with prominent RBPs including TRA2A, FUBP3, SFPQ, and HNRNPK (Figure 4K; Supplementary Table S3), which were also recently identified in Rsx interactome mapping (McIntyre et al., 2024). Intriguingly, model predictions also indicated striking differences based on interaction potential between 5′ and 3′ halves (approximately) of Rsx as indicated by SFPQ and SRSF9 interactions (Figures 4L, M), which suggests the ability to predict functional and/or regulatory differences across the transcript.
Altogether, these results between human and mouse Xist, and extending further to marsupial Rsx, suggests our approach could be useful in determining functional conservation or divergence across species, including for syntenic or non-syntenic lncRNAs.
Deep learning-based approaches are increasingly used to characterize biological molecules, including for the prediction of intermolecular interactions (Horlacher et al., 2023; Moore and 't Hoen, 2019; Pan et al., 2019; Yamada and Hamada, 2022). Using BERT-RBP, we leveraged natural language processing principles applicable to genome-wide nucleic acid sequence contexts (Ji et al., 2021; Zhou et al., 2023; Yamada and Hamada, 2022), to design a practical and applicable machine learning-based approach to study lncRNAs, since they remain poorly understood.
Foundationally, we tested the predictive utility using well-established RBP-mRNA interactions. We then found that predictions for lncRNAs aligned with prior interactions observed in experimentation, including CLIP-Seq and mass spectrometry-based analyses (Pandya-Jones et al., 2020; Yi et al., 2020; Chu et al., 2015; McHugh et al., 2015; Minajigi et al., 2015; Van Nostrand et al., 2016). Altogether therefore, we successfully predicted both coding- and noncoding RNA-RBP interactions, capturing differing functions, gene lengths, and regulatory qualities. Since lncRNA genes outnumber mRNA genes in the human genome (Quinn and Chang, 2016), this approach can provide a foundation for de novo predictions for uncharacterized lncRNAs to generate candidate interactions to guide experimentation such as in vivo perturbation analysis via CRISPR. We anticipate that additional training data including from complementary approaches (Wolin et al., 2023) or cell- and/or context-specific binding data would support broader prediction of interactions.
Our predictive approach can fill experimental gaps by imputing protein binding sites for transcripts showing little to no expression, as has been observed for many lncRNAs (Rinn and Chang, 2020; St Laurent et al., 2015; Mattick et al., 2023). To capture such variation, models may require additional training data, with a major limitation of these approaches being differences in training data sets used by different research groups (Horlacher et al., 2023). Inherent data quality and predictive issues are limited by experimentally queried RBPs, experimental CLIP protocols and associated limitations including resolution, and computational analysis design including training and evaluation parameters. Deviation between CLIP-Seq and our model predictions may be due to our decision to prioritize precision vs. recall, or other elements influencing model optimization including RNA structure or combinations of RBP interactions (Sun et al., 2021).
Importantly, as shown for Rsx, our approach can be used for lncRNAs that may have convergently evolved similar function, or to inform on the function of syntenic lncRNAs, both cases in which sequence conservation across species is lacking (Ulitsky, 2016; Necsulea et al., 2014; Johnsson et al., 2014). While an abundance of experimental data such as CLIP-seq for numerous proteins exists for human samples (Van Nostrand et al., 2020a; Gerstberger et al., 2014), such data are often limiting for other species, even for conventional animal models such as mouse, and more so for more divergent or non-model species. Our approach therefore provides a foundation for further study across many organisms since RBP properties are generally evolutionarily conserved.
Interestingly, we found that some predictions aligned preferentially with CLIP-Seq data from a specific cell line (e.g., in HepG2 but not K562). Such contextual specificity is anticipated given that CLIP-Seq output is dependent on protein target abundance in a given cell type (Van Nostrand et al., 2020a; Van Nostrand et al., 2016; Van Nostrand et al., 2020b). Our results therefore suggest that models can detect contextual elements for specific RBP-RNA interactions, with only sequence data as an input. These intricacies are important considerations for positive and negative labels in classification methods, as a negative sequence in a specific cell type may not be negative in another. Future work may determine whether models effectively learn cell-specific interactions to identify a spectrum of interactions that may be missed in a single study, e.g., due to cell type, experimental timing, or experimental technique limitations.
Since model fine-tuning produces a set of weights that provide sufficient solutions for the given task, different sets of weights can create models with similar overall performance, but which vary in their response to a particular input. Therefore, one way to improve our approach would be to create an ensemble of models, either with additional instances of BERT-RBP models that have been trained with a different subset of training data or have been initialized with different random seeds, or with additional types of models. Additional improvements might be obtained by converting these models from binary classifiers to multi-class models.
Analysis parameters can be adjusted to favor increased precision to capture high-confidence interactions vs. recall to identify all potential binding sites within a specific transcript more comprehensively. Future work to experimentally validate predictions will aid in setting thresholds and analysis parameters, and studies characterizing validated interactions will enhance our understanding of how RBP-lncRNA interactions direct lncRNA function. Predictions that consider combinatorial RBP interactions would also increase the capacity to predict RBP impact on associated RNAs, to influence the capacity to predict more granular facets of lncRNA biology.
A major advantage of our approach is that predictions are based solely on sequence, lowering the barrier for lncRNA characterization, which is of particular importance since most lncRNAs are uncharacterized with limited/no experimental data. Moreover, our easy-to-use method bridges the gap between computational fields focused on developing machine learning algorithms, and wet lab work, by providing experimental targets for biochemistry and molecular biology studies.
The data presented in the study are deposited in the figshare repository, accession number doi.org/10.6084/m9.figshare.27022657.v1.
MK: Conceptualization, Data curation, Formal Analysis, Investigation, Methodology, Software, Validation, Visualization, Writing–original draft, Writing–review and editing. KS: Conceptualization, Formal Analysis, Funding acquisition, Investigation, Methodology, Project administration, Resources, Supervision, Visualization, Writing–original draft, Writing–review and editing.
The author(s) declare that financial support was received for the research, authorship, and/or publication of this article. This work was supported by the Hypothesis Fund, the National Science Foundation (award numbers: 2243666, 2243562), and the School of Medicine at the University of North Carolina at Chapel Hill.
We thank Colin Raffel and Joshua Starmer for feedback on model optimization, and Prabuddha Chakrabotry, Dominic Ciavatta, Noel Murcia, and Karl Shpargel for critical comments on the manuscript.
The authors declare that the research was conducted in the absence of any commercial or financial relationships that could be construed as a potential conflict of interest.
All claims expressed in this article are solely those of the authors and do not necessarily represent those of their affiliated organizations, or those of the publisher, the editors and the reviewers. Any product that may be evaluated in this article, or claim that may be made by its manufacturer, is not guaranteed or endorsed by the publisher.
The Supplementary Material for this article can be found online at: https://www.frontiersin.org/articles/10.3389/frnar.2024.1473293/full#supplementary-material
SUPPLEMENTARY FIGURE S1 | (A) Genome browser shots of the mouse Xist RNA showing conservation (PhyloP) and model predictions displayed as BED tracks for HNRNPC, HNRNPK, PTBP1, and MATR3, established interactors with Xist. (B) Genome browser shots of the mouse Malat1 RNA showing conservation (PhyloP) and model predictions displayed as BED tracks. (C) Genome browser shots of the mouse H19 RNA showing conservation (PhyloP) and model predictions displayed as BED tracks.
SUPPLEMENTARY FIGURE S2 | Genome browser shots illustrating RBPs which are predicted to have differential interaction propensity with the XIST RNA in human or mouse, respectively.
SUPPLEMENTARY TABLE S1 | Training Hyperparameters.
SUPPLEMENTARY TABLE S2 | Model performance metrics.
SUPPLEMENTARY TABLE S3 | Binding predictions for Opossum Rsx.
SUPPLEMENTARY TABLE S4 | RNA Sequence information.
Andergassen, D., and Rinn, J. L. (2022). From genotype to phenotype: genetics of mammalian long non-coding RNAs in vivo. Nat. Rev. Genet. 23, 229–243. doi:10.1038/s41576-021-00427-8
Arun, G., Aggarwal, D., and Spector, D. L. (2020). MALAT1 long non-coding RNA: functional implications. Noncoding RNA 6, 22. doi:10.3390/ncrna6020022
Ayala, Y. M., De Conti, L., Avendaño-Vázquez, S. E., Dhir, A., Romano, M., D’Ambrogio, A., et al. (2011). TDP-43 regulates its mRNA levels through a negative feedback loop. EMBO J. 30, 277–288. doi:10.1038/emboj.2010.310
Bhardwaj, A., Myers, M. P., Buratti, E., and Baralle, F. E. (2013). Characterizing TDP-43 interaction with its RNA targets. Nucleic Acids Res. 41, 5062–5074. doi:10.1093/nar/gkt189
Briata, P., and Gherzi, R. (2020). Long non-coding RNA-ribonucleoprotein networks in the post-transcriptional control of gene expression. Noncoding RNA 6, 40. doi:10.3390/ncrna6030040
Brockdorff, N. (2002). X-chromosome inactivation: closing in on proteins that bind Xist RNA. Trends Genet. 18, 352–358. doi:10.1016/s0168-9525(02)02717-8
Brockdorff, N., Bowness, J. S., and Wei, G. (2020). Progress toward understanding chromosome silencing by Xist RNA. Genes Dev. 34, 733–744. doi:10.1101/gad.337196.120
Chu, C., Zhang, Q. C., da Rocha, S., Flynn, R. A., Bharadwaj, M., Calabrese, J. M., et al. (2015). Systematic discovery of Xist RNA binding proteins. Cell 161, 404–416. doi:10.1016/j.cell.2015.03.025
Delás, M. J., and Hannon, G. J. (2017). lncRNAs in development and disease: from functions to mechanisms. Open Biol. 7, 170121. doi:10.1098/rsob.170121
Devlin, J., Chang, M.-W., Lee, K., and Toutanova, K. (2018). BERT: pre-training of deep bidirectional transformers for language understanding. arXiv. doi:10.48550/arxiv.1810.04805
Ferrè, F., Colantoni, A., and Helmer-Citterich, M. (2016). Revealing protein–lncRNA interaction. Briefings Bioinforma. 17, 106–116. doi:10.1093/bib/bbv031
Furlan, G., and Rougeulle, C. (2016). Function and evolution of the long noncoding RNA circuitry orchestrating X-chromosome inactivation in mammals. WIREs RNA 7, 702–722. doi:10.1002/wrna.1359
Gerstberger, S., Hafner, M., and Tuschl, T. (2014). A census of human RNA-binding proteins. Nat. Rev. Genet. 15, 829–845. doi:10.1038/nrg3813
Grant, J., Mahadevaiah, S. K., Khil, P., Sangrithi, M. N., Royo, H., Duckworth, J., et al. (2012). Rsx is a metatherian RNA with Xist-like properties in X-chromosome inactivation. Nature 487, 254–258. doi:10.1038/nature11171
Hentze, M. W., Castello, A., Schwarzl, T., and Preiss, T. (2018). A brave new world of RNA-binding proteins. Nat. Rev. Mol. Cell Biol. 19, 327–341. doi:10.1038/nrm.2017.130
Horlacher, M., Cantini, G., Hesse, J., Schinke, P., Goedert, N., Londhe, S., et al. (2023). A systematic benchmark of machine learning methods for protein-RNA interaction prediction. Briefings Bioinforma. 24, bbad307. doi:10.1093/bib/bbad307
Huang, Y., Qiao, Y., Zhao, Y., Li, Y., Yuan, J., Zhou, J., et al. (2021). Large scale RNA-binding proteins/LncRNAs interaction analysis to uncover lncRNA nuclear localization mechanisms. Briefings Bioinforma. 22, bbab195. doi:10.1093/bib/bbab195
Iuchi, H., Matsutani, T., Yamada, K., Iwano, N., Sumi, S., Hosoda, S., et al. (2021). Representation learning applications in biological sequence analysis. Comput. Struct. Biotechnol. J. 19, 3198–3208. doi:10.1016/j.csbj.2021.05.039
Jacobson, E. C., Pandya-Jones, A., and Plath, K. (2022). A lifelong duty: how Xist maintains the inactive X chromosome. Curr. Opin. Genet. and Dev. 75, 101927. doi:10.1016/j.gde.2022.101927
Ji, Y., Zhou, Z., Liu, H., and Davuluri, R. V. (2021). DNABERT: pre-trained bidirectional encoder representations from transformers model for DNA-language in genome. Bioinformatics 37, 2112–2120. doi:10.1093/bioinformatics/btab083
Johnsson, P., Lipovich, L., Grandér, D., and Morris, K. V. (2014). Evolutionary conservation of long non-coding RNAs; sequence, structure, function. Biochimica Biophysica Acta (BBA) - General Subj. 1840, 1063–1071. doi:10.1016/j.bbagen.2013.10.035
Jonas, K., Calin, G. A., and Pichler, M. (2020). RNA-binding proteins as important regulators of long non-coding RNAs in cancer. Int. J. Mol. Sci. 21, 2969. doi:10.3390/ijms21082969
Kagda, M. S., Lam, B., Litton, C., Small, C., Sloan, C. A., Spragins, E., et al. (2023). Data navigation on the ENCODE portal. arXiv. doi:10.48550/arxiv.2305.00006
Karolchik, D., Hinrichs, A. S., Furey, T. S., Roskin, K. M., Sugnet, C. W., Haussler, D., et al. (2004). The UCSC Table Browser data retrieval tool. Nucleic Acids Res. 32, D493–D496. doi:10.1093/nar/gkh103
Kent, W. J., Sugnet, C. W., Furey, T. S., Roskin, K. M., Pringle, T. H., Zahler, A. M., et al. (2002). The human genome browser at UCSC. Genome Res. 12, 996–1006. doi:10.1101/gr.229102
Kirk, J. M., Kim, S. O., Inoue, K., Smola, M. J., Lee, D. M., Schertzer, M. D., et al. (2018). Functional classification of long non-coding RNAs by k-mer content. Nat. Genet. 50, 1474–1482. doi:10.1038/s41588-018-0207-8
Kuret, K., Amalietti, A. G., Jones, D. M., Capitanchik, C., and Ule, J. (2022). Positional motif analysis reveals the extent of specificity of protein-RNA interactions observed by CLIP. Genome Biol. 23, 191. doi:10.1186/s13059-022-02755-2
Lambert, N., Robertson, A., Jangi, M., McGeary, S., Sharp, P. A., and Burge, C. B. (2014). RNA Bind-n-Seq: quantitative assessment of the sequence and structural binding specificity of RNA binding proteins. Mol. Cell 54, 887–900. doi:10.1016/j.molcel.2014.04.016
Lee, J., Nguyen, P. T., Shim, H. S., Hyeon, S. J., Im, H., Choi, M.-H., et al. (2019). EWSR1, a multifunctional protein, regulates cellular function and aging via genetic and epigenetic pathways. Biochimica Biophysica Acta (BBA) - Mol. Basis Dis. 1865, 1938–1945. doi:10.1016/j.bbadis.2018.10.042
Li, J.-H., Liu, S., Zhou, H., Qu, L.-H., and Yang, J.-H. (2014). starBase v2.0: decoding miRNA-ceRNA, miRNA-ncRNA and protein-RNA interaction networks from large-scale CLIP-Seq data. Nucleic Acids Res. 42, D92–D97. doi:10.1093/nar/gkt1248
Liang, W.-C., Fu, W.-M., Wong, C.-W., Wang, Y., Wang, W.-M., Hu, G.-X., et al. (2015). The lncRNA H19 promotes epithelial to mesenchymal transition by functioning as miRNA sponges in colorectal cancer. Oncotarget 6, 22513–22525. doi:10.18632/oncotarget.4154
Loda, A., and Heard, E. (2019). Xist RNA in action: past, present, and future. PLoS Genet. 15, e1008333. doi:10.1371/journal.pgen.1008333
Matouk, I. J., Halle, D., Gilon, M., and Hochberg, A. (2015). The non-coding RNAs of the H19-IGF2 imprinted loci: a focus on biological roles and therapeutic potential in Lung Cancer. J. Transl. Med. 13, 113. doi:10.1186/s12967-015-0467-3
Mattick, J. S., Amaral, P. P., Carninci, P., Carpenter, S., Chang, H. Y., Chen, L.-L., et al. (2023). Long non-coding RNAs: definitions, functions, challenges and recommendations. Nat. Rev. Mol. Cell Biol. 24, 430–447. doi:10.1038/s41580-022-00566-8
McHugh, C. A., Chen, C.-K., Chow, A., Surka, C. F., Tran, C., McDonel, P., et al. (2015). The Xist lncRNA interacts directly with SHARP to silence transcription through HDAC3. Nature 521, 232–236. doi:10.1038/nature14443
McIntyre, K. L., Waters, S. A., Zhong, L., Hart-Smith, G., Raftery, M., Chew, Z. A., et al. (2024). Identification of the RSX interactome in a marsupial shows functional coherence with the Xist interactome during X inactivation. Genome Biol. 25, 134. doi:10.1186/s13059-024-03280-0
Minajigi, A., Froberg, J., Wei, C., Sunwoo, H., Kesner, B., Colognori, D., et al. (2015). Chromosomes. A comprehensive Xist interactome reveals cohesin repulsion and an RNA-directed chromosome conformation. Science 349. doi:10.1126/science.aab2276
Moore, K. S., and 't Hoen, P. A. C. (2019). Computational approaches for the analysis of RNA-protein interactions: a primer for biologists. J. Biol. Chem. 294, 1–9. doi:10.1074/jbc.REV118.004842
Necsulea, A., Soumillon, M., Warnefors, M., Liechti, A., Daish, T., Zeller, U., et al. (2014). The evolution of lncRNA repertoires and expression patterns in tetrapods. Nature 505, 635–640. doi:10.1038/nature12943
Noh, J. H., Kim, K. M., McClusky, W. G., Abdelmohsen, K., and Gorospe, M. (2018). Cytoplasmic functions of long noncoding RNAs. Wiley Interdiscip. Rev. RNA 9, e1471. doi:10.1002/wrna.1471
Pan, X., Fang, Y., Li, X., Yang, Y., and Shen, H.-B. (2020). RBPsuite: RNA-protein binding sites prediction suite based on deep learning. BMC Genomics 21, 884. doi:10.1186/s12864-020-07291-6
Pan, X., Yang, Y., Xia, C.-Q., Mirza, A. H., and Shen, H.-B. (2019). Recent methodology progress of deep learning for RNA-protein interaction prediction. Wiley Interdiscip. Rev. RNA 10, e1544. doi:10.1002/wrna.1544
Pandya-Jones, A., Markaki, Y., Serizay, J., Chitiashvili, T., Mancia Leon, W. R., Damianov, A., et al. (2020). A protein assembly mediates Xist localization and gene silencing. Nature 587, 145–151. doi:10.1038/s41586-020-2703-0
Quinn, J. J., and Chang, H. Y. (2016). Unique features of long non-coding RNA biogenesis and function. Nat. Rev. Genet. 17, 47–62. doi:10.1038/nrg.2015.10
Ransohoff, J. D., Wei, Y., and Khavari, P. A. (2018). The functions and unique features of long intergenic non-coding RNA. Nat. Rev. Mol. Cell Biol. 19, 143–157. doi:10.1038/nrm.2017.104
Rinn, J. L., and Chang, H. Y. (2012). Genome regulation by long noncoding RNAs. Annu. Rev. Biochem. 81, 145–166. doi:10.1146/annurev-biochem-051410-092902
Rinn, J. L., and Chang, H. Y. (2020). Long noncoding rnas: molecular modalities to organismal functions. Annu. Rev. Biochem. 89, 283–308. doi:10.1146/annurev-biochem-062917-012708
Ross, C. J., Rom, A., Spinrad, A., Gelbard-Solodkin, D., Degani, N., and Ulitsky, I. (2021). Uncovering deeply conserved motif combinations in rapidly evolving noncoding sequences. Genome Biol. 22, 29. doi:10.1186/s13059-020-02247-1
Sahakyan, A., Yang, Y., and Plath, K. (2018). The role of xist in X-chromosome dosage compensation. Trends Cell Biol. 28, 999–1013. doi:10.1016/j.tcb.2018.05.005
Sprague, D., Waters, S. A., Kirk, J. M., Wang, J. R., Samollow, P. B., Waters, P. D., et al. (2019). Nonlinear sequence similarity between the Xist and Rsx long noncoding RNAs suggests shared functions of tandem repeat domains. RNA 25, 1004–1019. doi:10.1261/rna.069815.118
St Laurent, G., Wahlestedt, C., and Kapranov, P. (2015). The Landscape of long noncoding RNA classification. Trends Genet. 31, 239–251. doi:10.1016/j.tig.2015.03.007
Sun, Y., Arslan, P. E., Won, A., Yip, C. M., and Chakrabartty, A. (2014). Binding of TDP-43 to the 3’UTR of its cognate mRNA enhances its solubility. Biochemistry 53, 5885–5894. doi:10.1021/bi500617x
Sun, L., Xu, K., Huang, W., Yang, Y. T., Li, P., and Tang, L. (2021). Predicting dynamic cellular protein-RNA interactions by deep learning using in vivo RNA structures. Cell Res. 31, 495–516. doi:10.1038/s41422-021-00476-y
Teng, X., Chen, X., Xue, H., Tang, Y., Zhang, P., Kang, Q., et al. (2020). NPInter v4.0: an integrated database of ncRNA interactions. Nucleic Acids Res. 48, D160–D165. doi:10.1093/nar/gkz969
Ule, J., Hwang, H.-W., and Darnell, R. B. (2018). The future of cross-linking and immunoprecipitation (CLIP). Cold Spring Harb. Perspect. Biol. 10, a032243. doi:10.1101/cshperspect.a032243
Ulitsky, I. (2016). Evolution to the rescue: using comparative genomics to understand long non-coding RNAs. Nat. Rev. Genet. 17, 601–614. doi:10.1038/nrg.2016.85
Van Nostrand, E. L., Freese, P., Pratt, G. A., Wang, X., Wei, X., Xiao, R., et al. (2020a). A large-scale binding and functional map of human RNA-binding proteins. Nature 583, 711–719. doi:10.1038/s41586-020-2077-3
Van Nostrand, E. L., Pratt, G. A., Shishkin, A. A., Gelboin-Burkhart, C., Fang, M. Y., Sundararaman, B., et al. (2016). Robust transcriptome-wide discovery of RNA-binding protein binding sites with enhanced CLIP (eCLIP). Nat. Methods 13, 508–514. doi:10.1038/nmeth.3810
Van Nostrand, E. L., Pratt, G. A., Yee, B. A., Wheeler, E. C., Blue, S. M., Mueller, J., et al. (2020b). Principles of RNA processing from analysis of enhanced CLIP maps for 150 RNA binding proteins. Genome Biol. 21, 90. doi:10.1186/s13059-020-01982-9
Wolin, E., Guo, J. K., Blanco, M. R., Perez, A. A., Goronzy, I. N., Abdou, A. A., et al. (2023). SPIDR: a highly multiplexed method for mapping RNA-protein interactions uncovers a potential mechanism for selective translational suppression upon cellular stress. BioRxiv, 543769. doi:10.1101/2023.06.05.543769
Wu, Y., Huang, C., Meng, X., and Li, J. (2015). Long noncoding RNA MALAT1: insights into its biogenesis and implications in human disease. Curr. Pharm. Des. 21, 5017–5028. doi:10.2174/1381612821666150724115625
Yamada, K., and Hamada, M. (2022). Prediction of RNA-protein interactions using a nucleotide language model. Bioinforma. Adv. 2, vbac023. doi:10.1093/bioadv/vbac023
Yi, W., Li, J., Zhu, X., Wang, X., Fan, L., Sun, W., et al. (2020). CRISPR-assisted detection of RNA-protein interactions in living cells. Nat. Methods 17, 685–688. doi:10.1038/s41592-020-0866-0
Zhang, X., Hamblin, M. H., and Yin, K.-J. (2017). The long noncoding RNA Malat1: its physiological and pathophysiological functions. RNA Biol. 14, 1705–1714. doi:10.1080/15476286.2017.1358347
Keywords: long noncoding RNA, RNA binding protein, machine learning, deep learning, natural language processing, CLIP-seq
Citation: Kratz MB and Smith KN (2024) Predicting conserved functional interactions for long noncoding RNAs via deep learning. Front. RNA Res. 2:1473293. doi: 10.3389/frnar.2024.1473293
Received: 30 July 2024; Accepted: 09 September 2024;
Published: 01 October 2024.
Edited by:
Simranjeet Kaur, Steno Diabetes Center Copenhagen (SDCC), DenmarkReviewed by:
Yiliang Ding, John Innes Centre, United KingdomCopyright © 2024 Kratz and Smith. This is an open-access article distributed under the terms of the Creative Commons Attribution License (CC BY). The use, distribution or reproduction in other forums is permitted, provided the original author(s) and the copyright owner(s) are credited and that the original publication in this journal is cited, in accordance with accepted academic practice. No use, distribution or reproduction is permitted which does not comply with these terms.
*Correspondence: Keriayn N. Smith, a25zQGVtYWlsLnVuYy5lZHU=
Disclaimer: All claims expressed in this article are solely those of the authors and do not necessarily represent those of their affiliated organizations, or those of the publisher, the editors and the reviewers. Any product that may be evaluated in this article or claim that may be made by its manufacturer is not guaranteed or endorsed by the publisher.
Research integrity at Frontiers
Learn more about the work of our research integrity team to safeguard the quality of each article we publish.