- Department of Computational Biology, Indraprastha Institute of Information Technology, New Delhi, India
Long non-coding RNAs (lncRNAs) play a vital role in biological processes, and their dysfunctions lead to a wide range of diseases. Due to advancements in sequencing technology, more than 20,000 lncRNA transcripts have been identified in humans, almost equivalent to coding transcripts. One crucial aspect in annotating lncRNA function is predicting their subcellular localization, which often determines their functional roles within cells. This review aims to cover the experimental techniques, databases, and in silico tools developed for identifying subcellular localization. Firstly, we discuss the experimental methods employed to determine the subcellular localization of lncRNAs. These techniques provide valuable insights into the precise cellular compartments where lncRNAs reside. Secondly, we explore the available computational resources and databases contributing to our understanding of lncRNAs, including information on their subcellular localization. These computational methods utilize algorithms and machine learning approaches to predict lncRNA subcellular locations using sequence and structural features. Lastly, we discuss the limitations of existing methodologies, future challenges, and potential applications of subcellular localization prediction for lncRNAs. We highlight the need for further advancements in computational methods and experimental validation to enhance the accuracy and reliability of subcellular localization predictions. To support the scientific community, we have developed a platform called LncInfo, which offers comprehensive information on lncRNAs, including their subcellular localization. This platform aims to consolidate and provide accessible resources to researchers studying lncRNAs and their functional roles (http://webs.iiitd.edu.in/raghava/lncinfo).
1 Introduction
Human genomes contain around 3 billion base pairs, whereas the Human Genome Project (HGP) shows that the human genome contains only 20,000 protein-coding genes. It means only a fraction of the human genome codes for proteins that indicate the role of the non-coding region of the genome (ENCODE Project Consortium, 2012). Among the non-coding genome transcripts, lncRNA has gained significant interest due to its involvement in biological and disease-related functions of specific genes. The ENCODE and FANTOM projects have provided insights into the mammalian transcriptome and indicate that the genome has a significant number of lncRNAs. One of the major challenges in the postgenomic era is annotating these lncRNAs’ function. In the past, a significant focus was on protein-coding transcripts. Identifying domains, family classification, subcellular localization, and biological function are the primary aspects of functional annotation of a biological entity.
Subcellular localization forms an integral part of functional annotation because, for lncRNA genes, the ultimate product is RNA. Therefore, lncRNA functions depend on proximity-based RNA physical interactions. Studying lncRNA subcellular localization and its dynamic changes is a crucial step toward elucidating the functions and mechanisms of newly discovered lncRNAs. The specific localization of lncRNAs within subcellular compartments allows them to exert precise regulatory effects on gene expression. Recent reviews have discussed how nuclear-localized lncRNAs, can interact with chromatin, participate in epigenetic modifications that influence transcriptional regulation and chromatin organization, and are crucial for organizing the three-dimensional architecture of the genome, contributing to genome organization, transcriptional regulation, and genome stability (Romero-Barrios et al., 2018; Pisignano and Ladomery, 2021; Statello et al., 2021).
According to the review by Dykes and Emanueli (2017), lncRNAs localized to the cytoplasm can be associated with ribonucleoprotein granules, such as P-bodies or stress granules, modulating mRNA stability and translation. In the review by Statello et al. (2021) they have discussed how specific lncRNAs localize to specialized subcellular domains, such as neuronal dendrites or axons, where they are involved in RNA transport and local translation, impacting synaptic plasticity and neuronal development. LncRNAs also contribute to signaling pathways by localizing to specific cellular compartments, such as the plasma membrane or cytoplasmic signaling bodies, interacting with signaling proteins, acting as scaffolds, or regulating protein localization. In summary, the precise localization of lncRNAs within cells is essential for their regulatory roles in gene expression, cellular trafficking, signaling, and nuclear organization, highlighting their diverse and significant biological importance. Recent studies have identified certain lncRNAs that may have been misannotated, as they possessed short open reading frames (sORFs) (Carlevaro-Fita et al., 2016; Hartford and Lal, 2020; Andjus et al., 2024). Some of these sORFs encode small proteins or micropeptides with a wide array of fundamental biological functions, including cell division, transcription regulation and cell signaling (Chen et al., 2020; Wei and Guo, 2020).
Several experimental techniques like fluorescent in-situ hybridization (FISH), MS2-system-based techniques, and high-throughput RNA sequencing are used for in vitro visualization of mRNA location to trace its subcellular localization (Fagerberg et al., 2014; Wang et al., 2021; Zhang et al., 2021). These methods remain the gold standard for studying the subcellular localization of lncRNAs. These experimental techniques have limitations, including cost, time, and the need for sophisticated instrumentation as discussed by Savulescu et al. (2021) in their perspective article. FISH-based methods suffer from artifacts when multiplexing is done. Also, the detection efficiency decreases as the number of RNA targets increases in FISH-based methods. Current methods find it hard to balance target number and detection efficiency, highlighting the significant limitations in generating subcellular localization information.
These issues highlight the need to develop computational methods for lncRNA subcellular localization prediction. An extensive repertoire of computational resources has already been developed to help predict the subcellular localization of lncRNA. However, the dataset remains the same, more or less sourced from RNALocate version 1. The number of lncRNA sequences in RNALocate (version 2) has decreased significantly, leaving researchers needing more localization information. Other existing databases harboring localization data must be updated frequently, making them outdated for computational model development.
Few review articles have recently been published discussing the advances in tools designed for predicting lncRNA subcellular localization. Among these, Wang et al. (2021) published a review on lncRNA subcellular localization prediction tools. They have covered four tools in terms of—dataset used, data preprocessing, feature extraction, and algorithm for prediction. Firstly, they provided an overview of all the tools and then described each attribute separately. However, the review by Asim et al. (2021) has a broader domain—covering RNA classification and RNA subcellular localization. Much of the review focuses on the RNA classification tool, as much work has been done in that field. A brief description of the tools designed to predict subcellular localization is provided, and they cover three types of RNA—mRNA, miRNA, and lncRNA. In this article, we will complement the information provided in existing reviews by including newer methods. Additionally, we have reviewed all the databases that harbor information on lncRNA subcellular localization.
To consolidate all the information, we collected while writing this review, we have developed a web resource called—“LncInfo,” developed using HTML5 and Apache.
2 Existing databases on lncRNA
Due to the rapid growth in lncRNA research, large-scale data is being generated, and this information is annotated correctly and stored in various databases that are designed to aid researchers. Most of the databases were developed post-2015 when there was a surge in high throughput methods for studying lncRNA. A list of the popular databases based on lncRNA is provided in Table 1. All the existing databases have been graphically presented in Figure 1.
2.1 General databases
The FANTOM5/CAT project is dedicated to exploring the regulatory landscape of human and mouse genomes by generating comprehensive transcriptomic data (Hon et al., 2017). Researchers may use this vast collection of transcriptome data from many biological contexts as a valuable tool to study the control of gene expression, transcriptional networks, and functional components of the mammalian genome. GENCODE is dedicated to maintaining protein-coding and non-coding genes (Frankish et al., 2019). Its main objective is to provide a thorough, current, and accurate annotation of these genomes by locating and describing the protein-coding genes, long non-coding RNAs (lncRNAs), and other functional non-coding RNAs. The annotation provides crucial details for the discovered genes and transcripts, such as gene loci, exon boundaries, coding sequences, untranslated regions (UTRs), and functional and structural annotations. There are now 62,703 genes reported by GENCODE, 19,393 of which are protein-coding and 19,928 long non-coding RNAs. In order to create these annotations, the research combines experimental and computational techniques, including high-throughput sequencing technologies, transcriptome data, protein-coding potential analysis, comparative genomics, and manual curation.
2.2 Expression databases
The ENCODE is a comprehensive repository of experimental data and metadata generated by the ENCODE project (Luo et al., 2020). The portal utilizes a standardized metadata architecture that facilitates understanding data in biological terms, enabling the representation of experiments and their analyses. The ENCODE database provides researchers access to a wide range of data, including genomic sequences, epigenetic marks, and transcription factor binding sites. LncExpDB is an expression database focused on human long non-coding RNA (lncRNA) genes (Li et al., 2021). Its main objective is to provide a wide-ranging collection of expression profiles for lncRNA genes, enabling the exploration of their expression characteristics, capacities, and potential functional significance. The database also aims to establish connections between lncRNAs and protein-coding genes across different biological contexts and conditions. LncBook, an extensive repository of lncRNAs) (Li et al., 2023), which is widely used in various studies. The updated version, LncBook 2.0, offers significant improvements and enhancements. This resource empowers users to unravel the functional significance of lncRNAs within different biological contexts.
The Genotype-Tissue Expression (GTEx) project is a valuable resource for studying human gene expression, regulation, and its association with genetic variation (Hon et al., 2017). This initiative involves collecting and analyzing gene expression data from tissues obtained from deceased human donors and their corresponding genotypic information. GTEx is an invaluable resource for researchers investigating the intricate relationship between genetic variation, gene expression, and the specific biology of different tissues. Furthermore, it establishes a valuable foundation for investigating the regulatory mechanisms governing gene expression. TANRIC is a database that maintains cancer-associated lncRNAs mainly extracted from TCGA. The Gene Expression Omnibus (GEO) is a globally accessible public repository that houses functional genomic data sets obtained through high-throughput microarray and next-generation sequencing technologies. These resources are indexed, crosslinked, and made searchable to facilitate easy access and exploration (Barrett et al., 2013).
2.3 Sequence annotation
LNCipedia is a database for collecting and annotating human long non-coding RNA (lncRNA) sequences (Volders et al., 2019). One of its main features is the consolidation of redundant transcripts from various sources, resulting in a consistent and reliable database with grouped transcripts. LNCipedia 5 has expanded its content by incorporating new lncRNAs from resources like FANTOM CAT. It also includes minor improvements, such as an enhanced filtering pipeline and support for official HGNC gene names. CANTATADb 2.0 is a database of LncRNAs that maintain lncRNA from plants and algae species (Szcześniak et al., 2019). Reads from hundreds of RNA-SEQ libraries were aligned with corresponding plant genomes. Gene prediction software was used to re-annotate plant genomes, and annotation data was used as a reference. NONCODE is a comprehensive database of non-coding RNAs, with a particular emphasis on lncRNAs (Zhao et al., 2021). The number of lncRNAs in NONCODEV6 has reached 644,510 including 173,112 human lncRNA transcript.
RNAcentral is a state-of-the-art database that consolidates the non-coding sequences of 44 RNA resources, including more than 18 million ncRNA sequences from various organisms (RNAcentral Consortium, 2021). The new version of RNAcentral also contains the secondary structure of 13 million sequences, making it the world’s most extensive 2D structure database. CHESS is an extensive collection of human genes derived from approximately 10,000 RNA sequencing experiments (Pertea et al., 2018). The database comprises a comprehensive set of genes, encompassing 19,838 protein-coding genes and 17,624 lncRNA) genes. RNA Atlas is an experimentally derived database that advances our understanding of human non-coding RNAs (ncRNAs). By analyzing a diverse set of 300 human samples, including 45 tissues, 162 cell types, and 93 cell lines, researchers have expanded the existing catalog of ncRNAs, identifying a total of 44,428 long non-coding RNAs (lncRNAs). This comprehensive dataset, accessible through the R2 webtool, provides a foundation for further exploration of RNA biology and function (Lorenzi et al., 2021).
2.4 Subcellular localization
LncATLAS is a subcellular localization database of lncRNA, where information has been obtained from RNA-sequencing data of human cell-lines (Mas-Ponte et al., 2017). The RNA-seq datasets were obtained from the ENCODE database. In order to quantify subcellular localization, a measure called relative concentration index (RCI) was introduced. LncSLdb is a specialized database designed to collate and manage qualitative and quantitative information on the subcellular localization of lncRNAs (Wen et al., 2018). The latest release of LncSLdb encompasses data on more than 11,000 lncRNA transcripts derived from three species (human, mouse, and fruit fly). RNALocate v2.0 is a comprehensive repository of information on RNA subcellular localization. It is compiled from scientific literature, public databases, and RNA sequencing datasets (Cui et al., 2022). It contains around 200 thousand entries, and each entry provides detailed information about RNA, including their subcellular localization. Additionally, it provides three prediction tools to cater to different user requirements.
2.5 Disease association
The LncRNADisease2 database is dedicated to maintaining ncRNA-associated diseases, including cancer, cardiovascular diseases, and neurological disorders (Bao et al., 2019). It contains a collection of experimentally validated lncRNAs linked to specific diseases, detailed annotations on their molecular functions, subcellular localizations, interacting proteins, and experimental evidence. The LncRNADisease database documents 205,959 lncRNA-disease associations and 2,297 lncRNA causative associations. The Lnc2Cancer v3.0 database is a comprehensive resource focusing on experimentally validated associations between lncRNAs and cancers (Gao et al., 2021). The updated version of Lnc2Cancer includes new features, such as an increased number of cancer-associated lncRNA entries. The database also offers details on microRNAs, transcription factors, genetic variations, methylation, enhancers, and other regulatory mechanisms.
3 Experimental techniques to investigate subcellular localization
One of the primary aspects of the functional annotation of lncRNA is their subcellular localization. The function of lncRNA heavily depends on its location in cells as it interacts with biological molecules in the exact location of the cell (Savulescu et al., 2021). In order to investigate subcellular localization, many experimental techniques have been developed. Figure 2 provides an overview of all the experimental techniques developed in this regard. Deep-sequencing studies have unveiled numerous long non-coding RNAs (lncRNAs) expressed in mammalian genomes, but most remain poorly understood. Essential aspects such as subcellular localization and absolute abundance in single cells remain largely unknown. Knowledge of lncRNA localization can provide insights into their biological functions, while their abundance is crucial for understanding molecular mechanisms. RNA fluorescence in situ hybridization (RNA FISH) has been instrumental in uncovering the localization of specific lncRNAs, but conventional methods lack the sensitivity to detect low-abundance lncRNAs. One of the pioneer subcellular localization experiments where X-inactivation by lncRNA XIST was first studied (Brown et al., 1991; Brockdorff et al., 1992), utilized a primitive RNA-FISH method to understand the mechanism of action (Brown et al., 1992) fully. Previously, it was known that XIST could inactivate one X chromosome in females. However, RNA-FISH explained the same—indicating how XIST stays in the nucleus and coats the inactive X-chromosome (Brown et al., 1991).
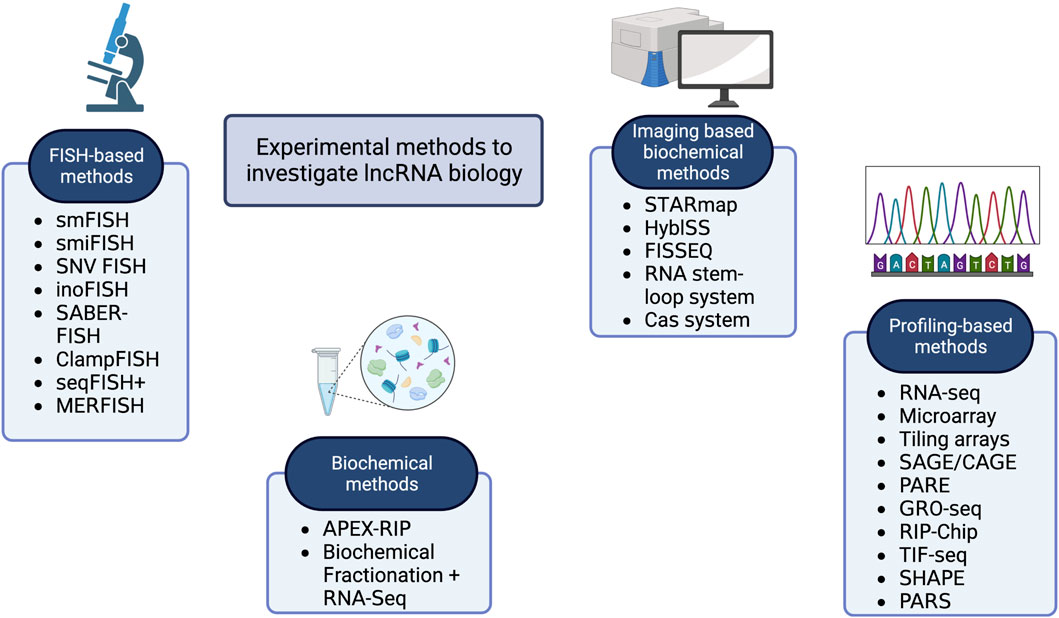
Figure 2. A graphical representation of the major classes of experimental methods used in lncRNA research.
Further improvement in resolution was achieved when Cabili et al. (2015) deployed single-molecule RNA-FISH to systematically quantify and categorize the subcellular localization patterns of a representative set of 61 lncRNAs in three different cell types. However, there needs to be a more systematic exploration of lncRNA localization and abundance using these techniques, especially for intergenic lncRNAs (lincRNAs). They observed a wide range of localization patterns, with lncRNAs predominantly localizing to the nucleus. They also found that the low abundance of lncRNAs is not due to a small subset of highly expressing cells and that lncRNAs exhibit similar cell-to-cell heterogeneity as mRNAs.
In this section, we have covered most of the popular experimental techniques. A summary of the existing experimental methods is provided in Table 2.
3.1 FISH-based methods
Fluorescence In Situ Hybridization (FISH) is a molecular cytogenetic technique commonly used to identify the location of biological molecules like DNA and entire chromosomes in a cell. One of the significant features of FISH is that it allows the detection of many biological molecules simultaneously. Single-molecule FISH (smFISH) is a modified version of FISH for single-cell resolution. It allows for visualizing the localization and abundance of individual RNA molecules within cells or tissues (Femino et al., 1998; Raj and Tyagi, 2010; Lubeck and Cai, 2012). In smFISH, RNA molecules are hybridized with fluorescently labeled probes to generate a fluorescent signal that can be detected using fluorescence microscopy. Single Molecule Inexpensive FISH (smiFISH) is a user-friendly and versatile approach for visualizing and quantifying RNA. It uses fluorescently tagged secondary detector oligonucleotides and unlabeled primary probes (Tsanov et al., 2016). The primary probes, which are gene-specific, are unlabeled, making them cost-effective to synthesize. This cost advantage allows for using more probes per mRNA, significantly improving detection efficiency. Single-nucleotide variant FISH (SNV-FISH) is a highly sophisticated method that detects single-nucleotide variations in an RNA transcript (Levesque et al., 2013; Symmons et al., 2019). One more variety of FISH is inosineFISH or inoFISH, which capture image of adenosine-to-inosine RNA altering occasions with single-particle resolution (Mellis et al., 2017).
SABER, an acronym for signal amplification by exchange reaction, is a technique that enhances the signals obtained from oligonucleotide-based FISH probes by attaching long, single-stranded DNA chains (Kishi et al., 2019). It can amplify RNA/DNA signals in cells and tissues. Click-amplifying FISH is a precise method that achieves remarkable signal amplification with a high gain (Rouhanifard et al., 2018). It allows the detection of RNA species using low-magnification microscopy and RNA-based flow cytometry. Sequential FISH (seqFISH) is an advanced technology that identifies thousands of molecules within single cells while preserving their spatial context (Eng et al., 2019). SeqFISH allows researchers to obtain super-resolved images that provide insights into genomic-level processes with exceptionally high efficiency and accuracy. Multiplexed error-robust FISH (MERFISH) is a powerful single-molecule imaging technique developed for determining copy numbers of thousands of RNA molecules (Chen et al., 2015; Xia et al., 2019). It revolutionizes transcriptome-scale RNA imaging at the single-cell level by utilizing error-robust barcodes to encode individual RNA species. Notably, the MERFISH protocol has undergone updates to enhance detection efficiency, allowing the transcriptome-scale imaging of approximately 1,000 RNA species in single cells.
3.2 Imaging based biochemical methods
STARmap is a novel approach that allow to measure gene expression at single cell compartment in intact tissue, providing insights into the activity of over a thousand genes (Wang et al., 2018). In the future, integrating this intact-system gene expression measurement with complementary methodologies will offer cellular-resolution analysis. One of the powerful technologies in this category is in situ sequencing (ISS), which utilizes padlock probes and rolling circle amplification. An enhanced version of ISS, known as HybISS (hybridization-based in situ sequencing), has been developed to improve the spatial detection of RNA transcripts (Gyllborg et al., 2020). HybISS incorporates modifications in probe design, enabling a new barcoding system through sequence-by-hybridization chemistry. The HybISS design offers increased flexibility, allowing for enhanced multiplexing and improved signal-to-noise ratio, all while maintaining the efficiency required for imaging large fields of view. Fluorescent In Situ Sequencing (FISSEQ) is a molecular technique that allows the simultaneous visualization of RNA molecules and their sequences in intact biological samples (Lee et al., 2015). FISSEQ uses barcoded oligonucleotide probes to hybridize targeted RNA molecules. These probes are amplified and labeled with fluorescent dyes, allowing the visualization of the RNA molecules. In the widely adopted RNA labeling method, query RNA molecule is labeled with a specific stem-loop. This stem-loop sequence binds to the bacteriophage coat protein and fused to a fluorescent protein that enables the visualization of the labeled RNA using fluorescence microscopy (Heinrich et al., 2017). Using CRISPR-Cas based RNA imaging, which offers target programmability without genetically altering the target, it is possible to track a variety of endogenous long-chain RNAs (like mRNA) in live cells in real-time (Abudayyeh et al., 2017; Chen et al., 2020). The CRISPR-mediated RNA imaging technique involves the use of a modified CRISPR-Cas system that targets specific RNA molecules and recruit fluorescent proteins.
3.3 RNA-seq based techniques
In the last decade, RNA sequencing (RNA-seq) has been heavily used for a wide range of omics, including a comprehensive analysis of gene expression and sequencing of the transcriptome. It now encompasses various methods that enable the investigation of diverse aspects of RNA biology. Furthermore, the advent of long-read sequencing and direct RNA-seq technologies, coupled with improved computational tools for data analysis, has revolutionized RNA-seq (Wang et al., 2009). In contrast to classical sequencing technologies, RNA-Seq offers superior coverage and enhanced resolution. Microarray RNA profiling is an older technique that allows the simultaneous detection and quantification of thousands of RNA transcripts in a single experiment (Schena et al., 1995). This procedure has limitations, including its reliance on pre-designed probes, which may not detect all RNA transcripts. A tiling array consists of short nucleic acid fragments fixed onto a substrate (Bertone et al., 2004). These arrays are specifically designed to cover the entire genome of the target species. Tiling arrays are widely utilized in various applications, such as ChIP assays, to determine genome binding or to identify transcribed regions. By employing tiling arrays, researchers can obtain valuable insights into the binding patterns of the genome in ChIP assays.
Serial analysis of gene expression (SAGE) is a well-established technique developed to study the transcriptome by identifying and quantifying transcripts, including non-coding RNAs (ncRNAs) (Velculescu et al., 1995). This method relies on restriction enzymes to generate short, unbiased cDNA sequences known as SAGE tags. It involves several steps, including isolating and converting mRNA into cDNA, cutting the cDNA into smaller fragments, and adding tags to each fragment. The fragments are then combined and sequenced, providing information about which genes are present and how many copies of each gene are being expressed. The limited length of the sequence tags in SAGE may need to provide more information to accurately determine the identity of a new sequence. To address this limitation, a modified version of SAGE that utilizes more extended tags has been developed, demonstrating increased usefulness in transcript identification. Cap analysis gene expression (CAGE) provides information about transcript abundance and promoter identification (Shiraki et al., 2003). It is a high throughput method that can identify and quantify 5′ ends of capped RNAs. It has been used for study of RNA subcellular localization (Djebali et al., 2012) and identification of transcription start sites (ENCODE Project Consortium, 2012). The PARE is another technology used to identify and quantify small RNA molecules in a sample (German et al., 2009). PARE works by capturing the 5′ ends of RNA fragments using a technique called ligation-mediated PCR. It is often used to study the targets and functions of miRNAs.
GRO-seq was developed to study the transcriptional activity of the genome at a global scale. It works by labeling nascent RNA transcripts using BrUTP (bromouridine triphosphate) and isolating and sequencing the labeled RNA molecules (Gardini, 2017). This method allows researchers to identify the specific genomic regions where transcription occurs and the direction and rate of transcription. GRO-seq is often used to study gene expression regulation and identify novel non-coding RNAs.
RIP-Chip is used for isolating and sequencing RNA transcripts that bind to RNA-binding proteins (Keene et al., 2006). Various downstream methods, such as high-throughput sequencing, characterize the RNA molecules associated with the RNA binding proteins. RIP is often used to study post-transcriptional regulation of gene expression, particularly the regulation of mRNA stability and translation. Transcript IsoForm Sequencing (TIF-Seq) is a powerful technology that has been used to study transcript isoforms at the genome scale (Pelechano et al., 2013). In TIF-Seq, both the 5′ and 3′ ends are simultaneously sequenced and it allows the user to accurately determine the stand and end sites of individual RNA molecules. Selective 2′-hydroxyl acylation analyzed by primer extension (SHAPE) was developed to study the structure and folding of RNA molecules at single nucleotide resolution (Smola et al., 2015). SHAPE experiments use the reactivity of the RNA ribose 2′-OH towards hydroxyl-selective electrophilic reagents to model the secondary and tertiary structure of RNA molecules. PARS is another method for studying RNA structure and folding (Wan et al., 2013).
3.4 Biochemical methods
APEX-RIP allows the identification of RNA-protein interactions by combining two techniques: APEX (Ascorbate Peroxidase-mediated Protein Localization and Protein-Protein Interaction Profiling) and RIP (RNA Immunoprecipitation) (Kaewsapsak et al., 2017). APEX-RIP has been used to identify RNA-binding proteins and their associated RNAs in a variety of systems, including cancer cells, neuronal cells, and embryonic stem cells. Biochemical fractionation is a technique used to isolate specific cellular components or organelles from complex mixtures such as whole cells or tissues. The distribution and expression of RNA molecules in different subcellular fractions is studied by combining biochemical fractionation and RNA-seq (Tilgner et al., 2012). By fractionating cells or tissues into different organelles or subcellular compartments, researchers can isolate RNAs that are localized or enriched in specific cell regions. This approach has been used to study various aspects of RNA biology, including RNA localization.
4 Creation of datasets by different tools
Majority of the datasets used by the tools are derived from RNALocate or ENCODE RNA-seq data. An overview of datasets published by existing methods has been provided in Table 3.
4.1 RNALocate based methods
RNAlocate is a comprehensive resource that provides information on the subcellular localization of different types of RNA molecules (Zhang et al., 2017). The current version of RNALocate contains a vast collection of over 37,700 manually curated entries, each supported by experimental evidence of RNA-associated subcellular localization. The database encompasses more than 21,800 coding and non-coding RNAs across 65 species. It covers a wide range of 42 subcellular locations, focusing on Homo sapiens and Mus musculus. RNALocate is a valuable repository for researchers seeking knowledge about RNA localization and its functional implications. The wealth of data available in RNALocate has been utilized in the development of several popular datasets. Notably, it has contributed to creating datasets used in lncLocator (Cao et al., 2018) and iLoc-lncRNA (Su et al., 2018). These resources leverage the comprehensive information provided by RNALocate to enhance the study of subcellular localization patterns in long non-coding RNAs (lncRNAs) and other RNA molecules.
The dataset for lncLocator was extracted from RNALocate version 1 (Zhang et al., 2017). A total of 1,361 lncRNA entries with curated subcellular localization were retrieved from the RNAlocate database, and multiple entries for the same lncRNA were combined. Only those lncRNA were retained, with their sequence information in NCBI (Sayers et al., 2022) and Ensembl (Cunningham et al., 2022). Seven subcellular locations were used for classification, and there were 19 combinations of these locations in the dataset. Redundancy was removed using CD-HIT, which was used to remove sequences with more than 80% similarity. Multi-locational lncRNAs were removed, and lncRNAs associated with only one location were allowed. Two locations (Endoplasmic reticulum and synapse) were removed as they had very few samples. Finally, a dataset of 612 lncRNA was created, covering five subcellular locations.
The dataset used in iLoc-lncRNA (Su et al., 2018) was created from RNALocate version 1 (Zhang et al., 2017). 923 lncRNA sequences with annotated subcellular localizations were obtained, and CD-HIT reduced redundancy at 80% similarity. After removing similar sequences, 655 non-redundant lncRNA sequences were obtained. The dataset comprises 156 lncRNAs from the nucleus, 426 lncRNAs from the cytoplasm, 43 lncRNAs from the ribosome, and S4 contains 30 lncRNAs from the exosome. This dataset was also used in lncLocation, Locate-R, and lncLocPred.
The training dataset used in KD-KLNMF (Zhang and Qiao, 2020) is obtained from Yang et al. (2020), where they have used 923 sequences for the training dataset. CD-HIT was used at a similarity index threshold of 80% to remove redundant sequences from the dataset. The final training dataset contains 644 lncRNA sequences - 154 sequences from the nucleus, 417 sequences from the cytoplasm, 43 from the ribosome, and 30 from the exosome. In order to balance the dataset, SMOTE (Synthetic Minority Oversampling Technique) was applied to the dataset multiple times post-feature extraction, such that the number of samples in the minority classes equaled that of the majority class. In DeepLncLoc, LncRNAs located in only one location were selected for model construction, the dataset was created from RNALocate v1 (Zeng et al., 2022). GM-lncLoc (Cai et al., 2023) obtained datasets from iLoc-lncRNA and lncLocator, which were originally extracted from RNALocate. Both datasets were subjected to oversampling using SMOTE, and all the classes were equally represented. Dataset1 had 292 lncRNA samples, while dataset2 had 417 samples. Also, an independent dataset was created using the test dataset from DeepLncLoc. GraphLncLoc (Li et al., 2023) uses the benchmark dataset of DeepLncLoc for training their graph convolution network-based model.
4.2 RNA-seq derived datasets
Apart from RNALocate, some methods have used RNA-seq data to generate localization data by quantifying the gene expression in subcellular locations.
DeepLncRNA (ENCODE Project Consortium, 2012) used 93 RNA-seq samples from 14 human immortalized cell lines from the ENCODE database (ENCODE Project Consortium, 2012), of which 45 were from the cytosol, and 48 were from the nucleus. Differential transcript expression was used to quantify the differences in lncRNA transcript abundances between nuclear and cytosolic cellular fractions for each cell type. Log2 fold change was used to determine enrichment in the nucleus or cytosol. A sequence was assigned to the cytosol if the log2 fold change <0, and it will be assigned to the nucleus if the log2 fold change >2.8. Applying this log2 fold change threshold resulted in a dataset containing 4,380 cytosolic lncRNAs and 4,298 nuclear lncRNAs.
LncLocator 2.0 (Lin et al., 2021) sourced data from two databases—nucleotide sequences from the GENCODE (Frankish et al., 2019) project and localization information from lncATLAS (Mas-Ponte et al., 2017), combined by using common gene IDs. lncATLAS uses relative concentration index (RCI) for quantifying subcellular localization. RCI is defined as the log ratio between concentrations measured by Fragments Per Kilobase Million (FPKM) in two samples (Cytoplasm/Nucleus). Different numbers of lncRNA samples are available based on the type of cell line as they have filtered out the lncRNA with Cytoplasm/Nucleus RCI in the range [−1, 1]. The dataset used in lncLocator 2.0 was also used to develop the model in TACOS (Jeon et al., 2022), which is also a cell-line-specific predictor. However, TACOS utilizes only ten cell lines to develop their classifier.
5 Methods and their performance
Over the past few years, there have been several advancements in predicting the subcellular localization of lncRNA sequences. Typically, these advancements involve utilizing machine learning techniques to create prediction models, with Support Vector Machine (SVM) being the most frequently employed algorithm. However, recent progress has shifted towards deep learning-based methods, which eliminate the need for manual feature selection and improve performance on benchmark datasets. For a comprehensive overview of the current tools available for subcellular localization prediction, please see Table 4. Additionally, Table 5 provides information regarding the efficacy of these tools in terms of predictive accuracy and other evaluation metrics.
LncLocator (Cao et al., 2018) used an unsupervised stacked autoencoder (AE) engine that deployed on k-mer features to learn efficient data representations. Both the raw and autoencoded features were used for training the models. lncLocator achieves accuracy, F1-score, and recall of 0.591, 0.367, and 0.363, respectively, on the oversampled dataset, whereas the accuracy, F1-score, and recall of the method on the original dataset were 0.598, 0.343 and 0.356, respectively. iLoc-lncRNA (Su et al., 2018) is an SVM-ensemble method that utilizes a combination of Pseudo K-tuple Nucleotide Composition and 8-mer composition. Furthermore, feature selection was performed using Iterative Feature Selection (IFS). lncLocation (Feng et al., 2020) utilizes a combination of multiple features for training its model. These features encompass different aspects such as sequence composition, basic lncRNA characteristics, physical-chemical properties, and multi-scale secondary structural features. Additionally included are multi-scale secondary structural characteristics and physicochemical properties. By considering multiple feature combinations and utilizing SVM, lncLocation achieves its predictive capabilities for the given task.
Locate-R (Ahmad et al., 2020) was developed using k-mer and n-gapped k-mer composition as features. The value of k was varied from 1-6 while generating k-mers and, in the process, generating 5,460 features. N-gapped k-mer composition was also used, where the number of gaps n, was varied from 1-10, and the value of k was varied from 1 to 3. 39,312 new features were generated using n-gapped k-mer composition. A non-linear version of SVM, called locally deep support vector machine (LD-SVM), was used for developing the model. The model achieved an overall accuracy of 90.69%. lncLocPred (Fan et al., 2020) is based on a logistic regression model and three different types of features. The selected k-mer features were merged with two other types of features—Pseudo-Dinucleotide Composition (PseDNC) and Local Structure-Sequence Triplet Element (Triplet). While Triplet makes use of the structural information of RNA sequences, PseDNC uses the sequential information and physicochemical characteristics of the nucleotide sequence. F-score-based feature selection was applied to this set of features, and an optimal set of features was obtained. lncLocPred manages to achieve an overall accuracy of 92.37%, which is an improvement of 2% over other state-of-the-art tools.
KD-KLNMF (Zhang and Qiao, 2020) employed k-mer composition and dinucleotide-based spatial autocorrelation for generating features. Kullback-Leibler divergence-based nonnegative matrix factorization (KLNMF) was employed as a feature selection method to enhance the features’ effectiveness. The Support Vector Machine (SVM) with a Radial Basis Function (RBF) kernel demonstrated the best performance among various classifiers. The model achieved an impressive overall accuracy of 97.24%, as determined by the jackknife test. DeepLncLoc (Zeng et al., 2022) utilizes deep learning algorithms and natural language processing techniques to develop a classifier for subcellular localization. The generation of features in DeepLncLoc involves subsequence embedding. Following subsequence embedding, an average pooling layer is used to capture the patterns of each subsequence, resulting in a matrix representation of the lncRNA sequence. Lastly, a textCNN is employed to learn high-level features and perform the prediction task. GM-lncLoc (Cai et al., 2023) used a Graph Convolution Network along with Model-agnostic meta-learning to develop the classification model.
Methods developed using RNA-seq data are primarily binary classifiers. They are designed to compare the expression of lncRNA between two locations. DeepLncRNA (Gudenas and Wang, 2018) uses a neural network to classify lncRNA samples into cytoplasm/nucleus based on their location labels. Three different types of features were combined to generate a feature set for the dataset. Genome loci and the presence of RNA-binding protein motifs were also used as features. lncLocator 2.0 (Lin et al., 2021) is a regression-based tool that predicts the Cytosol/Nucleus Relative Concentration Index (CNCRI). lncLocator 2.0 uses GloVe for sequence embedding and generating word vectors for downstream training. CNN is used for learning features from the word vectors. CNN and Bi-LSTM structure take the word vectors as the input and obtain a fixed-length feature vector. This fixed-length vector is provided to an MLP classifier, which uses the continuous CNRCI values and acts as a regressor model.
6 Challenges and future perspectives
The remarkable progress in experimental techniques has significantly contributed to the generation of large-scale data on lncRNAs. Fluorescence in situ hybridization (FISH), initially developed for visualizing single nucleotide targets, has evolved over time to enable the visualization of thousands of nucleotide targets at single-cell resolution. However, despite the advancements, the data produced by these methods are often not readily available in the form of public databases. One primary disadvantage of FISH-based methods is that they do not allow de novo identification of transcripts due to the requirement of probes. Also, when multiplexing is performed, overcrowding of signals can cause issues in the detection of transcripts. It has been observed that the detection efficiency drops with increasing RNA targets. Amplification of signals by techniques like hybridization chain reaction can create artifacts and increase the number of false positives. Identification and decoding barcodes, in the case of multiplexed RNA-FISH techniques, require complex software and heavy computational power.
Profiling methods have also made considerable strides, transitioning from microarrays to bulk RNA-seq and eventually to single-cell sequencing. The improved annotation of lncRNAs by resources like GENCODE has facilitated the quantification of lncRNA expression. However, single-cell expression data poses its own set of challenges, primarily related to low read counts of lncRNA transcripts. Unlike protein-coding genes, lncRNAs often lack well-defined functional domains, making their functional characterization more challenging using sequencing data alone.
Despite these challenges, the continuous advancement of experimental techniques and annotation resources has significantly enhanced our understanding of lncRNAs. Future efforts should focus on addressing the limitations associated with data availability, cost, and functional characterization. By promoting data-sharing practices, ensuring comprehensive annotations, and integrating complementary experimental approaches, we can overcome these obstacles and gain deeper insights into the functional roles and mechanisms of lncRNAs.
The field of lncRNA research has witnessed the development of numerous databases aimed at facilitating sequence and functional annotation. These comprehensive databases encompass various aspects related to lncRNAs, including sequence annotation, expression data, functional annotation, disease associations, and subcellular localization. Such resources have become indispensable in managing the vast volumes of data generated by high-throughput methods. However, certain limitations hinder the full utility of these databases. One prevalent issue is the outdated nature of some databases. Several well-known databases, such as LNCpedia and LncRNADisease2, have not been updated since 2018, rendering them inadequate for covering the newly generated lncRNA sequences. This lack of timely updates limits their relevance and usefulness within the rapidly evolving field. Another significant problem lies in the lack of uniformity in the naming nomenclature of lncRNA sequences across different annotation databases. Each database tends to assign its own set of names to the lncRNA sequences, resulting in confusion and defeating the purpose of accurate annotation. This inconsistency hampers effective data integration and comparability across databases. Furthermore, when it comes to subcellular localization, lncATLAS, a database specifically focused on this aspect, falls short. Last updated in 2017, lncATLAS only covers two subcellular locations, namely, the nucleus and cytoplasm. This limited coverage restricts the usability of the data, as other existing databases include annotations for major organelles beyond the nucleus and cytoplasm. Outdated databases fail to keep pace with the rapid expansion of lncRNA knowledge, naming inconsistencies hinder integration, and the limited coverage of subcellular localization databases restricts their applicability. Overcoming these limitations is essential for enhancing the effectiveness and utility of lncRNA annotation databases in advancing our understanding of these important regulatory molecules.
A primary challenge in the area of subcellular localization is the scarcity of sufficient training and validation data for developing prediction methods. The training datasets used to develop these prediction tools are often limited in size and diversity, which can impact the accuracy and robustness of the predictions. With the exception of RNALocate, there is a lack of well-annotated databases that provide comprehensive subcellular localization information. Many existing prediction tools rely on data from RNALocate version 1, even though an updated version 2 was released in 2021. Additionally, cell-line-specific subcellular localization information available in ENCODE is underutilized despite its potential relevance. RNA-seq data, which is commonly used for localization prediction, can be noisy and prone to inaccuracies due to variations in expression values caused by differing cell states and analysis workflows. Moreover, it has been observed that the localization of lncRNAs can vary between fixed cells and live cells, adding another layer of complexity to the prediction process. Currently, the MERFISH technique provides a promising solution by enabling the identification of RNAs associated with subcellular compartments with high sensitivity and low false discovery rates at a genome-wide scale. The availability of MERFISH datasets would greatly enhance researchers’ ability to quantify subcellular localization more accurately. Addressing these limitations will require concerted efforts to expand and diversify training datasets. Recent advances in spatial transcriptomics, enables the profiling of transcripts at subcellular resolution, providing insights into the spatial localization of RNAs within cells. This technique has become increasingly important in understanding the complex regulation of gene expression within cells, particularly in the context of disease formation and cellular processes. The availability of image data generated from spatial transcriptomics techniques offers a rich resource for investigating the spatial expression patterns of genes in different cell types and in response to environmental stimuli. These datasets can be used to identify the spatial distribution of RNAs within cells, which is crucial for understanding cellular processes such as RNA localization and its role in disease.
Despite the advancements in lncRNA subcellular localization prediction, the current methods still face limitations in terms of their accuracy, and further research is necessary to improve their performance. While these tools may demonstrate high accuracy when evaluated on their own training datasets, their performance on independent datasets remains notably poor. For instance, lncLocPred, Locate-R, and iLoc-lncRNA exhibited high accuracy rates of 92.37%, 90.69%, and 86.72%, respectively, on their training datasets. However, when these methods were tested on an independent dataset, their accuracies dropped significantly to 44.44%, 38.64%, and 35.86%, respectively. This decline in performance suggests the presence of bias in the machine-learning algorithms used by these methods. A similar trend was observed when GM-LncLoc compared its own method with iLoc-lncRNA, Locate-R, and lncLocPred based on an independent dataset. While these models achieved high accuracies of 94.20%, 86.72%, 90.69%, and 92.39% on their training datasets, their accuracy drastically decreased to 46.21%, 35.86%, 38.64%, and 44.44%, respectively, on the independent dataset. These findings underscore the need for further investigation into the underlying biases and limitations of current machine-learning algorithms employed in lncRNA subcellular localization prediction.
7 Conclusion
Despite all the challenges, functional annotation has made significant progress over the last few years. The generation of data using high throughput techniques should be prioritized, and the data should be made publicly accessible using well-annotated databases. The availability of large volumes of data will lead to the development of better subcellular localization prediction tools. Improved algorithms, training datasets, and rigorous validation of independent datasets are essential for the development of more accurate and robust lncRNA subcellular localization prediction methods.
Data availability statement
Publicly available datasets were analyzed in this study. This data can be found here: https://webs.iiitd.edu.in/raghava/lncinfo/index.php.
Author contributions
SC: Conceptualization, Data curation, Formal Analysis, Investigation, Methodology, Validation, Visualization, Writing–original draft, Writing–review and editing. AR: Data curation, Investigation, Methodology, Writing–original draft, Writing–review and editing. GR: Conceptualization, Formal Analysis, Funding acquisition, Investigation, Project administration, Resources, Supervision, Writing–original draft, Writing–review and editing.
Funding
The authors declare that financial support was received for the research, authorship, and/or publication of this article. This work was supported by the BIC grant from Department of Biotechnology, India (BT/PR40158/BTIS/137/24/2021).
Acknowledgments
Authors also are thankful to the Department of Science and Technology (DST-INSPIRE) and University Grants Commission (UGC) for fellowships and the financial support. Authors are also thankful to Department of Computational Biology, IIITD New Delhi for infrastructure and facilities. We thank the Department of Biotechnology (DBT) for providing an infrastructure grant to the institute (Grant BT/PR40158/BTIS/137/24/2021).
Conflict of interest
The authors declare that the research was conducted in the absence of any commercial or financial relationships that could be construed as a potential conflict of interest.
The authors declared that they were an editorial board member of Frontiers, at the time of submission. This had no impact on the peer review process and the final decision.
Publisher’s note
All claims expressed in this article are solely those of the authors and do not necessarily represent those of their affiliated organizations, or those of the publisher, the editors and the reviewers. Any product that may be evaluated in this article, or claim that may be made by its manufacturer, is not guaranteed or endorsed by the publisher.
References
Abudayyeh, O. O., Gootenberg, J. S., Essletzbichler, P., Han, S., Joung, J., Belanto, J. J., et al. (2017). RNA targeting with CRISPR-Cas13. Nature 550, 280–284. doi:10.1038/nature24049
Ahmad, A., Lin, H., and Shatabda, S. (2020). Locate-R: subcellular localization of long non-coding RNAs using nucleotide compositions. Genomics 112, 2583–2589. doi:10.1016/j.ygeno.2020.02.011
Andjus, S., Szachnowski, U., Vogt, N., Gioftsidi, S., Hatin, I., Cornu, D., et al. (2024). Pervasive translation of Xrn1-sensitive unstable long non-coding RNAs in yeast. RNA 30, 662–679. doi:10.1261/rna.079903.123
Asim, M. N., Ibrahim, M. A., Imran Malik, M., Dengel, A., and Ahmed, S. (2021). Advances in computational methodologies for classification and sub-cellular locality prediction of non-coding RNAs. Int. J. Mol. Sci. 22, 8719. doi:10.3390/ijms22168719
Bao, Z., Yang, Z., Huang, Z., Zhou, Y., Cui, Q., and Dong, D. (2019). LncRNADisease 2.0: an updated database of long non-coding RNA-associated diseases. Nucleic Acids Res. 47, D1034–D1037. doi:10.1093/nar/gky905
Barrett, T., Wilhite, S. E., Ledoux, P., Evangelista, C., Kim, I. F., Tomashevsky, M., et al. (2012). NCBI GEO: archive for functional genomics data sets--update. Nucleic Acids Res. 41, D991–D995. doi:10.1093/nar/gks1193
Bertone, P., Stolc, V., Royce, T. E., Rozowsky, J. S., Urban, A. E., Zhu, X., et al. (2004). Global identification of human transcribed sequences with genome tiling arrays. Science 306, 2242–2246. doi:10.1126/science.1103388
Brockdorff, N., Ashworth, A., Kay, G. F., McCabe, V. M., Norris, D. P., Cooper, P. J., et al. (1992). The product of the mouse Xist gene is a 15 kb inactive X-specific transcript containing no conserved ORF and located in the nucleus. Cell 71, 515–526. doi:10.1016/0092-8674(92)90519-i
Brown, C. J., Hendrich, B. D., Rupert, J. L., Lafrenière, R. G., Xing, Y., Lawrence, J., et al. (1992). The human XIST gene: analysis of a 17 kb inactive X-specific RNA that contains conserved repeats and is highly localized within the nucleus. Cell 71, 527–542. doi:10.1016/0092-8674(92)90520-m
Brown, C. J., Lafreniere, R. G., Powers, V. E., Sebastio, G., Ballabio, A., Pettigrew, A. L., et al. (1991). Localization of the X inactivation centre on the human X chromosome in Xq13. Nature 349, 82–84. doi:10.1038/349082a0
Cabili, M. N., Dunagin, M. C., McClanahan, P. D., Biaesch, A., Padovan-Merhar, O., Regev, A., et al. (2015). Localization and abundance analysis of human lncRNAs at single-cell and single-molecule resolution. Genome Biol. 16, 20. doi:10.1186/s13059-015-0586-4
Cai, J., Wang, T., Deng, X., Tang, L., and Liu, L. (2023). GM-lncLoc: LncRNAs subcellular localization prediction based on graph neural network with meta-learning. BMC Genomics 24, 52. doi:10.1186/s12864-022-09034-1
Cao, Z., Pan, X., Yang, Y., Huang, Y., and Shen, H.-B. (2018). The lncLocator: a subcellular localization predictor for long non-coding RNAs based on a stacked ensemble classifier. Bioinformatics 34, 2185–2194. doi:10.1093/bioinformatics/bty085
Carlevaro-Fita, J., Rahim, A., Guigó, R., Vardy, L. A., and Johnson, R. (2016). Cytoplasmic long noncoding RNAs are frequently bound to and degraded at ribosomes in human cells. RNA 22, 867–882. doi:10.1261/rna.053561.115
Chen, J., Brunner, A.-D., Cogan, J. Z., Nuñez, J. K., Fields, A. P., Adamson, B., et al. (2020a). Pervasive functional translation of noncanonical human open reading frames. Science 367, 1140–1146. doi:10.1126/science.aay0262
Chen, K. H., Boettiger, A. N., Moffitt, J. R., Wang, S., and Zhuang, X. (2015). RNA imaging. Spatially resolved, highly multiplexed RNA profiling in single cells. Science 348, aaa6090. doi:10.1126/science.aaa6090
Chen, S., Wang, R., Lei, C., and Nie, Z. (2020b). CRISPR-Cas system for RNA detection and imaging. Chem. Res. Chin. Univ. 36, 157–163. doi:10.1007/s40242-019-0030-5
Cui, T., Dou, Y., Tan, P., Ni, Z., Liu, T., Wang, D., et al. (2022). RNALocate v2.0: an updated resource for RNA subcellular localization with increased coverage and annotation. Nucleic Acids Res. 50, D333–D339. doi:10.1093/nar/gkab825
Cunningham, F., Allen, J. E., Allen, J., Alvarez-Jarreta, J., Amode, M. R., Armean, I. M., et al. (2022). Ensembl 2022. Nucleic Acids Res. 50, D988–D995. doi:10.1093/nar/gkab1049
Djebali, S., Davis, C. A., Merkel, A., Dobin, A., Lassmann, T., Mortazavi, A., et al. (2012). Landscape of transcription in human cells. Nature 489, 101–108. doi:10.1038/nature11233
Dykes, I. M., and Emanueli, C. (2017). Transcriptional and post-transcriptional gene regulation by long non-coding RNA. Genomics Proteomics Bioinforma. 15, 177–186. doi:10.1016/j.gpb.2016.12.005
Eng, C.-H. L., Lawson, M., Zhu, Q., Dries, R., Koulena, N., Takei, Y., et al. (2019). Transcriptome-scale super-resolved imaging in tissues by RNA seqFISH+. Nature 568, 235–239. doi:10.1038/s41586-019-1049-y
Fagerberg, L., Hallström, B. M., Oksvold, P., Kampf, C., Djureinovic, D., Odeberg, J., et al. (2014). Analysis of the human tissue-specific expression by genome-wide integration of transcriptomics and antibody-based proteomics. Mol. Cell. Proteomics 13, 397–406. doi:10.1074/mcp.M113.035600
Fan, Y., Chen, M., and Zhu, Q. (2020). lncLocPred: predicting LncRNA subcellular localization using multiple sequence feature information. IEEE Access 8, 124702–124711. doi:10.1109/ACCESS.2020.3007317
Femino, A. M., Fay, F. S., Fogarty, K., and Singer, R. H. (1998). Visualization of single RNA transcripts in situ. Science 280, 585–590. doi:10.1126/science.280.5363.585
Feng, S., Liang, Y., Du, W., Lv, W., and Li, Y. (2020). LncLocation: efficient subcellular location prediction of long non-coding RNA-based multi-source heterogeneous feature fusion. Int. J. Mol. Sci. 21, 7271. doi:10.3390/ijms21197271
Frankish, A., Diekhans, M., Ferreira, A.-M., Johnson, R., Jungreis, I., Loveland, J., et al. (2019). GENCODE reference annotation for the human and mouse genomes. Nucleic Acids Res. 47, D766–D773. doi:10.1093/nar/gky955
Gao, Y., Shang, S., Guo, S., Li, X., Zhou, H., Liu, H., et al. (2021). Lnc2Cancer 3.0: an updated resource for experimentally supported lncRNA/circRNA cancer associations and web tools based on RNA-seq and scRNA-seq data. Nucleic Acids Res. 49, D1251–D1258. doi:10.1093/nar/gkaa1006
Gardini, A. (2017). Global run-On sequencing (GRO-seq). Methods Mol. Biol. 1468, 111–120. doi:10.1007/978-1-4939-4035-6_9
German, M. A., Luo, S., Schroth, G., Meyers, B. C., and Green, P. J. (2009). Construction of Parallel Analysis of RNA Ends (PARE) libraries for the study of cleaved miRNA targets and the RNA degradome. Nat. Protoc. 4, 356–362. doi:10.1038/nprot.2009.8
Gudenas, B. L., and Wang, L. (2018). Prediction of LncRNA subcellular localization with deep learning from sequence features. Sci. Rep. 8, 16385. doi:10.1038/s41598-018-34708-w
Gyllborg, D., Langseth, C. M., Qian, X., Choi, E., Salas, S. M., Hilscher, M. M., et al. (2020). Hybridization-based in situ sequencing (HybISS) for spatially resolved transcriptomics in human and mouse brain tissue. Nucleic Acids Res. 48, e112. doi:10.1093/nar/gkaa792
Hartford, C. C. R., and Lal, A. (2020). When long noncoding becomes protein coding. Mol. Cell. Biol. 40, 005288–e611. doi:10.1128/MCB.00528-19
Heinrich, S., Sidler, C. L., Azzalin, C. M., and Weis, K. (2017). Stem-loop RNA labeling can affect nuclear and cytoplasmic mRNA processing. RNA 23, 134–141. doi:10.1261/rna.057786.116
Hon, C.-C., Ramilowski, J. A., Harshbarger, J., Bertin, N., Rackham, O. J. L., Gough, J., et al. (2017). An atlas of human long non-coding RNAs with accurate 5’ ends. Nature 543, 199–204. doi:10.1038/nature21374
Jeon, Y.-J., Hasan, M. M., Park, H. W., Lee, K. W., and Manavalan, B. (2022). TACOS: a novel approach for accurate prediction of cell-specific long noncoding RNAs subcellular localization. Briefings Bioinforma. 23, bbac243. doi:10.1093/bib/bbac243
Kaewsapsak, P., Shechner, D. M., Mallard, W., Rinn, J. L., and Ting, A. Y. (2017). Live-cell mapping of organelle-associated RNAs via proximity biotinylation combined with protein-RNA crosslinking. Elife 6, e29224. doi:10.7554/elife.29224
Keene, J. D., Komisarow, J. M., and Friedersdorf, M. B. (2006). RIP-Chip: the isolation and identification of mRNAs, microRNAs and protein components of ribonucleoprotein complexes from cell extracts. Nat. Protoc. 1, 302–307. doi:10.1038/nprot.2006.47
Kishi, J. Y., Lapan, S. W., Beliveau, B. J., West, E. R., Zhu, A., Sasaki, H. M., et al. (2019). SABER amplifies FISH: enhanced multiplexed imaging of RNA and DNA in cells and tissues. Nat. Methods 16, 533–544. doi:10.1038/s41592-019-0404-0
Lee, J. H., Daugharthy, E. R., Scheiman, J., Kalhor, R., Ferrante, T. C., Terry, R., et al. (2015). Fluorescent in situ sequencing (FISSEQ) of RNA for gene expression profiling in intact cells and tissues. Nat. Protoc. 10, 442–458. doi:10.1038/nprot.2014.191
Levesque, M. J., Ginart, P., Wei, Y., and Raj, A. (2013). Visualizing SNVs to quantify allele-specific expression in single cells. Nat. Methods 10, 865–867. doi:10.1038/nmeth.2589
Li, J., Han, L., Roebuck, P., Diao, L., Liu, L., Yuan, Y., et al. (2015). TANRIC: an interactive open platform to explore the function of lncRNAs in cancer. Cancer Res. 75, 3728–3737. doi:10.1158/0008-5472.CAN-15-0273
Li, M., Zhao, B., Yin, R., Lu, C., Guo, F., and Zeng, M. (2023a). GraphLncLoc: long non-coding RNA subcellular localization prediction using graph convolutional networks based on sequence to graph transformation. Briefings Bioinforma. 24, bbac565. doi:10.1093/bib/bbac565
Li, Z., Liu, L., Feng, C., Qin, Y., Xiao, J., Zhang, Z., et al. (2023b). LncBook 2.0: integrating human long non-coding RNAs with multi-omics annotations. Nucleic Acids Res. 51, D186–D191. doi:10.1093/nar/gkac999
Li, Z., Liu, L., Jiang, S., Li, Q., Feng, C., Du, Q., et al. (2021). LncExpDB: an expression database of human long non-coding RNAs. Nucleic Acids Res. 49, D962–D968. doi:10.1093/nar/gkaa850
Lin, Y., Pan, X., and Shen, H.-B. (2021). lncLocator 2.0: a cell-line-specific subcellular localization predictor for long non-coding RNAs with interpretable deep learning. Bioinformatics 37, 2308–2316. doi:10.1093/bioinformatics/btab127
Lorenzi, L., Chiu, H.-S., Avila Cobos, F., Gross, S., Volders, P.-J., Cannoodt, R., et al. (2021). The RNA Atlas expands the catalog of human non-coding RNAs. Nat. Biotechnol. 39, 1453–1465. doi:10.1038/s41587-021-00936-1
Lubeck, E., and Cai, L. (2012). Single-cell systems biology by super-resolution imaging and combinatorial labeling. Nat. Methods 9, 743–748. doi:10.1038/nmeth.2069
Luo, Y., Hitz, B. C., Gabdank, I., Hilton, J. A., Kagda, M. S., Lam, B., et al. (2020). New developments on the Encyclopedia of DNA Elements (ENCODE) data portal. Nucleic Acids Res. 48, D882–D889. doi:10.1093/nar/gkz1062
Mas-Ponte, D., Carlevaro-Fita, J., Palumbo, E., Hermoso Pulido, T., Guigo, R., and Johnson, R. (2017). LncATLAS database for subcellular localization of long noncoding RNAs. RNA 23, 1080–1087. doi:10.1261/rna.060814.117
Mellis, I. A., Gupte, R., Raj, A., and Rouhanifard, S. H. (2017). Visualizing adenosine-to-inosine RNA editing in single mammalian cells. Nat. Methods 14, 801–804. doi:10.1038/nmeth.4332
Pelechano, V., Wei, W., and Steinmetz, L. M. (2013). Extensive transcriptional heterogeneity revealed by isoform profiling. Nature 497, 127–131. doi:10.1038/nature12121
Pertea, M., Shumate, A., Pertea, G., Varabyou, A., Breitwieser, F. P., Chang, Y.-C., et al. (2018). CHESS: a new human gene catalog curated from thousands of large-scale RNA sequencing experiments reveals extensive transcriptional noise. Genome Biol. 19, 208. doi:10.1186/s13059-018-1590-2
Pisignano, G., and Ladomery, M. (2021). Epigenetic regulation of alternative splicing: how LncRNAs tailor the message. Noncoding RNA 7, 21. doi:10.3390/ncrna7010021
Raj, A., and Tyagi, S. (2010). Detection of individual endogenous RNA transcripts in situ using multiple singly labeled probes. Methods Enzym. 472, 365–386. doi:10.1016/S0076-6879(10)72004-8
Romero-Barrios, N., Legascue, M. F., Benhamed, M., Ariel, F., and Crespi, M. (2018). Splicing regulation by long noncoding RNAs. Nucleic Acids Res. 46, 2169–2184. doi:10.1093/nar/gky095
Rouhanifard, S. H., Mellis, I. A., Dunagin, M., Bayatpour, S., Jiang, C. L., Dardani, I., et al. (2018). ClampFISH detects individual nucleic acid molecules using click chemistry-based amplification. Nat. Biotechnol. 37, 84–89. doi:10.1038/nbt.4286
Savulescu, A. F., Bouilhol, E., Beaume, N., and Nikolski, M. (2021). Prediction of RNA subcellular localization: learning from heterogeneous data sources. iScience 24, 103298. doi:10.1016/j.isci.2021.103298
Sayers, E. W., Bolton, E. E., Brister, J. R., Canese, K., Chan, J., Comeau, D. C., et al. (2022). Database resources of the national center for biotechnology information. Nucleic Acids Res. 50, D20–D26. doi:10.1093/nar/gkab1112
Schena, M., Shalon, D., Davis, R. W., and Brown, P. O. (1995). Quantitative monitoring of gene expression patterns with a complementary DNA microarray. Science 270, 467–470. doi:10.1126/science.270.5235.467
Shiraki, T., Kondo, S., Katayama, S., Waki, K., Kasukawa, T., Kawaji, H., et al. (2003). Cap analysis gene expression for high-throughput analysis of transcriptional starting point and identification of promoter usage. Proc. Natl. Acad. Sci. U. S. A. 100, 15776–15781. doi:10.1073/pnas.2136655100
Smola, M. J., Rice, G. M., Busan, S., Siegfried, N. A., and Weeks, K. M. (2015). Selective 2’-hydroxyl acylation analyzed by primer extension and mutational profiling (SHAPE-MaP) for direct, versatile and accurate RNA structure analysis. Nat. Protoc. 10, 1643–1669. doi:10.1038/nprot.2015.103
Statello, L., Guo, C.-J., Chen, L.-L., and Huarte, M. (2021). Gene regulation by long non-coding RNAs and its biological functions. Nat. Rev. Mol. Cell Biol. 22, 96–118. doi:10.1038/s41580-020-00315-9
Su, Z.-D., Huang, Y., Zhang, Z.-Y., Zhao, Y.-W., Wang, D., Chen, W., et al. (2018). iLoc-lncRNA: predict the subcellular location of lncRNAs by incorporating octamer composition into general PseKNC. Bioinforma. Oxf. Engl. 34, 4196–4204. doi:10.1093/bioinformatics/bty508
Sweeney, B. A., Petrov, A. I., Ribas, C. E., Finn, R. D., Bateman, A., Szymanski, M., et al. (2021). RNAcentral 2021: secondary structure integration, improved sequence search and new member databases. Nucleic Acids Res. 49, D212–D220. doi:10.1093/nar/gkaa921
Symmons, O., Chang, M., Mellis, I. A., Kalish, J. M., Park, J., Suszták, K., et al. (2019). Allele-specific RNA imaging shows that allelic imbalances can arise in tissues through transcriptional bursting. PLoS Genet. 15, e1007874. doi:10.1371/journal.pgen.1007874
Szcześniak, M. W., Bryzghalov, O., Ciomborowska-Basheer, J., and Makałowska, I. (2019). CANTATAdb 2.0: expanding the collection of plant long noncoding RNAs. Methods Mol. Biol. 1933, 415–429. doi:10.1007/978-1-4939-9045-0_26
The ENCODE Project Consortium (2012). An integrated encyclopedia of DNA elements in the human genome. Nature 489, 57–74. doi:10.1038/nature11247
Tilgner, H., Knowles, D. G., Johnson, R., Davis, C. A., Chakrabortty, S., Djebali, S., et al. (2012). Deep sequencing of subcellular RNA fractions shows splicing to be predominantly co-transcriptional in the human genome but inefficient for lncRNAs. Genome Res. 22, 1616–1625. doi:10.1101/gr.134445.111
Tsanov, N., Samacoits, A., Chouaib, R., Traboulsi, A.-M., Gostan, T., Weber, C., et al. (2016). smiFISH and FISH-quant - a flexible single RNA detection approach with super-resolution capability. Nucleic Acids Res. 44, e165. doi:10.1093/nar/gkw784
Velculescu, V. E., Zhang, L., Vogelstein, B., and Kinzler, K. W. (1995). Serial analysis of gene expression. Science 270, 484–487. doi:10.1126/science.270.5235.484
Volders, P.-J., Anckaert, J., Verheggen, K., Nuytens, J., Martens, L., Mestdagh, P., et al. (2019). LNCipedia 5: towards a reference set of human long non-coding RNAs. Nucleic Acids Res. 47, D135–D139. doi:10.1093/nar/gky1031
Wan, Y., Qu, K., Ouyang, Z., and Chang, H. Y. (2013). Genome-wide mapping of RNA structure using nuclease digestion and high-throughput sequencing. Nat. Protoc. 8, 849–869. doi:10.1038/nprot.2013.045
Wang, D., Zhang, Z., Jiang, Y., Mao, Z., Wang, D., Lin, H., et al. (2021a). DM3Loc: multi-label mRNA subcellular localization prediction and analysis based on multi-head self-attention mechanism. Nucleic Acids Res. 49, e46. doi:10.1093/nar/gkab016
Wang, T., Cai, J., Sun, S., Tang, L., and Liu, L. (2021b). “A review on predicting subcellular localization of lncRNA,” in 2021 13th international conference on intelligent human-machine systems and cybernetics (IHMSC) (IEEE). doi:10.1109/ihmsc52134.2021.00043
Wang, X., Allen, W. E., Wright, M. A., Sylwestrak, E. L., Samusik, N., Vesuna, S., et al. (2018). Three-dimensional intact-tissue sequencing of single-cell transcriptional states. Science 361, eaat5691. doi:10.1126/science.aat5691
Wang, Z., Gerstein, M., and Snyder, M. (2009). RNA-Seq: a revolutionary tool for transcriptomics. Nat. Rev. Genet. 10, 57–63. doi:10.1038/nrg2484
Wei, L.-H., and Guo, J. U. (2020). Coding functions of “noncoding” RNAs. Science 367, 1074–1075. doi:10.1126/science.aba6117
Wen, X., Gao, L., Guo, X., Li, X., Huang, X., Wang, Y., et al. (2018). lncSLdb: a resource for long non-coding RNA subcellular localization. Database (Oxford) 2018, 1–6. doi:10.1093/database/bay085
Xia, C., Fan, J., Emanuel, G., Hao, J., and Zhuang, X. (2019). Spatial transcriptome profiling by MERFISH reveals subcellular RNA compartmentalization and cell cycle-dependent gene expression. Proc. Natl. Acad. Sci. U. S. A. 116, 19490–19499. doi:10.1073/pnas.1912459116
Yang, X.-F., Zhou, Y.-K., Zhang, L., Gao, Y., and Du, P.-F. (2020). Predicting LncRNA subcellular localization using unbalanced pseudo-k nucleotide compositions. Curr. Bioinforma. 15, 554–562. doi:10.2174/1574893614666190902151038
Zeng, M., Wu, Y., Lu, C., Zhang, F., Wu, F.-X., and Li, M. (2022). DeepLncLoc: a deep learning framework for long non-coding RNA subcellular localization prediction based on subsequence embedding. Briefings Bioinforma. 23, bbab360. doi:10.1093/bib/bbab360
Zhang, S., and Qiao, H. (2020). KD-KLNMF: identification of lncRNAs subcellular localization with multiple features and nonnegative matrix factorization. Anal. Biochem. 610, 113995. doi:10.1016/j.ab.2020.113995
Zhang, T., Tan, P., Wang, L., Jin, N., Li, Y., Zhang, L., et al. (2017). RNALocate: a resource for RNA subcellular localizations. Nucleic Acids Res. 45, D135–D138. doi:10.1093/nar/gkw728
Zhang, Z.-Y., Yang, Y.-H., Ding, H., Wang, D., Chen, W., and Lin, H. (2021). Design powerful predictor for mRNA subcellular location prediction in Homo sapiens. Briefings Bioinforma. 22, 526–535. doi:10.1093/bib/bbz177
Keywords: subcellular localization, multi-label localization, lncRNA, databases, prediction methods, experimental techniques
Citation: Choudhury S, Rathore AS and Raghava GPS (2024) Compilation of resources on subcellular localization of lncRNA. Front. RNA Res. 2:1419979. doi: 10.3389/frnar.2024.1419979
Received: 19 April 2024; Accepted: 17 May 2024;
Published: 06 June 2024.
Edited by:
Alessandro Paolini, Bambino Gesù Children’s Hospital (IRCCS), ItalyReviewed by:
Giovannino Silvestri, University of Maryland Medical Center, United StatesMaxime Wery, Institut Curie, France
Copyright © 2024 Choudhury, Rathore and Raghava. This is an open-access article distributed under the terms of the Creative Commons Attribution License (CC BY). The use, distribution or reproduction in other forums is permitted, provided the original author(s) and the copyright owner(s) are credited and that the original publication in this journal is cited, in accordance with accepted academic practice. No use, distribution or reproduction is permitted which does not comply with these terms.
*Correspondence: Gajendra P. S. Raghava, cmFnaGF2YUBpaWl0ZC5hYy5pbg==