- 1Epidemiology Branch, National Institute of Environmental Health Sciences, Durham, NC, United States
- 2Public Health Sciences, Social / Scientific Systems, Inc., a DLH Company, Durham, NC, United States
- 3Department of Clinical Services and Evidence, Ovia Health, Boston, MA, United States
Background: Mobile applications (apps) present a new opportunity to study menstrual cycles and time to pregnancy. Understanding the characteristics of cycle tracking app users is important to evaluate the feasibility of recruiting participants for preconception research.
Methods: Users of a cycle tracking smartphone app, Ovia Fertility, aged 18 or older in the U.S. were randomly invited via email to complete a “fertility research” questionnaire that included demographic and reproductive characteristics. Among those attempting pregnancy without medical assistance, attempt duration, factors influencing pregnancy planning, health history and behaviors while attempting to conceive were queried. Respondents could choose to enter a raffle for a $50 gift card.
Results: Initially, 639 people responded to the demographics portion of the survey representing 49 states and Washington DC. Of these, 344 (54%) were trying to conceive and of those, 297 (86%) were not using medical treatments. Of those not trying to conceive, 12% reported that they planned to start in the next 3 months. Most participants were ages 26–35 (63%), of White race (70%), reported non-Hispanic ethnicity (87%), had at least a bachelor's degree (56%) and an income between $50,000 and $200,000 (58%). One-third were of recommended BMI (35%), 24% overweight, and 41% obese. Most participants reported no fertility-related health conditions (58%). Forty-eight participants (17%) had been trying to conceive for 1 month or less, 88 (31%) had been trying for 2 months or less, and 122 (43%) for 3 months or less. Interruptions in pregnancy attempts were common, 31% reported periods without intercourse. Of those attempting pregnancy, 47% of partners completed their own questionnaire.
Conclusion: This first-of its-kind analysis describes users of a cycle-tracking smartphone app who could be eligible for recruitment to a prospective time-to-pregnancy study. Survey respondents were diverse with respect to geographic location, BMI, and income. However, special recruitment efforts will be needed to recruit participants and partners who identify as other than non-Hispanic White. Participants with fertility-related conditions are not overly represented among app users who are trying to conceive. Targeting and pre-enrolling app users who are planning to begin a pregnancy attempt in the next 3 months may be an advantage of app-based recruitment.
Introduction
Mobile applications (apps) for tracking menstrual cycles and aiding conception are increasing in popularity. Using an app can help people time their intercourse in relation to the fertile window of the menstrual cycle to either increase the probability of conception (1) or to avoid conception (2). Data collection through the apps is fast, convenient, and helps users understand their own menstrual cycle patterns (3). This increase in popularity means that millions of people contribute to large databases of menstrual cycles and time to pregnancy. These data present a new opportunity to study menstrual cycles and time to pregnancy with detailed data and sample sizes that have never been available before in this research field (4). Before embarking on a large prospective study of menstrual cycles or time to pregnancy, however, it is important to understand the underlying population using these apps. While cycle tracking apps may provide a diverse database of potential study participants for research purposes, this has just begun to be empirically examined; the demographic, behavioral, and reproductive characteristics of app users need further investigation (3).
To plan for app-based recruitment to a time-to-pregnancy study, it is important to characterize app users’ decisions about planning to conceive, the distribution of ongoing attempt timing, and their behaviors while trying to conceive. There is also reason to believe that users of cycle tracking apps may be more likely to have subfertility challenges and are therefore more predisposed to tracking their cycle more closely with the assistance of a digital tool. These individuals may therefore be overrepresented in app-based recruitment studies, thus understanding the prevalence of subfertility and related conditions in these userbases is crucial. Finally, it is important to gauge app users’ interest in research participation, their willingness to provide data beyond that routinely collected by the app, and their partner's interest in study participation.
To address these literature gaps, this pilot study investigates the characteristics of users of a menstrual cycle tracking app called Ovia Fertility who agreed to participate in an online research study. The objectives of this study were to: (1) describe the demographic characteristics of all questionnaire respondents recruited through Ovia Fertility and compare with the Ovia Fertility userbase, (2) estimate the proportion of individuals using the app who plan to start a pregnancy attempt in the next 3 months and could therefore be eligible for enrollment in a time-to-pregnancy study; and (3) estimate the proportion of users currently attempting pregnancy without medical interventions who would be immediately eligible for a time-to-pregnancy study. Additionally, among those who were currently attempting to conceive without medical interventions, we aimed to: (4) assess the diversity of their demographic and reproductive characteristics and describe characteristics of their current pregnancy attempt; (5) describe the factors that influence app users’ decisions around pregnancy planning; and (6) assess willingness of partners of active app users to participate in an app-based research study. The data provided here will inform study design, questionnaire development, sample size calculations, approximate study recruitment timelines, and provide preliminary data on diversity and representativeness.
Materials and methods
The Ovia Fertility app is a free downloadable menstrual cycle tracking app available on both iOS and Android operating systems (https://info.oviahealth.com/enroll). Data that can be recorded by the user include historic and current menstrual period duration and symptom data such as basal body temperature, cervical fluid, physical and emotional symptoms, medications, diet, and exercise. The app is customizable so users can track data points that are most interesting to them, such as symptoms, mood, exercise, sex, or nutrition. Summary data are provided to the user, such as average cycle length, period length, and most frequent symptoms.
To investigate the demographics of Ovia Fertility users likely to participate in research about time-to-pregnancy or preconception studies, we used the Ovia Fertility user database to send emails to a subset of Ovia Fertility users, randomly selected from all users aged 18 or older in the United States. Emails were sent between August 21–25, 2020 and September 17–October 7, 2020 to 90,725 users. The email described a research study of “women's reproductive health” and “factors that influence fertility” and contained a unique link to an electronic questionnaire (Supplementary Figure S1). The number of emails sent, the number of emails opened, and the number of unique link clicks was tracked. Our aim was not to recruit every person who received an email, but to obtain at least 250 responses. Respondents who were willing to provide their contact information were entered into a drawing for one of four $50 gift cards.
All respondents to the questionnaire answered simple demographic questions, such as age, race, ethnicity, and education (N = 639, Figure 1). Data from these respondents are used to describe the demographic characteristics of participants recruited from a cycle tracking app userbase (objective 1). In addition, Ovia Health collects demographic variables upon user enrollment, such as race, ethnicity, education level, and relationship status. These statistics reflect the composition of the Ovia Fertility userbase as of 2022. These aggregate statistics were compared to the demographics of these survey respondents.
The next series of questions identified those who were attempting to become pregnant (Figure 1). First, those who were currently pregnant were identified and excluded from the remainder of the questionnaire (N = 107). Second, participants were asked, “Are you currently using any form of birth control (including but not limited to, the pill, implant, IUD, condoms, abstinence, etc)?” if they said yes, they were considered not trying to become pregnant (N = 78). Third, those who were not pregnant and not using birth control were asked, “Are you currently trying to become pregnant? While there are many ways to become pregnant, in this case we are talking about currently having regular unprotected intercourse with a male partner.” If the participant answered “no” to this question they were considered not trying to conceive (N = 68). The latter two groups of participants were further asked, “Are you planning to try to become pregnant in the next 3 months?” and this was the last question they were asked. Data from those on birth control and those not currently trying were used to estimate the proportion of app users who plan to start a pregnancy attempt in the next 3 months and might then be eligible for enrollment in a time-to-pregnancy study (objective 2) (N = 146). Finally, respondents who reported attempting to become pregnant, were asked, “Are you currently receiving any medical treatments to help you get pregnant?” and if they answered yes, they were excluded from the remainder of the questionnaire (N = 47).
Additional questions were asked of those who were attempting to become pregnant without medical intervention (N = 297) to assess the diversity of their demographic and reproductive characteristics and to describe the current pregnancy attempt and the factors that affect pregnancy planning (objectives 3–6). The additional questions included pregnancy attempt duration, factors influencing pregnancy planning, reproductive and birth control history, and health history and behaviors while attempting to conceive. Body mass index was calculated from the reported height and weight. Users could select multiple factors when identifying influences on their pregnancy planning and their behaviors while attempting to conceive. Finally, they were asked to invite their partners to complete the partner portion of the questionnaire, which included similar domains to those previously described, including demographics and behaviors while attempting to conceive. The partner questions were at the end of the questionnaire that was sent to the app user. We characterized questionnaire responses with frequencies and percentages.
Results
Objective 1: Describe the demographic characteristics of all questionnaire respondents recruited through Ovia Fertility and compare with the Ovia Fertility userbase
In 12 days, a total of 90,725 emails were delivered to unique email addresses,6,771 were opened, and there were 722 unique link clicks (Figure 1). Of these, 639 people answered at least a portion of the survey. Of these, 107 were currently pregnant and 31 were missing pregnancy status, 78 were currently using birth control, and 68 were not currently trying to become pregnant. This left 344 participants who were trying to become pregnant of whom 47 were receiving medical treatments to become pregnant. The remaining 297 participants were trying to become pregnant without medical intervention and eligible for recruitment to a prospective time-to-pregnancy study.
The median time spent answering the survey was 4 min (25th, 75th percentiles: 1, 10 min). Among the 639 who responded to the demographics and descriptive portion of the survey, over 500 responded within 1 day, and 600 responded within 5 days. All respondents participated within 10 days of receiving the questionnaire. The majority of respondents were aged 26–35, self-reported their race as White and their ethnicity as non-Hispanic, had at least a bachelor's degree, were married, and most had an income of $50,000 to $200,000 (Table 1). Respondents came from 49 states and Washington DC, with the largest proportion from California (Table 1).
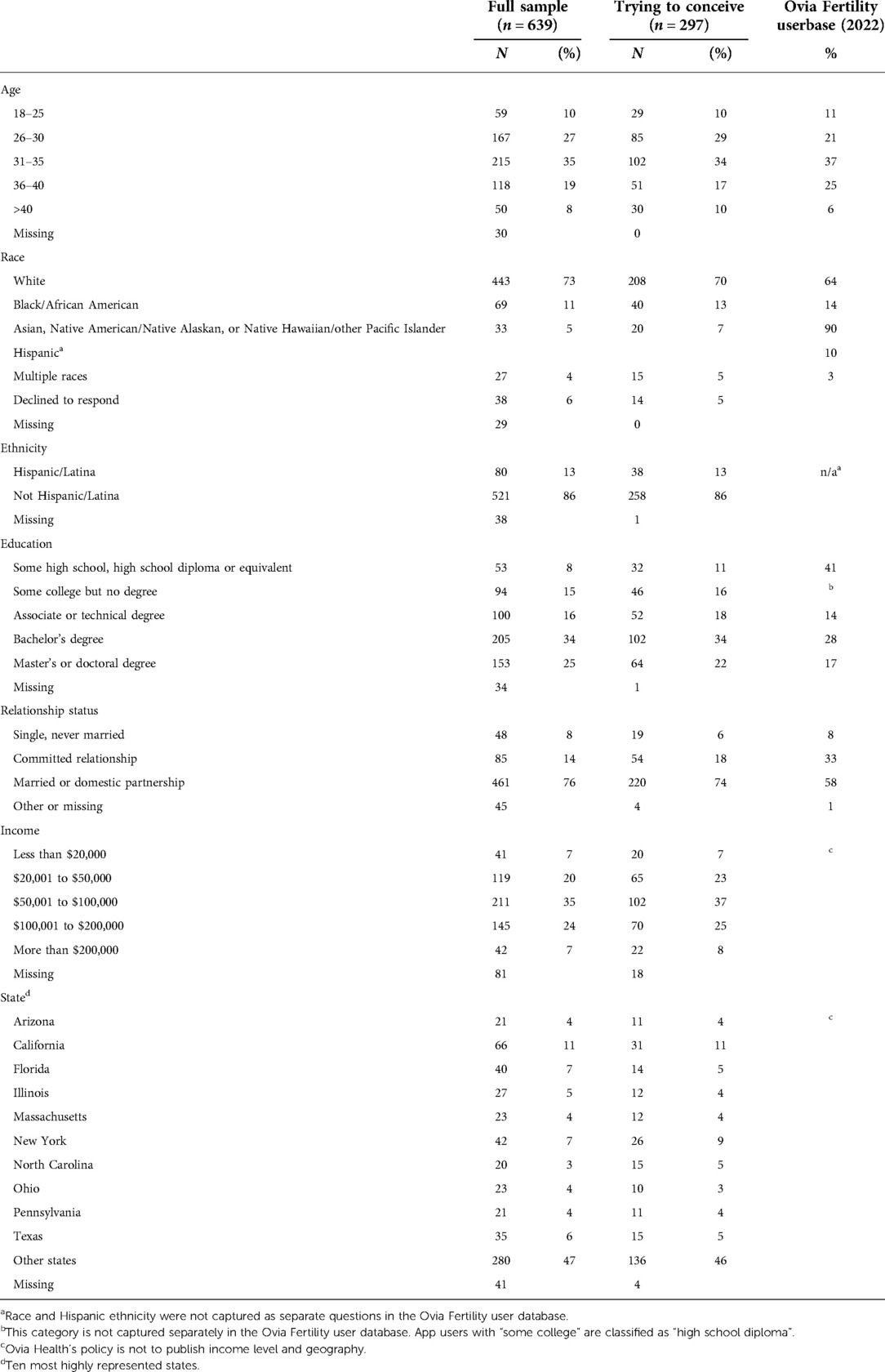
Table 1. Demographics of all survey respondents compared with respondents who were trying to conceive, and the Ovia Fertility U.S. userbase.
In the Ovia Fertility userbase 60% report that they are trying to conceive while 40% report not trying to conceive. The proportion trying to conceive was similar in our survey (344/639 or 54%, Figure 1). Compared with the Ovia Fertility userbase, the survey respondents were slightly younger, had higher educational attainment and were more likely to be married (Table 1). A higher proportion of survey respondents reported their race as White (73%) compared with the Ovia Fertility userbase (64%), while a similar proportion reported their race as Black or African-American (11% vs. 14%) however, the Ovia Fertility userbase does not query race separately from Hispanic ethnicity, which limits the direct comparison of these proportions.
Objective 2: Estimate the proportion of individuals using the app who plan to start a pregnancy attempt in the next 3 months, and would soon be eligible for enrollment in a time-to-pregnancy study
Of the 146 respondents who were not currently trying to conceive, 12% did plan to try in 3 months, 3% were unsure about trying in the next 3 months, 69% were not planning to start trying in the next 3 months and this decision was unrelated to COVID-19, and 16% of respondents were not planning to start trying or were unsure whether they would start trying at least partially due to COVID-19.
Objective 3: Estimate the proportion of individuals who are currently attempting pregnancy without medical treatments and could therefore be immediately eligible for a time-to-pregnancy study
Of the 639 respondents, 344 (54%) were currently trying to become pregnant and of those, 297 (86%) were not using medical treatments to conceive (46% of the total sample).
Objective 4: Among those who were currently attempting to conceive without medical interventions, assess the diversity of the demographic and reproductive characteristics and describe characteristics of their current pregnancy attempt
Demographic characteristics of those trying to conceive were similar to those of all of the survey respondents (Table 1). About one-third of the participants were of recommended BMI, 24% were overweight, and 41% were in obese categories (Table 2). A small proportion of respondents reported ever smoking (19%) and of these, few were current smokers (7%). About one-third of participants self-reported a history of infertility (Table 2). For almost 60% of participants, the longest attempt time was 3 months or less.
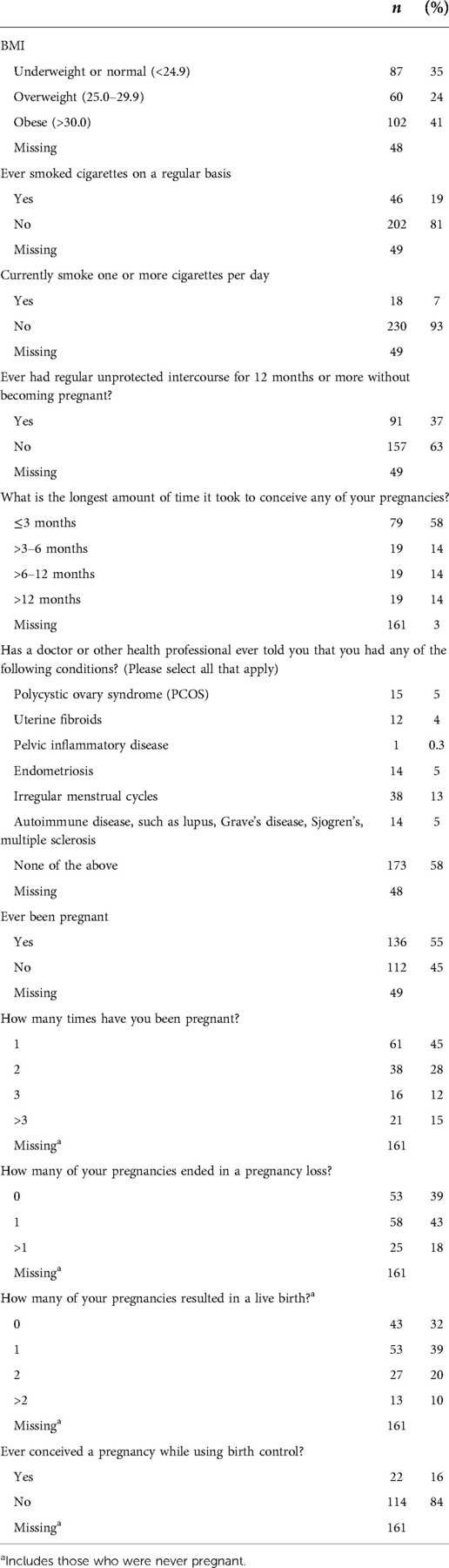
Table 2. Behavioral and reproductive characteristics of participants who were trying to conceive (n = 297).
Most participants reported no history of health conditions related to fertility (58%). Irregular cycles had the highest prevalence (13%) (Table 2). Over half of the sample had been pregnant before (55%). Of those who had been pregnant, 85% had been pregnant 3 times or less, 39% had never had a pregnancy loss, and 43% had one previous loss.
Forty-eight participants (17%) had been trying to conceive for 1 month or less, 88 (31%) had been trying for 2 months or less, and 122 (43%) had been trying for 3 months or less. Among the 73 participants who reported a history of infertility but did not report an attempt time of 12 months or more for any of their pregnancies, 63% had been trying for at least a year in their current attempt, which indicates that the current attempt was the longest attempt in most cases. It is also possible that previous long attempts did not result in a pregnancy (Table 3).
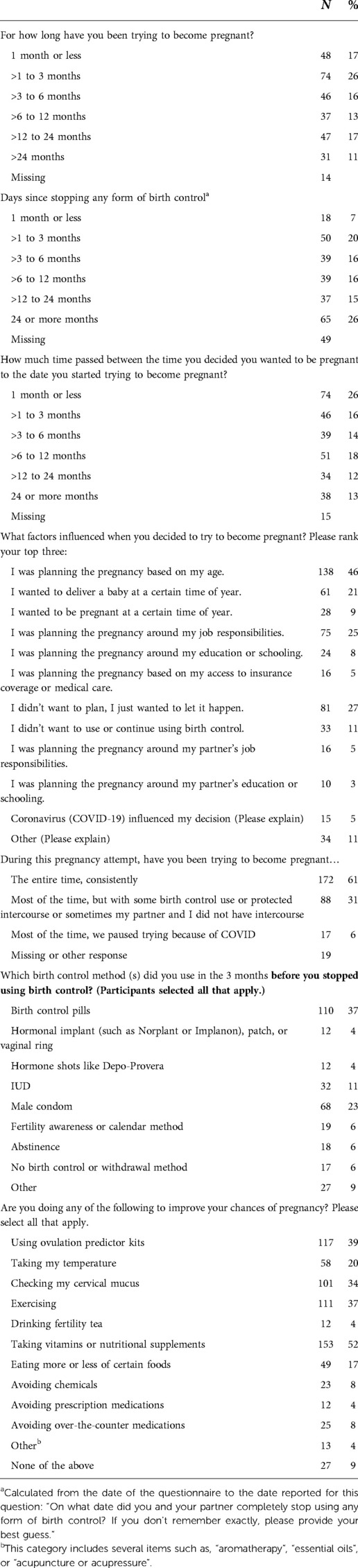
Table 3. Characteristics of the current pregnancy attempt among those who were trying to conceive (n = 297).
One-quarter had stopped birth control within the past 3 months (27%), about one-third had stopped 3–12 months prior (31%), and 41% had stopped more than 12 months prior (Table 3).
A little more than half (52%) reported 6 months or more between deciding to become pregnant and starting to try, however, the most common response was 1 month or less (26%) (Table 3). The median time for women under 30 was 243 days (25th, 75th percentiles: 36, 403) which was longer than the time for women 30 and over, 137 days (25th, 75th percentiles: 30, 365). The median time for those with a high school education or less was 198 days (25th, 75th percentiles: 30, 730) which was slightly longer than those with a masters or doctoral degree, 122 days (25th, 75th percentiles: 30, 365). Participants who reported their race as White reported a median time of 122 days (25th, 75th percentiles: 30, 365) while those who reported their race as Black or African American reported a median time of 365 days (25th, 75th percentiles: 91, 730).
Over one-third of participants reported interruptions in their pregnancy attempt (37%), 31% due to periods of time without intercourse, and 6% due to COVID19 (Table 3).
Respondents to our questionnaire reported a high level of engagement with the app, using it daily (44%) or multiple times per week (29%) (Table 4).
Objective 5: Among those who were currently attempting to conceive without medical intervention we aimed to describe the factors that influence app users’ decisions around pregnancy planning
The most frequently endorsed factor that influenced starting to try was age (46%) (Table 3). The next most frequent responses were “I didn't want to plan, I just wanted to let it happen” (27%), “I was planning the pregnancy around my job responsibilities” (25%), or “I wanted to deliver a baby at a certain time of year” (21%). Among women less than 30 years of age, age was still the most commonly reported factor (39%), however, younger women were more likely than older women to report that they “just wanted to let it happen,” (33% vs. 25%) (Supplementary Table S1). Among participants who reported their race as Black or African American the most common response was wanting to “let it happen” (48%) and then age (37%). Among those with a high school diploma or less, the most common response was “let it happen” and this was far more common in this group than in other education groups (62% vs. 11%–39%). Wanting to “let it happen” was more commonly reported among those who reported a BMI consistent with obesity (35%). Finally, respondents from the western U.S. were least likely to report wanting to “let it happen” (19%) compared with other regions (25%–34%). Respondents in the Midwest (27%) and West (28%) were more likely to report wanting to deliver a baby at a certain time of year. Participants from the South were more likely to report not wanting to use birth control (17%) compared with other regions (7%–9%).
The most frequently selected items were, “taking vitamins or nutritional supplements,” (52%), “using ovulation predictor kits,” (39%), “exercising,” (37%), and “checking my cervical mucus,” (34%) (Table 3). About 9% selected “none of the above”. The amount of time trying was shorter for those who selected “none of the above” (N = 27, median = 60 days, 56% trying less than 90 days) compared with those who reported at least one behavior (N = 222, median = 152 days, 31% trying less than 90 days).
Objective 6: Among those who were currently attempting to conceive without medical intervention, assess the willingness of partners to participate in a research study
Nearly half (47%) of partners agreed to participate in the survey (Table 5). The characteristics of partners mirrored the characteristics of respondents, although they were slightly older (Table 5). The most frequently selected behavior was “exercising” (53%), followed by “taking vitamins or nutritional supplements” (26%). About one-third (36%) reported not doing any of the behaviors listed.
Discussion
We aimed to describe the cycle tracking app users who were interested in participating in pre-pregnancy research studies. In a recruitment period of less than 2 weeks, over 90,000 emails were delivered to Ovia Fertility users. This resulted in a total of 639 respondents of whom 297 respondents reported actively trying to conceive without using a medical intervention. Most questionnaires were completed within a few days of the email being sent. This descriptive analysis is the first of its kind to describe the characteristics of cycle tracking app users likely to be recruited for prospective time-to-pregnancy studies or for preconception pregnancy studies.
Like previously described volunteer, time-to-pregnancy studies (5–8), participants recruited for this study through Ovia Fertility tended to have at least a college degree and identified their race as White. However, our survey respondents were diverse with respect to income, geographic location, and body mass index. To increase representation, an invitation might be targeted to historically underrepresented racial or ethnic groups if the app has collected those data. Also, our study sent a single invitation email to each address and additional follow-up emails could be sent especially to groups with lower initial response rates.
Most participants reported that they had no history of health conditions related to fertility. The prevalence of any one of the queried conditions that might reduce fertility (PCOS, endometriosis, or uterine fibroids) among respondents was around 5%, which is the same or lower than most population estimates (9). The prevalence of irregular cycles in this sample was approximately 13%, which is similar to the 10% reported in the Nurses’ Health Study II (10) but lower than a European cohort which reported 19% (11). This suggests that people with diagnosed conditions affecting fertility would not be disproportionately recruited from app users.
A high proportion of respondents trying to conceive self-reported a history of pregnancy loss (61%), which might indicate that people begin using an app after experiencing a pregnancy loss. Additionally, a substantial proportion of respondents reported a history of infertility (37%). The current pregnancy attempt was longer than 12 months for 28% of the sample, but 43% of respondents had been trying to conceive for 3 months or less. This distribution is somewhat shifted to longer attempt times compared with other time-to-pregnancy cohorts. One previous cohort reported 56% had been trying to conceive for less than 3 months at enrollment and 9% for at least 12 months (12). Another study reported a median attempt time of 3 months at enrollment (in our study it was about 5 months) (13). This may be partially explained by length-biased sampling (14) which occurs when people are recruited to a study after their pregnancy attempt has begun. To address this selection bias and reduce inclusion of infertile participants, time-to-pregnancy studies may choose to limit study enrollment to those who have not previously experienced infertility and enroll app users who have only been trying for a short time, such as less than 30, 60, or 90 days, or even limit to women planning to start trying in the near future.
The distribution of attempt time was shorter, overall, than the distribution of the time since stopping birth control. This could be due to many things such as a prior pregnancy followed by a period without birth control before trying again, a waiting period after hormonal birth control, or a change in family situation. There may also have been women who were having sex and not preventing pregnancy, but it was only later that they considered themselves to be “trying”. Future queries will be needed to understand the reasons for the differences between these two responses and to develop questions that can be used to assess this interesting phenomenon in future time to pregnancy studies.
Respondents reported doing several things to improve their chances of pregnancy including taking vitamins, using ovulation predictor kits and cervical mucus monitoring, exercising, and eating more or less of different foods. Further research on the effects of these behaviors on time to pregnancy would thus be feasible, given their prevalence. On the other hand, behaviors such as aromatherapy, essential oils, acupuncture/acupressure were rare and would be more difficult to research. The amount of time trying was shorter for those who reported not doing any of the behaviors listed compared with those who did report at least one behavior listed. This likely indicates that couples are more likely to add or change their behaviors to try to increase the chance of conception as their pregnancy attempt lengthens, which has been reported previously (15). It will be helpful in prospective time-to-pregnancy studies to regularly ask, potentially at the beginning of each menstrual cycle, what the couple might be doing to help them conceive and to include a response option of just “letting it happen”.
Among those who were currently using birth control or were not trying to become pregnant, 12% were planning to start a pregnancy attempt in the next 3 months and another 15% were unsure. Among those who were trying to conceive, we asked the amount of time between deciding to become pregnant and starting to try. This question characterizes the amount of time a person knows they are going to try to conceive but hasn't yet started their attempt. The longer this time window is, the more likely a time-to-pregnancy study could find and invite this person to pre-enroll in the study before the attempt begins. This would allow for monthly follow-up of pregnancy prevention methods and unprotected intercourse events so that variation in what is meant by “starting to try to conceive” can be described. Targeting and pre-enrolling app users who are planning to begin a pregnancy attempt may be a special advantage of app-based recruitment because it would allow for more precision in identifying the true start of a pregnancy attempt. Our data indicated that for one quarter of the respondents the planning window that could be targeted for pre-enrollment is quite short, 1 month or less. Another 30% reported a planning window of 1–6 months, and about 44% of the respondents reported a window of more than 6 months. In addition, although the sample was small, we found some evidence that this time is more than twice as long in Black or African American participants compared with White participants. Differences by age and education were much smaller.
When recruiting participants for a prospective preconception study, it is useful to recognize the influences on timing the start of a pregnancy attempt. Age was the most frequently reported influence, but timing of the year was also important, indicating that there may be seasonal patterns to pregnancy attempts (16). This may influence selection and recruitment efficiency across the year. A substantial proportion of respondents also reported wanting to “let it happen.” We are unaware of any data on how these factors that affect timing of an attempt might also influence willingness to participate in a research study or the subsequent quality of data collection for those who do enroll, for example, does wanting to “let it happen” influence a person's willingness to enroll in a detailed prospective study? While the sample of Black and African American participants was small, there was some evidence that wanted to “let it happen” was the most common motivation for starting a pregnancy attempt and was even more frequently reported than age. Further research on factors that influence pregnancy attempt timing would be valuable.
Our data show that 40% of respondents experienced interruptions in their attempt to become pregnant. Despite the survey being deployed during the COVID19 pandemic, most interruptions were unrelated to the pandemic and were instead related to other factors, such as employment, preference, or other reasons. This highlights the importance of collecting information regarding these interruptions during a prospective study, which is supported by a previous study that examined women's cycle-to-cycle variability in pregnancy intention (17). The frequency and length of the interruptions may be related to other demographic or behavioral variables that are being examined for their association with time to pregnancy. Unaccounted for interruptions will contribute to measurement error, and if errors differ by exposure status, the estimates will be biased.
About half of the partners of respondents were willing to participate in this research questionnaire. The importance of partners for time to pregnancy research has been increasingly recognized (18). Further research on partners’ motivation for joining a research study is important for improving participation rates. Of the partners that responded to our survey, about half reported exercising and one quarter taking supplements to improve their partner's chances of pregnancy. These data indicate that research on these behaviors might have adequate sample size.
In summary, recruiting menstrual tracking app users for prospective time-to-pregnancy studies could have several advantages. Cycle tracking mobile applications have become a popular tool for millions of individuals to track and better understand their reproductive health. These applications generate massive datasets related to fertility, menstrual cycle data collection, and other reproductive health indicators. Time-to-pregnancy studies can be limited by small sample sizes and are time-consuming to enroll. Recruitment for time-to-conception or pregnancy research through cycle tracking apps can be rapid and geographically diverse. The large base population of users can allow for stratified study enrollment based on specific factors such as race and ethnicity so that inclusivity of enrollment is assured.
Data availability statement
The data generated for this study can be obtained by written request from the corresponding author.
Ethics statement
The initial email contained the information about the study. If the potential participant clicked on the link for the survey, this was considered willingness to participate. Participants were also free to discontinue the survey at any time. Ovia Health received IRB exemption for their role in the project through an independent review board, Advarra, and NIEHS investigators were considered not involved in human subjects' research. The studies involving human participants were reviewed and approved by Advarra. Written informed consent for participation was not required for this study in accordance with the national legislation and the institutional requirements.
Author contributions
AMZJ conceived of the project and contributed to the design of the study, and the data analysis. HRJ and NM contributed to data analysis. DB contributed to the design and implementation of the study. SMM contributed to data analysis. DDB contributed to the design of the study. All authors contributed to the article and approved the submitted version.
Funding
This research was supported by the intramural research program of the National Institute of Environmental Health Sciences under award number Z01ES103333.
Acknowledgments
We appreciate feedback from Dana Alhasan and Joseph Stanford who provided feedback on an earlier version of this manuscript.
Conflict of interest
SM is employed by Ovia Health. DB was employed by Ovia Health at the time of this study. NM is employed by Social / Scientific Systems, Inc., a DLH Company. The remaining authors declare that the research was conducted in the absence of any commercial or financial relationships that could be construed as a potential conflict of interest.
Publisher's note
All claims expressed in this article are solely those of the authors and do not necessarily represent those of their affiliated organizations, or those of the publisher, the editors and the reviewers. Any product that may be evaluated in this article, or claim that may be made by its manufacturer, is not guaranteed or endorsed by the publisher.
Supplementary material
The Supplementary Material for this article can be found online at: https://www.frontiersin.org/articles/10.3389/frph.2022.981878/full#supplementary-material.
Supplementary Table 1: Factors that influenced the timing of a pregnancy attempt stratified by participant characteristics.
Supplementary Image 1: Recruitment email sent to selected Ovia Fertility app users.
References
1. Stanford JB, Willis SK, Hatch EE, Rothman KJ, Wise LA. Fecundability in relation to use of mobile computing apps to track the menstrual cycle. Hum Reprod. (2020) 35(10):2245–52. doi: 10.1093/humrep/deaa176
2. Pearson JT, Chelstowska M, Rowland SP, Benhar E, Kopp-Kallner H, Berglund Scherwitzl E, et al. Contraceptive effectiveness of an FDA-cleared birth control app: results from the natural cycles U.S. cohort. J Womens Health. (2021) 30(6):782–8. doi: 10.1089/jwh.2020.8547
3. Schantz JS, Fernandez CSP, Anne Marie ZJ. Menstrual cycle tracking applications and the potential for epidemiological research: a comprehensive review of the literature. Curr Epidemiol Rep. (2021) 8(1):9–19. doi: 10.1007/s40471-020-00260-3
4. Jukic AM. Challenges and future directions in menstrual cycle research. Paediatr Perinat Epidemiol. (2020) 34(3):328–30. doi: 10.1111/ppe.12664
5. Buck Louis GM, Sundaram R, Sweeney AM, Schisterman EF, Maisog J, Kannan K. Urinary bisphenol A, phthalates, and couple fecundity: the longitudinal investigation of fertility and the environment (LIFE) study. Fertil Steril. (2014) 101(5):1359–66. doi: 10.1016/j.fertnstert.2014.01.022
6. Crowe HM, Wesselink AK, Wise LA, Wang TR, Horsburgh CR, Mikkelsen EM, et al. Antibiotics and fecundability among female pregnancy planners: a prospective cohort study. Hum Reprod. (2021) 36(10):2761–8. doi: 10.1093/humrep/deab173
7. Jukic AMZ, Baird DD, Weinberg CR, Wilcox AJ, McConnaughey DR, Steiner AZ. Pre-conception 25-hydroxyvitamin D (25(OH)D) and fecundability. Hum Reprod. (2019) 34(11):2163–72. doi: 10.1093/humrep/dez170
8. Schisterman EF, Mumford SL, Schliep KC, Sjaarda LA, Stanford JB, Lesher LL, et al. Preconception low dose aspirin and time to pregnancy: findings from the effects of aspirin in gestation and reproduction randomized trial. J Clin Endocrinol Metab. (2015) 100(5):1785–91. doi: 10.1210/jc.2014-4179
9. Bozdag G, Mumusoglu S, Zengin D, Karabulut E, Yildiz BO. The prevalence and phenotypic features of polycystic ovary syndrome: a systematic review and meta-analysis. Hum Reprod. (2016) 31(12):2841–55. doi: 10.1093/humrep/dew218
10. Wang YX, Shan Z, Arvizu M, Pan A, Manson JE, Missmer SA, et al. Associations of menstrual cycle characteristics across the reproductive life span and lifestyle factors with risk of type 2 diabetes. JAMA Netw Open. (2020) 3(12):e2027928. doi: 10.1001/jamanetworkopen.2020.27928
11. Real FG, Svanes C, Omenaas ER, Anto JM, Plana E, Janson C, et al. Menstrual irregularity and asthma and lung function. J Allergy Clin Immunol. (2007) 120(3):557–64. doi: 10.1016/j.jaci.2007.04.041
12. Wise LA, Rothman KJ, Mikkelsen EM, Stanford JB, Wesselink AK, McKinnon C, et al. Design and conduct of an internet-based preconception cohort study in North America: pregnancy study online. Paediatr Perinat Epidemiol. (2015) 29(4):360–71. doi: 10.1111/ppe.12201
13. Schisterman EF, Silver RM, Perkins NJ, Mumford SL, Whitcomb BW, Stanford JB, et al. A randomised trial to evaluate the effects of low-dose aspirin in gestation and reproduction: design and baseline characteristics. Paediatr Perinat Epidemiol. (2013) 27(6):598–609. doi: 10.1111/ppe.12088
14. Weinberg CR, Wilcox AJ, Jukic AM. Reproductive epidemiology. In: Lash TL, VanderWeele TJ, Haneuse S, Rothman KJ, editors. Modern epidemiology vol. 4. Philadelphia: Wolters Kluwer (2021).
15. Wise LA, Wesselink AK, Hatch EE, Weuve J, Murray EJ, Wang TR, et al. Changes in behavior with increasing pregnancy attempt time: a prospective cohort study. Epidemiology. (2020) 31(5):659–67. doi: 10.1097/EDE.0000000000001220
16. Wesselink AK, Wise LA, Hatch EE, Mikkelsen EM, Sorensen HT, Riis AH, et al. Seasonal patterns in fecundability in North America and Denmark: a preconception cohort study. Hum Reprod. (2020) 35(3):565–72. doi: 10.1093/humrep/dez265
17. Stanford JB, Smith KR, Varner MW. Impact of instruction in the creighton model fertilitycare system on time to pregnancy in couples of proven fecundity: results of a randomised trial. Paediatr Perinat Epidemiol. (2014) 28(5):391–9. doi: 10.1111/ppe.12141
Keywords: menstrual cycle, tracking app, mobile application, digital health, time to pregnancy, fertility
Citation: Jukic AMZ, Jahnke HR, MacNell N, Bradley D, Malloy SM and Baird DD (2022) Feasibility of leveraging menstrual cycle tracking apps for preconception research recruitment. Front. Reprod. Health 4:981878. doi: 10.3389/frph.2022.981878
Received: 29 June 2022; Accepted: 14 September 2022;
Published: 30 September 2022.
Edited by:
Linda G. Kahn, New York University, United StatesReviewed by:
Talya Shaulov, University of Montreal Hospital Centre (CRCHUM), CanadaElizabeth Hatch, Boston University, United States
© 2022 Jukic, Jahnke, MacNell, Bradley, Malloy and Baird. This is an open-access article distributed under the terms of the Creative Commons Attribution License (CC BY). The use, distribution or reproduction in other forums is permitted, provided the original author(s) and the copyright owner(s) are credited and that the original publication in this journal is cited, in accordance with accepted academic practice. No use, distribution or reproduction is permitted which does not comply with these terms.
*Correspondence: Anne Marie Z. Jukic anVraWNhQG5pZWhzLm5paC5nb3Y=
Specialty Section: This article was submitted to Reproductive Epidemiology, a section of the journal Frontiers in Reproductive Health