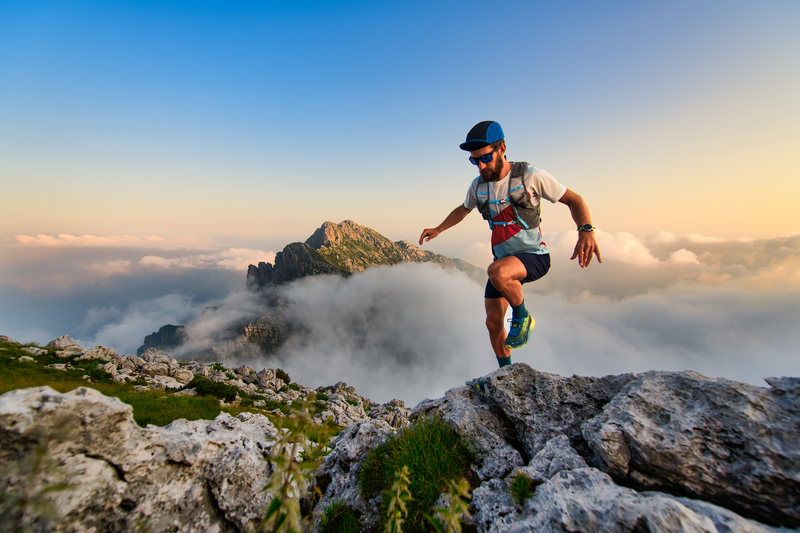
95% of researchers rate our articles as excellent or good
Learn more about the work of our research integrity team to safeguard the quality of each article we publish.
Find out more
ORIGINAL RESEARCH article
Front. Remote Sens. , 13 March 2025
Sec. Land Cover and Land Use Change
Volume 6 - 2025 | https://doi.org/10.3389/frsen.2025.1550331
This article is part of the Research Topic Leveraging Open-source Geospatial Data for Advancing Soil Erosion Research View all articles
Soil erosion has emerged as a significant global concern, posing a critical challenge particularly affecting natural resources and agriculture in emerging nations. Understanding the extent and spatial pattern of soil erosion is vital for effective planning and the implementation of targeted soil conservation strategies, especially under limited resource conditions. This research was carried out in the Gununo watershed, where soil erosion endangers agricultural productivity and environmental health. Primary and secondary datasets such as coordinate points, soil samples, digital soil map, meteorological data, digital elevation model (DEM), and Landsat images were collected. Using RUSLE model in the GIS environment, this study calculated a mean annual soil loss, identified high-risk areas, and prioritized subwatersheds (WHs) for intervention. The overall analysis was carried out by multiplying input factors together in a raster calculator to quantify soil loss of the entire watershed. The analysis revealed that annual erosion varied between 0 and 360 t ha−1 yr−1, with an average of 22 t ha−1 yr−1. Approximately 36% of the area was classified as experiencing moderate to very severe classes, contributing 72.2% of the annual soil loss. The finding indicated that cultivated and bare lands are the most vulnerable land use classes which comprise 73% of the annual loss. The northeast and central-west zones of the study area emerged as erosion hotspots. Based on average annual erosion rate, the subwatersheds WH-4, WH-5, WH-7, WH-8, WH-3, WH-9, WH-6, WH-2, WH-10, and WH-1, were assigned sequential priority levels from 1–10. Among these, the first six consecutive WHs, covering 57.5% of the total landmass, exceeded tolerable soil loss rates, highlighting their urgent need for intervention. This research highlights the significance of earth observation in advancing sustainable land management and contributing to the goals of the SDG 2030 agenda.
Soil erosion is the displacement of soil and its materials from their original source to other deposition areas, facilitated by agents such as air and water through the process of detachment, transportation, and deposition. In the 21st-century, water-induced soil erosion is the major global challenge that poses a threat to agricultural productivity and the sustainability of the environmental systems (Abdo, 2022; Kanito, 2020; Mohammed et al., 2020; Richi, 2025). Despite the ambitious goal set by the most recent UN Sustainable Development Goals (SDGs) report to achieve zero land degradation by 2030, soil erosion has emerged the worst form of land degradation, exerting a sustained and severe impact on the environment, SOC, agricultural productivity, and water resources (Sims et al., 2021). The problem is worsened by a growing population with diverse interests causing heightened demands on land resources for agriculture expansion, overgrazing, alterations in land use land cover (LULC), and extensive rainstorms coupled with the expansion of agricultural into marginal and fragile ecosystems (Han et al., 2020; Hossain et al., 2020; Kanito, 2020; Wolde et al., 2023). Furthermore, uncontrolled urbanization, deforestation, inappropriate farming practices and cultivation without necessary soil and water conservation measures directly contribute to soil erosion (Liliwirianis et al., 2023).
In the Ethiopian highlands, significant soil erosion induced severe land degradation began with cultivating marginal lands and deforestation for agricultural purposes (Wassie, 2020). The rising human population and associated demands for food and fuelwood have further amplified these practices, resulting in environmental degradation, particularly in arid and semi-arid areas. Meng et al. (2021) indicated that higher soil loss on cultivated land without protective measures compared to vegetated land. The Gununo watershed, with a population density of nearly 450 individuals km-1, experiences intense resources pressure especially deforestation of natural forest to expand agricultural land to satisfy growing population demand. This has exacerbated erosion problems, necessitating an assessment of soil erosion is essential to identify erosion hotspot areas and to plan and construct appropriate conservation strategies. Remote sensing, when integrated with GIS offers a practical and efficient approach for evaluating soil erosion due to its simplicity and rapid and cost-effective means for monitoring remote and inaccessible areas (Lin et al., 2024; Yadeta et al., 2021; Anderson et al., 2017). In previous studies, several researchers have demonstrated the effectiveness of integrating GIS and remote sensing in assessing soil erosion in various regions. For instance, studies by Mehwish et al. (2024) and Weslati and Serbaji (2024) emphasize how remote sensing data, in conjunction with GIS tools, can help identify erosion-prone areas, map soil loss, and support the planning of targeted conservation strategies. Furthermore, Teku et al. (2024) also highlighted the value of remotely sensed data for evaluating soil erosion in Ethiopia, noting its accuracy in detecting soil erosion hotspots. These findings underscore the importance of GIS and remote sensing as complementary tools for effective soil erosion assessment and management, aligning with the approach taken in this study.
The RUSLE model has been tested and modified with valid results in different parts of the world (Gayen et al., 2019). In Ethiopia, the RUSLE model has been tested, modified with some of its factors, and proved to be valid (Belayneh et al., 2019). Thus, this study used the RUSLE model which is applicable with the limited available data and ease use of its parameters calibrated for Ethiopian conditions. This study leverages newly available rainfall datasets from Water and Land Resource Centre (WALRC) to develop R factor for RULSE. These datasets are being applied in this context for the first time, providing a more comprehensive and granular insight that was previously unexplored. This data provides a more comprehensive and granular insights that were previously unexplored. The innovative aspect of this work also lies in establishing a clear baseline for SDG15.3.1 monitoring while simultaneously offering practical guidance for conservation planning.
The Soil Conservation Research Program (SCRP) initiated in the study area during the 1980s to promote land conservation and water management efforts (SCRP, 2000). However, the impacts of these efforts fell short of expectations and soil erosion persisted due to factors like deforestation, unsuitable conservation measures, and insufficient data on erosion prone areas. In the Gununo watershed, there has been consistent decline in agricultural productivity and arable land size, significantly impacting the lower watershed regions and contributing substantial sediment to the Gibe III project. Besides, the widespread occurrence of erosion visibly highlights the severity of the problem. Though the watershed is substantially affected by water-induced erosion, limited efforts have been made to identify erosion hotspot areas and implement conservation strategies specifically tailored to high erosion risk zones (Mazengia et al., 2007).
However, considering the financial constraints and the labor and time-intensive nature of watershed management, addressing all the degraded lands and erosion-prone areas through short-term conservation measures may not be feasible when monitoring progress towards SDGs. Therefore, to establish a baseline for the SDG15.3.1, optimize the use of limited resources, and protect the study area from further degradation, it is essential to identify subwatersheds on the basis of the severity of erosion hazard and prioritize them for the recommendation of appropriate conservation measures. This study aims to quantify the mean soil loss rate, identify areas with high erosion vulnerability, and prioritize subwatersheds recognized as erosion hotspots. These objectives are designed to guide the planning and implementation of effective conservation strategies within the Gununo watershed, contributing to sustainable land management, and the mitigation of soil erosion.
The study area (Figure 1c) is located in the midstream section of the Omo-Gibe River basin (Figure 1B), which is among the twelve main River basins of Ethiopia. It covers an area of 204.41 km2 and is geographically positioned between 6° 44′24″N to 6° 55′33.6″N latitude, and 37° 34′48″E to 37° 46′40.8″E longitude. The elevation within the study area spans from 1,576 m to 2,913 m.a.s.l, with a mean elevation of roughly 1,906 m.a.s.l (Figure 2). Additionally, the slope gradient within the study area varies from nearly flat (0°) to extremely steep (49.4°).
Figure 1. Location map (a) Ethiopian main River Basins (b) Omo-Gibe River Basin (c) Gununo watershed.
As stated by Hurni et al. (2016), the study area is primarily classified into the Moist Weyna Dega and Moist Dega agroecological zones, with Moist Weyna Dega being the predominant zone. Additionally, the rainfall pattern in the region follows a bimodal distribution. Climatic data from the Gununo weather station over the thirty-two-year period (1989–2020) indicates an average annual temperature of 19°C with annual temperature ranges showed a peak of 26.1°C and a low of 11.9°C. The total rainfall during this period ranged from 756 mm to 1,394 mm with an average of 1,184 mm.
The geology of the study area consists of volcanic rocks of ignimbrites, rhyolite, trachites, and tuffs (Shiferaw et al., 2013). The soils in the study area are categorized as (Table 1).
To achieve the objectives, both primary and secondary data were collected. Primary data included field observations using GPS for ground truth verification, random field visits to collect points from different LULC classes, and soil sampling to validate soil color using the Munsell color chart. Secondary data included digital soil maps, climatic data, Landsat images, and digital elevation models (DEMs) obtained from various sources. The soil map in vector format was acquired from the Ministry of Water and Irrigation of Ethiopia (MoWIE), and 58 soil samples were collected across the watershed to verify soil color. Landsat-8 OLI/TIRS images from 2020 were acquired from USGS for the LULC map, while SRTM DEM data was obtained from NASA’s Earth Explorer website for watershed delineation and slope map generation (https://earthexplorer.usgs.gov). Mean monthly rainfall data spanning the years 1989–2020 were gathered from the respective meteorological agency for the study area. For data processing, the RUSLE model was used within ArcGIS 10.8, where erosion factor layers were formed in raster format to calculate mean annual soil loss. Supervised image classification using the maximum likelihood classification (MLC) was applied for LULC mapping. Kappa coefficient and error matrix were used to evaluate the agreement between the reference data and classified image and the overall classification accuracy respectively.
The RUSLE has been revised from the USLE by adapting the input factors (Allafta and Opp, 2022; Kumar et al., 2022). As the RUSLE model represents revised version of the USLE, it conserves the basic structure of the USLE model, maintaining a similar factor that determine soil loss. The RUSLE model estimates soil loss by incorporating factors such as conservation practices, climate, land cover, topography, and soil properties. To assess the average annual soil loss and the spatial distribution of its risk, the RUSLE model factors were structured in five independent multiplicative raster formats (Equation 1; Renard et al., 1997).
Erosivity estimation in the RUSLE model is computed by multiplying the total storm energy with the 30-min rainfall intensity, represented as R = EI30. Several empirical equations have been developed for areas with insufficient rainfall data. Similarly, Hurni (1985) developed an empirical equation for computing R factor value using average annual rainfall data, specifically for Eritrean and Ethiopian highlands, adopting the USLE. This particular study utilized 2–5 years of research data from six stations of the SCRP. In the following equation, R represents the erosivity factor (MJ mm h−1 ha−1 yr−1), and P denotes the average annual rainfall (mm) (Equation 2). Using 32 years of average annual rainfall, the R factor for both within the study area (Gununo) and adjacent meteorological stations (Areka, Sodo Zuria and Boditi) was estimated.
The K value is typically determined using nomographs created by Wischmeier and Smith (1978a). The result obtained from the nomograph satisfactorily works for the situations resembling those for which the nomograph was originally developed. Furthermore, the obtained K value is influenced by soil physical properties, including texture, organic matter content, structure, and permeability. However, limitations to determine soil properties is a serious obstacle for estimation of soil erodibility on a large spatial scale (Efthimiou, 2020). Hence, in data-scares regions such as Ethiopia, in situ determination of erodibility factor across large watersheds has become impractical. Thus, this study adopted for the soil type-color approach developed by (Hellden, 1987; Hurni, 1985; Table 2).
In this study, the LS factor values were extracted from the 30 * 30 m DEM using Equation 3, as proposed by Moore and Wilson (1992) which has been broadly utilized, tested, and proven successful in many studies in the Ethiopian context (Getachew et al., 2022).
were, α = represents flow accumulation; χ = represents grid cell size; 22.13 represents the standard plot length in the RUSLE model and Ɵ = represents the slope angle (calculated as the slope of the DEM in degree multiplied by 0.01745 to convert it to radians).
For estimation of the C value, the most common approaches involve using the LULC map and the normalized difference vegetation index (NDVI). For this study, the LULC classification technique was chosen, as it provides more accurate C values compared to NDVI (Lin et al., 2017). A supervised image classification approach was applied with 180 ground truth points (30 for each land cover type) collected. An independent set of 150 reference points, distinct from the training data, was used to perform the accuracy assessment of the classification results. A stratified random sampling technique was implemented to collect reference data, as recommended by Dong et al. (2022). To determine the C values, the watershed was classified into different land use classes, including cultivated land, grass land, built-up, bare land, forestland, and shrub land, based on Landsat imagery from 2020. The C values for corresponding LULC class were assigned based on values recommended and applied in previous studies (Table 3).
Table 3. Land use classes (2020) along with their corresponding assigned C values in the study area.
The P factor represents the ratio of soil erosion occurring after the application of soil conservation practices to the soil loss from conventional straight-row cultivation on slopes (Sinshaw et al., 2021; Unger, 2023). Previously, in part of the study watershed, conservation practices were implemented by the SCRP. However, onsite field observations across the entire watershed confirmed that the constructed conservation measures were poorly designed and maintained resulting in the complete and partial destruction of structures. As a result, this study faced a shortage of data on conservation measures to map the P factor for the respective conservation practices. Hence, owing to the aforementioned facts, it is not reasonable to use existing conservation measures to develop the P factors. Instead, this research utilized an alternative approach by combining the slope map with the reclassified LULC map as suggested by Wischmeier and Smith (1978b). This technique had been adopted by other researchers who carried out similar studies in Ethiopia (Endalew and Biru, 2022; Mengie et al., 2022).
The LULC map of the study area was reclassified into agricultural and other land use categories following the guidelines of Wischmeier and Smith (1978b). Give the land management activity varies with slope, arable land was further divided into different slope classes based on the slope percentage. The reclassified LULC map was then overlaid with the slope map to create a combined land use-slope map. Subsequently, the appropriate P-value was allocated to each land use-slope combination based on empirical values adjusted for the Ethiopian context (Table 6).
The RUSLE parameter layers were transformed into raster format and aligned to the UTM Zone 37N projection with the WGS 1984 datum. Moreover, all five vector layers were resampled to maintain a uniform spatial resolution. The annual soil loss per hectare and its spatial distribution were then computed using Equation 1. Stepwise procedures were implemented to develop soil loss map of the study watershed as shown in Figure 3.
The R-factor map revealed that erosivity within the study area ranged from 657.29 MJ mm ha−1 hr−1 yr−1 (Gununo station) to 773.21 MJ mm ha−1 h−1 year−1 (Areka station) with an average value calculated to be 734.97 MJ mm ha−1 hr−1 yr−1 (Figure 4a). The central-eastern part of the study watershed has a relative high R value and tends to decrease towards the northwestern part of the watershed. This finding indicates that the consequence of rainfall on erosion is lower in the northwest portion of the watershed and it gradually increased to the eastern central portion of the watershed. The relatively high erosivity values observed in certain areas of the watershed may result from a combination of higher annual rainfall distribution and the proximity of interpolation stations. Generally, interpolation accuracy improves with closer proximity to the interpolation point and diminishes as the distance increases.
Figure 4. Spatial variability of parameters in the study area: rainfall erosivity factor (a), soil erodibility factor (b), topographic factor (c), land use land cover (d), support practice factor (e), cover management factor (f), and soil erosion map (g) and Soil erosion severity class (h).
The K value of the watershed ranged from 0.12 t h MJ−1 mm−1 to 0.25 t h MJ−1 mm−1 with a mean of 0.156 t h MJ−1 mm−1 (Figure 4b). Soils with higher K values are more susceptible to erosion, whereas those with lower values exhibit greater resistance. Accordingly, the lowest erodibility values were observed in the Humic Nitisols, followed by the Eutric Vertisols (Table 2). The relatively low erodibility of the Humic Nitisols may be attributed to their well-aggregated soil structure and high clay content, which improve infiltration and minimize soil dispersion (FAO/IUSS-WRB, 2015). This intrinsic resistance to erosion is further supported by studies in tropical regions with similar soils, where Nitisols demonstrated reduced erodibility due to their stable granular structure and high organic matter content (Scatena, 1991). Similarly, the Eutric Vertisols, which often occur in flat to gently sloping terrain, exhibit low susceptibility to erosion due to minimal surface runoff generation under such topographic conditions. This aligns with findings by Bedadi et al. (2023), who noted that Vertisols in Ethiopia are less erodible in flat landscapes due to their high bulk density and lower permeability, which reduce particle detachment.
On the other hand, the highest erodibility values were observed in the Humic Alisols, which are predominantly located along the central-western border of the watershed. These areas are characterized by higher elevations and rugged terrain, increasing their susceptibility to erosion. The high K values of Humic Alisols may primarily be attributed to their unstable surface horizons, which are rich in silt and low in organic matter, making them highly prone to water erosion (FAO/IUSS-WRB, 2015). Furthermore, studies such as those by Morgan (2005) emphasize that soils in steep, rugged terrains with low organic matter are especially vulnerable to high detachment and transport rates due to increased runoff velocity and reduced cohesion.
The LS values ranged from 0 to 34.85, with a mean value of 2.13 (Figure 4c). Regions with steep slopes and high elevations exhibit high LS values, reflecting greater soil susceptibility to erosion (Figure 4g). For instance, the upper right and central west regions, which are characterized by rugged and mountainous terrain with slopes display the highest LS values. These areas correspond to degraded landscapes prone to severe soil erosion due to their steep slopes and lack of adequate vegetation cover. This observation aligns with the findings of Panagos et al. (2015) and Yadeta et al. (2021), who emphasized that the combined slope length and slope angle (LS-factor) has the greatest influence on soil loss at the European and Ethiopian scale respectively. Conversely, the low LS values were recorded in areas with a gentle slope (0%–5%), such as the southeastern parts of the watershed, suggesting that these areas are less susceptible to erosion. Approximately 31.86% of the watershed, predominantly characterized by cultivated land on level to gentle slopes (0%–5%), exhibits low soil loss. This aligns with findings by Setyawan et al. (2019), who reported that flat to gently sloping areas tend to have lower erosion rates due to reduced runoff velocity and deposition of eroded materials. Similarly, Panagos et al. (2015) emphasized that areas with low LS values are less prone to soil loss, as topography plays a key role in reducing erosion intensity. Moreover, it is critical to highlight that while other parameters such as vegetation cover, soil texture, and conservation practices significantly influence erosion. This interaction is also evident in studies such as Yadeta et al. (2021) and Hurni (1985), which demonstrated that areas with high vegetation cover on gentle slopes exhibit lower erosion despite their land use. On the other hand, the remaining 68.67% of the study area is classified as having sloping to very steep terrain, with slopes ranging from 5% to over 49.4% (Table 4). These steep slopes are predominantly found in the upper reaches of the watershed and along major escarpments, where erosion is exacerbated by intense rainfall and limited vegetation. This spatial variation underscores the critical role of topography in influencing soil erosion patterns (Guo et al., 2021).
The primary land use/land cover in the watershed is cultivated land, which is widely distributed across the entire area. The remaining portions are primarily covered by forest, followed by grassland, bare land, built-up areas, and shrublands (Table 3; Figure 4d). The classified image exhibits an overall accuracy of 89% indicating accurate classification with a kappa coefficient of 0.86 confirming that the reference data and classified image have a good agreement (Table 5).
The C factor map result show that C values range from 0.01 to 1 (Figure 4f). Bare land with no vegetation cover was assigned the highest C value (1), followed by cultivated land (0.15) signifying higher susceptibility to erosion (Table 3). In contrast, forested areas had lower C values reflecting their reduced vulnerability to erosion. Moderate C values were assigned to the remaining land use classes indicating that these areas are moderately susceptible to erosion. The C factor values were collected from different studies for different land use types.
All the classes grouped within other land uses including forestland, grassland, built-up areas, bare land, and shrub lands, were assigned a P value of 1 irrespective of their slope class. However, cultivated land was assigned varying values based on its slope class (Table 6).
As illustrated in Figure 4e, the P factor distribution within the watershed varies between 0.1 and 1. The highest P-value of the watershed is predominantly found in the central northwest, southeast, and northeastern regions. This implies the dominance by forest, grasses and shrubs. In contrast, the lower P-values are prevalent in the medium stream and around the outlet parts of the watershed.
The distribution of annual soil loss across the watershed ranged from 0 primarily in the lower regions to 360 t ha−1 yr−1 in the central regions with an average annual soil loss of 22 t ha−1 yr−1 (Figure 4g). Notably, this average exceeds the maximum tolerable soil loss threshold of 18, a value recommended by Hurni (1985) for different agroecology of Ethiopia. This indicates that the agricultural productivity and life-supporting system in the Gununo watershed is at risk.
This study agrees with previous research conducted in the regions having similar conditions. For instance, the average soil loss of 22 t ha−1 yr−1 calculated in current research is comparable to the 27.5 t ha−1 yr−1 reported from Upper Blue Nile River (Haregeweyn et al., 2017), 23.7 t ha−1 yr−1 in the Geleda watershed (Gashaw et al., 2018), and 17.05 t ha−1 yr−1 in the Winike watershed of the Omo-Gibe Basin (Abreham et al., 2018). Generally, the average soil loss in the present study is lower than the national average of 29.9 t ha−1 yr−1 as reported by Haregeweyn et al. (2015).
However, similar studies conducted in other parts of the Ethiopian highlands have reported comparatively higher mean soil loss rates. For example, the study’s soil loss rate is less than those observed in the Koga watershed (47.4 t ha−1 yr−1) (Gelagay and Minale, 2016) and the Rib watershed (68 t ha−1 yr−1) (Moges and Bhat, 2017). Moreover, Gizaw and Degife (2018) documented about 62.98 t ha-1 yr-1 from the Gilgel Gibe-1 catchment and 69 t ha−1 yr−1 from the whole Omo-Gibe basin (Rediet and Eshetu, 2020). The relatively lower average annual soil loss observed in the watershed under study is likely due to the lower LS factor recorded here, contrarily to the higher LS values recorded in other studies. Furthermore, this result could be influenced by the existence of approximately 31.86% of cultivated areas in the level to gentle slope of the watershed. Therefore, this study aligns with Abdo (2021), demonstrating that integrating the RUSLE model with GIS and remote sensing is an effective and adaptable method for assessing soil erosion and devising targeted conservation strategies across diverse geographic and climatic contexts, as evidenced by the Wadi-Qandeel basin in Syria.
In contrast, other researchers have reported significantly lower mean soil loss rates. For instance, Tiruneh and Ayalew (2015) observed about 4.81 t ha−1 yr−1 in the Enfraz watershed, while Gizachew (2015) calculated that the Zingin watershed in the Ethiopian highlands experiences an average yearly soil loss of 9.1 t ha−1 yr−1. Bekele et al. (2019) reported about 4.27 t ha−1 yr−1 in the Karesa Watershed, South West Ethiopia. The difference in these results could be attributed to the changing C factors of the watershed. For example, the Karesa watershed has a maximum C value of 0.15. Recorded on cultivated land that covering about 41.21% of the entire watershed. In contrast, the present study area has maximum C values of 1 (7.73%) and 0.15 (50.14%) for bare land and cultivated land respectively.
The estimated potential soil loss of this finding is inline with studies conducted in the Omo-Gibe basin and Ethiopian highlands. It was determined that the entire watershed experiences an annual soil loss of 1,456,760 tons. This study’s estimated soil loss is almost equivalent to 1.3 million tons annually from 29,500 ha of Koga watershed as estimated by Tegegne and Biniam (2017). Similarly, the annual soil loss of 951,210 tons and 1,243,574 tons in Winike watershed (91,559.1 ha) of the Omo-Gibe basin and the Blue Nile Basin (23,970 ha), respectively was reported (Abreham et al., 2018; Yesuph and Dagnew, 2019).
Erosion risk map (Figure 4h) for the watershed was classified into five severity categories (low, moderate, high, very high, and severe) based on soil loss tolerance (T-value). The class thresholds (0–5, 5–15, 15–30, 30–50, and >50 t ha−1 yr−1) were adapted from Haregeweyn et al. (2017) for Ethiopian highland watersheds and calibrated to local conditions during field survey. According to the map, about 16.12% and 8.91% of the watershed are classified as experiencing severe and very severe erosion rates, respectively (Table 7). Collectively, these classes contributed to about 964,030.42 tons (66.18%) of the total annual soil loss from the watershed. This can be due to higher LS-factor values and a lack of adequate vegetation cover. This signifies that majority of soil loss originated from these areas characterized by high erosion. The central northwest and upper northeast sections of the watershed were predominantly categorized under these risk classes emphasizing the need priority intervention to implement conservation measures (Figure 4g).
The slope of the watershed was reclassified based on the FAO (2006) guidelines to see evaluate the impact of slope class on erosion intensity and to ensure methodological consistency and comparability with global and regional erosion studies. The finding shows that the higher soil loss rate was noticed in areas where relatively large LS values were recorded (Figure 4g). These were areas with steep slope in Gununo watershed, particularly at the northwestern and northeastern regions of the study area. In relation to the slope classes, 65.61% of erosion is from moderately steep slope (41.52%), steep slope (17.01%), and very steep slope (7.08) class categories with mean erosion rate of 34.76, 70.39, and 61.70 t ha−1 yr−1. Across the whole watershed, the steep and very steep slope classes cover an area of 3.38%; but relatively this area contributes high soil loss (24.09%) than the other classes with larger area coverage. This suggests that soil loss rises as slope length and gradient increase (Table 8). This study aligns with the findings of Gashaw et al. (2018) and Woldemariam et al. (2018) who reported a strong relationship between soil loss and slope gradient in Geleda and Gobele watersheds, respectively.
A large portion of the study area is covered by cultivated land (50.14%) which contributes the highest (52.18%) amount of soil loss (Table 9). Following cultivated land, bare land recorded the next highest soil loss, accounting for 20.59% of the total soil loss across the entire study area. The respective mean soil losses for these land types are 53.65 and 74.05 t ha−1 yr−1, which are significantly higher than the tolerable erosion rate in the Ethiopian highlands. Cultivation of steep slopes (27.5%) and the practice of fragile land cultivation and sole cropping are likely principal contributing to the highest soil loss in cultivated land. This is consistent with the findings of Hurni et al. (2016) who identified the cultivation of steep slopes as a major factor contributing to soil loss in Ethiopia. Similarly, in the Gumar watershed, Belayneh et al. (2019) documented higher erosion rates from cultivated land, bare land, and built-up areas in order of their significance. In addition, Sarkar et al. (2024), estimated higher soil erosion in cultivated and fallow lands compared to other LULC classes. On the other hand, forest land is the least vulnerable land-use type to soil erosion, contributing about 2.84% of the watershed’s overall soil loss.
This study prioritized subwatersheds based on their mean annual soil loss to guide the implementation of targeted conservation measures (Table 10). The study findings revealed that WH-5, accounting for 14.18% of the total watershed area, contributed the highest proportion of soil loss, totaling 740,759.4 tons (50.85%) with an average erosion rate of 32.1 t ha−1 yr−1 (Table 10). Similarly, WH-4, which comprises 6.92% of the watershed, contributed significantly about 427,928.6 tons (29.38%) to the total soil loss. This significant soil loss in these subwatersheds can be explained by the combined effects of slope gradient and length, which increase runoff velocity and accelerate soil erosion (Figures 5, 4g). This aligns with findings conducted in the Ethiopian highlands (Haregeweyn et al., 2017), the entire Afghanistan (Ansari and Tayfur, 2023), and the Loess Plateau in China (Sun et al., 2014), where the LS factor strongly correlates with soil loss. In contrast, WH-6, despite covering a larger area, contributed only 45,642.62 tons (3.13%) of the total soil loss, with an average rate of 17.47 t ha−1 yr−1. Besides, WH-10 covering 6.66% of the watershed, contributed the least soil loss 14,490.94 tons (0.99%) with an average erosion rate of 16.9 t ha−1 yr−1. The lower soil loss in WH-10 is likely due to dominance of gentle slopes of the area.
Considering the mean annual soil loss, WH-4, WH-5, WH-7, WH-8, WH-3, WH-9, WH-6, WH-2, WH-10, and WH-1 were assigned priority levels ranging from 1 to 10, reflecting their mean annual soil loss. The average erosion rate across subwatersheds varied from 16.53 to 39.82 t ha−1 yr−1 in WH-1 and WH-4 respectively. Besides, the findings indicated that the average erosion rate in some WHs exceeded the maximum tolerable soil loss rate (18 t ha−1 yr−1) estimated for the country. WH-4, WH-5, WH-7, WH-8, WH-3, and WH-9 surpassed the highest acceptable rate of soil loss, collectively covering 11,741.95 ha (57.45%) of the entire watershed. This highlights the significant vulnerability of the Gununo watershed to soil erosion, posing risks to agricultural production and life-sustaining systems. This study successfully identified and prioritized the degraded land portion and clarified the negative effect of soil erosion on achieving land degradation neutrality. The sub-watershed prioritization results of this study align with the objectives of SDG15.3.1 by enabling targeted interventions to reduce land degradation. These results facilitate efficient allocation of resources to critical erosion hotspots, directly supporting sustainable land management practices in the study area. Thus, urgent attention is required for subwatersheds with mean annual soil loss exceeding the maximum tolerable rate and implementing conservation strategies to prevent further erosion and restore degraded land.
The annual potential soil loss of the watershed was found to be 1.456760 million tons, with a mean soil loss rate of 22 t ha−1 yr−1 which exceeds the maximum tolerable soil loss rate of the country. Besides, the annual soil loss rate in the study area varies from 0 t ha−1 yr−1 in level slope to 360 t ha−1 yr−1 in the very steep slope regions of the watershed. It is found that the higher soil loss is prevailed in areas characterized by steep slopes. 36.06% of the watershed is classified under moderate to very severe erosion class contributing substantial amount of soil loss (72.21%) and are dominantly situated in the central-west and upper-northeast parts of the study area. Among the 10 subwatersheds, 50.85% of soil loss was generated from WH-5. Priority levels ranging from 1 to 10 were assigned to WH-4, WH-5, WH-7, WH-8, WH-3, WH-9, WH-6, WH-2, WH-10, and WH-1. Six subwatersheds (WH-4, WH-5, WH-7, WH-8, WH-3, and WH-9), covering 57.45% of the total land area exceeded the maximum tolerable soil rate enabled to distinguish them as hotspot areas of the watershed. Thus, these WHs need immediate intervention to mitigate soil loss and sustain agricultural production. Overall, the finding support evidence-based decision making and contribute to the strategic implementation of targeted soil and water conservation practices particularly in resource limited contexts. It facilitates the estimating soil loss, providing a baseline for SDG15.3 and contributes to achieving a land degradation neutral world. These benefits not only address local challenges but also offer a methodological framework that can be adopted to other regions facing similar issues of land degradation. This study does not account for gully erosion, which may result in an underestimation of the overall soil loss from the watershed. Future research integrating gully erosion processes is essential to provide a more comprehensive assessment and to recommend more effective intervention strategies.
The datasets presented in this study can be found in online repositories. The names of the repository/repositories and accession number(s) can be found in the article/supplementary material.
DK: Conceptualization, Data curation, Formal Analysis, Writing–original draft. BB: Supervision, Validation, Writing–review and editing. SF: Supervision, Validation, Writing–review and editing. AK-B: Validation, Writing–review and editing. SK: Validation, Writing–review and editing.
The author(s) declare that no financial support was received for the research, authorship, and/or publication of this article.
Authors are very grateful to Ministry of Water and Irrigation of Ethiopia, National Meteorological Agency, and Water and Land Resource Center. Additionally, the authors acknowledge the USGS for SRTM DEM and satellite imageries obtained. Special appreciation is extended to the College of Petroleum Engineering and Geosciences at King Fahd University of Petroleum and Mineral (KFUPM) for the financial support in covering the publication fee.
The authors declare that the research was conducted in the absence of any commercial or financial relationships that could be construed as a potential conflict of interest.
The author(s) declare that no Generative AI was used in the creation of this manuscript.
All claims expressed in this article are solely those of the authors and do not necessarily represent those of their affiliated organizations, or those of the publisher, the editors and the reviewers. Any product that may be evaluated in this article, or claim that may be made by its manufacturer, is not guaranteed or endorsed by the publisher.
Abdo, H. G. (2021). Estimating water erosion using RUSLE, GIS and remote sensing in Wadi-Qandeel river basin, Lattakia, Syria. Proc. Indian Natl. Sci. Acad. 87 (3), 514–523. doi:10.1007/s43538-021-00047-0
Abdo, H. G. (2022). Evaluating the potential soil erosion rate based on RUSLE model, GIS, and RS in Khawabi river basin, Tartous, Syria. DYSONA - Appl. Sci. 3 (1), 24–32. doi:10.30493/DAS.2021.311044
Abreham, B. A., Eyasu, E., Teshome, S., and Gudina, L. F. (2018). Land use/land cover change effect on soil erosion and sediment delivery in the Winike watershed, Omo Gibe Basin, Ethiopia. Sci. Total Environ., 138776. doi:10.1016/j.scitotenv.2020.138776
Allafta, H., and Opp, C. (2022). Soil erosion assessment using the RUSLE model, remote sensing, and GIS in the shatt Al-arab basin (Iraq-Iran). Appl. Sci. Switz. 12 (15), 7776. doi:10.3390/app12157776
Anderson, K., Ryan, B., Sonntag, W., Kavvada, A., and Friedl, L. (2017). Earth observation in service of the 2030 Agenda for Sustainable Development. Geo-Spat. Inf. Sci. 20 (2). doi:10.1080/10095020.2017.1333230
Ansari, A., and Tayfur, G. (2023). Comparative analysis of estimation of slope-length gradient (LS) factor for entire Afghanistan. Geomatics, Nat. Hazards Risk 14 (1). doi:10.1080/19475705.2023.2200890
Bedadi, B., Beyene, S., Erkossa, T., and Fekadu, E. (2023). “Soil management,” in The Soils of Ethiopia (Springer), 193–234.
Bekele, B., Alemayehu, M., and Nigatu, W. (2019). Geographic information system (GIS) based soil loss estimation using universal soil loss equation model (USLE) for soil conservation planning in Karesa watershed, dawuro zone, South West Ethiopia. Int. J. Water Resour. Environ. Eng. 11 (8), 143–158. doi:10.5897/ijwree2018.0820.143-158
Belayneh, M., Yirgu, T., and Tsegaye, D. (2019). Potential soil erosion estimation and area prioritization for better conservation planning in Gumara watershed using RUSLE and GIS techniques. Environ. Syst. Res. 8, 20–17. doi:10.1186/s40068-019-0149-x
Bewket, W., and Teferi, E. (2009). Assessment of soil erosion hazard and prioritization for treatment at the watershed level: case study in the Chemoga watershed, Blue Nile basin, Ethiopia. Land Degrad. Dev. 20 (6), 609–622. doi:10.1002/ldr.944
Boul, S. W., Southard, R. J., Graham, R. C., and McDaniel, P. A. (2013). Soil genesis and classification. Fifth edn. Ames, USA: Iowa state University Press.
Dong, S., Guo, H., Chen, Z., Pan, Y., and Gao, B. (2022). Spatial stratification method for the sampling design of LULC classification accuracy assessment: a case study in Beijing, China. Remote Sens. 14 (4), 865. doi:10.3390/rs14040865
Efthimiou, N. (2020). The new assessment of soil erodibility in Greece. Soil Tillage Res. 204, 104720. doi:10.1016/j.still.2020.104720
Endalew, T., and Biru, D. (2022). Soil erosion risk and sediment yield assessment with revised universal soil loss equation and GIS: the case of nesha watershed, southwestern Ethiopia. Results Geophys. Sci. 12, 100049. doi:10.1016/j.ringps.2022.100049
FAO/IUSS-WRB (2015). World reference base for soil resources 2014, update 2015. International soil classification system for naming soils and creating legends for soil maps. Rome: FAO.
FDRE (2017). Estimation of soil erosion rate (upland erosion), river sedimentation and the soil resources review.
Fenta, A., Yasuda, H., Shimizu, K., Nigussie, H., and Negussie, A. (2016). Dynamics of soil erosion as influenced by watershed management practices: a case study of the Agula watershed in the semi-arid highlands of northern Ethiopia. Environ. Manag. 58, 889–905. doi:10.1007/s00267-016-0757-4
Gashaw, T., Tulu, T., and Argaw, M. (2018). Erosion risk assessment for prioritization of conservation measures in Geleda watershed, Blue Nile basin, Ethiopia. Environ. Syst. Res. 6 (1), 1. doi:10.1186/s40068-016-0078-x
Gayen, A., Saha, S., and Pourghasemi, H. R. (2019). Soil erosion assessment using RUSLE model and its validation by FR probability model. Geocarto Int. 35, 1750–1768. doi:10.1080/10106049.2019.1581272
Gelagay, H. S., and Minale, A. S. (2016). Soil loss estimation using GIS and Remote sensing techniques: a case of Koga watershed, Northwestern Ethiopia. Int. Soil Water Conservation Res. 4 (2), 126–136. doi:10.1016/j.iswcr.2016.01.002
Getachew, W., Kim, D., Li, Q., Eu, S., and Im, S. (2022). Assessing the long-term impact of land-use and land-cover changes on soil erosion in Ethiopia’s Chemoga Basin using the RUSLE model. Landsc. Ecol. Eng. 18 (4), 461–475. doi:10.1007/s11355-022-00518-6
Gizachew, A. (2015). Geographic information system based soil loss and sediment estimation in zingin watershed for conservation planning, highlands of Ethiopia. Int. J. Sci. Technol. Soc. 3 (1), 28.
Gizaw, T., and Degife, T. (2018). Soil erosion modeling using GIS based RUSEL model in Gilgel Gibe-1 catchment, South West Ethiopia. Int. J. Environ. Sci. Nat. Res. 15 (5), 555923. doi:10.19080/IJESNR.2018.15.555923
Guo, L., Liu, R., Men, C., Wang, Q., Miao, Y., Shoaib, M., et al. (2021). Multiscale spatiotemporal characteristics of landscape patterns, hotspots, and influencing factors for soil erosion. Sci. Total Environ. 779, 146474. doi:10.1016/j.scitotenv.2021.146474
Han, J., Wenyan, G., Hei, Z., Cong, C., Ma, C., Xie, M., et al. (2020). Agricultural land use and management weaken the soil erosion induced by extreme rainstorms. Agric. Ecosyst. and Environ. 301, 107047. doi:10.1016/j.agee.2020.107047
Haregeweyn, N., Tsunekawa, A., Nyssen, J., Poesen, J., Tsubo, M., Meshesha, D., et al. (2015). Soil erosion and conservation in Ethiopia: a review progress in physical geography. Earth Environ. 39 (6), 750–774. doi:10.1177/0309133315598725
Haregeweyn, N., Tsunekawa, A., Poesen, J., Tsubo, M., Meshesha, D., Fenta, A., et al. (2017). Comprehensive assessment of soil erosion risk for better land use planning in river basins: case study of the Upper Blue Nile River. Sci. Total Environ. 574, 95–108. doi:10.1016/j.scitotenv.2016.09.019
Hellden, U. (1987). An assessment of woody biomass, community forests, land use and soil erosion in Ethiopia. Lund: Lund University Press.
Hossain, A., Krupnik, T. J., Timsina, J., Mahboob, M. G., Chaki, A. K., Farooq, M., et al. (2020). Agricultural land degradation: processes and problems undermining future food security. Environ. Clim. Plant Veg. Growth, 17–61. doi:10.1007/978-3-030-49732-3_2
Hurni, H. (1985). “Erosion-productivity-conservation systems in Ethiopia,” in Proceeding 4th international conference on soil conservation, Maracacy, Venezuela, 654–674.
Hurni, K., Zeleke, G., Kassie, M., Tegegne, B., Kassawmar, T., Teferi, E., et al. (2016). Soil degradation and sustainable land management in the rainfed agricultural areas of Ethiopia: an assessment of the economic implications. Econ. Land Degrad. (ELD) Ethiop. Case Study Rep. Econ. Land Degrad. Initiative.
Kanito, D. (2020). Participatory identification of major natural resource constraints and potentials under koka-lewate watershed, tembaro woreda, kambata tambaro zone of southern Ethiopia. Int. J. Energy Environ. Sci. 5 (1), 1–6. doi:10.11648/j.ijees.20200501.11
Kumar, M., Sahu, A. P., Sahoo, N., Dash, S. S., Raul, S. K., and Panigrahi, B. (2022). Global-scale application of the RUSLE model: a comprehensive review. Hydrological Sci. J. 67 (Issue 5), 806–830. doi:10.1080/02626667.2021.2020277
Liliwirianis, N., Isa, N. N. M., and Suratman, M. N. (2023). Land resources and its degradation in asia. Land Environ. Manag. through For., 23–45. doi:10.1002/9781119910527.ch2
Lin, D., Gao, Y., Wu, Y., Shi, P., Yang, H., and Wang, J. (2017). A conversion method to determine the regional vegetation cover factor from standard plots based on large sample theory and TM images: a case study in the eastern farming-pasture ecotone of northern China. Remote Sens. 9 (10), 1035. doi:10.3390/rs9101035
Lin, L., Di, L., Zhang, C., Guo, L., Zhao, H., Islam, D., et al. (2024). Modeling urban redevelopment: a novel approach using time-series remote sensing data and machine learning. Geogr. Environ. Sustain. 5 (2). doi:10.1016/j.geosus.2024.02.001
Mazengia, W., Gamiyo, D., Amede, T., Daka, M., and Mowo, J. (2007). Challenges of collective action in soil and water conservation: the case of Gununo watershed, southern Ethiopia. Afr. Crop Sci. Conf. Proc. 8 (January).
Mehwish, M., Nasir, M. J., Raziq, A., Al-Quraishi, A. M. F., and Ghaib, F. A. (2024). Soil erosion vulnerability and soil loss estimation for Siran River watershed, Pakistan: an integrated GIS and remote sensing approach. Environ. Monit. Assess. 196 (1), 104. doi:10.1007/s10661-023-12262-x
Meng, X., Zhu, Y., Yin, M., and Liu, D. (2021). The impact of land use and rainfall patterns on the soil loss of the hillslope. Sci. Rep. 11 (1), 16341. doi:10.1038/s41598-021-95819-5
Mengie, M. A., Hagos, Y. G., Malede, D. A., and Andualem, T. G. (2022). Assessment of soil loss rate using GIS–RUSLE interface in Tashat Watershed, Northwestern Ethiopia. J. Sediment. Environ. 7 (3), 617–631. doi:10.1007/s43217-022-00112-8
Moges, D. M., and Bhat, H. G. (2017). Integration of geospatial technologies with RUSLE for analysis of land use/cover change impact on soil erosion: case study in Rib watershed, north - western highland Ethiopia. Environ. Earth Sci. 76 (765), 765–814. doi:10.1007/s12665-017-7109-4
Mohammed, S., Alsafadi, K., Talukdar, S., Kiwan, S., Hennawi, S., Alshihabi, O., et al. (2020). Estimation of soil erosion risk in southern part of Syria by using RUSLE integrating geo informatics approach. Remote Sens. Appl. Soc. Environ. 20, 100375–100414. doi:10.1016/j.rsase.2020.100375
Moore, D., and Wilson, J. (1992). Length slope factor for the revised universal soil loss equation: simplifed method of solution. J. Soil Water Conserv. 47 (5), 423–428.
Panagos, P., Borrelli, P., Meusburger, K., van der Zanden, E. H., Poesen, J., and Alewell, C. (2015). Modelling the effect of support practices (P-factor) on the reduction of soil erosion by water at European scale. Environ. Sci. Policy 51, 23–34. doi:10.1016/j.envsci.2015.03.012
Rediet, G., and Eshetu, G. (2020). Spatial modeling of erosion hotspots using GIS-RUSLE interface in Omo-Gibe river basin, Southern Ethiopia: implication for soil and water conservation planning. Environ. Syst. Res. 9, 1–14. doi:10.1186/s40068-020-00180-7
Renard, K. G., Foster, G. R., Weesies, G. A., and Porter, J. P. (1997). RUSLE: revised universal soil loss equation. J. Soil Water Conserv. 46, 30–33.
Richi, S. M. (2025). Integrated RUSLE-GIS modeling for enhancing soil erosion management in Ghamima River Basin, Syria. DYSONA - Appl. Sci. 6 (1), 104–112. doi:10.30493/DAS.2024.479955
Sarkar, B., Rahman, A., Islam, A., Rahman, A., Haque, S. M., Talukdar, S., et al. (2024). Soil erosion and sediment yield estimation in a tropical monsoon dominated river basin using GIS-based models. Geocarto Int. 39 (1), 2309181. doi:10.1080/10106049.2024.2309181
Scatena, F. N. (1991). Soil erosion in the tropics: principles and management. Rattan lal. Q. Rev. Biol. 66 (4), 511. doi:10.1086/417401
SCRP (2000). Area of Gununo, sidamo, Ethiopia: long-term monitoring of the agricultural environment (1981-1994), soil conservation research project (SCRP), center for development and environment (CDE). Switzerland: University of Bern.
Setyawan, C., Lee, C. Y., and Prawitasari, M. (2019). Investigating spatial contribution of land use types and land slope classes on soil erosion distribution under tropical environment. Nat. Hazards 98 (2), 697–718. doi:10.1007/s11069-019-03725-x
Shiferaw, A., Hurni, H., and Zeleke, G. (2013). Long-term changes in soil-based ecological services at three sites in Ethiopia. J. Environ. Microbiol. 1 (1), 136–144. doi:10.5897/jene2013.0392
Sims, N. C., Newnham, G. J., England, J. R., Guerschman, J., Cox, S. J. D., Roxburgh, S. H., et al. (2021). Good practice guidance for sustainable development goal (SDG) indicator 15.3.1. Available online at: https://catalogue.unccd.int/1768_UNCCD_GPG_SDG-Indicator-15.3.1_version2_2021.pdf.
Sinshaw, B. G., Belete, A. M., Mekonen, B. M., Wubetu, T. G., Anley, T. L., Alamneh, W. D., et al. (2021). Watershed-based soil erosion and sediment yield modeling in the Rib watershed of the Upper Blue Nile Basin, Ethiopia. Energy Nexus 3, 100023. doi:10.1016/j.nexus.2021.100023
Sun, W., Shao, Q., Liu, J., and Zhai, J. (2014). Assessing the effects of land use and topography on soil erosion on the Loess Plateau in China. Catena 121, 151–163. doi:10.1016/j.catena.2014.05.009
Tegegne, M., and Biniam, S. (2017). Estimating soil erosion risk and evaluating erosion control measures for soil conservation planning at Koga watershed in the highlands of Ethiopia. Solid earth. 8, 13–25. doi:10.5194/se-8-13-2017
Teku, D., Kesete, N., and Abebe, A. (2024). GIS based annual soil loss estimation with revised universal soil loss equation (RUSLE) in the upper Meki sub-catchment, rift valley sub-basin, Ethiopia. Cogent Food Agric. 10 (1). doi:10.1080/23311932.2024.2311802
Tiruneh, G., and Ayalew, M. (2015). Soil loss estimation using geographic information system in enfraz watershed for soil conservation planning in highlands of Ethiopia. Int. J. Agric. Res. Innov. Technol. 5 (2), 21–30. doi:10.3329/ijarit.v5i2.26265
Unger, P. W. (2023). Common soil and water conservation practices. Soil Eros. Conservation, Rehabilitation, 239–266. doi:10.1201/9781003418177-11
Wassie, S. B. (2020). Natural resource degradation tendencies in Ethiopia: a review. Environ. Syst. Res. 9 (Issue 1), 33. doi:10.1186/s40068-020-00194-1
Weslati, O., and Serbaji, M. M. (2024). Spatial assessment of soil erosion by water using RUSLE model, remote sensing and GIS: a case study of Mellegue Watershed, Algeria–Tunisia. Environ. Monit. Assess. 196 (1), 14. doi:10.1007/s10661-023-12163-z
Wischmeier, W. H., and Smith, D. D. (1978a). Predicting rainfall erosion losses a: guide to conservation planning. Agriculture handbook, 282. USA: USDA-ARS.
Wischmeier, W. H., and Smith, D. D. (1978b). “Predicting soil erosion losses: a guide to conservation planning,” in USDA agricultural handbook, 537.
Wolde, B., Moges, A., and Grima, R. (2023). Assessment of the combined effects of land use/land cover and climate change on soil erosion in the Sile watershed, Ethiopian Rift Valley Lakes Basin. Cogent Food Agric. 9 (2). doi:10.1080/23311932.2023.2273630
Woldemariam, G., Iguala, A., Tekalign, S., and Reddy, R. (2018). Spatial modeling of soil erosion risk and its implication for conservation planning: the case of the Gobele watershed, east hararghe zone, Ethiopia. Land 7 (1), 25. doi:10.3390/land7010025
Yadeta, S. K., Nega, T. E., Berhanu, G. S., and Atinkut, H. B. (2021). Modeling soil erosion using RUSLE and GIS at watershed level in the upper beles, Ethiopia. Environ. Challenges 2, 1–10. doi:10.1016/j.envc.2020.100009
Yesuph, A. Y., and Dagnew, A. B. (2019). Soil erosion mapping and severity analysis based on RUSLE model and local perception in the Beshillo Catchment of the Blue Nile Basin, Ethiopia. Environ. Syst. Res. 8, 17–21. doi:10.1186/s40068-019-0145-1
Keywords: RUSLE, SDGs, Omo-Gibe basin, erosion modelling, subwatershed prioritization, soil loss
Citation: Kanito D, Bedadi B, Feyissa S, Kabo-Bah AT and Kaka S (2025) Spatial assessment of soil erosion for prioritizing efforts on SDG15.3 in Ethiopia. Front. Remote Sens. 6:1550331. doi: 10.3389/frsen.2025.1550331
Received: 23 December 2024; Accepted: 25 February 2025;
Published: 13 March 2025.
Edited by:
Kwanele Phinzi, University of Zululand, South AfricaReviewed by:
Ernieza Suhana Mokhtar, Centre of Studies for Surveying Science and Geomatics, MalaysiaCopyright © 2025 Kanito, Bedadi, Feyissa, Kabo-Bah and Kaka. This is an open-access article distributed under the terms of the Creative Commons Attribution License (CC BY). The use, distribution or reproduction in other forums is permitted, provided the original author(s) and the copyright owner(s) are credited and that the original publication in this journal is cited, in accordance with accepted academic practice. No use, distribution or reproduction is permitted which does not comply with these terms.
*Correspondence: Dawit Kanito, ZzIwMjM5MjQ5MEBrZnVwbS5lZHUuc2E=
†ORCID: Dawit Kanito, orcid.org/0000-0002-4030-9331; Bobe Bedadi, orcid.org/0000-0003-2304-8827; Samuel Feyissa, orcid.org/0000-0002-9041-2919; SanLinn Kaka, orcid.org/0000-0003-1229-4627
Disclaimer: All claims expressed in this article are solely those of the authors and do not necessarily represent those of their affiliated organizations, or those of the publisher, the editors and the reviewers. Any product that may be evaluated in this article or claim that may be made by its manufacturer is not guaranteed or endorsed by the publisher.
Research integrity at Frontiers
Learn more about the work of our research integrity team to safeguard the quality of each article we publish.