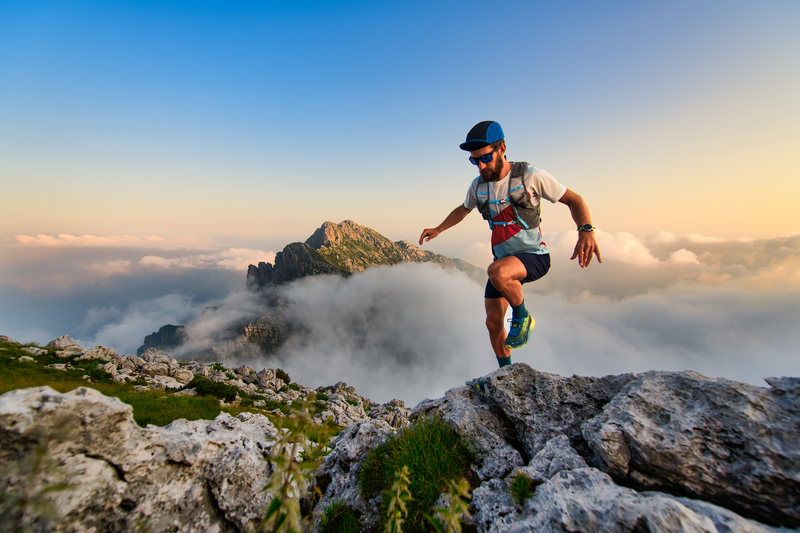
94% of researchers rate our articles as excellent or good
Learn more about the work of our research integrity team to safeguard the quality of each article we publish.
Find out more
REVIEW article
Front. Remote Sens.
Sec. Terrestrial Carbon Cycle
Volume 6 - 2025 | doi: 10.3389/frsen.2025.1532280
This article is part of the Research Topic One Forest Vision Initiative (OFVi) for Monitoring Tropical Forests: The Remote Sensing Pilar View all 4 articles
The final, formatted version of the article will be published soon.
You have multiple emails registered with Frontiers:
Please enter your email address:
If you already have an account, please login
You don't have a Frontiers account ? You can register here
African tropical forests play a crucial role in global carbon dynamics, biodiversity conservation, and climate regulation, yet monitoring their structure, diversity, carbon stocks and changes remains challenging. Remote sensing techniques, including multi-spectral data, lidar-based canopy height and vertical structure detection, and radar interferometry, have significantly improved our ability to map forest composition, estimate height and biomass, and detect degradation and deforestation features at a finer scale. Machine learning approaches further enhance these capabilities by integrating multiple data sources to produce improved maps of forest attributes and track changes over time. Despite these advancements, uncertainties remain due to limited ground-truth validation, and the structural complexity and large spatial heterogeneity of African forests. Future developments in remote sensing should examine how multi-sensor integration of high-resolution data from instruments such as Planet, Tandem-X, SPOT and improved AI methods can refine forest composition, carbon storage and function maps, enhance large-scale monitoring of tree height and biomass dynamics, and improve forest degradation and deforestation detection down to tree level. These advancements will be essential for supporting science-based decision-making in forest conservation and climate mitigation.
Keywords: Remote sensing-, deep learning, Congo basin, Carbon, biomass, Canopy height, Forest typology, Forest degradation
Received: 21 Nov 2024; Accepted: 24 Feb 2025.
Copyright: © 2025 Bossy, Ciais, Renaudineau, Wan, Ygorra, Adam, Barbier, Bauters, Delbart, Frappart, Gara, Hamunyela, Averti, Jaffrain, Maisongrande, Mugabowindekwe, Mugiraneza, Normandin, Obame, Peaucelle, Pinet, Ploton, Sagang, Schwartz, Sollier, Sonké, Tresson, De Truchis, Vo Quang and Wigneron. This is an open-access article distributed under the terms of the Creative Commons Attribution License (CC BY). The use, distribution or reproduction in other forums is permitted, provided the original author(s) or licensor are credited and that the original publication in this journal is cited, in accordance with accepted academic practice. No use, distribution or reproduction is permitted which does not comply with these terms.
* Correspondence:
Thomas Bossy, Université de Versailles Saint-Quentin-en-Yvelines, Versailles, France
Disclaimer: All claims expressed in this article are solely those of the authors and do not necessarily represent those of their affiliated organizations, or those of the publisher, the editors and the reviewers. Any product that may be evaluated in this article or claim that may be made by its manufacturer is not guaranteed or endorsed by the publisher.
Research integrity at Frontiers
Learn more about the work of our research integrity team to safeguard the quality of each article we publish.