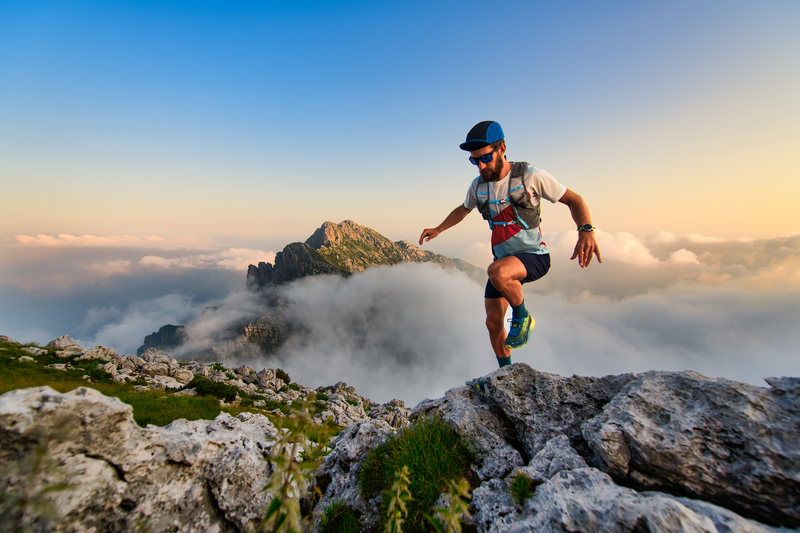
94% of researchers rate our articles as excellent or good
Learn more about the work of our research integrity team to safeguard the quality of each article we publish.
Find out more
ORIGINAL RESEARCH article
Front. Remote Sens.
Sec. Image Analysis and Classification
Volume 6 - 2025 | doi: 10.3389/frsen.2025.1468918
The final, formatted version of the article will be published soon.
You have multiple emails registered with Frontiers:
Please enter your email address:
If you already have an account, please login
You don't have a Frontiers account ? You can register here
Monitoring coastlines is a significant challenge in understanding their evolution, temporal and spatial variability, and impact on local marine ecosystems. Coastal anthropization, or the influence of waves and currents, can significantly alter the coastal landscape and morphology, presenting a daunting task. Severe consequences have been demonstrated, leading to the import of sand to eroding coasts and altering living conditions for marine ecosystems. Effectively managing these changes is essential, especially in countries like the United Arab Emirates (UAE), for their rich coastal biodiversity. The focus on the UAE is crucial to highlight the anthropic and natural development of its coastal cities through time. Several studies have extracted coastlines or measured erosion and accretion rates to track these changes, typically employing satellite imagery. Yet, the coastline of arid areas is not often monitored, raising concerns about the anthropic development of coastal cities and the preservation of their biodiversity on the long term. To address this gap, an algorithm was developed to automatically extract the coastline from satellite imagery using the Direct Difference Water Index (DDWI), an index that recently showed its efficiency in coastline extraction, the Otsu threshold, a fill operator and the Canny edge detector. The results reveal a significant evolution, along over 770 km of coastline, highlighting substantial anthropic development prior to 2013 associated with urban expansion into the sea. Sandbanks also exhibited a surprising increase in surface area around cities. Overall, excluding anthropic development, the UAE’s coast has remained relatively stable, with minor erosion observed in the west of the country, potentially attributed due to natural processes. This trend became more apparent after 2013, indicating a correlation between the maintenance of coastline stability and reduced urban development. This study offers an analysis of the coastline dynamics, that contributes to a deeper understanding of the effects of anthropisation on the unique coastal ecosystems of the UAE. It also provides insights for effective coastal management and urban planning.
Keywords: coastlines, Marine Ecosystems, Coastal urban development, Coastal morphology, Sandbanks
Received: 22 Jul 2024; Accepted: 04 Mar 2025.
Copyright: © 2025 Sarrau, Al Abdouli and Abuelgasim. This is an open-access article distributed under the terms of the Creative Commons Attribution License (CC BY). The use, distribution or reproduction in other forums is permitted, provided the original author(s) or licensor are credited and that the original publication in this journal is cited, in accordance with accepted academic practice. No use, distribution or reproduction is permitted which does not comply with these terms.
* Correspondence:
Justine Sarrau, United Arab Emirates University, Al-Ain, United Arab Emirates
Disclaimer: All claims expressed in this article are solely those of the authors and do not necessarily represent those of their affiliated organizations, or those of the publisher, the editors and the reviewers. Any product that may be evaluated in this article or claim that may be made by its manufacturer is not guaranteed or endorsed by the publisher.
Research integrity at Frontiers
Learn more about the work of our research integrity team to safeguard the quality of each article we publish.