- Department of Environmental Science, Faculty of Science and Technology, University of Latvia, Riga, Latvia
This study investigates land use and land cover changes in the Teiči Strict Nature Reserve, Latvia, from 1982 to 2023 and models potential future changes based on four legislative scenarios extending to 2064. The research aims to assess the effectiveness of existing conservation zoning in relation to historical and projected changes in land use and land cover, addressing the ongoing debate between fixed and adaptable conservation strategies. The study employs remote sensing imagery, geographical information system data, and land use and land cover modeling methods to analyze historical changes and predict future trends. The results suggest substantial land use and land cover changes over the past four decades, including intensified urbanization, agricultural expansion, and a decline in peat bogs and forested areas. Scenario projections indicate that continued land use and land cover changes could further challenge the efficiency of current conservation strategies, with varying outcomes depending on legislative measures and climate change impacts. The study concludes that adaptive management and variable conservation zoning are necessary to address these dynamic changes and preserve the reserve’s ecological integrity. The results emphasize the importance of integrating predictive modeling into conservation planning to improve flexibility and sustainability in protected areas.
1 Introduction
A protected area is a region that is officially recognized, designated, and managed through a series of legal or other effective means to ensure the long-term preservation of the natural environment (Stojanović et al., 2024). Strict nature reserves, category Ia of the International Union for Conservation of Nature classification, are a specific category of protected areas that are subjected to especially stringent protection measures to preserve their conservation values (Mierauskas, 2004; Zhang et al., 2023). The primary difference between a “regular” nature reserve and a strict nature reserve lies in the level of permitted human activity and regulation. The primary goal of a strict nature reserve is to ensure that natural processes happen without interference, thus restricting human activities. At the same time, “regular” nature reserves allow for some level of human intervention (Mierauskas, 2004). While both types of nature reserves are invaluable environments, strict nature reserves are imperative for maintaining intact ecosystems and natural processes, which is essential for long-term biodiversity preservation (Stolton et al., 2013).
Conservation zoning is a crucial part of strict protection measures aimed at sustaining the preservation values of nature reserves (Hu N. et al., 2024; Lamounier et al., 2024). By applying zones, nature reserves can efficiently manage different environmental areas based on their ecological significance and conservation needs. However, land use and land cover (LULC) changes present substantial challenges for conservation zoning. For instance, habitat loss and fragmentation can undermine the efficiency of conservation zoning by reducing the area available for core zones (e.g., strict regime zone, regulatory regime zone) and disordering ecological connectivity between different zones (Chen et al., 2023a; Chen et al., 2023b; Vieira-Alencar et al., 2023). Furthermore, changed ecosystem functions can affect the ecological integrity of conservation zones, requiring adjustments in zoning and management strategies to address new ecological conditions (Rohwer and Marris, 2021; Karr et al., 2022). Moreover, increased human pressure can result in encroachment into buffer or core zones, increased pollution, and disruptions that challenge the existing conservation measures (Cheng et al., 2023; Legutko-Kobus et al., 2023). Besides, biodiversity shifts may demand modifications in zoning to accommodate new or displaced species or to address changed ecological interactions (Schlaepfer and Lawler, 2023; Chauvier-Mendes et al., 2024). Hence, systematic updates and changes to zoning plans may be required to respond to new challenges and maintain conservation objectives. In addition, LULC changes frequently intersect with climate change, intensifying challenges for conservation zoning. For example, deforestation can aggravate climate change effects, such as changed precipitation patterns, which in turn affect conservation strategies (Adam et al., 2024). To address these challenges, conservation zoning must be adaptive and feature strategies for monitoring and responding to LULC changes.
Modeling plays a crucial role in addressing the challenges posed by changes in LULC for conservation zoning in nature reserves. For instance, LULC modeling can predict future changes in land cover based on current trends and drivers (Dietz et al., 2023; Fogang et al., 2023; Zarandian et al., 2023). Moreover, by modeling different land use scenarios, LULC models help evaluate the potential outcomes of various development or conservation strategies (Zarandian et al., 2023; Hu Y. et al., 2024). Furthermore, LULC models can assess the potential impacts of changes on biodiversity, habitat fragmentation, and ecosystem services (Biswas et al., 2023; Boussema et al., 2023; Lahon et al., 2023; Munthali et al., 2023). This data is vital for adjusting zoning plans to protect key habitats and maintain ecological connectivity. Lastly, LULC models can integrate climate change projections, which is essential for designing adaptive management strategies that consider both land use and climate factors (Salaudeen et al., 2023).
Recent technological advances have substantially improved conservation monitoring and planning through innovations in LULC modeling. For example, remote sensing provides detailed, real-time imagery of LULC changes (Mustaquim, 2024; Mohiuddin and Mund, 2024). When combined with geographical information systems (GIS), it allows for detailed mapping and monitoring of conservation zones (Marzialetti et al., 2024; Pham et al., 2024; Zhao et al., 2024). This integration aids in detecting changes and updating zoning plans based on current data. Furthermore, GIS enables assessing the impact of LULC changes on habitat fragmentation and ecological connectivity, which in turn supports effective zoning by visualizing how different land use patterns affect conservation priorities. At the same time, machine learning algorithms and artificial intelligence (AI) methods can improve the capacity to predict potential impacts on conservation zones and develop adaptive strategies as; by using these methods, it is possible to analyze large amounts of historical and real-time LULC data to predict future land use scenarios with high accuracy (Acuña-Alonso et al., 2024; Pande et al., 2024; Yimer et al., 2024). Moreover, the integration of real-time data from remote sensing and GIS with predictive models allows for adaptive management strategies; thus, conservation zoning can be updated dynamically in response to the latest information and changing environmental conditions. Essentially, the convergence of these technological developments adds to LULC modeling by providing high-resolution data, improving predictive capabilities, and enabling adaptive management. This incorporation can ensure that conservation zoning is more accurate, responsive, and capable of addressing the challenges posed by LULC changes efficiently.
However, the advancements in technology intersect significantly with the ongoing debate about static versus dynamic conservation strategies and the effectiveness of different approaches (Margules and Pressey, 2000; Hannah et al., 2002; Bengtsson et al., 2003; Lee et al., 2007; Rayfield et al., 2008; Garden et al., 2015; Bengtsson et al., 2021). For instance, while static zones may provide clear boundaries and are simpler to manage, they may become outdated as LULC and environmental conditions change. While remote sensing and GIS advancements can help monitor these zones more effectively, the fundamental inflexibility of static zoning cannot adapt well to rapid changes. At the same time, dynamic zones can be adjusted based on real-time data and predictive models. Machine learning and AI enhance the ability to develop and manage dynamic zones by providing updated predictions and scenario analyses (Pande et al., 2024; Yimer et al., 2024). These tools aid in creating responsive management strategies that can adapt to the latest information and changing conditions. In addition, debate also surrounds the integration of socio-economic factors into conservation planning, with some arguing that local community involvement and economic incentives enhance effectiveness, while others caution that these may compromise conservation goals (Pretty and Smith, 2004; Wunder, 2007; Redford and Adams, 2009). Nonetheless, advanced technologies enable the integration of socio-economic data with ecological data. GIS and LULC modeling can map socio-economic factors alongside conservation needs, aiding in the design of strategies that align with both community interests and conservation goals. For instance, machine learning and AI can assist in finding an equilibrium between socio-economic development and conservation objectives by modeling how different scenarios affect both aspects. Nevertheless, it should be noted that different LULC models can produce variable conclusions about the efficiency of conservation zones. Some models might support broad, landscape-scale measures due to their ability to capture large-scale trends, while others might highlight the need for targeted interventions based on individual high-value areas.
This study aims to address a critical but underexplored aspect of conservation management: the effectiveness of internal zoning within protected areas in achieving biodiversity conservation goals. While much research has evaluated whether protected areas as a whole fulfill their conservation missions, fewer studies have specifically assessed whether the zoning structures within protected areas adequately support these objectives. In this study, we examine the Teiči Strict Nature Reserve as a case study, from its foundation in 1982 until today (2023) and model future development under four legislative scenarios up to 2064, to analyze how different management zones—each with distinct regulations and conservation intentions—respond to LULC changes. By evaluating the alignment between regulatory frameworks and observed and projected LULC dynamics, this study provides insights into the role of zoning as a targeted conservation strategy within protected areas.
Preliminary findings suggest that the effectiveness of the existing conservation zoning in Teiči Strict Nature Reserve may be compromised without proactive adjustments. Historical analysis reveals increased external pressures on the reserve, while future scenarios indicate significant LULC changes. These results emphasize the need for revising current conservation zoning to address anticipated challenges efficiently.
2 Materials and methods
2.1 Data collection and preprocessing
The first phase of the research involved defining the case study area with clearly specified boundaries. The case study area covers the Teiči Strict Nature Reserve and its surrounding buffer zone for a total of 58,795.0 ha. Geographically (Figure 1), it is in eastern Latvia, centered around 56°38′59.4″N and 26°27′24.5″E, with spatial extent defined using the WGS 84/UTM zone 35N (EPSG: 32,635) coordinate system (EPSG.io, 2024). Three distinct conservation zones divide the reserve: the strict regime zone (5,067.3 ha), the nature park zone (114.1 ha), and the regulated regime zone (14,579.8 ha). The remaining 24,041.9 ha determine the buffer zone (Lāce and Krauklis, 2021; Legal Acts of the Republic of Latvia, 2024; Nature Conservation Agency, 2024a; Nature Conservation Agency, 2024b). The Teiči Strict Nature Reserve is the only strict nature reserve in Latvia and a prime example of an intact wetland ecosystem in Northern Europe. It covers a large area of wetland and forested areas.
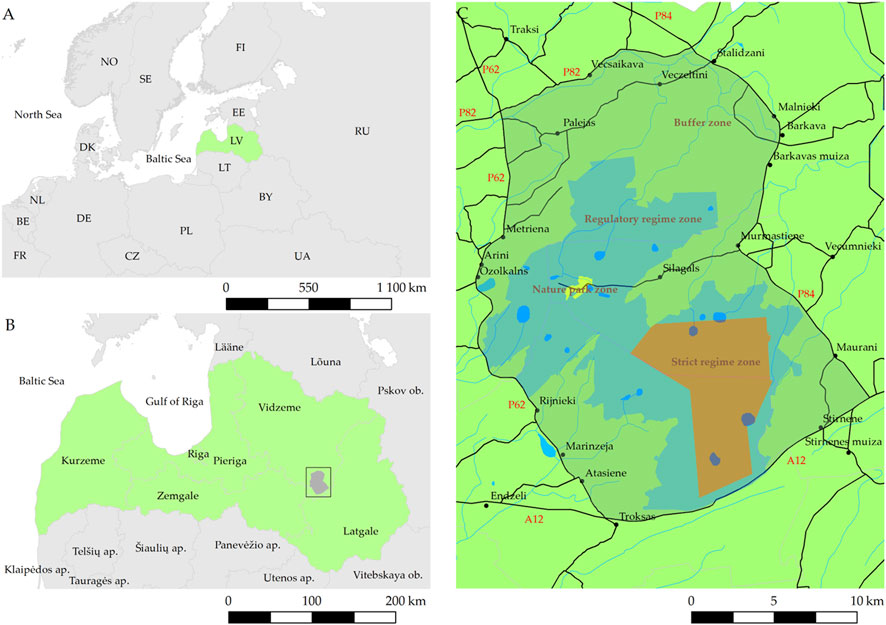
Figure 1. Geographical context of the case study area. (A) Position within Europe; (B) Specific location within Latvia; (C) Detailed local setting of the study area.
This study relies on multiple remote sensing datasets and supplementary in-house survey data to analyze LULC changes in the Teiči Strict Nature Reserve over time. Hence, the second phase entailed collecting remote sensing imagery of the designated study area for the years 1982, 1991, and 2023. Remote sensing imagery was obtained from the database of the United States Geological Survey (data collected by the Landsat series of Earth-observing satellites) (United States Geological Survey, 2024). Primary data sources included Landsat 5, Landsat 8, and Sentinel-2 satellite imagery, chosen for their temporal coverage and spatial resolution suitable for landscape-scale analysis. Landsat 5 provided data for earlier years, while Landsat 8 and Sentinel-2 covered the 2023 analysis with enhanced spectral and spatial accuracy. Landsat imagery was obtained at a 30-m resolution, with red, green, blue, and near-infrared bands. Sentinel-2 imagery, at a higher resolution of 10 m for primary bands and 20 m for additional spectral bands, allowed for greater detail and comparison. Key spectral indices, the normalized difference vegetation index and normalized difference built-up index, were calculated from Sentinel-2 data to support distinguishing LULC classes. To ensure data consistency across datasets, comprehensive pre-processing was applied. Atmospheric correction was conducted using the dark object subtraction method for Sentinel-2 and ATCOR for Landsat imagery to minimize atmospheric disturbances. Geometric corrections aligned the imagery to the Universal Transverse Mercator (UTM) coordinate system, Zone 35N, based on the WGS 84 datum. Radiometric calibration normalized pixel values for inter-comparison, while all imagery was resampled to a common 30-m resolution to harmonize the data, allowing older Landsat data to match newer Sentinel-2 data for consistency. Additional ancillary data, such as the data on elevation, the water catchment area, the distribution of roads, population density, and soil types, were acquired from the GIS Latvia 10.2 database (Envirotech ltd., 2024). Supplementary data regarding policies and zoning of the case study area were obtained from the Nature Conservation Agency and the Legal Acts of the Republic of Latvia (Nature Conservation Agency, 2024b; Legal Acts of the Republic of Latvia, 2024). Meanwhile, as the climatic variable for future projections, the Representative Concentration Pathway (RCP) 4.5 climate change scenario (temperature, precipitation, and vegetation season) was obtained from the database of the Potsdam Institute for Climate Impact Research (Smith and Wigley, 2006; Potsdam Institute, 2024).
During the third phase, remote sensing imagery was transformed into false-color composites (Humboldt State University, 2024). The procedure was accomplished by merging a sequence of Landsat sensor spectral bands (United States Geological Survey, 2024). For the year 1982, bands 4 (0.5–0.6 µm), 5 (0.6–0.7 µm), and 6 (0.7–0.8 µm) were used to create the false color composite. For 1991, bands 2 (0.52–0.60 µm), 3 (0.63–0.69 µm), and 4 (0.76–0.90 µm) were employed, while for 2023, bands 3 (0.53–0.59 µm), 4 (0.64–0.67 µm), and 5 (0.85–0.88 µm) were utilized to generate the composite. In addition, during the third stage, the elevation data (Envirotech ltd., 2024) were used to generate a digital elevation model (Mamajonova et al., 2024). Subsequently, the digital elevation model was then used to calculate slope, aspect, and hillshade (SunCalc.org., 2024) (spring equinox, noon, altitude 33.76°, azimuth 173.6°), which were then used as additional key variables for modeling. False-color composites, the digital elevation model, and derived key variables were generated using ArcMap 10.8.2.
In the fourth phase, false-color composites were used to create training samples for LULC classification (Bharathi et al., 2021; Shetty et al., 2021). At first, areas in each false-color composite that clearly represent all LULC classes were visually identified. Next, around the identified representative areas, at least 15 polygons were digitized.
During the fifth and final phase, the training samples were utilized as input for a supervised classification algorithm (maximum likelihood classification) to generate complete LULC maps for the defined study area (Polat and Kaya, 2021; Ganesh et al., 2023; Chowdhury, 2024). The LULC classification scheme followed a standardized structure, categorizing land cover types. This classification scheme aligns with the European CORINE Land Cover framework but was customized to reflect the specific characteristics of the study area. An object-based image analysis approach was applied, using spectral indices and spatial attributes within the eCognition software. Supervised classification algorithms, trained with sample points from field surveys in 2023 and verified with high-resolution aerial imagery, enabled accurate identification of each LULC category. Model training used 70% of labeled data, with the remaining 30% reserved for validation. Cross-referencing with historical records and in-field verification resulted in classification accuracy exceeding 85% across intervals.
For supervised classification, training sets were selected to represent the primary LULC classes in the study area, including forest, agriculture, urban (discontinuous urban fabric), water bodies, peat bogs, and transitional woodland-shrub. Training samples were chosen based on ground truth data from high-resolution aerial imagery to ensure accurate representation of each LULC class. A total of 100 training samples per LULC category were selected across all LULC classes (1,100 in total), with 70 of these samples used for training and 30 for validation.
Each defined LULC class was assigned an identification number in accordance with the Coordination of Information on the Environment land cover (CORINE) nomenclature (Copernicus, 2024). Generated LULC maps were cross verified with orthophotography of the case study area (The Latvian Geospatial Information Agency, 2024). To ensure comparability, as the final stage, the LULC maps and key variable data were harmonized for subsequent application in LULC change analysis and modeling (INSPIRE Knowledge Base, 2024).
2.2 Land use and land cover change analysis
The acquired and preprocessed data were subsequently utilized to examine the changes in LULC between the years 1982, 1991, and 2023.
Data processing involved multiple software tools, including ArcGIS Pro, eCognition Developer, Terrset. Pre-processed imagery was analyzed in ArcGIS Pro, while eCognition facilitated classification and feature extraction through its multi-resolution segmentation capabilities. Normalization techniques accounted for sensor variability and ensured that LULC classes were comparable across time periods, allowing for consistent LULC change analysis. For future projections (2023–2064), Markov Chain analysis modeled transitions across four scenarios: baseline, conservation-focused, agriculture-prioritized, and balanced scenarios, reflecting varying policy and environmental conditions.
In the first phase, the LULC map from 1982 and the LULC map from 1991 were used as input parameters for the land change modeler (LCM) session (Eastman and Toledano, 2018; Qacami et al., 2023). In addition, the road layer and digital elevation model variables were also included. The following execution of the run command resulted in the gathering of both gains and losses for each LULC category between 1982 and 1991. It also provided information on the overall change in each LULC category and the factors (other LULC classes) contributing to this change. The command was repeated to collect data that covered the period from 1991 to 2023. The LCM session was conducted using TerrSet 2000 version 19.0.8 (Labs, 2024).
The next phase comprised creating LULC change maps, which represented changes across two specific periods (1982–1991, 1991–2023). This included examining the persistence of LULC maps, identifying gains and losses in all LULC categories, LULC swap change and total change, documenting transitions between different LULC categories, and establishing exchanges between various LULC categories.
During the third phase, the spatial pattern of transition from one LULC category to another was generated.
As the final phase, to address LULC fragmentation over time, LULC patch analysis was performed, including defining the type of LULC changes (e.g., aggregation, dissection), quantifying the number of LULC patches over time, and determining their compactness ratio. The analysis was conducted using habitat and biodiversity modeler (HBM) (Ansari, 2019).
2.3 Designing future land use and land cover change scenarios
To extrapolate potential future development trajectories of the case study area, four distinct scenario models were designed, based on changes in legislative measures (e.g., current, less stringent, more stringent) and climate change projections following the RCP 4.5 for 2011–2040 and 2041–2070 (Wise et al., 2009; Thomson et al., 2011; Climate Portal, 2024; Potsdam Institute, 2024). Climate data for the RCP 4.5 scenario was derived from CMIP5 projections, downscaled for the Baltic region through the EURO-CORDEX initiative. The RCA4 model, paired with the MPI-ESM global climate model, provided downscaled temperature and precipitation data at a spatial resolution of 12.5 km.
Scenario 1 (the base scenario) was designed to depict the potential for LULC transitions between 2023 and 2064 if the existing policies are maintained under the RCP 4.5 climate change scenario. The transition rules in this business-as-usual scenario reflect minimal intervention and continuity with existing land use trends and policies. Urban to agriculture transitions remain at a low probability, indicating stable urban areas that are unlikely to revert to agricultural use under current policies. However, agriculture to urban transitions is assigned to a higher probability near roads and urban centers, reflecting development pressures that may drive urban expansion. Forest and natural land cover transitions to agriculture have a moderate probability in the buffer zone, assuming limited policy restrictions that allow agricultural expansion, particularly in areas not immediately adjacent to core conservation zones. Additionally, a higher probability is set for forest transitioning to transitional woodland-shrub in minimally managed areas, supporting natural succession in line with existing land management practices. These rules together illustrate a business-as-usual approach, prioritizing development in certain areas while allowing natural processes to continue with minimal policy-driven adjustments.
Scenario 2 was constructed to describe the potential for LULC transitions under stricter legislative measures for nature conservation. This scenario restricts major agricultural expansion within the buffer zone of the case study area while following the RCP 4.5 climate change scenario. The transition rules in this scenario reflect a conservation-focused approach, particularly within buffer and core zones. An increased probability is set for agriculture transitioning to natural land cover, especially within buffer areas, aligning with stricter conservation policies that promote reversion to natural cover or forest. Transitions from urban to forest or natural land cover have a higher probability in non-core urban areas, potentially facilitating natural landscape restoration or green infrastructure projects within the buffer zone. Forest to transitional woodland-shrub transitions are permitted but have a low probability, prioritizing forest retention and minimizing fragmentation. Additionally, the transition probabilities for both agricultural and urban expansion are significantly reduced in buffer and core conservation zones, aiming to prevent encroachment on protected areas and uphold conservation objectives.
Scenario 3 was considered to project the potential for LULC changes under a policy regime that prioritizes the expansion of agricultural activities within the buffer zone of the case study area under the RCP 4.5 climate change scenario. The transition rules in this case emphasize agricultural expansion within the buffer zone, with a high probability set for transitions from natural land to agriculture, prioritizing agricultural growth over other land covers. Forest to agriculture transitions also have an increased probability within buffer zone, allowing agriculture to extend into previously undeveloped or minimally managed lands. A moderate probability is assigned for agriculture transitioning to urban areas near roads, facilitating potential urban expansion into nearby agricultural land, though the primary focus remains on increasing agricultural land cover. Natural succession, such as forest transitioning to transitional woodland-shrub, has a limited probability, as the emphasis on agricultural expansion discourages reversion to more natural land cover types.
Finally, scenario 4 was proposed to outline the potential for LULC change between 2023 and 2064 under a policy regime that seeks a balance between land use priorities while still following the RCP 4.5 climate change scenario. Only environmentally sustainable agriculture practices, such as agroforestry, were permitted in this instance. The transition rules were defined to prioritize sustainable land management and resilience, especially within conservation zones and the buffer area. A high probability was assigned for agriculture to transition into agroforestry or mixed land use, encouraging sustainable practices within the buffer zone to balance conservation with productive land use. To control urban expansion, the likelihood of agricultural land transitioning to urban areas was kept low, except near established urban centers, aiming to reduce urban sprawl and support landscape stability. For forested areas, a moderate probability was set for transition to transitional woodland-shrub, allowing for limited natural succession and reflecting an adaptive approach to fostering ecological resilience within conservation areas. Additionally, high stability was ensured for forested and natural areas by setting low probabilities for transitions to other land covers, thus protecting core conservation values while supporting sustainable practices in surrounding areas.
2.4 Future land use and land cover change modeling
The prediction was made in the LCM environment. In addition to satellite imagery, vector data were incorporated to refine the modeling process in the LCM environment. These vector layers included roads, hydrological networks, administrative boundaries, and existing protected area boundaries. This vector data was essential for defining transition probabilities and spatial relationships, such as zones of influence around infrastructure, which influence land cover transitions. For example, proximity to road networks and urban centers increased the likelihood of transitions to urban land cover, while proximity to conservation areas lowered the probability of such transitions, helping to preserve natural habitats. Vector data also supported the creation of transition rules that allowed the model to differentiate areas with varying probabilities of LULC change, based on both physical proximity and policy-driven constraints. This approach ensured that transitions adhered to logical land cover patterns observed in the study area, creating realistic predictive scenarios.
The LULC predictions for 2064 were based on transition rules and statistical models embedded in the LCM. Transition rules were formulated based on observed historical trends in LULC and spatial dependencies in the study area. For instance, areas adjacent to agricultural zones had a higher likelihood of transitioning to agriculture under scenarios prioritizing rural development, while areas close to core conservation zones were less likely to experience such transitions due to protective policies. This differentiation provided a nuanced understanding of potential LULC dynamics.
This study employed a combination of Logistic Regression, Markov Chain Analysis, and Multi-Layer Perceptron (MLP) Neural Networks within the LCM in TerrSet to predict future LULC changes.
The potential for transition was evaluated using Cellular Automata (CA)-Markov models (Tattoni et al., 2011; Rahnama, 2021; Kumar and Agrawal, 2023). Transition sub-models were subsequently generated for all plausible transitions among LULC categories, using evidence likelihood as the transformation type (Mondal et al., 2016; Shawul and Chakma, 2019). The present LULC (2023), road network, climatic variables, and settlements were defined as dynamic variables in the base scenario, while topographical variables were considered static. The transition probability matrix was altered to set the probability of certain LULC categories expanding to zero for the remaining scenarios. More specifically, the probability of the expansion of agriculture-related LULC categories was set to zero in scenario 2. The probability of the expansion of natural LULC categories into agriculture-related LULC categories was set to zero for scenario 3. Finally, the probability of the expansion of complex cultivation patterns was set to zero for scenario 4. Then each transition sub-model was run using a multi-layer perceptron classifier using learning rate decay (0.01 for the start learning rate and 0.001 for the end learning rate), a momentum factor of 0.5, and the standard logistic sigmoid function (α = 1.0) (Qacami et al., 2023; Labs, 2024). As a result, transition potential maps were generated for all four scenarios, which were then used to create maps for projected land cover in 2064 under scenarios 1–4 as a function within the LCM modeler. Finally, the LULC maps generated for the year 2064 were analyzed in accordance with the LULC methodology outlined in Section 2.2.
3 Results
3.1 Historical land use and land cover change
The study area shows a heterogeneous LULC composition (Figure 2), containing 11 distinct categories that show spatiotemporal variations in their distribution. The study reveals clear trends across different land cover types, which highlight both areas of stability and areas undergoing transformation. Each designated conservation zone (Legal Acts of the Republic of Latvia, 2024) exhibits a considerable degree of LULC diversity that changes over time, although the magnitude of this change varies between zones (Figures 2A–C). Essentially, the LULC categories within the case study site are characterized by a fragmented spatial distribution, forming a mosaic of land patches with variable dimensions and spatial configurations. For instance, forested areas within the core conservation zones exhibit high persistence, particularly in the strict and regulatory regime zones. This stability aligns with the protective regulations in these zones, underscoring the effectiveness of conservation policies in maintaining forest cover despite surrounding anthropogenic pressures.
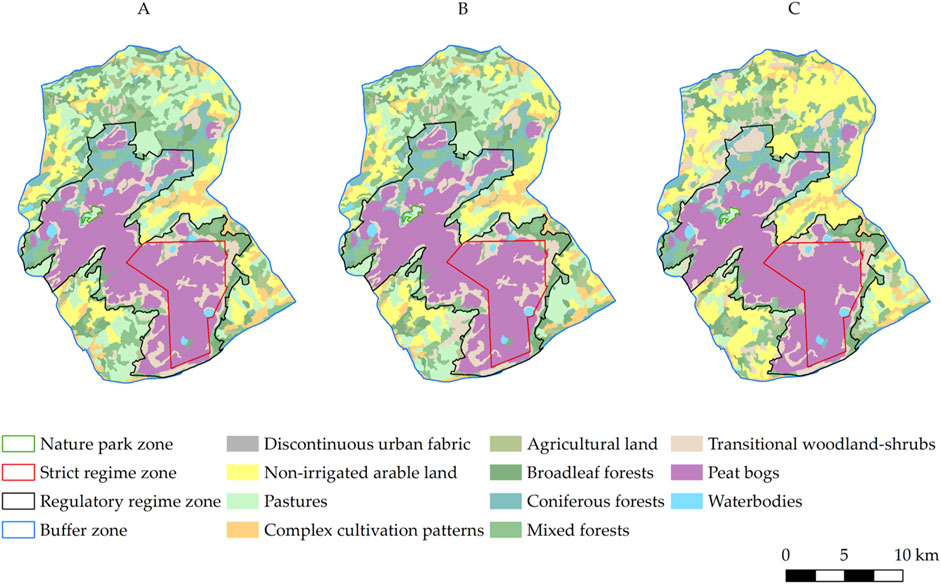
Figure 2. Digitized land use and land cover maps of the case study area for the years (A) 1982, (B) 1991, and (C) 2023. *“Agricultural land” refers to “Land principally occupied by agriculture with significant areas of natural vegetation”.
The total LULC change from 1982 to 2023 corresponds to approximately 33.7% of the initial landscape area. This percentage reflects the extent of transformation relative to the baseline area in 1982. Meanwhile, the total swap LULC change from 1982 to 2023 represents approximately 73.4% of the initial landscape area in 1982. This indicates a significant level of internal reorganization among LULC categories over the study period.
Table 1 provides an overview of LULC changes within the strict regime zone from 1982 to 2023. This summary captures the area (in hectares) and the number of patches for each LULC category across three time points: 1982, 1991, and 2023. This data allows us to examine shifts in land cover, understand stability or transformation over time, and identify potential trends. The stability observed between 1982 and 1991 contrasts with more dynamic changes observed by 2023, reflecting both natural processes and management adjustments within the zone.
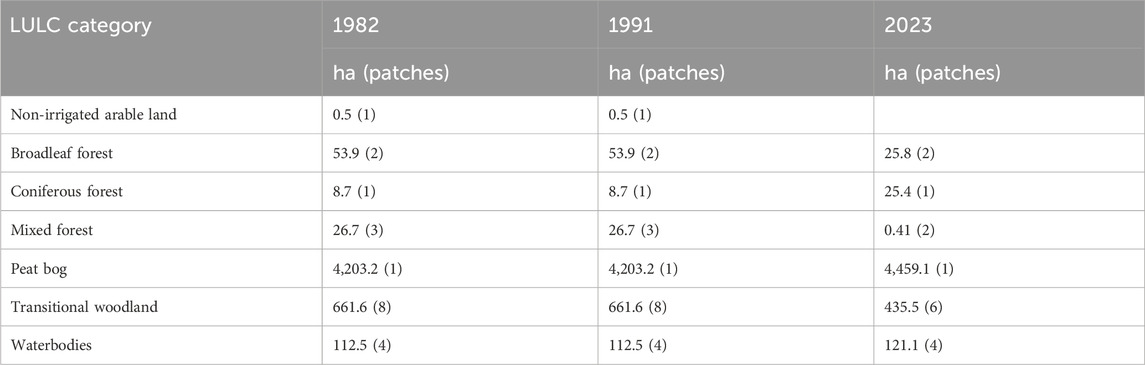
Table 1. Overview of LULC changes within the strict regime zone from 1982 to 2023, showing area (hectares) and number of patches for each LULC category at three time points (1982, 1991, and 2023).
The LULC data show several key processes influencing the strict regime zone over time. Most categories remained stable between 1982 and 1991, suggesting limited land-use interventions during this period. By 2023, however, notable shifts emerged. For instance, broadleaf forests and mixed forests both declined, possibly due to natural succession or selective management activities, while coniferous forests increased, indicating a shift in forest composition likely prompted by management. The expansion of peat bogs, the dominant cover type, suggests increased wetness and minimal human intervention, supporting natural bog formation. Similarly, waterbodies showed a slight increase, potentially due to hydrological changes or natural expansion. Transitional woodland-shrub areas declined, likely due to ecological succession leading to more stable forest types, further reflecting the zone’s low-intervention regime. Overall, the data illustrates that while the strict regime zone experienced minimal human intervention, natural processes such as succession, hydrological shifts, and forest composition dynamics significantly affected its LULC profile. These changes highlight the zone’s gradual evolution toward stable, mature ecosystems within a conservation-oriented context.
Table 2 summarizes the LULC changes within the nature park zone. This zone has maintained a relatively simple structure, with only minor changes in the distribution of land cover types over time, indicating low intervention and a steady-state land-use pattern until more dynamic shifts appeared by 2023.
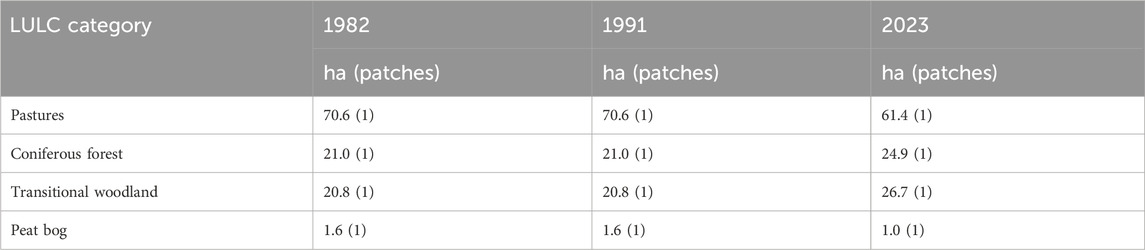
Table 2. Overview of LULC changes within the nature park zone from 1982 to 2023, showing area (hectares) and number of patches for each LULC category at three time points (1982, 1991, and 2023).
The data for the nature park zone demonstrates a relatively stable landscape between 1982 and 1991, with no notable changes in land cover during that period. This stability is likely due to the zone’s balance between conservation goals and traditional land-use practices, which maintained consistent LULC patterns. However, by 2023, the zone began to exhibit more dynamic changes. Pastures, the dominant land cover type, saw a reduction in area, potentially due to land abandonment or conversion to transitional woodland or forest types. The increase in coniferous forests indicates afforestation efforts aimed at enhancing forest cover. Similarly, the growth in transitional woodland-shrubs suggests natural succession processes, where open areas, such as pastures, have gradually transitioned to woodland. Peat bogs, though a minor land cover in the zone, showed a slight reduction in area, possibly due to dry conditions and subtle land-use changes. Overall, these shifts point to natural processes, such as succession and reforestation, driving the LULC changes within the nature park zone, alongside the potential effects of low-intensity land management practices.
Table 3 provides a comprehensive summary of the LULC dynamics within the regulatory regime zone. The data shows a complex interaction between agricultural activity, forest dynamics, and natural land cover types, indicative of the mixed management practices that characterize this zone.
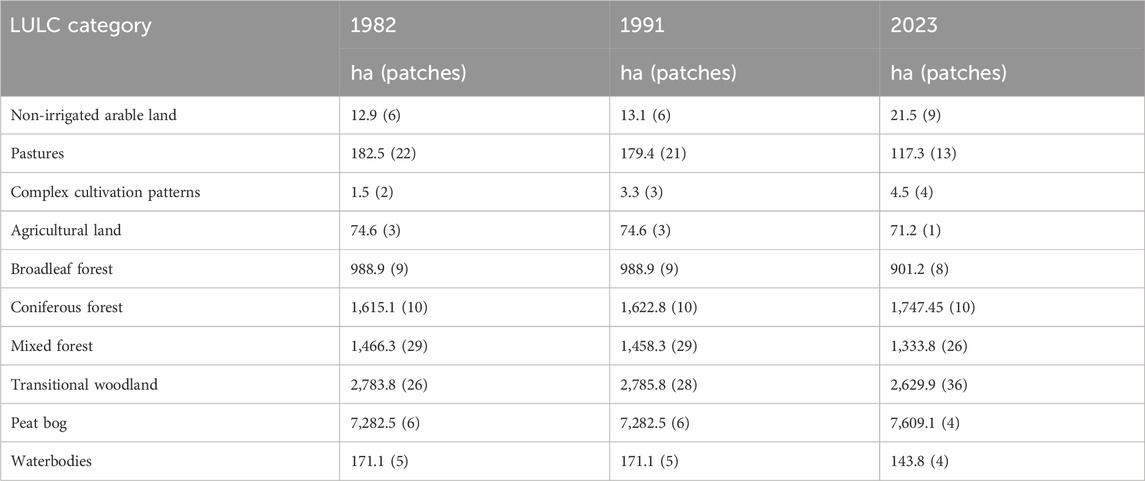
Table 3. Overview of LULC changes within the regulatory regime zone from 1982 to 2023, showing area (hectares) and number of patches for each LULC category at three time points (1982, 1991, and 2023).
The regulatory regime zone’s LULC profile highlights a diverse and evolving landscape, influenced by both natural processes and land management practices. Agricultural land, including non-irrigated arable land and complex cultivation patterns, expanded between 1982 and 2023, possibly due to increased demand for agricultural production. The consolidation of agricultural land into fewer patches by 2023 suggests more intensive land use practices and zoning adjustments to improve land efficiency. Forest dynamics reflect a shift in composition, with coniferous forest area increasing while mixed and broadleaf forests decreased. This trend suggests natural succession processes favoring coniferous forest and selective management practices aimed at increasing coniferous cover. Transitional woodland-shrub also declined, likely due to ecological succession toward more stable forest types. The area covered by peat bogs expanded, indicating the influence of natural hydrological conditions and conservation practices that limit drainage or disturbance, supporting peatland stability and growth. Meanwhile, a decrease in waterbody area suggests potential desiccation or changes in hydrological management that reduced the number or extent of aquatic areas. Overall, the LULC changes in the regulatory regime zone reflect a combination of conservation-driven management, natural forest succession, and the adaptation of agricultural practices, producing a landscape that is both diverse and responsive to regulatory policies.
Table 4 presents a summary of LULC changes within the buffer zone. The LULC composition indicates dynamic land-use activities, including urban expansion, agricultural shifts, and forest cover changes. The observed trends emphasize the dual role of this zone as both a buffer for the surrounding protected areas and a region of active land use.
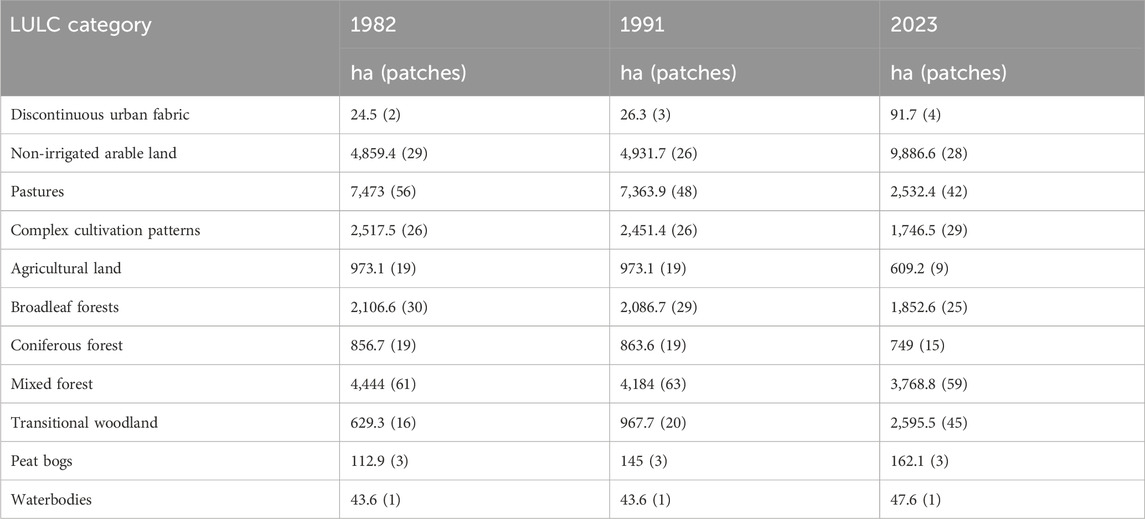
Table 4. Overview of LULC changes within the buffer zone from 1982 to 2023, showing area (hectares) and number of patches for each LULC category at three time points (1982, 1991, and 2023).
The buffer zone shows a landscape undergoing extensive transformation between 1982 and 2023. One of the most notable changes is the substantial increase in non-irrigated arable land, likely driven by agricultural expansion, which appears to have come at the expense of pastures and some forested areas. Pastures, previously a dominant land cover, decreased considerably, suggesting a shift in agricultural practices toward more intensive cultivation of arable land. Urban expansion is evident in the increase in discontinuous urban fabric, which aligns with pressures for development in the margin of protected areas. This trend of urbanization can contribute to increased fragmentation within the buffer zone, impacting ecosystem continuity and connectivity. Forested areas experienced a decline, likely due to conversion to agricultural and urban land uses. Conversely, transitional woodland-shrub showed a notable increase, potentially reflecting land abandonment and natural succession processes filling in previously open or disturbed areas. Peat bogs and waterbodies remained relatively stable, with slight increases, indicating preserved or naturally stable hydrological conditions in some parts of the buffer zone. The presence of these stable land-cover types, despite surrounding changes, suggests areas within the buffer zone may retain conservation value. The LULC changes observed underline the zone’s complex role in balancing development needs with ecosystem conservation, indicating a need for carefully managed land-use practices to maintain its buffering function. Furthermore, the buffer zone displays several transition hotspots, where the likelihood of shifts between agricultural, urban, and transitional land types is high. This variability suggests a dynamic landscape influenced by policy decisions, demographic shifts, and economic pressures. Managing these areas with adaptable zoning could help mitigate fragmentation and promote multifunctional land use that aligns with conservation goals.
3.2 Spatiotemporal dynamics of historical land use and land cover change
A spatiotemporal analysis of LULC dynamics between 1982 and 1991 reveals substantial shifts in the areal extent and fragmentation of LULC categories (Figure 3). During this period, notable decreases were observed in non-irrigated arable lands (−16%), pastures (−12%), and mixed forests (−4%). Conversely, non-irrigated arable lands also experienced the most significant gain (+17%), followed by pastures (+11%) and transitional woodland-shrubs (+8%). At the same time, categories such as agriculture with natural vegetation and waterbodies showed no significant changes throughout this period.
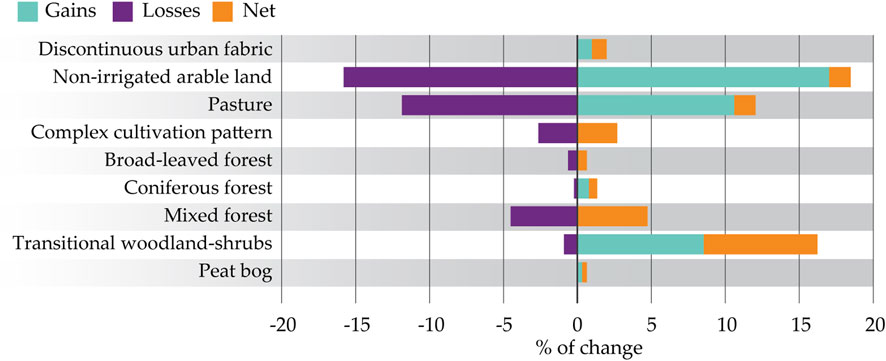
Figure 3. Gains, losses, and net changes (percentage of change) by land use and land cover category between 1982 and 1991.
Non-irrigated arable land showed both the most significant annual loss and gain, with a decrease of approximately −1.7% per year and an increase of 1.8% per year, indicating active agricultural expansion and retraction. Pastures followed a similar trend, with an annual decrease of −1.3% and an increase of 1.2% per year, reflecting both land conversion and re-establishment of pastures. Mixed forests experienced an annualized decrease of −0.4%, suggesting a gradual loss of mixed forest cover during this period. In contrast, transitional woodland-shrubs exhibited an annualized gain of 0.9%, likely due to natural succession processes in previously open or disturbed areas. Categories such as agriculture with natural vegetation and waterbodies remained largely unchanged, indicating stability in these land cover types over the period.
Meanwhile, the analysis of net LULC changes between 1982 and 1991 reveals a complex interaction of change processes. Transitional woodland-shrubs experienced the most notable increase (+8%), while mixed forests (−5%), complex cultivation patterns (−3%), and pastures (−1%) showed the largest net losses. To interpret the drivers behind these net changes, a source-sink matrix was employed (Pramanik and Punia, 2020; Guo and Fang, 2021). This matrix identified key contributors to net changes within specific LULC categories. For instance, pastures and complex cultivation patterns contributed to the net change in non-irrigated arable lands, while transitional woodland-shrubs played a significant role in the net change observed in broadleaf forests. Notably, mixed forests experienced net change influenced primarily by their own category and a decrease due to transitional woodland-shrubs (Figure 4).
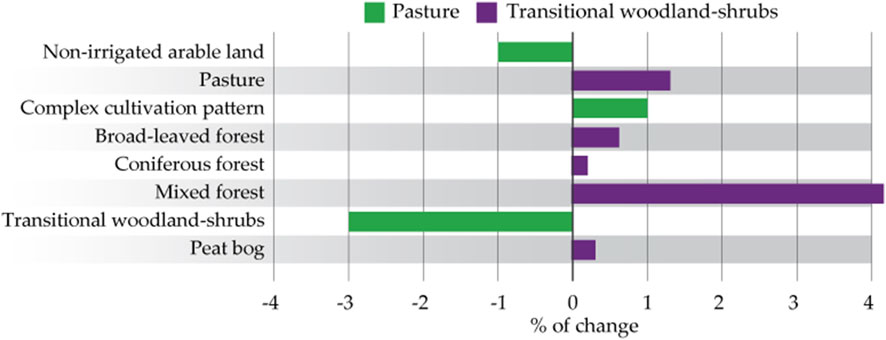
Figure 4. Contributors to the net change (percentage of change) in pastures and transitional woodland-shrubs between 1982 and 1991.
Between 1982 and 1991, the net LULC changes reveal a nuanced landscape development with varying annual rates of increase and decrease across land cover categories. Transitional woodland-shrubs exhibited the highest annualized net gain at approximately 0.9% per year, reflecting growth likely driven by natural succession and land abandonment. Conversely, mixed forests showed an annualized net loss of around −0.6% per year, suggesting a gradual decline in this category. Complex cultivation patterns and pastures also decreased, with annualized losses of −0.3% and −0.1% per year, respectively, indicating shifts away from traditional land-use practices.
Between 1982 and 1991, the case study area experienced significant LULC transformations (Figure 5). During this period, processes of land cover enlargement, attrition, aggregation, creation, and dissection were observed. Attrition and enlargement were the most prominent processes within the case study area. Attrition led to a decrease in land patch size and overall coverage of LULC categories within the buffer zone. Additionally, the buffer zone also saw substantial aggregation, where patches of non-irrigated land coalesced into larger units. Conversely, the regulatory regime zone and strict regime zone exhibited enlargement, characterized by area expansion without changes in land patch quantity. The northern sector of the case study area underwent dissection, resulting in an increased number of smaller land patches. To further understand the landscape dynamics, the compactness ratio of LULC categories was assessed. While the compactness ratio is highly varied (0.1–>0.8), generally it does not exceed 0.4, which suggests an environment where land cover types (e.g., forests, urban areas, agricultural lands) are dispersed and fragmented rather than forming large, contiguous, and compact land patches. This state can have substantial ecological implications, such as reduced habitat connectivity, increased edge effects, and greater vulnerability to environmental disturbances (Bo et al., 2023; Min et al., 2010; Das and Nautiyal, 2004).
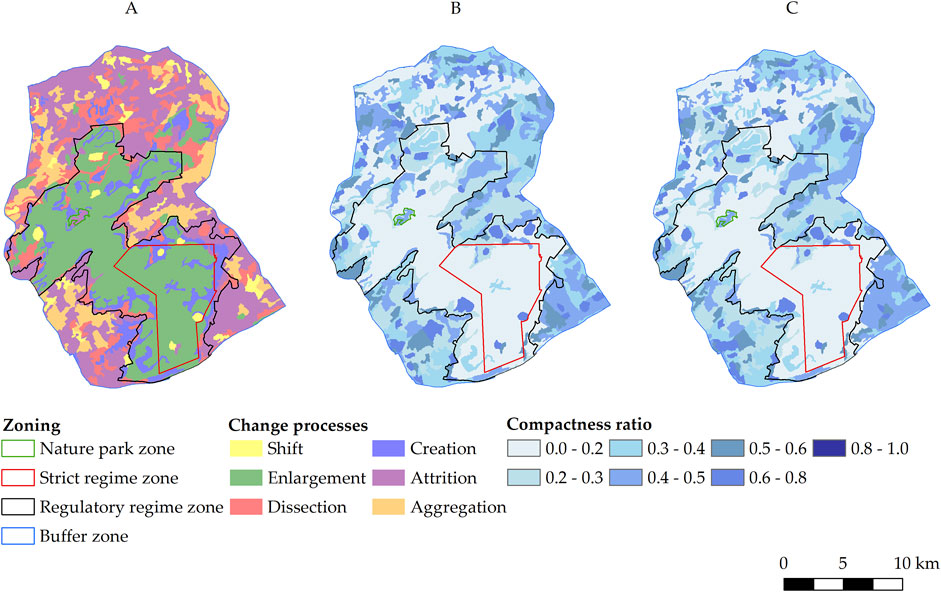
Figure 5. (A) Land use and land cover changes between 1982 and 1991, with land cover patch compactness ratio shown for (B) 1982 and (C) 1991.
Building on the dynamics observed from 1982 to 1991, the period from 1991 to 2023 reveals further transformations in LULC categories within the case study area (Figure 6). The most notable change during this period was the substantial expansion of discontinuous urban fabric, which increased by 80%. This was followed by notable growth in non-irrigated arable lands (+59%) and transitional woodland-shrubs (+50%). Agricultural land with natural vegetation also saw an increase of 47%. In contrast, pastures experienced the most considerable decline, with a decrease of 77%, alongside a significant reduction in agricultural land with natural vegetation (−65%). Complex cultivation patterns (−42%), broadleaf forests (−31%), and transitional woodland-shrubs (−35%) also faced notable decreases, highlighting a period of considerable reconfiguration in land cover within the case study area.
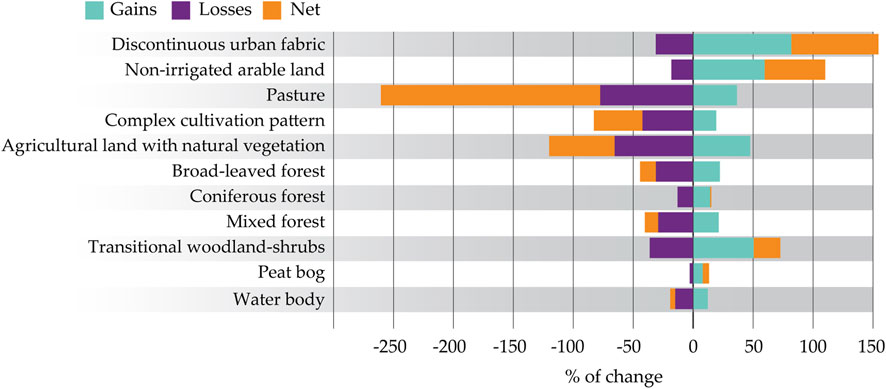
Figure 6. Gains, losses, and net changes (percentage of change) by land use and land cover category between 1991 and 2023.
Between 1991 and 2023, the case study area continued to experience significant annual LULC changes. Discontinuous urban fabric saw the highest annualized increase at approximately 1.8% per year, reflecting ongoing urban expansion. Non-irrigated arable land followed with an annual growth rate of 1.5%, indicating sustained agricultural expansion. Transitional woodland-shrub increased by 1.3% annually, likely due to natural succession, while agricultural land with natural vegetation expanded by 1.2% per year, suggesting a trend towards more extensive agricultural practices. Conversely, pastures experienced the steepest annual decline at −1.8% per year, indicating widespread conversion to other land uses. Agricultural land with natural vegetation also declined at −1.6% annually, likely due to repurposing for more intensive uses. Complex cultivation patterns decreased by −1.1% per year, and broadleaf forests saw an annual decline of −0.8%, indicating reduced forest cover. Additionally, transitional woodland-shrub faced a gradual decline of −0.9% per year, highlighting complex interactions within this category. These annualized rates demonstrate a period of significant transformation, marked by urbanization, agricultural expansion, and reorganization of natural land covers, with potential implications for ecological integrity and landscape connectivity.
At the same time, the net changes in LULC categories between 1991 and 2023 highlight a positive trend for discontinuous urban fabric (+72%) and non-irrigated arable lands (+50%), indicating ongoing urbanization and agricultural expansion. Conversely, pastures (−181%), complex cultivation patterns (−40%), and agricultural land with natural vegetation (−54%) experienced substantial net losses.
Between 1991 and 2023, net changes in LULC categories indicate ongoing urbanization and agricultural expansion alongside significant reductions in other land cover types. Discontinuous urban fabric showed an annualized net increase of approximately 1.7% per year, reflecting consistent urban growth. Non-irrigated arable land expanded at an annualized rate of 1.3%, indicating steady agricultural intensification. Conversely, pastures experienced the steepest annualized net loss at −3.3% per year, suggesting widespread conversion to other uses. Complex cultivation patterns decreased by −1.1% annually, possibly due to a shift towards simpler or more intensive land uses. Agricultural land with natural vegetation also declined at an annualized rate of −1.4%, likely reflecting changes in land management practices or repurposing for urban and agricultural needs. These trends highlight the substantial reconfiguration of land cover within the case study area, underscoring a shift towards urban and agricultural land use at the expense of natural and semi-natural landscapes.
Analysis of the primary drivers behind LULC changes reveals that the growth in discontinuous urban fabric was primarily driven by transitions from complex cultivation patterns (+2%). The expansion of non-irrigated arable land was achieved largely at the expense of pastures (+54%), complex cultivation patterns (+24%), and agricultural land with natural vegetation (+16%). The decline in pastures (Figure 7) was mainly due to conversions to non-irrigated arable lands (−83%), discontinuous urban fabric (−10%), transitional woodland-shrubs (−9%), and broadleaf forests (−9%). Complex cultivation patterns saw a contraction mainly due to transitions to discontinuous urban fabric (−244%) and non-irrigated arable lands (−12%). Agricultural land with natural vegetation also faced reductions from conversions to non-irrigated arable lands, complex cultivation patterns, broadleaf forests, and transitional woodland-shrubs. Broadleaf forests experienced mixed influences, with losses to transitional woodland-shrubs (−11%) partially countered by gains from agricultural land with natural vegetation (+5%) and pastures (+4%). Coniferous forests gained area primarily from mixed forests (+1%) and broadleaf forests (+1%), but lost areas to transitional woodland-shrubs (−2%) and waterbodies (−1%). Mixed forests saw a net decrease due to conversions to transitional woodland-shrubs (−14%) and minor gains from broadleaf forests (+4%) and non-irrigated arable lands (+3%). Transitional woodland-shrubs (Figure 7) expanded due to gains from agricultural land with natural vegetation (+13%), broadleaf forests (+15%), mixed forests (+11%), and water bodies (+11%). Peat bogs saw growth driven mainly by transitional woodland-shrubs (+13%), while waterbodies experienced a decrease primarily due to losses from transitional woodland-shrubs (−1%).
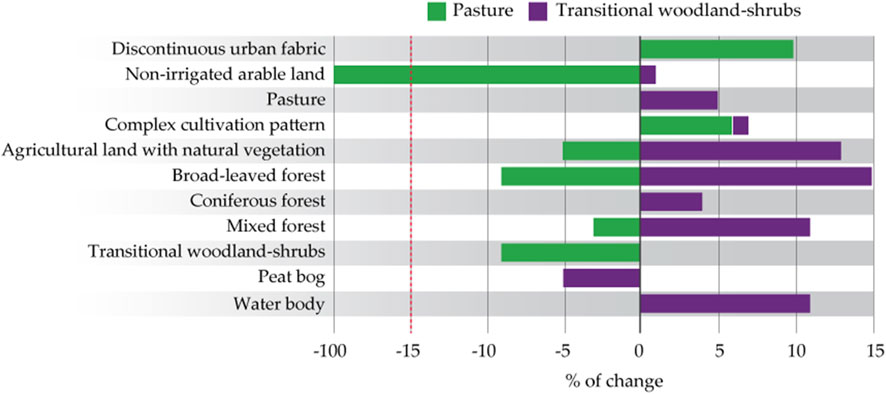
Figure 7. Contributors to the net change (percentage of change) in pastures and transitional wood-land-shrubs between 1991 and 2023.
The annualized values for LULC changes between 1991 and 2023 reveal specific trends across various land cover categories. Discontinuous urban fabric grew at a rate of approximately +0.1% per year, primarily driven by transitions from complex cultivation patterns. Non-irrigated arable lands expanded annually by +1.7% from pastures, +0.7% from complex cultivation patterns, and +0.5% from agricultural land with natural vegetation. Pastures, on the other hand, declined significantly, losing −2.6% per year to non-irrigated arable lands, −0.3% to discontinuous urban fabric, and −0.3% each to both transitional woodland-shrubs and broadleaf forests. Complex cultivation patterns contracted due to annual transitions of −7.6% to discontinuous urban fabric and −0.4% to non-irrigated arable lands. Broadleaf forests experienced a net annual loss of −0.3% to transitional woodland-shrubs, though they gained +0.2% per year from agricultural land with natural vegetation and +0.1% from pastures. Coniferous forests gained minimally, at +0.03% annually from both mixed and broadleaf forests, while experiencing small losses to transitional woodland-shrubs (−0.1%) and waterbodies (−0.03%) per year. Mixed forests saw a yearly net decrease of −0.4% due to transitions to transitional woodland-shrubs, with minor gains from broadleaf forests (+0.1%) and non-irrigated arable lands (+0.1%). Transitional woodland-shrubs expanded annually due to gains of +0.4% from agricultural land with natural vegetation, +0.5% from broadleaf forests, and +0.3% each from mixed forests and water bodies. Peat bogs grew at +0.4% per year from transitional woodland-shrubs, while waterbodies decreased slightly, losing −0.03% per year to transitional woodland-shrubs.
The period from 1991 to 2023 also saw significant changes in LULC spatial distribution, characterized by processes of aggregation, creation, and attrition (Figure 8). In the buffer zone, notable creation was observed, with new land cover patches appearing and existing patches decreasing in size. Conversely, the regulatory regime zone and strict regime zone experienced aggregation, where existing land patches coalesced into larger units. The compactness ratio of 2023 land cover is still highly varied (0.1–>0.8), and as before, it generally does not exceed 0.4. However, the overall compactness is considerably lower than in 1982 or 1991, especially within strict regime zone and regulatory regime zone, indicating increased loss in habitat connectivity, increased edge effects, and even greater vulnerability to environmental disturbances (Guo and Fang, 2021; Das and Nautiyal, 2004).
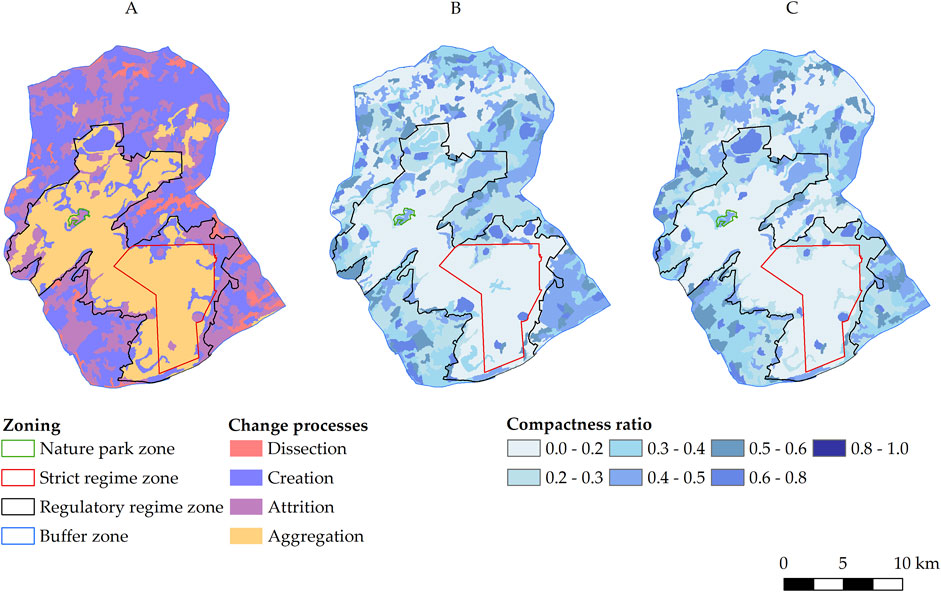
Figure 8. (A) Land use and land cover changes between 1991 and 2023, with land cover patch compactness ratio shown for (B) 1991 and (C) 2023.
3.3 Future of land use and land cover change
Projecting further into the future generally means greater uncertainty compared to modeling past changes. Furthermore, factors that have not significantly influenced LULC change in the past can become prominent in the future; this is especially true for climatic factors (Sannigrahi et al., 2020; Thurman et al., 2022; Thom, 2023; Guo et al., 2024). Nevertheless, it is expected that two dominant processes within the case study area between 2023 and 2064 will be the creation of new land patches and the dissection of the existing ones (Figure 9). At the same time, the overall compactness ratio in 2064 is expected to significantly decrease, especially for the strict regime zone and regulatory regime zone (<0.15), but also for the buffer zone, where most of the land fragmentation is expected to happen in the northern part of the case study area.
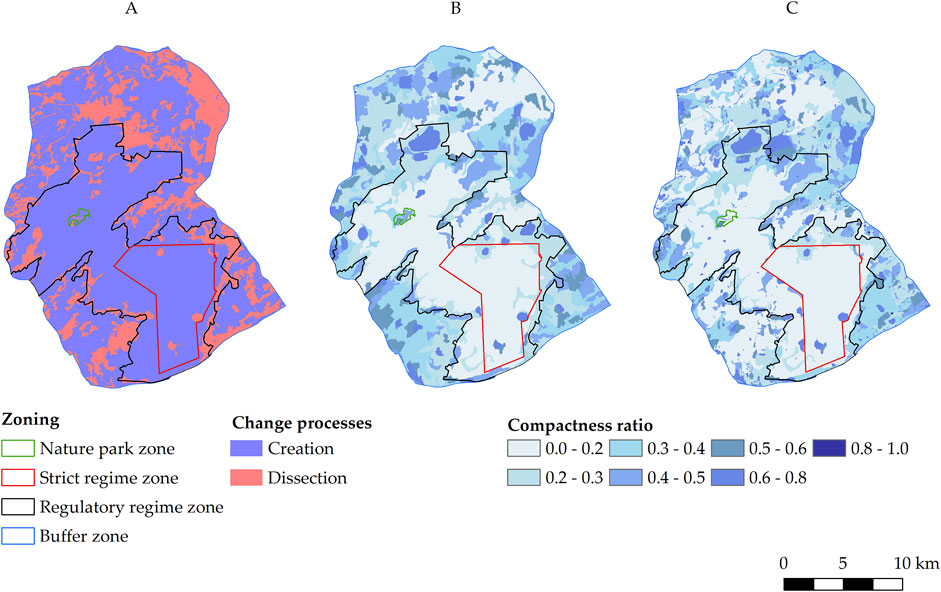
Figure 9. (A) Projected land use and land cover changes between 2023 and 2064, with land cover patch compactness ratio shown for (B) 2023 and (C) 2064 (projected).
To generalize potential future trajectories of the case study area, four distinct scenario projections were constructed and then simulated (Figure 10). Each scenario incorporated dynamic modeling of critical variables, including both legislative frameworks and environmental parameters. All four scenarios indicate that the highest probability for transition is in the buffer zone, generally more than 75%, while the probability for transition within strict regime zone and regulatory regime zone is considerably lower - <20% for strict regime zone and <15% for regulatory regime zone.
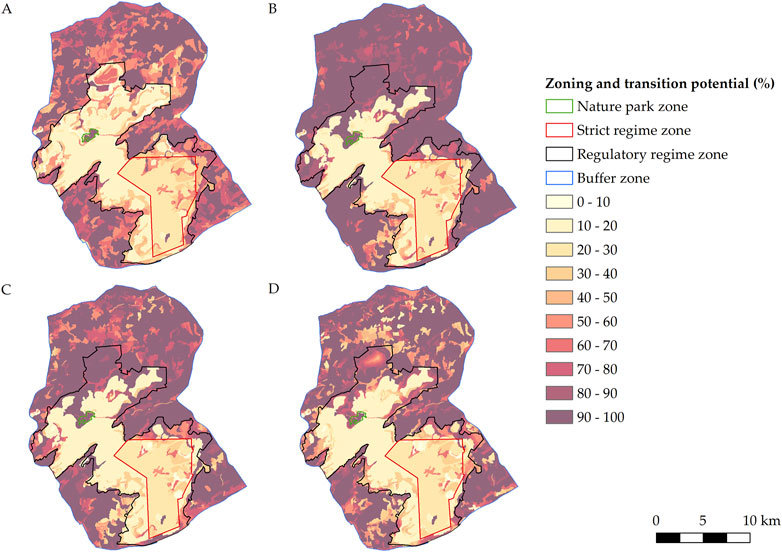
Figure 10. Projected potential for land use and land cover transitions during the 2023-2064 period under four development scenarios: (A) Scenario 1, (B) Scenario 2, (C) Scenario 3, and (D) Scenario 4.
Leveraging projections of potential LULC transitions over the 2023-2064 period, four potential outlooks for LULC in 2064 (Figure 11) were developed. All four maps show considerable differences, depending on the projected scenario.
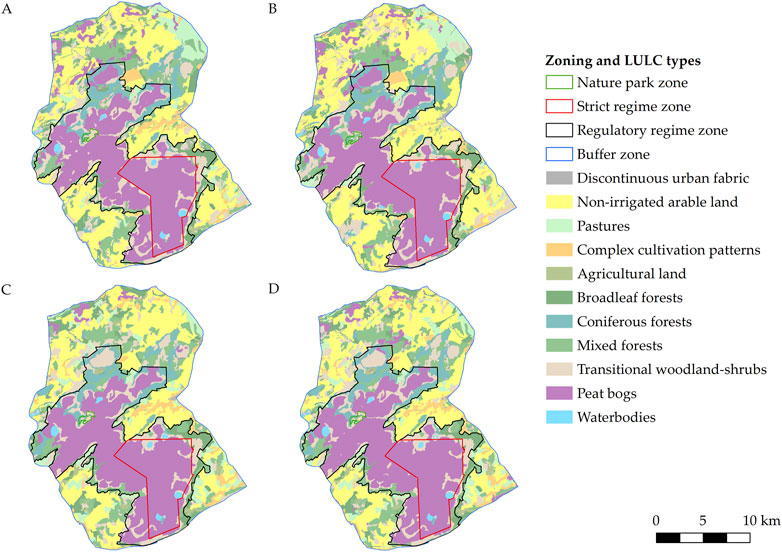
Figure 11. Projected land use and land cover in the case study area for the year 2064 under four modeled scenarios: (A) Scenario 1, (B) Scenario 2, (C) Scenario 3, and (D) Scenario 4. *“Agricultural land” refers to “Land principally occupied by agriculture with significant areas of natural vegetation”.
Scenario 1 projects the most significant LULC changes across all categories (Figure 12). Discontinuous urban fabric is expected to decrease by over 30%. Non-irrigated land will see both substantial losses exceeding 20% and gains surpassing 20%. Pastures may decline dramatically by more than 80%, though gains may exceed 70%. Complex cultivation patterns are projected to experience significant losses over 45%, with notable gains of more than 25%. Agricultural land could face a severe decline of over 70%, partially offset by around 40% gains. Forests, combined, are anticipated to show varied changes: broadleaf forests will grow by approximately 20% but will also face losses exceeding 30%; coniferous forests might experience a minor decrease of 15% with a 16% gain; mixed forests are projected to decline moderately by 27%, partially offset by 25% gains. Overall, forests are expected to experience a net loss. Transitional woodland-shrubs may grow by 45%, with some losses of 40%. Peat bogs are projected to gain modestly by 12% while facing minimal losses of 4%. Waterbodies might increase by 9% but also decrease by 17%. The estimated net changes between 2023 and 2064 are as follows: discontinuous urban fabric −51%, non-irrigated arable land +4%, pastures −44%, complex cultivation patterns −37%, agricultural land −88%, forests −7%, transitional woodlands +9%, peat bogs +8%, and waterbodies −10%.
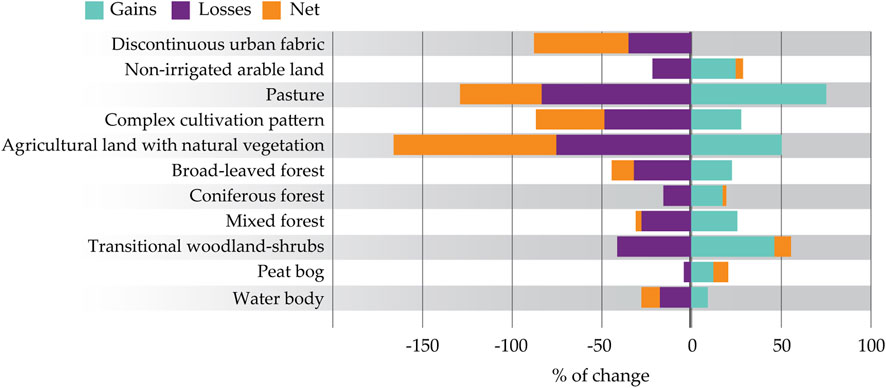
Figure 12. Potential gains, losses, and net changes (percentage of change) by land use and land cover category between 2023 and 2064 under Scenario 1.
Under Scenario 1, projected changes from 2023 to 2064 indicate substantial annualized transformations across all LULC categories. Discontinuous urban fabric is expected to decline by an annualized rate of −1.3%, reflecting an ongoing reduction in urban areas. Non-irrigated arable land is projected to fluctuate, with both annualized losses and gains exceeding 0.5%, suggesting dynamic agricultural transitions. Pastures may experience the most significant decline, with an annualized reduction of around −2.5%, counterbalanced by gains estimated at 1.2% annually. Complex cultivation patterns are expected to decrease at a rate of −1.5% per year, although they could see annual gains of around 0.7%. Agricultural land is projected to undergo a drastic annual reduction of −2.6%, with gains of approximately 1%, indicating intensive land use shifts. Forested areas are expected to see varied changes: broadleaf forests are projected to grow at an annualized rate of 0.4% while facing losses of −1%; coniferous forests might see a slight annualized decrease of −0.4%, balanced by gains of 0.4%; and mixed forests are expected to decline by −0.9% per year, partially offset by an annual growth rate of 0.7%. Despite these fluctuations, forests overall are projected to experience a net annual decline of about −0.2%. Transitional woodland-shrubs may expand at a rate of 1% annually, though losses of around −0.9% per year are anticipated. Peat bogs are expected to show modest annual gains of 0.3%, with minimal annual losses of −0.1%, while waterbodies might increase by 0.2% per year but also face declines of around −0.4%.
Scenario 2 (Figure 13), though covering changes across LULC categories, exhibits a significantly smaller net change compared to Scenario 1. Discontinuous urban fabric is expected to decline substantially by more than 30%, with possible increases exceeding 45%. Non-irrigated arable land is projected to lose around 20%, though gains may exceed 25%. Pastures might face a dramatic decline of over 80%, partially offset by gains exceeding 70%. Complex cultivation patterns are anticipated to experience notable losses nearing 50%, alongside gains over 30%. Agricultural land may see a severe decline exceeding 70%, mitigated by about 62% gains. Forests, on aggregate, are expected to show broadleaf forests with losses greater than 35% and gains around 24%; coniferous forests with a minor decrease of 16%, balanced by a 17% gain; mixed forests with a moderate decline of 34% and partial recovery of 27% gains. Overall, forests are likely to see a net decrease. Transitional woodland-shrubs may experience a 42% decline, partially offset by 46% gains. Peat bogs are expected to have minimal losses of 4% and gains of 12%. Waterbodies might decline by 19%, with a gain of 16%. The estimated net changes between 2023 and 2064 are: discontinuous urban fabric +22%, non-irrigated arable land +7%, pastures −36%, complex cultivation patterns −34%, agricultural land −40%, forests −9%, transitional woodlands +7%, peat bogs +8%, and waterbodies −4%.
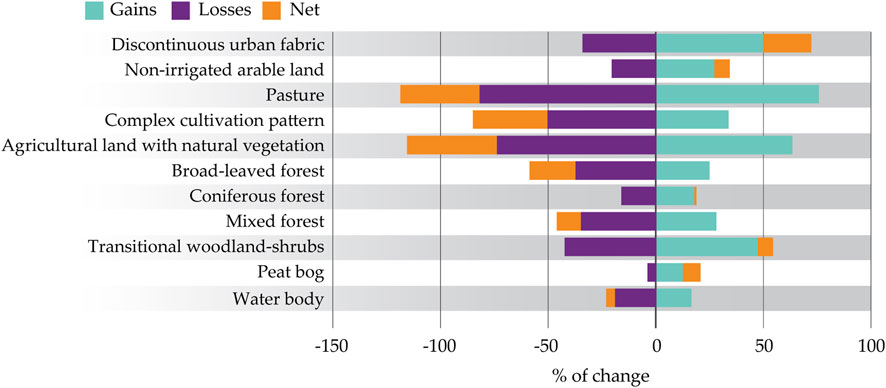
Figure 13. Potential gains, losses, and net changes (percentage of change) by land use and land cover category between 2023 and 2064 under Scenario 2.
Under Scenario 2, projected annualized changes between 2023 and 2064 indicate moderate transformations across LULC categories. Discontinuous urban fabric is expected to experience an annualized decline of approximately −0.6% per year, while potentially seeing gains up to 1.1% annually, reflecting a mixed trend of urban contraction and expansion. Non-irrigated arable land could see losses at a rate of −0.4% per year, balanced by an estimated annual gain of 0.6%, suggesting fluctuating agricultural land use. Pastures are expected to decline at an annual rate of −1.4%, though with partial offset by potential gains at around 1.2% per year. Complex cultivation patterns are expected to shrink by −1% per year, with annual gains reaching 0.6%, indicating ongoing adaptation within agricultural practices. Agricultural land could experience a significant annual decrease of −1.3%, offset by an estimated annual growth of 1%, reflecting varied use patterns. Forest types show a range of dynamics: broadleaf forests may decline at −0.7% per year with an annualized gain of 0.5%; coniferous forests might have a slight annual decline of −0.4% with gains up to 0.4%; and mixed forests are expected to decrease by −0.7% annually, with growth balancing out at 0.6%. Transitional woodland-shrubs may undergo an annual decrease of −0.9% but also see gains of up to 0.9%, suggesting ecological processes balancing out changes within these areas. Peat bogs show modest annual increases of 0.1% and small losses at −0.07% annually, while waterbodies may decrease at −0.4% with potential increases of 0.4% annually, likely due to hydrological changes. These annualized projections indicate a balanced landscape under Scenario 2, with moderate net losses in natural and agricultural lands alongside smaller, compensatory gains, reflecting a controlled and gradual shift in land use patterns.
Scenario 3 (Figure 14) forecasts the least significant changes in land cover. Discontinuous urban fabric is expected to decline moderately by 8%. Non-irrigated arable land may see a 5% reduction, slightly offset by 6% gains. Pastures are projected to decrease by 20%, with a partial recovery of 14%. Complex cultivation patterns are anticipated to fall by 12%, though gains of 5% are expected. Agricultural land may decline by 18%, partially countered by a 7% increase. Broadleaf forests are likely to experience an 8% loss, with 5% gains. Coniferous forests might see a minor decrease of 4%, balanced by a 4% gain. Mixed forests are projected to decline by 7%, with a partial recovery of 6%. Transitional woodland-shrubs are expected to decrease by 10%, offset by 12% gains. Peat bogs are anticipated to have minimal losses of 1% and 3%. Waterbodies may decline by 4%, with a 2% in-crease. The estimated net changes between 2023 and 2064 suggest predominantly negative trends: discontinuous urban fabric −8%, pastures −20%, complex cultivation patterns −7%, and agricultural land −13%. Broadleaf forests may have a slight net loss of 3%, mixed forests a minimal net loss of 1%, and waterbodies a net decline of 2%. Conversely, positive net changes are forecasted for non-irrigated arable land (+1%), coniferous forests (+0.4%), transitional woodland-shrubs (+2%), and peat bogs (+2%).
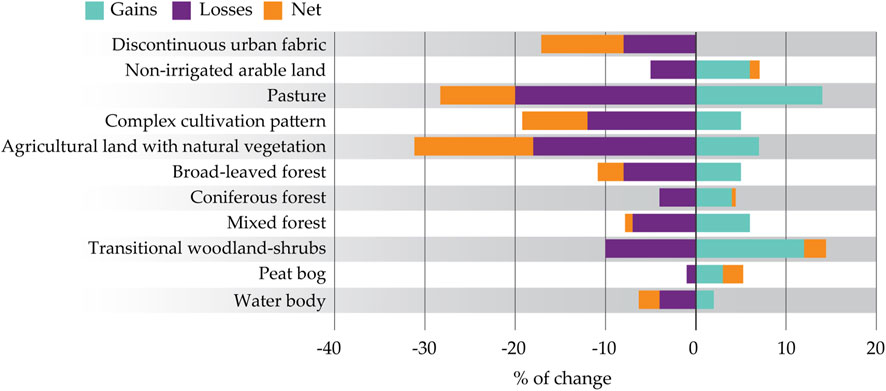
Figure 14. Potential gains, losses, and net changes (percentage of change) by land use and land cover category between 2023 and 2064 under Scenario 3.
Scenario 3 forecasts moderate, controlled changes across LULC categories from 2023 to 2064, reflecting a landscape with smaller annualized shifts. Discontinuous urban fabric is expected to see a minor annual decline of −0.2%, while non-irrigated arable land shows a small decrease of −0.1% per year, partially offset by annual gains of 0.1%. Pastures may face a moderate decline at an annual rate of −0.4%, though gains at approximately 0.3% per year indicate some recovery. Complex cultivation patterns are anticipated to decrease at a rate of −0.3% per year, with minor gains at 0.1% annually, reflecting slight adjustments in land use. Agricultural land could see a steady annual decline of −0.4%, with partial gains of 0.2%, suggesting controlled shifts in agricultural practices. Broadleaf forests are projected to experience a modest decrease of −0.2% per year, balanced by a 0.1% annual growth, while coniferous forests may have a slight reduction of −0.1%, with gains up to 0.1% annually. Mixed forests are expected to decline at −0.2% annually, countered by annualized growth of 0.1%. Transitional woodland-shrubs may face a decrease of −0.2% per year, balanced by growth of 0.3% annually, suggesting ongoing ecological succession. Peat bogs are anticipated to have a minimal annual loss of −0.02% with a slight gain of 0.07% annually, while waterbodies may see an annual decrease of −0.1%, countered by a gain of 0.05%. These annualized rates in Scenario 3 indicate a stable landscape with moderate net losses in agricultural and pasture lands, alongside steady increases in transitional woodlands and peat bogs, reflecting an environment with balanced, gradual land-use adjustments.
Scenario 4 (Figure 15) reveals moderate intensity in LULC changes. Discontinuous urban fabric is projected to decrease significantly by 17%. Non-irrigated arable land is expected to lose 10%, with a partial recovery of 12%. Pastures might face a sharp decline of 39%, though gains of 28% will provide some mitigation. Complex cultivation patterns are anticipated to drop by 23%, with a 10% increase partially offsetting the loss. Agricultural land could decline by 30%, mitigated by 9% gains. Broadleaf forests may experience a 15% loss, counterbalanced by a 10% gain. Coniferous forests might see a 7% decrease, offset by an 8% gain. Mixed forests are projected to decline by 13%, with 11% gains partially offsetting the reduction. Transitional woodland-shrubs may decrease by 17%, with gains of 21% somewhat mitigating this loss. Peat bogs are expected to see minimal losses of 2% and 6% gains. Waterbodies might decline by 9%, partially offset by a 4% increase. The estimated net changes between 2023 and 2064 are: discontinuous urban fabric −20%, non-irrigated arable land +2%, pastures −11%, complex cultivation patterns −12%, agricultural land −31%, broadleaf forests −5%, coniferous forests +1%, mixed forests −1%, transitional woodlands +4%, peat bogs +4%, and waterbodies −4%.
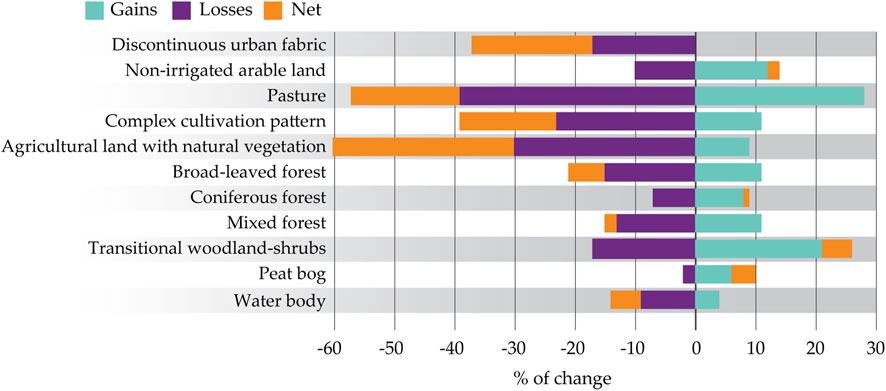
Figure 15. Potential gains, losses, and net changes (percentage of change) by land use and land cover category between 2023 and 2064 under Scenario 4.
Under Scenario 4, moderate LULC changes are anticipated from 2023 to 2064, with varied annualized rates across categories. Discontinuous urban fabric is projected to decline at an annualized rate of −0.4%, indicating a gradual contraction of urban areas. Non-irrigated arable land may decrease by −0.2% per year, with a partial recovery at 0.3% annually, showing modest fluctuation in agricultural land use. Pastures could face a notable decline, with an annual decrease of −0.8%, balanced by an annual gain of 0.6%, reflecting some recovery. Complex cultivation patterns are expected to contract at −0.5% per year, with gains at 0.2% annually, indicating a slight adaptation in land management practices. Agricultural land might decline steadily at −0.6% annually, with moderate gains at 0.2% per year, suggesting gradual shifts in agricultural land use. Broadleaf forests may experience an annual loss of −0.3%, countered by a yearly increase of 0.2%, while coniferous forests could face a minor decrease of −0.2%, offset by annual gains of 0.2%. Mixed forests are anticipated to decline at −0.3% per year, with growth at around 0.3% annually, indicating minimal net change. Transitional woodland-shrubs might decrease by −0.4% annually, with partial growth at 0.5%, suggesting dynamic natural processes within this category. Peat bogs are expected to see minimal annual losses at −0.05% alongside modest growth of 0.1%, while waterbodies could experience a slight annual reduction of −0.2%, with a minor gain of 0.1% per year. These projections indicate moderate, balanced shifts across LULC categories under Scenario 4, with steady contractions in agricultural and urban areas, partially mitigated by stable forest and transitional land gains.
Each protection zone in the Teiči Strict Nature Reserve experiences distinctive LULC dynamics driven by a combination of socio-economic, policy, environmental, and technological factors. In the strict conservation zone, natural ecosystem processes dominate, while policy frameworks in regulatory zones balance minimal human activities with biodiversity goals. Socio-economic and environmental pressures in the nature park and buffer zone highlight the need for adaptive policies, particularly as agricultural or urban pressures increase under certain scenarios.
Since the strict protection zone prohibits economic activities and restricts access, direct socio-economic drivers like urbanization or agricultural expansion are minimal. However, demographic shifts or nearby development could indirectly affect this zone by increasing edge effects or human disturbance along boundaries. This zone’s stringent regulations align with biodiversity and ecosystem protection goals, with limited activities allowed for research or conservation purposes. In scenarios with stricter conservation policies (Scenario 2), these regulations could intensify further safeguarding this area. Maintaining natural ecosystem dynamics in this zone will rely heavily on enforced regulations and effective perimeter controls. Climate change under RCP 4.5 may drive ecological shifts within the zone, potentially influencing vegetation patterns and species distributions. Natural ecosystem processes, such as succession, would likely dominate LULC changes here, with limited human intervention allowing for adaptation to environmental changes over time.
The regulatory regime zone supports minimal anthropogenic activities, which could include limited, controlled forestry or conservation-related tourism. Economic factors influencing demand for recreational access, or sustainable forestry could affect permitted activities, shaping LULC changes under scenarios that allow limited land use adjustments. Under stricter conservation scenarios (Scenario 2), regulatory oversight may limit forestry or recreational activities, emphasizing conservation. In contrast, in a scenario prioritizing agricultural expansion (Scenario 3), there could be pressures to loosen some restrictions, although they would still remain within conservation goals. Effective management in this zone depends on balancing conservation with regulated use, which could shift based on conservation priorities or policy adjustments. Climate-driven changes in ecosystem composition may influence land cover in this zone, especially where selective forestry or species protection efforts are active. Successional processes could occur in areas with reduced human impact, with natural vegetation potentially expanding as restrictions on intensive land use remain in place. Conservation-driven practices, like precision forestry and sustainable tourism infrastructure, could support the zone’s conservation objectives while maintaining minimal human disturbance.
Nature park zone balances conservation with local community interests, allowing traditional land management. Socio-economic factors, such as local demand for resources or tourism, might encourage certain traditional practices, like grazing, which can maintain historical landscape structures. Declining agricultural profitability or population shifts could, however, lead to reduced traditional management, allowing natural succession to take over. Scenario 4’s balanced approach, promoting agroforestry and sustainable practices, aligns well with this zone’s objectives. Conservation-focused scenarios (e.g., Scenario 2) may prioritize preservation of natural landscapes, potentially limiting traditional practices, while agricultural expansion scenarios could increase the pressure to convert more areas for farming. Adaptive zoning could maintain flexibility for sustainable practices while ensuring conservation goals are met. Climate change may impact traditional land use practices by altering the viability of certain agricultural or grazing activities. For instance, changes in precipitation patterns could influence pasture growth, indirectly affecting the landscape. If natural vegetation encroaches due to changing climate conditions, this could lead to a shift away from traditional land use structures in favor of more resilient, natural cover types. Agroforestry and other sustainable agriculture techniques are relevant to this zone, supporting both community needs and conservation. Precision agriculture technologies could facilitate low-impact traditional practices that maintain the landscape’s historical character while adapting to changing environmental conditions.
Most LULC changes are projected within the buffer zone, where the probability of transitions exceeds 75% in all scenarios. The strict and regulatory regime zones are expected to remain relatively stable, with transition probabilities below 20% and 15%, respectively. Spatial concentration in the northern buffer area indicates substantial fragmentation and new land patch creation, suggesting that this area is particularly susceptible to anthropogenic pressures. By comparing current distributions (Figure 2C) with projected scenarios (Figures 11A–D), the buffer zone’s vulnerability to changes, especially under scenarios favoring agriculture, is clear.
4 Discussion
4.1 Past land use and land cover change
From 1982 to 1991, the stable extent of urban areas suggests minimal development, likely tied to economic stagnation in Eastern Europe (Zubok, 2021). In contrast, the period from 1991 to 2023 saw increased urbanization, with significant areas of agricultural land converted to urban uses, aligning with findings from Eastern Europe, where economic transitions enabled urban growth primarily at the expense of agricultural land (Mueller et al., 2021; Balsalobre-Lorente et al., 2023). Yet, this transition differs from urban expansion trends in Western Europe, where forest conversions are more prevalent (Auch et al., 2016; Fahey and Casali, 2017), emphasizing that land-use priorities can vary regionally.
The gradual expansion of non-irrigated arable land from 1982 to 1991, primarily converted from pastures and complex cultivation patterns, reflects European trends towards arable land optimization (Meyer-Aurich et al., 1998; Šarapatka and Štěrba, 1998; Goslee et al., 2013; Klein et al., 2013; Alkimim et al., 2015; Memmah et al., 2015; Ye et al., 2022). Yet, the radical increase in arable land from 1991 to 2023, exceeding 4,000 ha involving forest conversions, mirrors global agricultural intensification trends driven by economic and population growth (Rudel et al., 2009). Meanwhile, the land principally occupied by agriculture with significant areas of natural vegetation remained relatively stable, indicating a balanced relationship between agricultural practices and the conservation of natural vegetation. This period of stability suggests that economic conditions and policy frameworks favored the preservation of natural elements within agricultural landscapes (Della Rocca et al., 2021; Priyadarshana et al., 2024). However, the loss of complex cultivation patterns during this period indicates a shift toward monoculture, which can increase yields but reduce landscape diversity (Matsuyama, 1992; Plourde et al., 2013; Loh et al., 2022). While these findings may show a methodological limitation in LULC classifications that underrepresent mixed land-use impacts on biodiversity (Abson, 2019), this could also indicate the increasing demand for arable land driven by economic and population growth (Potapov et al., 2022). Meanwhile, the decline in forested areas during this period further highlights the encroachment of agricultural activities into natural environments (Wang et al., 2023). The period between 1991 and 2023 saw an intensified decline in complex cultivation patterns, with significant losses to non-irrigated arable land, pastures, and urban areas. The growing emphasis on maximizing productivity and economic returns led to the further reduction of complex cultivation patterns, with land increasingly allocated to monocultures rather than maintaining a diversity of crops and land uses (Matsuyama, 1992; Plourde et al., 2013; Loh et al., 2022). Substantial changes occurred in the land principally occupied by agriculture with significant areas of natural vegetation, with large-scale conversions to non-irrigated arable land, reflecting global trends towards agricultural intensification and the prioritization of crop production over the maintenance of natural vegetation (Rudel et al., 2009; Goslee et al., 2013; Li et al., 2022; Matsuyama, 1992; Plourde et al., 2013). In addition, the reduction in forests and transitional woodland-shrubs within these mixed-use areas further highlights the increasing pressure on natural habitats from agricultural expansion.
Between 1982 and 1991, coniferous forests saw minor net gains from mixed forests, possibly due to natural succession and economic incentives favoring conifers (Knoke et al., 2008; Yamaura et al., 2016). At the same time, broadleaf forests experienced a relatively modest net loss, mainly attributed to conversions to transitional woodland-shrubs. This shift likely reflects localized ecological succession processes and natural forest expansion into adjacent shrublands (Alday and Martínez-Ruiz, 2022; Frei et al., 2024). However, from 1991 to 2023, significant declines in broadleaf forests due to agricultural expansion support the view that intensified agriculture compromises forest resilience (Fahrig et al., 2019). While mixed forests in this period showed gains despite ongoing pressures, this resilience aligns with studies on the recovery potential of mixed systems under reduced disturbance (Bauhus et al., 2017). However, this study’s LULC model may not fully capture patch-scale dynamics, which limits insights into micro-habitat effects on biodiversity (Yamaura et al., 2016). Nonetheless, the substantial loss of mixed forests to transitional woodland-shrubs points on the dynamic nature of forest boundaries (Herlin, 2001) and suggests significant ecological changes in these areas, possibly due to disturbances and targeted restoration efforts. Transitional woodland-shrubs saw a net gain, primarily from the conversion of pastures, broadleaf forests, and coniferous forests. This gain likely reflects overgrazing and land degradation (Kairis et al., 2015; Lasanta et al., 2019) that created openings for shrub species to establish. Furthermore, the transition of some peat bogs to shrublands during 1982–1991 suggests that changing environmental conditions and human interventions were altering these ecosystems. From 1991 to 2023, a more pronounced decline in broadleaf forests occurred, with significant losses to various LULC categories, but particularly pasture. This large-scale conversion likely reflects increased agricultural expansion, driven by economic incentives prioritizing pasture over forest conservation (Wassenaar et al., 2007). At the same time, the conversion of some broadleaf forests to coniferous and mixed forests suggests a transformation in forest composition, possibly driven by selective logging or other disturbances favoring certain tree species (Verburg and van Eijk-Bos, 2003; Hart and Chen, 2008). The period saw a significant expansion of coniferous forests, driven by conversions from non-irrigated arable land, pastures, agricultural lands with natural vegetation, and other forest types. This expansion can be attributed to increased afforestation efforts (Forster et al., 2021), natural regeneration processes (Malcolm et al., 2001), and forest management practices promoting the establishment of coniferous species on former agricultural and degraded lands (Gupta et al., 2020). The continued gains from transitional woodland-shrubs further highlight the role of these areas as potential sites for conifer forest establishment. The period from 1991 to 2023 marked a dramatic reversal, with mixed forests experiencing a net gain despite continued losses to transitional woodland-shrubs. However, ongoing pressure from agricultural expansion and urban sprawl continued to threaten these forests (Auch et al., 2016; Fahey and Casali, 2017), leading to fragmentation and reduced ecological connectivity.
Peat bog expansion from 1982 to 1991 likely resulted from favorable hydrological conditions and limited land-use pressure, reflecting similar trends observed in stable wetland areas with low agricultural demand (Zubok, 2021). From 1991 to 2023, peat bogs faced extensive losses to transitional woodland-shrubs, underscoring vulnerability to climate and land-use changes that drive hydrological shifts and vegetation encroachment (Goud et al., 2018; Xue et al., 2023). The conversion of peat bogs to transitional woodland-shrubs observed in the study is consistent with broader concerns regarding peatland degradation under climate and land-use pressures (Gallego-Sala and Prentice, 2013; Goud et al., 2018). This transformation highlights a critical methodological challenge: distinguishing between natural successional changes and anthropogenic degradation in LULC classification, as these processes have differing ecological implications (Xue et al., 2023). This transformation, internal succession, suggests vulnerability within Latvia’s peatland ecosystems, requiring targeted management to prevent further habitat loss. Additionally, gains in forest areas align with global trends of forest recovery through natural succession, reforestation, or reduced agricultural intensity (Hansen et al., 2013).
Waterbody stability from 1982 to 1991, marked by only seasonal changes, suggests consistent hydrological conditions, contrasting with the dynamic gains and losses observed from 1991 to 2023. These changes likely branch out from altered precipitation and increased extraction for agriculture, a trend seen in many global studies (Micklin, 2007). The encroachment of transitional woodland-shrubs on waterbodies indicates a shift to terrestrial ecosystems, emphasizing that even slight water cover reductions can lead to rapid vegetative transitions, a factor not fully captured in standard LULC change analyses (Haghighi and Kløve, 2015).
4.2 Land cover fragmentation
Historical trends in fragmentation are closely linked to LULC policies across different periods, shifts in conservation and protection policies, and evolving awareness of fragmentation’s effects on biodiversity and ecosystem services. Additionally, climate change impacts are noteworthy, as various biomes respond differently to these changes, potentially influencing fragmentation patterns.
Between 1982 and 1991, the case study area underwent notable processes such as attrition, enlargement, and aggregation, which directly influenced the compactness ratio of LULC patches. Attrition, particularly prominent in the case study area, led to a decrease in the size of land patches, thereby reducing the overall compactness ratio. As patches became smaller and more fragmented, the land cover’s ability to maintain large, contiguous habitats diminished, leading to increased edge effects and reduced habitat connectivity. During this period, most LULC categories were characterized by a high degree of fragmentation. Low compactness indicates a landscape where LULC categories are dispersed and broken up rather than forming large, cohesive units. Such a fragmented landscape has significant ecological implications, including reduced resilience to environmental disturbances, challenges to species movement and migration, and increased vulnerability to edge effects, which can alter microclimatic conditions and disrupt ecological processes (Das and Nautiyal, 2004; Min et al., 2010).
The period from 1991 to 2023 saw further fragmentation, with processes like creation, attrition, and aggregation continuing to change the landscape. The expansion of discontinuous urban fabric, along with the reduction of pastures and other agricultural lands, contributed to a more fragmented and less compact landscape structure. The analysis indicates that the overall compactness ratio in 2023 is even lower than in previous years, particularly within the strict regime and regulatory regime zones. This reduction in compactness further aggravates the ecological challenges associated with fragmentation. Lower compactness in 2023 suggests that land patches are more irregular and dispersed, which significantly impacts ecological processes. Moreover, the decline in compactness ratio implies a reduction in core habitat areas, which are critical for the survival of many species. The increased edge effects and fragmentation can lead to the loss of interior species and a rise in edge species, often resulting in a shift in biodiversity and ecosystem services.
An essential aspect of analyzing landscape compactness and fragmentation is understanding the role of naturalness within each LULC category. Natural LULC classes, such as forests, peat bogs, and transitional woodland-shrubs, naturally support ecological stability by offering continuous habitats that sustain biodiversity and buffer against environmental disturbances. Moreover, these natural classes tend to be more resilient to fragmentation, as they usually form larger, more cohesive land cover patches that support interior species and retain core ecological functions. In contrast, semi-natural and anthropogenic classes, including pastures, non-irrigated arable land, and discontinuous urban fabric, contribute to fragmentation, as they are more susceptible to conversion and reconfiguration, thereby reducing overall compactness and impacting the continuity of natural landscapes.
From 1982 to 1991, processes like attrition and enlargement significantly affected natural LULC categories, especially within the buffer and regulatory zones. Attrition in forest and peat bog patches led to a decline in compactness, resulting in fragmented habitats with reduced core areas essential for interior species. This fragmentation compromises the naturalness of the landscape, as these smaller, isolated patches cannot sustain the ecological interactions necessary for a strong ecosystem. Conversely, aggregation of non-irrigated arable lands in the buffer zone indicates an increase in compactness for anthropogenic classes, potentially at the expense of natural cover types, thereby intensifying fragmentation.
The period from 1991 to 2023 saw continued fragmentation, with processes of creation and attrition altering both natural and anthropogenic LULC classes. The creation of new land cover patches within the buffer zone increased the heterogeneity of the landscape, further fragmenting natural habitats and disrupting habitat connectivity. Meanwhile, aggregation processes within the strict and regulatory regime zones allowed some semi-natural land covers to form larger patches, although at the cost of overall compactness in the natural landscape. By 2023, the compactness ratio had fallen further, especially within natural LULC categories, amplifying the ecological impacts of fragmentation, including increased edge effects, reduced core habitats, and heightened vulnerability to environmental pressures.
4.3 Future land use and land cover change
4.3.1 Urban areas
Scenario 1 presents a potential reversal of recent urban expansion trends, projecting a net loss in discontinuous urban fabric primarily due to transition to complex cultivation patterns. This scenario suggests a possible shift towards agricultural land use, possibly driven by a growing emphasis on sustainable agriculture or evolving economic conditions that favor farming.
In contrast, Scenario 2 projects a net gain in discontinuous urban fabric, primarily through conversions from complex cultivation patterns, pastures, and mixed forests. This scenario aligns with the ongoing, although moderate, urban growth trajectory observed in the area between 1991 and 2023, indicating continued demand for residential and commercial development (Mueller et al., 2021; Balsalobre-Lorente et al., 2023). The susceptibility of complex cultivation patterns to urban expansion in this scenario likely stems from their closeness to existing urban infrastructure or their lower economic value compared to more intensively used agricultural lands. This pattern mirrors broader global trends of urban encroachment into agricultural areas (Cai et al., 2013; Samiullah et al., 2019), driven by population growth and economic pressures.
Scenario 3 envisions a more balanced interaction between urban and agricultural land uses, with a modest net loss of discontinuous urban fabric, primarily to complex cultivation patterns. This scenario suggests a potential stabilization of urban growth, possibly due to effective land-use planning, demographic shifts, or other factors that mitigate the pressure for further urban expansion, such as implementation of greenbelts (Pourtaherian and Jaeger, 2022).
Finally, Scenario 4 forecasts a more pronounced net loss of 15 ha in discontinuous urban fabric, determined by transitions to non-irrigated arable land and complex cultivation patterns. This scenario points to a stronger trend towards agricultural expansion or intensification, which would be in favor of food production and shifts in agricultural policy towards sustainable practices.
4.3.2 Agricultural areas
Scenario 1 projects substantial gains in non-irrigated arable land, driven by conversions from pastures, complex cultivation patterns, and agricultural land with significant natural vegetation. However, these gains come at the cost of broadleaf, coniferous, and mixed forests, as well as transitional woodland-shrubs and peat bogs. This pattern of agricultural intensification reflects historical trends in areas facing growing demands for food production, where natural ecosystems are often sacrificed to expand arable land (Hatna and Bakker, 2011). Furthermore, the consistent losses in forest ecosystems and other natural habitats emphasize the environmental costs of unregulated agricultural expansion, including potential declines in biodiversity and ecosystem services (Zabel et al., 2019; Williams et al., 2021).
Scenario 2 presents a more balanced outlook, with agricultural gains offset by both losses and some gains in natural habitats, particularly in mixed forests. This scenario suggests a dynamic where agricultural expansion continues alongside some degree of forest recovery, which could be done with reforestation efforts or better-managed land transitions. However, despite these gains, the overall reduction in transitional woodland-shrubs and other natural areas signals ongoing pressure on land resources. This scenario aligns with studies indicating that even moderate agricultural expansion can significantly impact natural habitats (de Moraes et al., 2017; Kehoe et al., 2017), emphasizing the need for more sustainable land management practices.
Scenario 3 projects moderate changes, with smaller shifts in land use. Losses to forests and transitional woodland-shrubs are less pronounced, while gains from pastures, complex cultivation patterns, and agricultural land with natural vegetation suggest a slower pace of agricultural intensification. This scenario reflects a potential shift towards more sustainable agricultural practices that seek to balance productivity with ecological preservation. The modest changes in land use align with current trends in sustainable agriculture (Ragkos and Psychoudakis, 2009; Naqvi et al., 2023), where there is a growing emphasis on minimizing environmental impacts.
Scenario 4 indicates the most extensive losses across various land categories, including significant declines in broadleaf, coniferous, and mixed forests, transitional wood-land-shrubs, and peat bogs. These losses would be driven by the expansion of non-irrigated arable land and more intensive agricultural practices and highlight the potential for significant environmental degradation. The substantial conversion of peat bogs and forests to arable land is particularly concerning, as these areas are crucial for carbon sequestration, biodiversity, and ecosystem services.
4.3.3 Forested areas
Scenario 1 suggests significant gains across broadleaf, coniferous, and mixed forests, primarily led by the transition of non-irrigated arable land, pastures, and agricultural land with significant areas of natural vegetation. However, the associated losses to transitional woodland-shrubs and peat bogs in this scenario exhibit ongoing fragmentation and land-use pressures. The substantial gains in transitional woodland-shrubs from various sources, including forests and agricultural lands, suggest substantial shifts in land cover dynamics, which would potentially be driven by disturbances, land abandonment, or natural succession. The expansion of transitional woodland-shrubs, however, comes at a cost, with major losses observed in peat bogs, highlighting a concerning trend of peatland degradation potentially linked to drainage, land-use changes, or climate-induced droughts (Goud et al., 2018; Xue et al., 2023).
Scenario 2 presents a mixed outlook, where gains in broadleaf and coniferous forests are compensated by losses to mixed forests and transitional woodland-shrubs. The continued gains from agricultural land suggest opportunities for forest recovery, possibly through reforestation or natural regeneration. However, the expansion of transitional woodland-shrubs at the expense of forests and agricultural lands, coupled with substantial losses of peat bogs, point out the persistent pressure on these ecosystems. This scenario, similar to Scenario 1, highlights the ongoing economic and environmental shifts favoring less intensive land management, leading to natural succession towards shrub-dominated landscapes.
Scenario 3 offers a more balanced but cautious perspective, with moderate gains and losses across all forest types. The moderation in forest dynamics reflected here supports findings that forest cover change globally is frequently characterized by small-scale gains and losses rather than large-scale shifts (Hansen et al., 2013). This scenario underlines the need for balanced land-use planning that considers both ecological and economic priorities.
Scenario 4 indicates a more pessimistic expectation, with potential losses for all forest types to transitional woodland-shrubs, peat bogs, and other competing land uses. However, the small gains from agricultural land conversion suggest resistance in forest ecosystems, potentially through natural regeneration or afforestation efforts. The moderate gains in transitional woodland-shrubs from forests and agricultural lands further emphasize the ongoing forest-to-shrubland transitions (O’Connor et al., 2020), that would likely to be driven by disturbances, changes in land management, and natural succession processes.
4.3.4 Peat bogs
Scenario 1 anticipates a substantial gain for peat bogs, primarily driven by the conversion of transitional woodland-shrubs. This trend marks a reversal of past declines (1991–2023), where peat bogs were lost to shrub encroachment and land degradation. The gains from mixed and broadleaf forests further emphasize the potential for certain forested areas to change to wetland conditions conducive to peat formation. Improved land management practices, including rewetting initiatives and conservation-driven rewilding, would be contributing to this positive trend. The minor losses to waterbodies are negligible compared to the overall gains, suggesting that the expansion of peat bogs could outweigh any small-scale hydrological changes.
Scenario 2 presents a similar outlook, projecting continued gains from transitional woodland-shrubs, mixed forests, and broadleaf forests. The consistent pattern across Scenario1 and 2 implies a strong potential for large-scale peat bog restoration, which could be supported by concerted conservation efforts and favorable environmental conditions. As in Scenario 1, the minimal losses to waterbodies reinforce the overall positive trajectory, suggesting that the hydrological balance may increasingly favor wetland expansion.
Scenario 3 suggests a continued net gain for peat bogs, although on a smaller scale compared to the previous scenarios. The gains from transitional woodland-shrubs, mixed forests, and broadleaf forests point to a more moderate recovery scenario, which would reflect less intensive land management efforts or slower natural processes. Despite the smaller scale of gains, the consistent pattern of minimal losses to waterbodies suggests that these changes will have a minor impact on the overall recovery of peat bogs.
Scenario 4 projects the largest potential net gain for peat bogs, with significant contributions from transitional woodland-shrubs, mixed forests, coniferous forests, broadleaf forests, and non-irrigated arable land. This scenario displays a strong recovery of peat bogs, potentially driven by extensive rewilding or restoration initiatives focused on rewetting degraded peatlands and converting less suitable land types into peat bogs. The gains from non-irrigated arable land suggest that areas previously used for agriculture could be repurposed or allowed to transform into wetland conditions, further supporting the expansion of peat bogs. As in other scenarios, the minor losses to waterbodies would not be significant enough to detract from the overall positive trend.
4.3.5 Waterbodies
Scenario 1 suggests a potential net loss for waterbodies, with minimal gains from peat bogs and non-irrigated arable land being offset by significant losses to transitional woodland-shrubs. This scenario indicates that the expansion of transitional woodland-shrubs, likely driven by natural succession or land abandonment, could encroach upon existing waterbodies, reducing their extent. The small gains from peat bogs may reflect localized rewetting or restoration efforts, but these would be insufficient to counter-balance the losses to transitional woodland-shrubs. This trend shows the vulnerability of waterbodies to land cover changes that alter hydrological conditions, potentially leading to the encroachment of shrubs and other vegetation into areas previously dominated by open water.
Scenario 2 also predicts a net loss for waterbodies, with similar small gains from peat bogs, non-irrigated arable land, coniferous forests, and mixed forests, again offset by losses to transitional woodland-shrubs. The consistent pattern of small gains across various LULC categories suggests that while some areas may experience localized hydrological improvements, the overall trend is towards a reduction in waterbody area. The persistent loss to transitional woodland-shrubs underscores the constant pressure from shrubland expansion into water-dominated settings, supporting the need for careful management of transitional woodland-shrubs to prevent further encroachment into waterbodies.
Scenario 3 projects a more significant net loss for waterbodies, primarily driven by conversions to transitional woodland-shrubs, which outweigh minor gains from peat bogs and non-irrigated arable land. This scenario suggests that, despite efforts to maintain or restore waterbodies, the encroachment of transitional woodland-shrubs could continue to reduce their extent. The smaller scale of gains in this scenario indicates that the factors contributing to waterbody loss—such as land degradation, climate change, or insufficient water management practice—might be more pronounced (Biggs et al., 2017; Szpakowska et al., 2022).
Scenario 4 presents a more positive expectation, with a potential net gain in waterbodies. In this scenario, losses to transitional woodland-shrubs might be counterbalanced by gains from peat bogs, complex cultivation patterns, and non-irrigated arable land. This scenario suggests potential for the expansion of waterbodies through targeted restoration efforts. The gains from peat bogs suggest that future rewetting and restoration initiatives could play a key role in supporting waterbody expansion, particularly in areas where peatlands are linked to nearby aquatic ecosystems. However, the continued pressure from transitional woodland-shrubs shows that even in a more favorable scenario, waterbodies would remain vulnerable to encroachment and require active management to maintain their extent.
4.4 Effectiveness of conservation zoning
The results suggest LULC changes in the strict regime zone, which is legally designated to support the natural development of ecosystems by prohibiting economic activities, construction, and general access. This regulatory intent implies a high level of protection meant to shield the zone from external influences and human impact. However, observed LULC shifts, such as transitions in forest cover and encroachment by transitional woodland-shrub, raise questions about the zone’s ability to fulfill its strict conservation directive under current pressures. Since access and economic activities are restricted to protect this zone’s ecosystems, any significant LULC changes could suggest either indirect anthropogenic impacts, such as edge effects or spillovers from neighboring zones, or natural succession processes. For instance, the encroachment of transitional woodland-shrub areas indicates that factors beyond human activity—like climate effects and ecosystem dynamics—are influencing landscape changes. These insights underline the complexity of maintaining static zoning in highly protected areas and suggest a need for monitoring natural shifts to determine if stricter perimeter controls or adaptive management strategies might help mitigate indirect pressures.
The regulatory regime zone of the Teiči Strict Nature Reserve is designed to balance conservation with limited, purposeful human activities to promote biodiversity, ecosystem study, and environmental awareness. This outline permits specific conservation actions and limited infrastructure development while restricting activities such as use of pesticides and fertilizers in agriculture and forestry. Given this regulatory intent, any observed or projected LULC changes should ideally align with minimal anthropogenic impact and should support the zone’s objectives of biodiversity conservation and ecosystem integrity. However, the results show significant shifts in land cover, such as increases in transitional woodland-shrub and encroachment by human activities, hence this may indicate regulatory challenges and external pressures that could undermine conservation goals. For instance, while some forestry is permitted for maintenance and biodiversity purposes, any large-scale vegetation changes or land cover fragmentation suggests that current land management practices and external factors are influencing the landscape beyond the intended minimal impact.
The nature park zone is regulated to preserve traditional environmental management while balancing conservation, education, and community interests. This zone prohibits intensive modifications, such as land use changes, final tree felling, or alterations to the hydrological regime, ensuring that traditional land uses and natural landscapes remain largely intact. The zone’s restrictions on harmful chemical usage, non-native species introduction, and mineral extraction also reflect its goal of maintaining ecological stability with minimal disruption. In light of these regulations, observed and projected LULC changes in this zone show stability, with minimal shifts that would suggest alignment with traditional land use practices. The slight increase in transitional woodland-shrub and coniferous forest areas, along with the reduction in pastures and peat bogs, may reflect natural successional processes or indirect human impact within this zone. Since the zone’s purpose is to preserve traditional land management practices alongside conservation, these changes could signify shifts away from traditional pasture management. This gradual increase in woody vegetation might align with succession dynamics due to limited active land management interventions. Given the nature park zone’s role in balancing conservation with local community interests, these shifts could warrant periodic assessment to determine if additional management actions, like controlled pasture maintenance or targeted shrub removal, are needed to maintain traditional land use practices. Such adjustments could help retain the zone’s intended cultural landscape, fulfilling both biodiversity conservation and socio-cultural preservation.
The buffer zone surrounding the Teiči Strict Nature Reserve is designed to mitigate economic and anthropogenic impacts on the reserve’s ecosystems by establishing specific regulations. This zone restricts intensive activities, such as hunting of certain bird species, altering water regimes, and using pesticides, while permitting infrastructure development and land use changes only with strict authorization. The buffer zone serves as a transitional area, helping to shield core conservation areas from external pressures by controlling high-impact activities and facilitating regulated development that adheres to environmental assessments. The notable expansion of urban areas and intensification of agriculture within the buffer zone suggests that static zoning may be insufficient to address ongoing land-use pressures. Fragmentation and patch reduction highlight potential vulnerabilities to external pressures, as fixed regulations alone may not effectively curb encroachment. This situation points to a need for adaptable zoning that can respond to observed trends, such as reclassifying high-risk areas for stricter protection or implementing new buffer strategies to prevent habitat loss and maintain connectivity. Projected future changes suggest that up to 75% of the buffer zone may experience LULC transitions by 2064, depending on external pressures and legislative responses. This projection indicates that static zoning may struggle to adapt to such high levels of transition. An adaptable zoning strategy that allows for periodic reassessment and reclassification could help manage these shifts, potentially adjusting boundaries or permitted activities to better match changing ecological and anthropogenic dynamics. Adaptive zoning could support conservation goals by periodically evaluating the effectiveness of the buffer zone in minimizing human impact on the core reserve. For example, adaptable zoning could introduce flexible boundaries that expand or contract in response to land-use changes, seasonal variations, or climate impacts. Additionally, it could adjust regulations to control expanding urban or agricultural activities within the buffer, possibly by prioritizing restoration in newly impacted areas. The increasing urban and agricultural encroachment on the buffer zone threatens the long-term ecological resilience of the reserve, as fragmentation and edge effects could impact species and habitat stability in the core conservation areas. Adaptable zoning could help by allowing responsive measures such as creating stricter land-use restrictions in areas undergoing rapid change or applying conservation buffers to reduce edge effects. Adaptable zoning also enables a more flexible approach to enforcement. Regular monitoring of LULC changes would allow authorities to respond dynamically, strengthening protection activities as pressures intensify or modifying land-use allowances in low-impact areas. For instance, adaptable zoning could limit or permit specific activities based on seasonal or yearly observations, such as restricting certain land uses during critical periods for biodiversity.
4.5 Future of conservation zoning
The historical and projected LULC changes in the Teiči Strict Nature Reserve, particularly the expansion of transitional woodland-shrubs and potential loss of peat bogs and waterbodies, raise important questions about the effectiveness of the existing conservation zoning. Static conservation strategies, reliant on fixed boundaries and land-use designations, are not adequately addressing the dynamic nature of these ecosystems and neither are they addressing the ongoing pressure from LULC change and climate change.
Adaptive management originated in literature in the mid-1970s, prompted by the recognition of the significant uncertainty inherent in management and dissatisfaction with efforts to utilize modeling for information integration and prediction (Rist et al., 2012). Several studies have accentuated the need for adaptive management and flexible conservation zoning in response to changing environmental conditions and land-use pressures. For instance, Hoekstra et al. (2005) highlighted the importance of integrating ecological dynamics and connectivity into conservation planning, recognizing that ecosystems are not static entities but rather constantly evolving in response to a range of factors. Margules and Pressey (2000) further argued that conservation planning should be an iterative process, allowing for adjustments in zoning and management strategies as current information becomes available and conditions change.
Despite being established to safeguard natural ecosystems from anthropogenic transformations, many protected areas continue to experience human-caused changes. Consequently, assessing the variation in protection afforded by different forms of protected area restrictions is a prevalent technique (Schmitz et al., 2023). In the context of the Teiči Strict Nature Reserve, the observed and projected LULC changes suggest that a reevaluation of the existing conservation zoning may be warranted. The expansion of transitional woodland-shrubs, while potentially beneficial for some species, could also pose a threat to peat bogs and waterbodies, which are crucial for biodiversity and ecosystem services. A static zoning approach may not adequately address these shifting dynamics, potentially leading to unintended consequences for conservation goals. Nonetheless, climatic and land-use changes undermine the effectiveness of protected areas globally, particularly in biodiverse areas (Liu et al., 2023), and the Teiči Strict Nature Reserve is no exception.
The development and implementation of successful protected area management partially relies on recognizing areas susceptible to conversion (Melillo et al., 2016; Siegel et al., 2022). Hence, the reevaluation of the conservation zoning should consider the dynamic nature of all LULC categories within the case study area, including natural succession processes and the potential impacts of climate change. Furthermore, maintaining and enhancing ecological connectivity between different habitat types is crucial for supporting biodiversity and ecosystem resilience in the future. It is essential to adopt an adaptive management approach that allows for adjustments in conservation zoning and management strategies based on ongoing monitoring and evaluation. Furthermore, involving local communities, scientists, and other stakeholders in this reevaluation process will be crucial to ensure that diverse perspectives are considered and that the zoning reflects both nature conservation and socio-economic needs. Lastly, employing predictive models (Gallardo and Martinez-Vega, 2018; Siegel et al., 2022) to assess the potential impacts of different zoning scenarios on biodiversity, ecosystem services, and land-use conflicts is vital for informing decision-making and promoting more effective conservation outcomes.
4.6 Limitations and potential shortcomings
One limitation of this study is the reliance on historical satellite imagery for LULC classification. While remote sensing provides valuable data, classification errors may occur due to sensor resolution or atmospheric conditions, potentially affecting the accuracy of the LULC maps. Additionally, the focus on the RCP 4.5 climate scenario may not account for more extreme future climate trajectories, which could alter the LULC dynamics projected in this study. Future work should consider multiple climate scenarios to better capture the range of possible LULC changes.
Another potential shortcoming lies in the simplification of land-use categories, particularly regarding agricultural land. The study groups various agricultural activities into broad classes, which may overlook the nuanced differences between types of farming practices, such as intensive monoculture versus sustainable agroforestry. These distinctions could affect how future LULC scenarios are interpreted, particularly in terms of conservation zoning and its impact on biodiversity.
4.7 Integration into the current understanding and advancing the field
This study contributes to the growing body of research on land-use dynamics in protected areas by highlighting the significant pressures that agricultural expansion and urbanization exert on conservation zones. In protected areas, land cover dynamics can vary based on protection levels, influencing LULC stability and resilience. Shahi et al. (2020) used remote sensing and modeling to monitor LULC changes within a protected area, highlighting how different levels of protection affect land cover persistence and transition patterns. Recent studies highlight the role that protected areas play in shaping land-use change dynamics, especially in biodiversity hotspots. For instance, Schmitz et al. (2023) found that the effectiveness of protected areas in controlling LULC changes varies significantly depending on the degree of protection, with stricter zones showing better resilience against encroachment. The findings align with studies that call for a reevaluation of static conservation zoning (Margules and Pressey, 2000; Hoekstra et al., 2005). As land-use patterns continue to evolve, static boundaries may no longer provide sufficient protection for vulnerable ecosystems such as peat bogs and forests.
Predictive models that incorporate spatiotemporal dependencies provide improved accuracy in LULC simulations. Liu et al. (2024) introduced a model that captures these dependencies, making it particularly useful for conservation planning where land cover transitions must be anticipated with greater precision. By integrating predictive modeling with historical LULC data, this study advances the field of conservation science by providing a framework for understanding how future land-use changes could impact conservation efforts. This research emphasizes the need for dynamic conservation zoning that can adapt to shifting land-use patterns and environmental conditions, thus aligning with recent calls for more flexible management approaches in protected areas.
4.8 Future directions and theoretical postulations
Future research should explore the impact of different climate scenarios on LULC dynamics, particularly under more extreme conditions such as those projected by the RCP 8.5 scenario. This would provide a broader understanding of how climate change may exacerbate land-use pressures on conservation zones. Additionally, future studies could incorporate socio-economic factors more explicitly, such as local community involvement in land management and the role of policy in shaping land-use patterns (Pretty and Smith, 2004). This would offer a more comprehensive approach to understanding the drivers of LULC change.
The use of advanced machine learning algorithms for LULC modeling, including deep learning techniques, could improve the accuracy of future projections by better capturing the complexity of land-use interactions (Acuña-Alonso et al., 2024). Additionally, future studies could examine the role of agroecological practices in mitigating the negative impacts of agricultural expansion on conservation zones. By promoting sustainable farming methods, it may be possible to balance food production with conservation objectives.
Lastly, future research could assess the hypothesis that adaptive conservation zoning, coupled with real-time LULC monitoring, leads to better outcomes for biodiversity and ecosystem services compared to static zoning. This could be achieved by implementing pilot programs that integrate dynamic zoning into existing protected areas and monitoring their ecological and social impacts over time.
5 Conclusion
The analysis of LULC changes from 1982 to 2023 highlights substantial shifts in land use within the Teiči Strict Nature Reserve, including a marked increase in urban and agricultural areas, as well as significant transitions in forested and peat bog areas. These findings underscore the impact of socio-economic drivers, such as economic development and population growth, on natural landscapes. For example, urban expansion has been one of the most significant changes, primarily at the expense of agricultural lands.
The results reveal that current zoning regulations may be insufficient to prevent the adverse effects of ongoing pressures on critical habitats. Notably, fragmentation and loss of compactness in core conservation zones, such as the strict regime and regulatory regime zones, indicate increased exposure to edge effects and reduced habitat connectivity, which could undermine the reserve’s ecological integrity.
Projections up to 2064 indicate that LULC changes are likely to continue, with the most significant transitions expected in the buffer zone. The scenarios suggest that without adaptive measures, further encroachment by urban and agricultural land uses will challenge the reserve’s conservation goals. Scenario 3, which prioritizes agricultural expansion, shows the highest potential for land-use intensification, emphasizing the need for proactive management interventions in the buffer area.
Given the projected pressures, adaptive zoning appears more suitable than static zoning for managing LULC dynamics within the reserve. The study advocates for a flexible approach that can respond to real-time changes and account for ecological succession, which could help mitigate the negative effects of urban and agricultural expansion. The success of adaptive management would rely on continuous LULC monitoring and responsive regulatory adjustments in line with observed trends.
The results illustrate the value of predictive models in assessing the potential impacts of different zoning policies. By integrating historical data with scenario modeling, this study demonstrates how varying legislative approaches can influence LULC dynamics and conservation outcomes. Predictive modeling can thus serve as a valuable tool for conservation planning, allowing stakeholders to anticipate land-use conflicts and prioritize areas for stricter management. The value of predictive modeling is demonstrated by the study’s use of scenarios to illustrate possible zoning impacts.
Data availability statement
The raw data supporting the conclusions of this article will be made available by the authors, without undue reservation.
Author contributions
JK: Conceptualization, Formal Analysis, Investigation, Methodology, Resources, Software, Validation, Visualization, Writing–original draft, Writing–review and editing. MK: Conceptualization, Data curation, Funding acquisition, Project administration, Supervision, Validation, Writing–original draft, Writing–review and editing. KS: Visualization, Writing–original draft, Writing–review and editing.
Funding
The author(s) declare that financial support was received for the research, authorship, and/or publication of this article. This research was funded by the Horizon Europe project ‘Towards Sustainable Land-use Strategies in the Context of Climate Change and Biodiversity Challenges in Europe’ (Grant Agreement No: 101081307).
Conflict of interest
The authors declare that the research was conducted in the absence of any commercial or financial relationships that could be construed as a potential conflict of interest.
Publisher’s note
All claims expressed in this article are solely those of the authors and do not necessarily represent those of their affiliated organizations, or those of the publisher, the editors and the reviewers. Any product that may be evaluated in this article, or claim that may be made by its manufacturer, is not guaranteed or endorsed by the publisher.
References
Abson, D. J. (2019). “The economic drivers and consequences of agricultural specialization,” in Agroecosystem diversity. Reconciling contemporary agriculture and environmental quality. Editors G. Lemaire, P. C. De Faccio Carvalho, S. Kronberg, and S. Recous (Cambridge, United States: Academic Press), 301–315. doi:10.1016/B978-0-12-811050-8.00019-4
Acuña-Alonso, C., Garcia-Ontiyuelo, M., Barba-Barragans, D., and Alvarez, X. (2024). Development of a convolutional neural network to accurately detect land use and land cover. MethodsX 12, 102719. doi:10.1016/j.mex.2024.102719
Adam, M. R., El-badawy, T., Shamsudin, M. S., Hubadillah, S. K., Jamalludin, M. R., Aziz, M. H. A., et al. (2024). “Deforestation influence on climate change,” in Advances and technology development in greenhouse gases: emission, capture and conversion. Editors M. R. Rahimpour, M. A. Makarem, and M. Meshksar (Amsterdam, Netherlands: Elsevier), 169–183. doi:10.1016/B978-0-443-19066-7.00010-2
Alday, J. G., and Martínez-Ruiz, C. (2022). Expansion of naturally regenerated forest. Forests 13, 456. doi:10.3390/f13030456
Alkimim, A., Sparovek, G., and Clarke, K. C. (2015). Converting Brazil's pastures to cropland: an alternative way to meet sugarcane demand and to spare forestlands. Appl. Geogr. 62, 75–84. doi:10.1016/j.apgeog.2015.04.008
Ansari, A. (2019). Predicting spatial habitat changes of haftad-gholleh protected area in markazi province, Iran. Iran. J. App. Ecol. 8 (2), 31–46. doi:10.29252/ijae.8.2.31
Auch, R. F., Drummond, M. A., Xian, G., Sayler, K. L., Acevedo, W., and Taylor, J. L. (2016). Regional differences in upland forest to developed (urban) land cover conversions in the conterminous U.S., 1973-2011. Forests 7, 132. doi:10.3390/f7070132
Balsalobre-Lorente, D., Shahbaz, M., Murshed, M., and Nuta, F. M. (2023). Environmental impact of globalization: the case of central and Eastern European emerging economies. J. Environ. Manage. 341, 118018. doi:10.1016/j.jenvman.2023.118018
Bauhus, J., Forrester, D. I., Gardiner, B., Jactel, H., Vallejo, R., and Pretzsch, H. (2017). “Ecological stability of mixed-species forests,” in Mixed-species forests. Editors H. Pretzsch, D. Forrester, and J. Bauhus (Berlin, Germany: Springer), 337–383. doi:10.1007/978-3-662-54553-9_7
Bengtsson, J., Angelstam, P., Elmqvist, T., Emanuelsson, U., Folke, C., Ihse, M., et al. (2003). Reserves, resilience, and dynamic landscapes. Ambio 32 (6), 389–396. doi:10.1579/0044-7447-32.6.389
Bengtsson, J., Angelstam, P., Elmqvist, T., Emanuelsson, U., Folke, C., Ihse, M., et al. (2021). Reserves, resilience, and dynamic landscapes 20 years later. Ambio 50, 962–966. doi:10.1007/s13280-020-01477-8
Bharathi, D., Karthi, R., and Geetha, P. (2021). “Building a land use and land cover (LULC) classifier using decadal maps,” in Proceedings of international conference on intelligent computing, information and control systems. Advances in intelligent systems and computing. Editors A. P. Pandian, R. Palanisamy, and K. Ntalianis (Singapore: Springer), 147–159. doi:10.1007/978-981-15-8443-5_12
Biggs, J., von Fumetti, S., and Kelly-Quinn, M. (2017). The importance of small waterbodies for biodiversity and ecosystem services: implications for policy makers. Hydrobiologia 793, 3–39. doi:10.1007/s10750-016-3007-0
Biswas, G., Sengupta, A., Alfaisal, F. M., Alam, S., Alharbi, R. S., and Jeon, B.-H. (2023). Evaluating the effects of landscape fragmentation on ecosystem services: a three-decade perspective. Ecol. Inf. 77, 102283. doi:10.1016/j.ecoinf.2023.102283
Bo, W., Yuxia, W., and Wu, X. (2023). Impact of land use compactness on the habitat services from green infrastructure in Wuhan, China. Urban For. Urban Green. 84, 127927. doi:10.1016/j.ufug.2023.127927
Boussema, S. B. F., Allouche, F. K., Ajmi, R., Chaanabe, B., and Gad, A.-A. (2023). Assessing and monitoring the effects of land cover changes in biodiversity. Case study: mediterranean coastal region, Sousse, Tunisia. J. Remote Sens. Space Sci. 26 (1), 185–196. doi:10.1016/j.ejrs.2023.01.002
Cai, H., Yang, X., and Xu, X. (2013). Spatiotemporal patterns of urban encroachment on cropland and its impacts on potential agricultural productivity in China. Remote Sens. 5, 6443–6460. doi:10.3390/rs5126443
Chauvier-Mendes, Y., Pollock, L. J., Verburg, P. H., Karger, D. N., Lavergne, S., Zimmermann, N. E., et al. (2024). Transnational conservation to anticipate future plant shifts in Europe. Nat. Ecol. Evol. 8, 454–466. doi:10.1038/s41559-023-02287-3
Chen, W., Gu, T., Xiang, J., Luo, T., and Zeng, J. (2023a). Assessing the conservation effectiveness of national nature reserves in China. Appl. Geogr. 161, 103125. doi:10.1016/j.apgeog.2023.103125
Chen, X., Yu, L., Cao, Y., Xu, Y., Zhao, Z., Zhuang, Y., et al. (2023b). Habitat quality dynamics in China's first group of national parks in recent four decades: evidence from land use and land cover changes. J. Environ. Manage. 325, 116505. doi:10.1016/j.jenvman.2022.116505
Cheng, A. T., Sims, K. R. E., and Yi, Y. (2023). Economic development and conservation impacts of China's nature reserves. J. Environ. Econ. Manage. 121, 102848. doi:10.1016/j.jeem.2023.102848
Chowdhury, M. S. (2024). Comparison of accuracy and reliability of random forest, support vector machine, artificial neural network, and maximum likelihood method in land use/cover classification of urban setting. Environ. Challenges 14, 100800. doi:10.1016/j.envc.2023.100800
Climate portal (2024). Climate projections. Available at: https://klimats.meteo.lv/(Accessed August 16, 2024).
Copernicus (2024). CORINE land cover nomenclature. Available at: https://land.copernicus.eu/content/corine-land-cover-nomenclature-guidelines/html/(Accessed August 16, 2024).
Das, J. K., and Nautiyal, J. (2004). Forest variability index: a vector quantifying forest stand diversity and forest compactness. For. Policy Econ. 6 (3-4), 271–288. doi:10.1016/j.forpol.2004.03.003
Della Rocca, F., Venturo, A., Milanesi, P., and Bracco, F. (2021). Effects of natural and seminatural elements on the composition and dispersion of carabid beetles inhabiting an agroecosystem in Northern Italy. Ecol. Evol. 11 (15), 10526–10537. doi:10.1002/ece3.7857
de Moraes, M. C. P., de Mello, K., and Toppa, R. H. (2017). Protected areas and agricultural expansion: biodiversity conservation versus economic growth in the Southeast of Brazil. J. Environ. Manage. 188, 73–84. doi:10.1016/j.jenvman.2016.11.075
Dietz, J., Treydte, A. J., and Lippe, M. (2023). Exploring the future of Kafue National Park, Zambia: scenario-based land use and land cover modelling to understand drivers and impacts of deforestation. Land Use Policy 126, 106535. doi:10.1016/j.landusepol.2023.106535
Eastman, J. R., and Toledano, J. (2018). “A short presentation of the land change modeler (LCM),” in geomatic approaches for modeling land change scenarios,” in Lecture notes in geoinformation and cartography. Editors M. Camacho Olmedo, M. Paegelow, J. F. Mas, and F. Escobar (Cham: Springer), 499–505.
Envirotech ltd (2024). GIS Latvia 10.2 database. Available at: https://www.gisbaltic.eu/content/dam/distributor-restricted/gisbaltic-eu/esri-lv/apmacibas/gislatvija10.2.zip (Accessed August 16, 2024).
EPSG.io (2024). WGS 84/UTM zone 35N. Available at: https://epsg.io/32635 (Accessed August 16, 2024).
Fahey, R. T., and Casali, M. (2017). Distribution of forest ecosystems over two centuries in a highly urbanized landscape. Land. Urban Plan. 164, 13–24. doi:10.1016/j.landurbplan.2017.03.008
Fahrig, L., Arroyo-Rodriguez, V., Bennet, J. R., Boucher-Lolonde, V., Cazetta, E., Currie, D. J., et al. (2019). Is habitat fragmentation bad for biodiversity? Biol. Conserv. 230, 179–186. doi:10.1016/j.biocon.2018.12.026
Fogang, L. F., Tiomo, I. F., Kamga, B. Y., Kpoumie, H. M., Nkondjoua, A. D. T., Nguetsop, V. F., et al. (2023). Predicting land use/land cover changes in the Santchou Wildlife Reserve (Santchou, West-Cameroon) using a CA-Markov model. Trees For. People 14, 100438. doi:10.1016/j.tfp.2023.100438
Forster, E. J., Healey, J. R., Dymond, C., and Styles, D. (2021). Commercial afforestation can deliver effective climate change mitigation under multiple decarbonisation pathways. Nat. Commun. 12 (1), 3831. doi:10.1038/s41467-021-24084-x
Frei, T., Espelta, J. M., Górriz-Mifsud, E., Hampe, A., Lefevre, F., Martin-Fores, I., et al. (2024). Can natural forest expansion contribute to Europe's restoration policy agenda? An interdisciplinary assessment. Ambio 53, 34–45. doi:10.1007/s13280-023-01924-2
Gallardo, M., and Martinez-Vega, J. (2018). “Modeling land-use scenarios in protected areas of an urban region in Spain,” in Geomatic approaches for modeling land change scenarios. Editors M. T. Camacho Olmedo, M. Paegelow, J.-F. Mas, and F. Escobar (Cham: Springer), 307–329.
Gallego-Sala, A., and Colin Prentice, I. (2013). Blanket peat biome endangered by climate change. Nat. Clim. Change 3, 152–155. doi:10.1038/nclimate1672
Ganesh, B., Vincent, S., Pathan, S., and Benitez, S. R. G. (2023). Utilizing LANDSAT data and the maximum likelihood classifier for analysing land use patterns in shimoga, Karnataka. J. Phys. 2571 (1), 012001. doi:10.1088/1742-6596/2571/1/012001
Garden, J. G., O’Donnell, T., and Catterall, C. P. (2015). Changing habitat areas and static reserves: challenges to species protection under climate change. Landsc. Ecol. 30, 1959–1973. doi:10.1007/s10980-015-0223-3
Goslee, S. C., Veith, T. L., Skinner, R. H., and Comas, L. H. (2013). Optimizing ecosystem function by manipulating pasture community composition. Basic Appl. Ecol. 14 (8), 630–641. doi:10.1016/j.baae.2013.09.009
Goud, E. M., Watt, C., and Moore, T. R. (2018). Plant community composition along a peatland margin follows alternate successional pathways after hydrologic disturbance. Acta Oecol. 91, 65–72. doi:10.1016/j.actao.2018.06.006
Guo, J., Li, F. Y., Tuvshintogtokh, I., Niu, J., Li, H., Shen, B., et al. (2024). Past dynamics and future prediction of the impacts of land use cover change and climate change on landscape ecological risk across the Mongolian plateau. J. Environ. Manage. 355, 120365. doi:10.1016/j.jenvman.2024.120365
Guo, X., and Fang, C. (2021). Integrated land use change related carbon source/sink examination in jiangsu province. Land 10, 1310. doi:10.3390/land10121310
Gupta, S. R., Dagar, J. C., and Teketay, D. (2020). “Agroforestry for rehabilitation of degraded landscapes: achieving livelihood and environmental security,” in Agroforestry for degraded landscapes. Editors J. C. Dagar, S. R. Gupta, and D. Teketay (Singapore City, Singapore: Springer), 23–68. doi:10.1007/978-981-15-4136-0_2
Haghighi, A. T., and Kløve, B. (2015). A sensitivity analysis of lake water level response to changes in climate and river regimes. Limnologica 51, 118–130. doi:10.1016/j.limno.2015.02.001
Hannah, L., Midgley, G. F., Lovejoy, T., Bond, W. J., Nush, M., Lovet, J. C., et al. (2002). Conservation of biodiversity in a changing climate. Conserv. Biol. 16 (1), 264–268. doi:10.1046/j.1523-1739.2002.00465.x
Hansen, M. C., Potapov, P. V., Moore, R., Hancher, M., Turubanova, S. A., Tyukavina, A., et al. (2013). High-resolution global maps of 21st-century forest cover change. Science 342 (6160), 850–853. doi:10.1126/science.1244693
Hart, S. A., and Chen, H. Y. N. (2008). Fire, logging, and overstory affect understory abundance, diversity, and composition in boreal forest. Ecol. Monogr. 78 (1), 123–140. doi:10.1890/06-2140.1
Hatna, E., and Bakker, M. M. (2011). Abandonment and expansion of arable land in Europe. Ecosystems 14, 720–731. doi:10.1007/s10021-011-9441-y
Herlin, I. S. (2001). Approaches to forest edges as dynamic structures and functional concepts. Land. Res. 26 (1), 27–43. doi:10.1080/01426390120024466
Hoekstra, J. M., Boucher, T. M., Ricketts, T. H., and Roberts, C. (2005). Confronting a biome crisis: global disparities of habitat loss and protection. Ecol. Lett. 8 (1), 23–29. doi:10.1111/j.1461-0248.2004.00686.x
Hu, N., Wang, G., Ma, Z., Zhao, M., Yuan, Y., Zhang, T., et al. (2024a). Multi-scenario simulation of land use dynamics and ecological risk: a case study of the Liaohe estuary national wetland reserve using PLUS-Markov and PSR models. Front. Environ. Sci. 12, 1361970. doi:10.3389/fenvs.2024.1361970
Hu, Y., Zhong, L., and Qi, W. (2024b). Identification and analysis of conservation gap of national nature reserves in China. Ecol. Indic. 158, 111525. doi:10.1016/j.ecolind.2023.111525
Humboldt State University (2014). Natural and false color composites. Available at: https://gsp.humboldt.edu/olm/Courses/GSP_216/lessons/composites.html (Accessed August 16, 2024).
INSPIRE Knowledge Base (2024). INSPIRE data specification on land cover – technical guidelines. Available at: https://knowledge-base.inspire.ec.europa.eu/publications/inspire-data-specification-land-cover-technical-guidelines_en (Accessed August 16, 2024).
Kairis, O., Karavitis, C., Salvati, L., Kounalaki, A., and Kosmas, K. (2015). Exploring the impact of overgrazing on soil erosion and land degradation in a dry mediterranean agro-forest landscape (crete, Greece). Arid. Land Res. Manage. 29 (3), 360–374. doi:10.1080/15324982.2014.968691
Karr, J. R., Larson, E. R., and Chu, E. W. (2022). Ecological integrity is both real and valuable. Conserv. Sci. Pract. 4 (2)–e583. doi:10.1111/csp2.583
Kehoe, L., Romero-Muñoz, A., Polaina, E., Estes, L., Kreft, H., and Kuemmerle, T. (2017). Biodiversity at risk under future cropland expansion and intensification. Nat. Ecol. Evol. 1, 1129–1135. doi:10.1038/s41559-017-0234-3
Klein, T., Holzkämper, A., Calanca, P., Seppelt, R., and Fuhrer, J. (2013). Adapting agricultural land management to climate change: a regional multi-objective optimization approach. Landsc. Ecol. 28, 2029–2047. doi:10.1007/s10980-013-9939-0
Knoke, T., Ammer, C., Stimm, B., and Mosandl, R. (2008). Admixing broadleaved to coniferous tree species: a review on yield, ecological stability, and economics. Eur. J. For. Res. 127, 89–101. doi:10.1007/s10342-007-0186-2
Kumar, V., and Agrawal, S. (2023). A multi-layer perceptron–Markov chain based LULC change analysis and prediction using remote sensing data in Prayagraj district, India. Environ. Monit. Assess. 195, 619. doi:10.1007/s10661-023-11205-w
Labs, C. (2024). TerrSet 2020 geospatial monitoring and modeling software. Available at: https://clarklabs.org/terrset/(Accessed August 16, 2024).
Lāce, I., and Krauklis, K. (2021). Zinātniskais pamatojums Teiču dabas rezervāta ārējās aizsargjoslas noteikšanai (Scientific basis for determining the buffer zone of the Teiči Nature Reserve – in Latvian). Riga: Riga Technical University.
Lahon, D., Sahariah, D., Debnath, J., Nath, N., Meraj, G., Kumar, P., et al. (2023). Assessment of ecosystem service value in response to LULC changes using geospatial techniques: a case study in the merbil wetland of the brahmaputra valley, Assam, India. ISPRS Int. J. Geo-Inf. 12, 165. doi:10.3390/ijgi12040165
Lamounier, W. L., dos Santos, J. S., Rodrigues, E. L., and Drumond, M. A. (2024). Defining priority areas for conservation based on multi-species functional connectivity. Biol. Conserv. 290, 110438. doi:10.1016/j.biocon.2023.110438
Lasanta, T., Arnáez, J., and Nadal-Romero, E. (2019). Soil degradation, restoration, and management in abandoned and afforested lands. Adv. Chem. Pollut. 4, 71–117. doi:10.1016/bs.apmp.2019.07.002
Lee, H., Guy, M., Andelman, S., Araújo, M., Hughes, G., Martinez-Meyer, E., et al. (2007). Protected area needs in a changing climate. Front. Ecol. Environ. 5 (3), 131–138. doi:10.1890/1540-9295(2007)5[131:paniac]2.0.co;2
Legal Acts of the Republic of Latvia (2024). Teiči strict nature reserve law. Available at: https://likumi.lv/ta/en/en/id/176245 (Accessed August 16, 2024).
Legutko-Kobus, P., Nowak, M., Petrisor, A.-I., Bărbulescu, D., Craciun, C., and Gârjoabă, A.-I. (2023). Protection of environmental and natural values of urban areas against investment pressure: a case study of Romania and Poland. Land 12, 245. doi:10.3390/land12010245
Li, C., Jin, X., Zhi, J., Luo, Y., Li, M., and Liu, W. (2022). Evaluating whether farmland consolidation is a feasible way to achieve a balance of potential crop production in southeastern coastal China. Land 11, 1918. doi:10.3390/land11111918
Liu, M., Luo, Q., Wang, J., Sun, L., Xu, T., and Wang, E. (2024). VST-PCA: a land use change simulation model based on spatiotemporal feature extraction and pre-allocation strategy. ISPRS Int. J. Geo-Inf. 13, 100. doi:10.3390/ijgi13030100
Liu, Z., Tian, S., Lu, S., Zhu, Z., Peng, Y., Li, X., et al. (2023). Climate and land-use changes threaten the effectiveness of protected areas for protecting Galliformes in Southeast Asia. Front. Ecol. Evol. 11. doi:10.3389/fevo.2023.1216769
Loh, S. K., Asubonteng, K. O., and Adanu, S. K. (2022). Effects of monocropping on land cover transitions in the wet evergreen agro-ecological zone of Ghana. Land 11, 1063. doi:10.3390/land11071063
Malcolm, D. C., Mason, W. L., and Clarke, G. C. (2001). The transformation of conifer forests in Britain—regeneration, gap size and silvicultural systems. For. Ecol. Manage. 151 (1-3), 7–23. doi:10.1016/S0378-1127(00)00692-7
Mamajonova, N., Oydin, M., Usmonali, T., Olimjon, A., Madina, A., and Margʻuba, M. (2024). Main methods of creating topographic maps: a comprehensive review. Hold. Reason. 2 (1), 421–424.
Margules, C., and Pressey, R. (2000). Systematic conservation planning. Nature 405, 243–253. doi:10.1038/35012251
Marzialetti, F., Cesarini, G., Acosta, A. T. R., and Battisti, C. (2024). Testing effectiveness in nature reserve management using GIS-based tools: a 25 years monitoring of land use change in a Mediterranean coastal wetland. Wetl. Ecol. Manage. 32, 423–435. doi:10.1007/s11273-024-09985-0
Matsuyama, K. (1992). Agricultural productivity, comparative advantage, and economic growth. J. Econ. Theory 58 (2), 317–334. doi:10.1016/0022-0531(92)90057-O
Melillo, J. M., Lu, X., Kicklighter, D. W., Reilly, J. M., Cai, Y., and Sokolov, A. P. (2016). Protected areas’ role in climate-change mitigation. Ambio 45, 133–145. doi:10.1007/s13280-015-0693-1
Memmah, M. M., Lescourret, F., Yao, X., and Lavigne, C. (2015). Metaheuristics for agricultural land use optimization. A review. A Rev. Agron. sustain. Dev. 35, 975–998. doi:10.1007/s13593-015-0303-4
Meyer-Aurich, A., Zander, P., Werner, A., and Roth, R. (1998). Developing agricultural land use strategies appropriate to nature conservation goals and environmental protection. Land. Urban Plan. 41 (2), 119–127. doi:10.1016/S0169-2046(97)00065-0
Micklin, P. (2007). The Aral sea disaster. Annu. Rev. Earth Planet. Sci. 35 (1), 47–72. doi:10.1146/annurev.earth.35.031306.140120
Mierauskas, P. (2004). An evaluation of the strict nature reserves management in Lithuania and their correspondence to international requirements. Environ. Res. Eng. Manag. 3 (29), 62–70.
Min, H., Fengjun, J., and Fath, B. D. (2010). Measurement and spatial distribution of urban land use compactness in Chaoyang District of Beijing, China. Chin. J. Popul. 8 (4), 3–9. doi:10.1080/10042857.2010.10684997
Mohiuddin, G., and Mund, J.-P. (2024). Spatiotemporal analysis of land surface temperature in response to land use and land cover changes: a remote sensing approach. Remote Sens. 16, 1286. doi:10.3390/rs16071286
Mondal, Md.S., Sharma, N., Garg, P. K., and Kappas, M. (2016). Statistical independence test and validation of CA Markov land use land cover (LULC) prediction results. J. Remote Sens. Space Sci. 19 (2), 259–272. doi:10.1016/j.ejrs.2016.08.001
Mueller, L., Eulenstein, F., Dronin, N. M., Mirschel, W., McKenzie, B. M., Antrop, M., et al. (2021). “Agricultural landscapes: history, status and challenges,” in Exploring and optimizing agricultural landscapes. Innovations in landscape research. Editors L. Mueller, V. G. Sychev, N. M. Dronin, and F. Eulenstein (Cham, Switzerland: Springer), 3–54. doi:10.1007/978-3-030-67448-9_1
Munthali, M. G., Kindu, M., Adeola, A. M., Davis, N., Botai, J. O., and Solomon, N. (2023). Variations of ecosystem service values as a response to land use and land cover dynamics in Central Malawi. Environ. Dev. Sustain. 25, 9821–9837. doi:10.1007/s10668-022-02461-w
Mustaquim, S. M. (2024). Utilizing remote sensing data and ArcGIS for advanced computational analysis in land surface temperature modeling and land use property characterization. N.a. J. Adv. Res. Rev. 21 (1), 1496–1507. doi:10.30574/wjarr.2024.21.1.0231
Naqvi, S. A. H., Rehman, A. U., Chohan, S., Umar, U. U. D., Mehmood, Y., Mustafa, G., et al. (2023). “Sustainable development in agriculture beyond the notion of minimizing environmental impacts,” in Disaster risk reduction in agriculture. Disaster resilience and green growth. Editors M. Ahmed,, and S. Ahmad (Singapore City, Singapore: Springer), 147–168. doi:10.1007/978-981-99-1763-1_8
Nature Conservation Agency (2024a). Teiči strict nature reserve. Available at: https://www.daba.gov.lv/en/teici-strict-nature-reserve (Accessed August 6, 2024).
Nature Conservation Agency (2024b). Teiču dabas rezervāta dabas aizsardzības plāna precizētā tūrisma infrastruktūra the tourist infrastructure specified in the nature protection plan of the Teiči Nature Reserve. Latvian. Available at: https://www.daba.gov.lv/lv/media/3140/download (Accessed August 16, 2024).
O’Connor, C. D., Falk, D. A., and Garfin, G. M. (2020). Projected climate-fire interactions drive forest to shrubland transition on an Arizona Sky Island. Front. Environ. Sci. 8, 00137. doi:10.3389/fenvs.2020.00137
Pande, C. B., Srivastava, A., Moharir, K. N., Radwan, N., Sidek, L. M., Alshehri, F., et al. (2024). Characterizing land use/land cover change dynamics by an enhanced random forest machine learning model: a Google Earth Engine implementation. Environ. Sci. Eur. 36, 84. doi:10.1186/s12302-024-00901-0
Pham, Q. B., Ali, S. K., Parvin, F., On, V. V., Sidek, L. M., Đurin, B., et al. (2024). Multi-spectral remote sensing and GIS-based analysis for decadal land use land cover changes and future prediction using random forest tree and artificial neural network. Adv. Space Res. 74 (1), 17–47. doi:10.1016/j.asr.2024.03.027
Plourde, J. D., Pijanowski, B. C., and Pekin, B. K. (2013). Evidence for increased monoculture cropping in the Central United States. Agric. Ecosyst. Environ. 165, 50–59. doi:10.1016/j.agee.2012.11.011
Polat, N., and Kaya, Y. (2021). Investigation of the performance of different pixel-based classification methods in land use/land cover (LULC) determination. TİHA 3 (1), 1–6. doi:10.51534/tiha.829656
Potapov, P., Turubanova, S., Hansen, M. C., Tyukavina, A., Zalles, V., Khan, A., et al. (2022). Global maps of cropland extent and change show accelerated cropland expansion in the twenty-first century. Nat. Food 3, 19–28. doi:10.1038/s43016-021-00429-z
Potsdam Institute (2024). RCP concentration calculations and data. Available at: https://www.pik-potsdam.de/∼mmalte/rcps/(Accessed August 16, 2024).
Pourtaherian, P., and Jaeger, J. A. G. (2022). How effective are greenbelts at mitigating urban sprawl? A comparative study of 60 European cities. Land. Urban Plan. 227, 104532. doi:10.1016/j.landurbplan.2022.104532
Pramanik, S., and Punia, M. (2020). Land use/land cover change and surface urban heat island intensity: source–sink landscape-based study in Delhi, India. Environ. Dev. Sustain. 22, 7331–7356. doi:10.1007/s10668-019-00515-0
Pretty, J., and Smith, D. (2004). Social capital in biodiversity conservation and management. Conserv. Biol. 18 (3), 631–638. doi:10.1111/j.1523-1739.2004.00126.x
Priyadarshana, T. S., Martin, E. A., Sirami, C., Woodcock, B. A., Goodale, E., Martinez-Nunez, C., et al. (2024). Crop and landscape heterogeneity increase bio-diversity in agricultural landscapes: a global review and meta-analysis. Ecol. Lett. 27 (3), e14412. doi:10.1111/ele.14412
Qacami, M., Khattabi, A., Lahssini, S., Rifai, N., and Meliho, M. (2023). Land-cover/land-use change dynamics modeling based on land change modeler. Ann. Reg. 70, 237–258. doi:10.1007/s00168-022-01169-z
Ragkos, A., and Psychoudakis, A. (2009). Minimizing adverse environmental effects of agriculture: a multi-objective programming approach. Oper. Res. Int. J. 9, 267–280. doi:10.1007/s12351-009-0058-2
Rahnama, M. R. (2021). Forecasting land-use changes in Mashhad Metropolitan area using Cellular Automata and Markov chain model for 2016-2030. Sustain. Cities Soc. 64, 102548. doi:10.1016/j.scs.2020.102548
Rayfield, B., James, P. M. A., Fall, A., and Fortin, M.-J. (2008). Comparing static versus dynamic protected areas in the Quebec boreal forest. Biol. Conserv. 141 (2), 438–449. doi:10.1016/j.biocon.2007.10.013
Redford, K. H., and Adams, W. M. (2009). Payment for ecosystem services and the challenge of saving nature. Conserv. Biol. 23 (4), 785–787. doi:10.1111/j.1523-1739.2009.01271.x
Rist, L., Campbell, B. M., and Frost, P. (2012). Adaptive management: where are we now? Environ. Conserv. 40 (1), 5–18. doi:10.1017/S0376892912000240
Rohwer, Y., and Marris, E. (2021). Ecosystem integrity is neither real nor valuable. Conserv. Sci. Pract. 3 (4)–e411. doi:10.1111/csp2.411
Rudel, T. K., Schneider, L., Uriarte, M., Turner, B. L., DeFries, R., Lawrence, D., et al. (2009). Agricultural intensification and changes in cultivated areas, 1970–2005. PNAS 106 (49), 20675–20680. doi:10.1073/pnas.0812540106
Salaudeen, A., Shahid, S., Ismail, A., Adeogun, B. K., Ajibike, M. A., Bello, A.-A. D., et al. (2023). Adaptation measures under the impacts of climate and land-use/land-cover changes using HSPF model simulation: application to Gongola River basin, Nigeria. Sci. Total Environ. 858, 159874. doi:10.1016/j.scitotenv.2022.159874
Samiullah, M. A. K., Rahman, A.-U., and Mahmood, S. (2019). Evaluation of urban encroachment on farmland: a threat to urban agriculture in Peshawar city district, Pakistan. Erdkunde 73 (2), 127–142. doi:10.3112/erdkunde.2019.02.04
Sannigrahi, S., Zhang, Q., Joshi, P. K., Sutton, P. C., Keesstra, S., Roy, P. S., et al. (2020). Examining effects of climate change and land use dynamic on biophysical and economic values of ecosystem services of a natural reserve region. J. Clean. Prod. 257, 120424. doi:10.1016/j.jclepro.2020.120424
Šarapatka, B., and Štěrba, O. (1998). Optimization of agriculture in relation to the multifunctional role of the landscape. Urban Plan. 41 (2), 145–148. doi:10.1016/S0169-2046(97)00069-8
Schlaepfer, M. A., and Lawler, J. J. (2023). Conserving biodiversity in the face of rapid climate change requires a shift in priorities. Rev. Clim. Change 14 (1)–e798. doi:10.1002/wcc.798
Schmitz, M. H., do Couto, E. V., Xavier, E. C., da Silva Tomadon, L., Leal, R. P., and Agostinho, A. A. (2023). Assessing the role of protected areas in the land-use change dynamics of a biodiversity hotspot. Ambio 52, 1603–1617. doi:10.1007/s13280-023-01886-5
Shahi, E., Karimi, S., and Jafari, H. R. (2020). Monitoring and modeling land use/cover changes in Arasbaran protected Area using and integrated Markov chain and artificial neural network. Model. Earth Syst. Environ. 6, 1901–1911. doi:10.1007/s40808-020-00801-1
Shawul, A. A., and Chakma, S. (2019). Spatiotemporal detection of land use/land cover change in the large basin using integrated approaches of remote sensing and GIS in the Upper Awash basin, Ethiopia. Environ. Earth. Sci. 78, 141. doi:10.1007/s12665-019-8154-y
Shetty, S., Gupta, P. K., Belgiu, M., and Srivastav, S. K. (2021). Assessing the effect of training sampling design on the performance of machine learning classifiers for land cover mapping using multi-temporal remote sensing data and google Earth engine. Remote Sens. 13, 1433. doi:10.3390/rs13081433
Siegel, K., Perez, A. F., Kinnebrew, E., Mills-Novoa, M., Ochoa, J., and Shoffner, E. (2022). Integration of qualitative and quantitative methods for land-use-change modeling in a deforestation frontier. Conserv. Biol. 36, e13924. doi:10.1111/cobi.13924
Smith, S. J., and Wigley, T. M. L. (2006). Multi-gas forcing stabilization with the MiniCAM. Energy J. 27, 373–391.
Stojanović, T., Trišić, I., Brđanin, E., Štetić, S., Nechita, F., and Candrea, A. N. (2024). Natural and sociocultural values of a tourism destination in the function of sustainable tourism development—an example of a protected area. Sustainability 16, 759. doi:10.3390/su16020759
Stolton, S., Shadie, P., and Dudley, N. (2013). Guidelines for applying protected area management categories including IUCN WCPA best practice guidance on recognizing protected areas and assigning management categories and governance types. Gland. Gland, Switzerland: IUCN.
SunCalc.org (2024). Solar data for the selected location (spring equinox, march 21st, 2024, noon). Available at: https://www.suncalc.org/#/56.611,26.4707,11/2024.03.21/08:57/1/1 (Accessed August 16, 2024).
Szpakowska, B., Świerk, D., Dudzińska, A., Pajchrowska, M., and Goldyn, R. (2022). The influence of land use in the catchment area of small waterbodies on the quality of water and plant species composition. Sci. Rep. 12, 7265. doi:10.1038/s41598-022-11115-w
Tattoni, C., Ciolli, M., and Ferreti, F. (2011). The fate of priority areas for conservation in protected areas: a fine-scale Markov chain approach. Environ. Manage. 47, 263–278. doi:10.1007/s00267-010-9601-4
The Latvian Geospatial Information Agency (2024). Color orthophoto map for the entire territory of Latvia with a resolution of 0.25 meters. Available at: https://www.lgia.gov.lv/en/ortophoto-maps (Accessed August 16, 2024).
Thom, D. (2023). Natural disturbances as drivers of tipping points in forest ecosystems under climate change – implications for adaptive management. For. Int. J. For. Res. 96 (3), 305–315. doi:10.1093/forestry/cpad011
Thomson, A. M., Calvin, K. V., Smith, S. J., Kyle, G. P., Volke, A., Patel, P., et al. (2011). RCP4.5: a pathway for stabilization of radiative forcing by 2100. Clim. Change 109, 77–94. doi:10.1007/s10584-011-0151-4
Thurman, L. L., Gross, J. E., Mengelt, C., Beever, E. A., Thompson, L. M., Schuurman, G. W., et al. (2022). Applying assessments of adaptive capacity to inform natural-resource management in a changing climate. Conserv. Biol. 36 (2), e13838. doi:10.1111/cobi.13838
United States Geological Survey (2024). USGS Earth explorer. Available at: https://earthexplorer.usgs.gov/(Accessed August 16, 2024).
Verburg, R., and van Eijk-Bos, C. (2003). Effects of selective logging on tree diversity, composition, and plant functional type patterns in a Bornean rain forest. J. Veg. Sci. 14 (1), 99–110. doi:10.1658/1100-9233(2003)014[0099:eoslot]2.0.co;2
Vieira-Alencar, J. P. S., Bolochio, B. E., Carmignotto, A. P., Sawaya, R. J., Silveira, L. F., Valdujo, P. H., et al. (2023). How habitat loss and fragmentation are reducing conservation opportunities for vertebrates in the most threatened savanna of the World. Perspect. Ecol. Conserv. 21 (2), 121–127. doi:10.1016/j.pecon.2023.02.004
Wang, L., Wei, F., and Svenning, J.-C. (2023). Accelerated cropland expansion into high integrity forests and protected areas globally in the 21st century. Iscience 26 (4), 106450. doi:10.1016/j.isci.2023.106450
Wassenaar, T., Gerber, P., Verburg, P. H., Rosales, M., Ibrahim, M., and Steinfeld, H. (2007). Projecting land use changes in the Neotropics: the geography of pasture expansion into forest. Glob. Environ. Change 17 (1), 86–104. doi:10.1016/j.gloenvcha.2006.03.007
Williams, D. R., Clark, M., Buchanan, G. M., Ficetola, G. F., Rondinini, C., and Tilman, D. (2021). Proactive conservation to prevent habitat losses to agricultural expansion. Nat. Sustain. 4, 314–322. doi:10.1038/s41893-020-00656-5
Wise, M. A., Calvin, K. V., Thomson, A. M., Clarke, L. E., Bond-Lamberty, B., Sands, R. D., et al. (2009). Implications of limiting CO2 concentrations for land use and energy. Science 324, 1183–1186. doi:10.1126/science.1168475
Wunder, S. (2007). The efficiency of payments for environmental services in tropical conservation. Conserv. Biol. 21 (1), 48–58. doi:10.1111/j.1523-1739.2006.00559.x
Xue, W., Ma, H., Xiang, M., Tian, J., and Liu, X. (2023). From Sphagnum to shrub: increased acidity reduces peat bacterial diversity and keystone microbial taxa imply peatland degradation. Land Degrad. Dev. 34 (17), 5259–5272. doi:10.1002/ldr.4842
Yamaura, Y., Shoji, Y., Mitsuda, Y., Utsugi, H., Tsuge, T., Kuriyama, K., et al. (2016). How many broadleaved trees are enough in conifer plantations? The economy of land sharing, land sparing and quantitative targets. J. Appl. Ecol. 53 (4), 1117–1126. doi:10.1111/1365-2664.12642
Ye, S., Song, C., Kuzyakov, Y., Cheng, F., Kong, X., Feng, Z., et al. (2022). Preface: arable land quality: observation, estimation, optimization, and application. Land 11, 947. doi:10.3390/land11060947
Yimer, S. M., Bouanani, A., Kumar, N., Tischbein, B., and Borgemeister, C. (2024). Comparison of different machine-learning algorithms for land use land cover mapping in a heterogenous landscape over the Eastern Nile River basin, Ethiopia. Adv. Space Res. 74 (5), 2180–2199. doi:10.1016/j.asr.2024.06.010
Zabel, F., Delzeit, R., Schneider, J., Seppelt, R., Mauser, W., and Vaclavik, T. (2019). Global impacts of future cropland expansion and intensification on agricultural markets and biodiversity. Nat. Commun. 10, 2844. doi:10.1038/s41467-019-10775-z
Zarandian, A., Mohammadyari, F., Mirsanjari, M. M., and Visockiene, J. S. (2023). Scenario modeling to predict changes in land use/cover using Land Change Modeler and InVEST model: a case study of Karaj Metropolis, Iran. Environ. Monit. Assess. 195, 273. doi:10.1007/s10661-022-10740-2
Zhang, P., Jiacheng, Z., and Krott, M. (2023). Strictest nature conservation: China's national park policy underpinned by power shift and turf dynamic. For. Policy. Econ. 154, 103033. doi:10.1016/j.forpol.2023.103033
Zhao, Y., Abdelkareem, M., and Abdalla, F. (2024). Remote sensing and GIS techniques in monitoring and mapping land system change in semi-arid environments. Environ. Earth. Sci. 83, 420. doi:10.1007/s12665-024-11706-y
Keywords: conservation zoning, land use and land cover modeling, nature reserves, protected areas, scenario modeling, remote sensing
Citation: Krumins J, Klavins M and Stankevica K (2024) Implications for conservation zoning in Teiči Strict Nature Reserve due to land use and cover change. Front. Remote Sens. 5:1497454. doi: 10.3389/frsen.2024.1497454
Received: 17 September 2024; Accepted: 18 November 2024;
Published: 28 November 2024.
Edited by:
Ahmet Cilek, Çukurova University, TürkiyeReviewed by:
Vilém Pechanec, Palacký University, Olomouc, CzechiaJavier Martínez-Vega, Spanish National Research Council (CSIC), Spain
Copyright © 2024 Krumins, Klavins and Stankevica. This is an open-access article distributed under the terms of the Creative Commons Attribution License (CC BY). The use, distribution or reproduction in other forums is permitted, provided the original author(s) and the copyright owner(s) are credited and that the original publication in this journal is cited, in accordance with accepted academic practice. No use, distribution or reproduction is permitted which does not comply with these terms.
*Correspondence: Janis Krumins, a3J1bWlucy5qYW5pc0BsdS5sdg==