- 1TETIS, INRAE, Montpellier, France
- 2Eco&Sols, CIRAD, Montpellier, France
- 3Eco&Sols, CIRAD, INRAE, IRD, Institut Agro, Université de Montpellier, Montpellier, France
- 4Kayrros SAS, Paris, France
- 5Laboratoire des Sciences du Climat et de l’Environnement, LSCE/IPSL, CEA-CNRS9 UVSQ, Université Paris-Saclay, Gif-sur-Yvette, France
- 6CESBIO, Université Toulouse III - Paul Sabatier, Toulouse, France
Canopy height is a key indicator of tropical forest structure. In this study, we present a deep learning application to map canopy height in French Guiana using freely available multi-source satellite data (optical and radar) and complementary environmental information. The potential of a U-Net architecture trained on sparse and unevenly distributed GEDI data to generate a continuous canopy height map at a regional scale was assessed. The developed model, named CHNET, successfully produced a canopy height map of French Guiana at a 10-m spatial resolution, achieving relatively good accuracy compared to a validation airborne LiDAR scanning (ALS) dataset. The study demonstrates that relevant environmental descriptors, namely, height above nearest drainage (HAND) and forest landscape types (FLT), significantly contribute to the model’s accuracy, highlighting that these descriptors bring important information on canopy structural properties and that the CHNET framework can efficiently use this information to improve canopy height prediction. Another critical aspect highlighted is the necessity of addressing GEDI data inaccuracies and geolocation uncertainties, which is essential for any GEDI-based application. However, challenges remain, particularly in characterizing tall canopies, as our CHNET model exhibits a tendency to underestimate canopy heights greater than 35 m. A large part of this error arises from the use of GEDI measurements as reference, given the fact these data exhibit certain saturation in tropical biomes. Future improvements in the analysis of GEDI signal as well as the implementation of robust models are essential for better characterization of dense and tall tropical forest ecosystems.
1 Introduction
Tropical forests play a major role in maintaining global ecological balance. Beyond the richness of their biodiversity, they are essential for carbon sequestration, absorbing significant amounts of carbon dioxide and thereby mitigating the effects of climate change (Pan et al., 2011). Mapping forest resources and carbon is crucial for enhancing forest management practices and achieving goals related to environmental preservation. The accurate characterization and monitoring of the standing aboveground biomass (AGB) have become imperative to understand the carbon sequestration potential of tropical ecosystems. In this regard, several studies have highlighted relationships between the structural characteristics of a forest and its AGB levels (Chave et al., 2005; Asner and Mascaro, 2014). In tropical regions like French Guiana, the dense and complex forest structure makes the precise estimation of AGB particularly challenging (Saatchi et al., 2011). Accurately describing forest structure, including canopy height, is essential for reliable biomass estimates at a regional scale (Joetzjer et al., 2017). Canopy height is indeed one of the most important inputs to estimate biomass, and most allometric equations incorporate this structural parameter to calculate AGB (Lefsky et al., 2005; Lima et al., 2012; Feldpausch et al., 2012).
Remote sensing is a powerful tool for forest monitoring because it offers the ability to collect extensive data across large and often inaccessible areas (Boyd and Danson, 2005). Light detection and ranging (LiDAR), in particular, is highly effective for characterizing vegetation profiles and structural parameters. Among LiDAR technologies, airborne LiDAR scanning (ALS) stands out as the most accurate and offers the highest resolution data (in terms of returned points over a given surface). However, it is also more costly for data users and its spatial coverage is limited to specific areas. In French Guiana, where accessibility is limited, ALS data have been widely used for localized studies (Ho Tong Ming et al., 2016; Joetzjer et al., 2017; Lahssini et al., 2022), but the high cost of this method limits its applicability for large-scale applications. In contrast, spaceborne sensors, while having a lower spatial resolution, offer better revisit times and global coverage, making them invaluable for large-scale and continuous monitoring. These sensors have demonstrated their capability to produce regional or global forest products (Tyukavina et al., 2015) and effectively map canopy height (Fayad et al., 2014; Pourrahmati et al., 2015). The Global Ecosystem Dynamics Investigation (GEDI) mission is the most recent advancement in spaceborne LiDAR technology, and it was specifically designed to measure the structure of the vegetation at a global scale (Dubayah et al., 2020). GEDI has proven its ability to map canopy height accurately and can be complemented with other remote sensing data sources to provide comprehensive information on forest structure and dynamics (Potapov et al., 2021; Lang et al., 2023). Continuous data sources are indeed useful to transform the sparse coverage of GEDI measurements into continuous canopy height maps.
One complementary data source often used with GEDI information is optical data, and studies have already used this complementarity in the context of French Guiana (Ngo et al., 2023). Optical sensors are extensively used to examine the state parameters of forest stands and surface vegetation. Using radiometric information obtained from optical systems, it is possible to characterize stand compositions and vegetation biophysical parameters by analyzing the spectral information contained in the reflectance images (Lemaire et al., 2008; Grabska et al., 2019). One of the primary advantages of most recent satellite optical sensors lies in their capacity to provide complete coverage and high-resolution (both spatial and temporal) multispectral imagery that offers accurate information on forest structure and composition. This information can be used in forest type classification, species identification, and monitoring of canopy cover (Hansen et al., 2013; Vancutsem et al., 2021). Many optical data products can be acquired freely by data users and are available from several satellite platforms, enabling long-term and large-scale assessments of tropical forests. Nonetheless, there are also limitations to consider. First and foremost, cloud cover can impede data acquisition, especially in tropical regions with unique environmental conditions and frequent cloud cover. Furthermore, optical sensors are passive systems that are sensitive to solar illumination conditions, which can limit data acquisition to specific times of the day. They are also sensitive to sun-sensor angle view effects, which are difficult to correct. Additionally, optical sensors are limited in their ability to penetrate canopy cover, thus impeding the study of understory vegetation and ground-level features. A combination of optical data with other remote sensing techniques can therefore offer a more comprehensive view of forest ecosystems.
In this perspective, synthetic aperture radar (SAR) technologies bring specific information on canopy structure and have been effectively employed alongside GEDI data in French Guiana (Ngo et al., 2023; Qi et al., 2023). SAR sensors use lower frequency electromagnetic waves (microwaves) than LiDAR but still offer advantages for studying forest ecosystems. One of their primary advantages is their ability to penetrate cloud cover and to operate in darkness, allowing for data acquisition in all weather conditions and at any moment of the day. Due to their longer wavelengths, radar signals can also penetrate the forest canopy to a certain extent (depending on the wavelength, i.e., deeper penetration with longer wavelengths) and provide information on forest structure and the lower layers of the canopy. Moreover, SAR data are highly sensitive to changes in canopy structure, which makes it suitable for detecting changes such as disturbances, deforestation, and degradation (Rahman and Sumantyo, 2010; Bouvet et al., 2018; Watanabe et al., 2018). Using different frequency bands can be advantageous for studying vegetation because they complement each other (Berninger et al., 2018; Sothe et al., 2022). On one hand, the L-band, with its longer wavelength can penetrate deeper into the vegetation and provide information on the lower layers of the canopy (Baghdadi et al., 2015). This information is particularly relevant for estimating forest structure variables (Mermoz et al., 2014). On the other hand, the C-band, with its shorter wavelength, is limited in its penetration capabilities but can still provide detailed information about the leaf density of upper canopies (Fagua et al., 2019), which is indirectly related to canopy height (McDowell et al., 2002). While LiDAR remains the most appropriate technology for deriving accurate structural descriptors of forest ecosystems, optical and radar data can provide complementary information and multimodal approaches allow combining the advantages of each individual sensor type. Indeed, spaceborne LiDAR sparse measurements do not exhibit a sufficient spatial density for accurate and continuous mapping of canopy height. Therefore, the complementarity between optical, radar, and LiDAR data is leveraged to produce canopy height maps.
Such multimodal approaches have been employed successfully over French Guiana to overcome the limitations of individual sensors in canopy height mapping. However, most existing studies have either focused on very localized areas, such as research plots (Ngo et al., 2023), or have been conducted at global scales (Potapov et al., 2021; Lang et al., 2023; Qi et al., 2023). In contrast, our study addresses the gap in regional-scale applications, which allows us to explore how data fusion approaches behave and can be optimized at an intermediate yet countrywide scale. Employing an appropriate data fusion framework is essential for the optimal processing of multiple data sources, with the goal of producing a final canopy height map of French Guiana. Standard approaches, such as linear models, regression analysis, and Random Forest provide widely accepted methods for combining data (Morin et al., 2019). As the richness and complexity of multimodal information from various sensors increase, deep learning (LeCun et al., 2015) approaches prove to be particularly useful and effective. Deep learning methods have become prevalent in remote sensing applications due to their powerful ability to analyze and process data from different sources, times, and scales (Hong et al., 2021). By stacking nonlinear functions, neural networks enable the modeling of complex relationships between input and output variables. In multimodal applications, two main strategies are employed to combine heterogeneous and complementary information: early fusion and late fusion (Hong et al., 2021). Early fusion combines data from different sources at the initial stage and then processes them through a neural network. In contrast, late fusion involves analyzing each data source separately using dedicated branches and then combining the intermediate results through additional neural network layers for final processing. Since the introduction of Convolutional Neural Networks (CNNs), there has been a substantial improvement in the accuracy of image analysis tasks. The U-Net architecture (Ronneberger et al., 2015), a specialized type of CNN widely used in image segmentation and remote sensing studies (Yuan et al., 2021), demonstrates an effective neural network model for these applications. U-Net’s design, which includes a contracting path to capture local context and a symmetric expanding path for precise localization, is particularly well-suited for handling spatial data. With the goal of predicting and mapping canopy height at a regional scale, this architecture is quite adequate because of its ability to integrate detailed spatial information from multiple remote sensing data. Consequently, U-Net has been extensively used in numerous studies for canopy height mapping using diverse remote sensing data sources (Gazzea et al., 2023; Wagner et al., 2024; Schwartz et al., 2024; Ge et al., 2022). However, most existing U-Net frameworks have exclusively utilized sensor data, and there is still a need to explore how ancillary information related to canopy structure and ecological context can be leveraged by this type of architecture in a regional-scale application.
In general, canopy height mapping approaches often rely exclusively on remote sensing data. However, considering environmental parameters alongside these data sources can provide significant added value. For instance, in some studies, integrating topographical data into the modeling process has led to improvements in the accuracy and reliability of forest biophysical variables estimation (Lahssini et al., 2022). Environmental parameters have indeed a direct impact on canopy dynamics. Identifying the most relevant descriptors to integrate into a deep learning architecture may bring an additional contribution, enabling the architecture to extract complex relationships between these descriptors and canopy height. In this study, we propose an end-to-end early fusion deep learning model that effectively leverages the complementarity between optical (Sentinel-2), radar (Sentinel-1 and ALOS), and environmental data to produce a 10-m resolution canopy height map of French Guiana (South America). The height above nearest drainage (HAND) and the forest landscape types (FLT) were retained as environmental descriptors that are directly related to canopy height. The proposed framework, named CHNET (Canopy Height estimation NETwork), implements an early fusion strategy through a U-Net architecture. GEDI measurements are used as reference canopy heights for the CHNET model calibration. The main objective of our study is to assess how tropical canopy height can be accurately estimated and mapped from GEDI information and multiple data sources. Our study operates at an intermediate regional scale by accounting for the specificities of the French Guiana context while still addressing the larger scale of the entire country. We aim at understanding how the enrichment and optimization of model inputs, such as integrating additional environmental variables and refining the GEDI data used for model calibration, can potentially lead to improved and more accurate results.
2 Materials and methods
2.1 Study area
French Guiana, a French Overseas Territory, is located in the Amazon forest biome on the northern coast of South America. It spans a total area of 83,534 km2, of which over 80,000 km2 are covered by forests (Fayad et al., 2016). The predominant forest type is mature old-growth tropical rainforest, with some regions consisting of secondary forests (Guitet et al., 2013). Coastal areas also feature savannas and mangroves, while rainforest accounts for more than 90% of the territory. Timber harvesting and agricultural activities are mainly confined to the sub-coastal areas near large towns and along the main roads (Guitet et al., 2015). The region’s topography is mostly flat, with elevations rarely exceeding 200 m, though small hills and mountains occasionally rise across the landscape (Guitet et al., 2013). Notably, about 70% of the terrain slopes are less than 5° (Fayad et al., 2016). French Guiana experiences a tropical and hot climate, with a Köppen climate classification of tropical rainforest (Af) across most of the territory (Beck et al., 2018). The mean annual temperature is approximately 26°C and rainfall varies significantly across the territory, with the northeast receiving up to 4,000 mm per year, while the southern and western areas receive around 2,000 mm annually (Guitet et al., 2015). The combination of climatic, geological, and geomorphological factors provides the conditions for the development of a wide range of forest structures at a regional scale. Canopy height in French Guiana’s tropical forests generally ranges between 20 and 40 m, with emergent trees occasionally reaching up to 60 m (Fayad et al., 2014). AGB levels vary significantly and generally range from around 150 Mg/ha to over 600 Mg/ha, with higher levels typically found in mature and undisturbed forest areas (Fayad et al., 2016).
2.2 Datasets and processing
2.2.1 GEDI data
GEDI, operated by NASA aboard the International Space Station (ISS) since December 2018, is a full-waveform LiDAR sensor designed to capture high-resolution vertical structure data of Earth’s forests. The system employs three 1,064 nm lasers emitting 242 pulses per second, generating energy return waveforms (L1B product) and derived height metrics (L2A product) within 25 m diameter circular footprints. One laser is split into two half-power beams (coverage beams), while the other two lasers remain at full power (power beams). These beams are then slightly dithered to generate eight parallel tracks of observations: four tracks for coverage beams and four tracks for power beams. These tracks cover a 4.2-km swath on the ground, with a 60-m spacing along the track and a 600-m spacing across tracks.
GEDI data are processed by NASA’s Land Processes Distributed Active Archive Center (LP DAAC). The L1B product includes geolocated and smoothed waveforms, along with ancillary parameters, and offers a horizontal location precision improved to 10 m (Dubayah et al., 2021). The L2A product delivers elevation and height metrics for each footprint, including measurements of ground elevation, canopy top height and relative height (RH) metrics. The L2A product is generated from the L1B waveforms using six distinct signal processing configurations, referred to as algorithm setting groups. These configurations define the thresholds and smoothing parameters used to analyze the received waveforms, thereby influencing the height metrics extracted in the L2A product. Comparing all available configurations indicates that algorithm setting group number five generally yields better accuracy on average compared to other groups in the tropical context of French Guiana (Lahssini et al., 2022). GEDI’s ability to estimate canopy height is contingent on its ability to detect the ground (Sun et al., 2022). Due to the dense vegetation in tropical areas, the ground peak in GEDI waveforms is often weaker in intensity and difficult to distinguish from the background noise. Algorithm setting group number five is characterized by the lowest waveform signal end threshold. In a dense environment, a low signal end threshold enhances the differentiation of weak ground returns from noise in the signal, while a higher threshold tends to lead to errors in the detection of the ground peak in the waveform. Conversely, in less dense forest ecosystems, a low threshold can result in the interpretation of noise as the ground peak, thus leading to an overestimation of canopy heights (Adam et al., 2020). GEDI waveforms obtained over tropical forests often present weak intensity ground peaks, suggesting that a lower signal end threshold is more suitable for these ecosystems. Considering the dense and homogeneous context of French Guiana, as well as the results of related works in the literature (Lahssini et al., 2022), we therefore utilized the L2A metrics that were computed using algorithm setting group number 5.
GEDI RH metrics are considered as a reliable representation of canopy heights and have been used as reference variables to calibrate height estimation models (Lang et al., 2023). The L2A product contains several RH metrics representing the height relative to the ground corresponding to a given percentile of total return energy. For instance, rh_95 represents the height relative to the ground which contains 95% of the waveform energy. High-percentile RH metrics are therefore good proxies of canopy height as they are related to the top-of-canopy detection. Theoretically, rh_100 would be expected to be the best representation of canopy height and should correspond to the distance between the top-of-canopy and the ground. However, this metric can be impacted by atmospheric conditions, incorrect ground peak detection in the waveform as well as variability in both vegetation and ground conditions (Schwartz et al., 2024). In this study, we chose to use rh_95 as a direct proxy of canopy height since it proved to yield the best performances in the tropical context of French Guiana (Lahssini et al., 2022). Several previous studies have also advocated for the use of rh_95 to model canopy height in tropical biomes (Potapov et al., 2021; Fayad et al., 2021; Lahssini et al., 2024).
Overall, 11,798,179 GEDI shots acquired between April 2019 and May 2022 over French Guiana were collected from the GEDI Level 2A Geolocated Elevation and Height Metrics product (GEDI02_A v002) made available by NASA (Dubayah et al., 2021). Atmospheric disturbances can strongly impact GEDI measurements, which is why the data meeting the following criteria were considered invalid and were removed: (1) shots with no mode detected (num_detectedmodes = 0), as they correspond to pure noise; (2) shots with a null signal-to-noise ratio (SNR), as they are also pure noise; (3) shots displaying an erroneous ground elevation, i.e., when the absolute difference between Shuttle Radar Topography Mission (SRTM) ground elevation (digital_elevation_model_srtm) and GEDI ground detection (elev_lowestmode) is greater than 100 m; (4) shots exhibiting an incomplete waveform, i.e., partial signals where the useable part of the waveform (search_end) is equal to the total number of bins in the waveform (rex_sample_count); (5) shots with rh_100 values lower than 3 m, as GEDI is well suited for vegetation but is unable to measure the height of small objects because of laser pulse width (Dubayah et al., 2020). Following these procedures, a total of 3,891,348 valid footprints (33%) were extracted. Furthermore, in order to provide our framework with useable and interpretable metrics, only GEDI shots with a SNR greater than 10 dB (2,127,076 shots, 18%) were retained as reference data for building our canopy height estimation models (Figure 1).
The corresponding GEDI rh_95 values were rasterized on a 10 m grid aligned with the other remote sensing data sources. For each GEDI shot, the associated rh_95 value was assigned to the pixel corresponding to the center of the footprint. This approach has been applied in several other studies to produce continuous canopy height maps. Lang et al., 2023 used a similar rasterizetion method in their global canopy height map to match GEDI data with the Sentinel-2 grid. They argued that this allows the model to optimize its loss function with respect to valid reference pixels during training. Potapov et al. (2021) employed the same approach in a study using Landsat data at a different spatial resolution (30 m), demonstrating that matching GEDI footprints’ centers to the grid of optical sensors is a widely accepted method for canopy height mapping. Despite the difference in resolutions between GEDI (25 m) and Sentinel-2 (10 m at best), rasterizing GEDI data to a finer grid can provide additional detail, especially in areas with heterogeneous canopy structures. Schwartz et al. (2024) explored this scale mismatch in their study on canopy height mapping in the Landes forest (France). They tested a modified prediction model at a 20-m resolution (i.e., rasterizing GEDI data to a 20-m grid and producing a canopy height map at 20 m), specifically designed to reduce the scale mismatch between GEDI and Sentinel data. They found that their original model with a 10-m resolution performed slightly better in terms of accuracy and that it was also better at capturing small-scale canopy variations such as gaps or holes. This suggests that rasterizing GEDI data to a higher-resolution grid can provide more detailed information, particularly in capturing heterogeneous canopy structures. All things considered, while the scale mismatch could contribute to some variability in canopy height estimates, this approach still retains meaningful spatial variations and has been widely used in similar studies with good results in practice.
2.2.2 Optical data
The Sentinel-2 (S2) satellite constellation, operated by the European Space Agency (ESA), consists of two satellites, Sentinel-2A and Sentinel-2B, which operate in tandem to ensure a high revisit frequency (5 days) and complete coverage. It provides high-resolution multispectral imagery in 13 spectral bands ranging from visible and near-infrared to shortwave infrared wavelengths. This radiometric diversity enables detailed characterization of stand compositions and vegetation biophysical parameters by analyzing the spectral information contained in the reflectance images (Karasiak et al., 2017; Grabska et al., 2019). The data acquired from S2 are first processed to Level-1C, providing top-of-atmosphere reflectance values that are geometrically corrected. In this study, we utilized Level-2A products, which include atmospherically corrected bottom-of-atmosphere reflectance values using ESA’s Sen2Cor processor (Main-Knorn et al., 2017).
Using the Google Earth Engine (GEE) data catalog and developing platform, we selected S2 images with a maximum cloud cover percentage of 80% (i.e., images with more than 80% cloud coverage were excluded) over the area of interest in the period between November 2019 and February 2021, with the goal of creating a cloud-free composite image for the year 2020. A cloud mask was applied to each image using sophisticated filters and thresholds from the PINO algorithm version 26 (Simonetti et al., 2021). The cloud and shadow mask computation was based on the Level-1C products and the results were eventually applied to the correspondent Level-2A images. After cloud masking, we generated a composite image for the year 2020 by computing the per-pixel median value across the collection of Level-2A S2 images. We opted for the median composite to mitigate the influence of outlier pixels, such as those affected by clouds or cloud shadows that may persist despite the cloud mask (Corbane et al., 2020; Pimple et al., 2022). Ten spectral bands were retained and, if necessary, resampled to 10 m: B2 (Blue), B3 (Green), B4 (Red), B5-B6-B7 (Vegetation Red Edge), B8 (Near Infrared, NIR), B8A (Narrow NIR), and B11-B12 (Short Wave Infrared, SWIR). The pixel values represent surface reflectance scaled by 10,000. The Sentinel-2 data used in this study were acquired from two distinct orbits. Despite S2 atmospheric corrections, which are more challenging in tropical contexts, differences in reflectance values between the two orbits remain. To mitigate this effect, and given that the two orbits overlap, we conducted a relative reflectance normalization for each spectral band separately. This process involved minimizing a cost function based on the mean and standard deviation within the overlapping area (Cresson and Saint-Geours, 2015).
2.2.3 Radar data
Radar data provide penetration capabilities through cloud cover and offer valuable information on forest structure (Baghdadi et al., 2015). Depending on their wavelength, radar signals can also penetrate the forest canopy to different depths, providing information on forest structure and on the lower layers of the canopy. Sentinel-1 (S1) is a C-band Synthetic Aperture Radar (SAR) system (wavelength of about 6 cm) while the Advanced Land Observing Satellite (ALOS) operates in the L-band (wavelength of about 25 cm), which allows us to benefit from the complementarity between these two bands.
S1 is comprised of two satellites with a 12-day repeat cycle, which results in a combined revisit time of 6 days for the same location on Earth. In this study, we utilized Ground Range Detected (GRD) scenes featuring dual-band cross-polarization: Vertical-Vertical (VV) and Vertical-Horizontal (VH), with a pixel size of 10 m. These scenes underwent preprocessing with S1 Toolbox, including thermal noise removal, radiometric calibration, and terrain correction using SRTM. The S1 GRD products provide Sigma-0 backscattering coefficients (in dB), which quantify the microwave radiation scattered back to the radar system. Using GEE interface, we extracted S1 images encompassing the area of interest in the period between November 2019 and February 2021 and the Sigma-0 values were calibrated using Gamma-0 normalization in order to correct the effects of radar signal attenuation due to varying incidence angles. Subsequently, we separated the data into ascending and descending orbits to generate mean composite images for the year 2020 for each orbit category and polarization. While median compositing is commonly used for optical imagery to create cloud-free composites, SAR data are not affected by clouds, enabling the use of mean compositing for constructing a data stack (Verhegghen et al., 2016; Barenblitt et al., 2024). This process resulted in a composite stack composed of four layers: VV ascending, VH ascending, VV descending, and VH descending.
ALOS Phased Array-type L-band SAR (PALSAR) is a system operated by the Japan Aerospace Exploration Agency (JAXA), featuring a sun-synchronous orbit and an observation frequency of 46 days. In this study, we utilized Normalized Radar Backscatter (NRB) scenes from the ALOS-2 PALSAR-2 ScanSAR Level 2.2 data product, which consists of dual-polarization observations: Horizontal-Horizontal (HH) and Horizontal-Vertical (HV), initially at a spatial resolution of 25 m that was resampled to 10 m. These scenes were subject to ortho-rectification as well as radiometric terrain correction and are provided in the Gamma-0 backscatter convention (in dB). Through the GEE platform, we selected ALOS-2 PALSAR-2 images covering the study site between November 2019 and February 2021 to produce a mean composite image for each dual-polarization, resulting in a composite stack comprised of two bands: HH and HV.
2.2.4 Environmental data
Topography has an impact on both remote sensing acquisitions and forest structure (Liu et al., 2021; Kutchartt et al., 2022). French Guiana is fairly flat, which is why topographic factors were not taken into account to correct GEDI waveforms, as their impact on canopy height estimates is minimal in these conditions (Fayad et al., 2021). Nonetheless, topography remains a crucial hydrologic driver because it determines the direction and velocity of water flows. Flow directions establish hydrological connections between various points within a basin, which in turn directly impact forest structure and dynamics (Ferry et al., 2010; Jucker et al., 2018; Muscarella et al., 2020). Consequently, canopy features are closely linked with soil and hydrological conditions, relationships that optical or radar imagery alone cannot fully reveal. For instance, in the Amazon, it is challenging to differentiate non-floodable swampy forests, which are characterized by waterlogged soils but are not subject to regular river flooding, from non-floodable terra-firme forests, characterized by well-drained soils, using only two-dimensional spectral data (Rennó et al., 2008). To address this, the height above nearest drainage (HAND) algorithm was developed to introduce the concept of height difference along flow channels, or drainage capacity, as a distinctive terrain descriptor (Rennó et al., 2008). This algorithm generates a normalized digital elevation model, which normalizes topography to the relative heights along the drainage network. Each pixel value corresponds to the vertical distance in meters to the nearest drainage. As a result, HAND provides essential context for interpreting canopy height variations. In this study, we utilized the Global HAND product available in the GEE catalog. In French Guiana, we extracted the global 30 m HAND product using a threshold of 100 river head cells, and we resampled it to a spatial resolution of 10 m to match the expected resolution of our canopy height map (Figure 2). The threshold of river head cells specifies the minimum number of grid cells required to contribute to water flow and establish the starting point of a stream or a river. This threshold determines where the flow accumulation is sufficient to initiate a river head in the digital elevation model and sets the scale for drainage network extraction. It controls the density and extent of the river network identified in the HAND model.
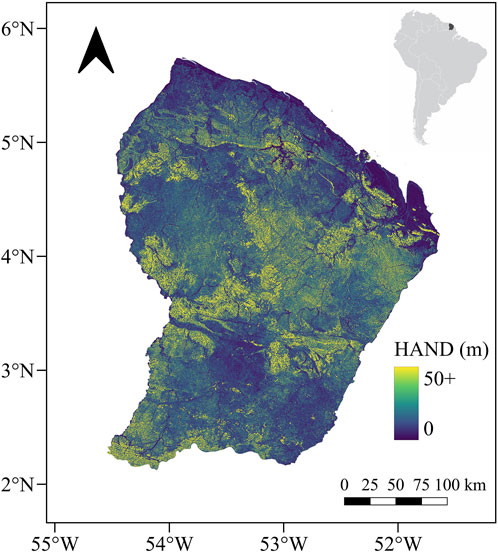
Figure 2. Height above nearest drainage (HAND) over French Guiana. 93% of pixels present a HAND value between 0 and 50 m. The maximum HAND value is 639 m.
Forest landscape types (FLT) provide information on the broader ecological context of French Guiana. This territory may appear simply as a vast and uniform area, but it is in fact composed of interconnected and complex ecosystems. In this study, a map delineating 20 forest classes, produced by the French National Forests Office (ONF), was utilized (Catalogue des habitats forestiers de Guyane, 2015). This FLT map was computed from several spatial analyses: (1) a geomorphological landscape map generated by ONF using a spatial analysis of a digital surface model derived from SRTM data (Guitet et al., 2013); (2) a predictive map of low-lying areas also developed by ONF using the same SRTM data and adapting the HAND algorithm; (3) a forest vegetation map based on SPOT-VEGETATION images, which allows representing forest structure variation (Gond et al., 2011); (4) a land cover layer for the coastal strip produced by ONF from aerial photography to provide precise delineation of specific coastal habitats; (5) a human footprint map highlighting disturbances related to forestry and mining activities (De Thoisy et al., 2010), supplemented with mapping of areas exploited since 1945. These datasets allowed building a comprehensive basis for describing the diverse forest ecosystems in French Guiana. The incorporation of environmental data, such as forest landscapes, into canopy height or biomass characterization approaches holds significant interest and has been explored in various studies to produce improved results (Fayad et al., 2016; Morin et al., 2022; Morin et al., 2023; Fassnacht et al., 2021). The FLT map used in this study was integrated in our prediction models in the form of a raster at a spatial resolution of 10 m, with each pixel value corresponding to a given class of forest landscape (Figure 3).
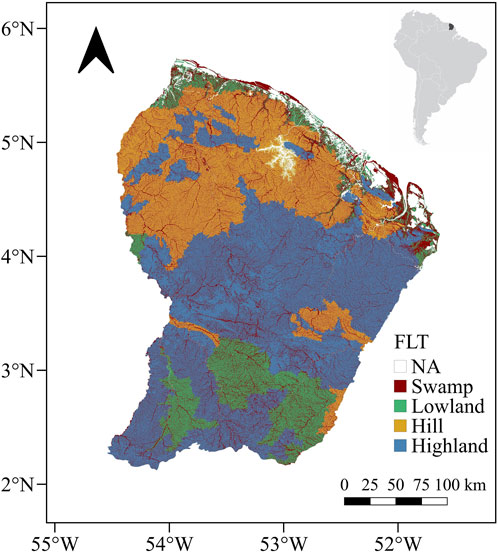
Figure 3. Main forest landscape types (FLT) of French Guiana. Each main class is divided into subclasses, resulting in a total of 20 FLT. NA represents non-forested areas, which include regions affected by past human disturbances such as logging and mining activities prior to 2015, as well as naturally non-forested areas. However, this map does not capture more recent disturbances and natural forest degradations.
2.2.5 ALS data
ALS data were employed as reference ground truth to validate our canopy height map. These data were acquired by ONF through several surveys over various study sites in French Guiana between 2017 and 2020. The ALS acquisitions were characterized by technical specifications that included an average of 10–12 points per m2 in average, with each pulse having a diameter of approximately 20 cm, and a wide scan angle of ±30°. A canopy height model (CHM) was derived by ONF at a resolution of 1 m (maximum height per 1-m grid cell), covering an area of 1,562 km2. The CHM was resampled to a resolution of 10 m using the maximum value to match the resolution of our canopy height map. The choice of the maximum value in the resampling process is theoretically justified by the fact it better represents the top-of-canopy signal captured in GEDI waveforms, which are used as training data to build our prediction models. Adam et al. (2020) supported this approach, noting that the signal in return waveforms starts at the highest point of vegetation within the GEDI footprint. In a similar comparative study, Hilbert and Schmullius (2012) demonstrated that using the maximum rather than the mean value of ALS reference heights yielded a better correlation with GEDI metrics. Furthermore, Lahssini et al. (2024) found that GEDI-derived canopy heights exhibited stronger correlations with the maximum ALS heights within GEDI footprints.
2.3 Methods
2.3.1 Multimodal U-Net architecture
To produce a continuous canopy height map, we implemented a pixel-wise regression, which is a specialized task in machine learning to predict continuous values for each pixel in an image. This approach is particularly useful and relevant in our application, where each pixel represents a unique geospatial location and our goal is to estimate the canopy height for each of these locations based on their spectral and spatial features. In particular, fully convolutional networks (FCNs) are a type of deep learning model designed for spatially dense prediction tasks. They have been transformed from classical convolutional networks to handle inputs of any size and generate outputs of corresponding dimensions with efficient processing during both training and inference phases (Long et al., 2014). For the purpose of our study, a U-Net neural network architecture (Milesi, 2022) was used due to its proven efficacy in regression tasks for forest characterization involving multiple remote sensing data sources (Gazzea et al., 2023; Wagner et al., 2024; Schwartz et al., 2024; Ge et al., 2022). To manage and exploit the available multimodal information, each geospatial location (i.e., a pixel of 10 m) is described by means of multiple information sources as detailed in Subsection 2.2. U-Net is particularly good at capturing complex spatial dependencies in images and interpreting the local spatial context. Also, one of the key strengths of deep learning frameworks such as U-Net lies in their ability to integrate and leverage the complementarity between different datasets. The U-Net architecture is shaped like a ‘U’ because it sequentially downsamples then upsamples images. This design allows the network to learn robust feature representations at multiple scales. In the encoder part (contracting path), the input image undergoes two 3 × 3 convolutions, which are then followed by a Rectified Linear Unit (ReLU) activation function. This sequence is subsequently downsampled using a 2 × 2 max pooling operation with a stride of 2. This process is repeated four times, with the number of feature channels being doubled at each step to effectively capture hierarchical features at different scales. In total, there are 10 convolutional layers and 4 max pooling layers in the encoder part of the architecture. The decoder part (expansive path) mirrors the encoder but in reverse. It starts with bilinear upsampling, followed by concatenation with the corresponding feature map from the contracting path. This output is then processed through two 3 × 3 convolutions followed by a ReLU activation function. This process is also repeated four times similarly to the contracting path. This design helps in preserving spatial information throughout the network and improving the network’s ability to localize features accurately. The final layer of the network consists of a 1 × 1 convolution, which produces a single-channel output image. This output image is the result of the pixel-wise regression performed by the U-Net model, i.e., a canopy height map derived from the multi-band input image. The decoder part of the network thus consists of 8 convolutional layers and 4 bilinear upsampling layers, maintaining symmetry with the encoder. The model, referred to as CHNET (Canopy Height estimation NETwork), and its components are described in detail in Figure 4. This figure provides a complete overview of the model’s architecture as well as the flow of data through the network’s layers, highlighting the encoder-decoder structure and the pixel-wise regression approach.
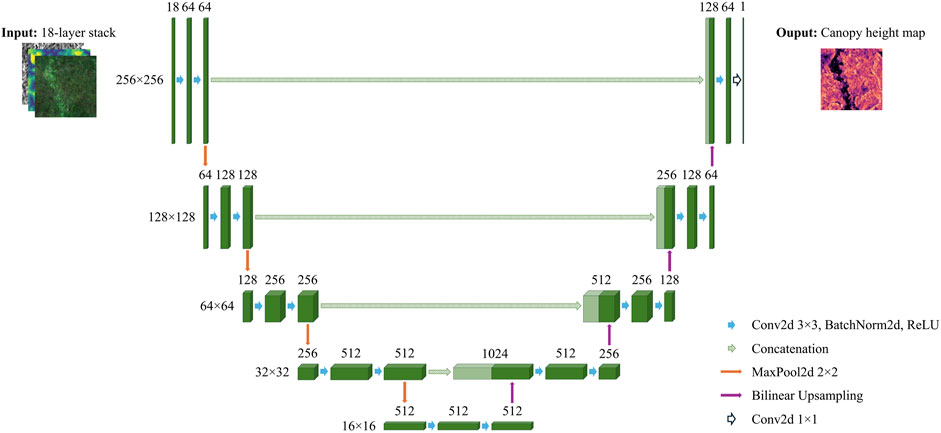
Figure 4. Overview of the CHNET framework. The input data consist of up to 18 layers depending on the training scenario. The output is a canopy height map. Image width and height as well as the number of channels are presented at each step of the model.
2.3.2 Experimental settings
To train the CHNET model, we employed a structured training process involving model calibration through a training/validation procedure (Ienco et al., 2017). The values of the different input sources were scaled between 0 and 1 (using minimum-maximum normalization) to help the model converge more quickly and efficiently by ensuring all features contribute proportionately to the learning process. The reference canopy height values (i.e., the GEDI rh_95 values) were spatially separated into training, validation, and test datasets. To do so, French Guiana was divided into tiles of 768 × 768 pixels, with each tile covering an area of approximately 60 km2. For model calibration, we only considered tiles with a minimum of 500 GEDI footprints, resulting in a total of 1,281 tiles. This dataset was allocated into training, validation, and test sets in an 80%-10%-10% split, corresponding to 1,024, 128, and 129 tiles, respectively. To avoid overfitting and improve the model’s generalization to unseen data, we employed random spatial sampling during the training process to increase the diversity of the training samples. Specifically, from each training tile, we extracted random subsets of 256 × 256 pixels containing at least one GEDI shot. This sampling process introduced variability by ensuring that each training batch included different spatial regions of the tiles. While the model still learns meaningful patterns, this approach prevents it from simply memorizing specific configurations present in the training data. Instead, this randomized extraction of image patches encourages the model to learn more general and robust features while reducing the likelihood of overfitting to specific data configurations, as it exposes the network to diverse spatial contexts and pixel-level variations. Each 256 × 256 input image comprised normalized layers from the various data sources described in Subsection 2.2, while the corresponding reference image contained rasterized GEDI rh_95 values.
The CHNET model’s output was compared to these reference heights using a robust adaptive loss function (Barron, 2017), which computed the loss only for geospatial locations with a valid rh_95 value. This loss function is a versatile definition that generalizes the Welsch (Dennis and Welsch, 1978), Geman-McClure (Geman and McClure, 1985), Cauchy (Black and Anandan, 1996), pseudo-Huber (Charbonnier et al., 1994), generalized Charbonnier (Sun et al., 2010), and L2 loss (least squared) functions. It is advantageous due to its capacity to adapt to varying noise levels and outliers in the data, providing a more stable and robust training process. As it unifies multiple common loss functions into a single formulation, it can adapt its shape based on the data it is optimizing and therefore it can handle a wide range of data distributions and noise levels. The loss function is defined as:
The parameters
2.3.3 Training scenarios
To understand the importance of each input data source, we designed four training scenarios based on combinations of different input layers and reference data. The first CHNET model (Scenario 1) was trained on optical and radar data as well as all the GEDI shots retained in the process described in Subsection 2.2. A first canopy height map of French Guiana at 10 m resolution was produced using this model. Following this first step, GEDI data were filtered again using the Scenario 1 canopy height map: for rh_95 values lower than 20 m, GEDI shots that presented a difference between Scenario 1 canopy height and rh_95 greater than 10 m were discarded. The goal of this filter was to remove low-height GEDI footprints that the model significantly overestimated, as these likely indicate unreliable GEDI data due to issues with vegetation signal penetration. Similarly, for rh_95 values greater than 50 m, GEDI shots that exhibited a difference between rh_95 and Scenario 1 canopy height greater than 10 m were removed. The goal of this filter was to eliminate GEDI shots that strongly overestimate height because of noise in the waveform that is interpreted as ground return by algorithm setting group number 5. The resulting refiltered GEDI database (1,875,608 shots, 16% of the initial dataset) was used as reference in Scenario 2. For the third model (Scenario 3), we enriched the Scenario 2 configuration with the environmental descriptors: HAND and FLT were added to the model input data. Finally, in Scenario 4 we accounted for GEDI geolocation uncertainty. Geolocation uncertainties in the context of GEDI refer to inaccuracies in determining precisely the spatial locations of the footprints on the Earth surface. The GEDI geolocation requirement as provided in the version 2 of the data products is that each footprint center is horizontally georeferenced to within 10 m, assuming normally distributed geolocation errors with a 0 m mean and a 10 m standard deviation (Dubayah et al., 2021). To address geolocation errors in our dataset, we implemented a controlled spatial perturbation (geo-correction) of each GEDI footprint center, systematically shifting them within both the X and Y directions across a radius of 10 m. Subsequently, for each possible spatial location, the optimal corrected footprint center was chosen based on the closest match to Scenario 3 canopy height map. The resulting corrected GEDI database was used as reference data for new model training in Scenario 4. Table 1 summarizes the characteristics of each scenario. The assessment of the models’ performances was done considering the independent GEDI test set, the ALS ground truth data, and the global canopy height map produced by Lang et al. (2023). The bias, the root mean square error (RMSE), and the relative RMSE (rRMSE) were chosen as performance metrics to evaluate the accuracies of our models.
2.3.4 Canopy height map production
To produce the final canopy height map of French Guiana at a spatial resolution of 10 m, the study site was divided into tiles of 256 × 256 pixels for the purpose of applying the trained CHNET model to predict canopy height. Each tile was processed individually, and the results were subsequently merged to produce the final canopy height map. Due to the inherent nature of convolutional layers in the U-Net architecture, CHNET tends to struggle with accurately predicting edge values within each tile. This is because convolutions, while effective at capturing local spatial patterns, can introduce boundary artifacts because of padding and reduce prediction accuracy at the edges. To solve this issue, we divided the study area into overlapping tiles to ensure that the edges of one tile overlap with the central region of adjacent tiles. This overlap allows for the edge values in the final canopy height map to be replaced by the corresponding values from the overlapping regions of the adjacent tiles, which are predicted with higher accuracy.
3 Results
3.1 Evaluation with GEDI data
The accuracies of each training scenario are assessed against the independent GEDI test dataset established during the data preparation for CHNET calibration (cf. Subsection 2.3). This dataset was not used in the model training and validation process and was kept specifically for assessing performances. Table 2 shows, for each training scenario, the accuracy metrics between canopy height predictions and rh_95 values from the GEDI test dataset.
The evaluation of canopy height estimates produced by CHNET against the GEDI test dataset reveals notable trends across the different scenarios. The model consistently demonstrates improvement across Scenarios 1 to 4, reflected in decreasing bias and RMSE values. The optimization of GEDI data used as reference height for model training (Scenario 2) allows reaching lower errors and relatively less biased estimates compared to the original GEDI dataset (Scenario 1). The integration of terrain and landscape descriptors (Scenario 3) further improves the results and exhibits a performance gain of about 10% in terms of RMSE compared to the model trained on optical and radar data only (Scenario 2). Notably, Scenario 3 also shows a transition from a negative to positive bias value, indicating a tendency to mitigate the underestimation observed in preceding scenarios. Accounting for GEDI geolocation uncertainty through the iterative geo-correction process (Scenario 4) brings a significant improvement and produces the best model in terms of accuracy, with an RMSE value of 5.7 m and a minimal bias of 0.7 m. All things considered, both the enhancement and the enrichment of input data induce notable improvements in the accuracies of CHNET, with a global RMSE gain of about 35% between Scenarios 1 and 4.
The GEDI test set is important for evaluating the behavior of our models on an independent dataset that was not involved in the learning process. It allows us to assess the generalizability and the robustness of the models. Additionally, characterizing the canopy height map using the entire GEDI reference data, including the training and validation datasets, is essential to evaluate the quality and accuracy of each scenario’s final map. By using all the available data, we can assess how the models perform across diverse conditions and regions. To achieve this, we computed the residuals of the models, defined as the differences between CHNET predicted canopy heights and GEDI reference values.
In particular, one key parameter to investigate is how the models perform across different canopy height classes, which is interesting given the height ranges found in French Guiana. Since most canopy heights in French Guiana forests range between 25 and 40 m (60% of the GEDI reference database), we analyzed the residuals according to the following height classes (in m): [15, 25], [25, 30], [30, 35], [35, 40], and >40. Heights below 15 m are quite infrequent in the study site and thus were excluded from the analysis. In the most relevant height ranges, i.e., between 25 and 40 m, we used a finer step of 5 m for each class. The box plot in Figure 5 illustrates the residuals (measured in m) of CHNET estimates across the GEDI reference height intervals for all training scenarios. The results show that for all scenarios, the residuals generally decrease as the GEDI reference height increases, indicating that the models tend to overestimate lower rh_95 values on one hand, and underestimate higher rh_95 values on the other hand. Regarding the overestimation, when reaching a reference height of up to 30 m, all scenarios still show positive residuals. The enhancement of GEDI data implemented in Scenario 2 and the inclusion of additional environmental descriptors in Scenario 3 do not yield better residuals for heights below 30 m compared to Scenario 1. Conversely, the geo-correction process developed in Scenario 4 results in lower residuals for heights between 15 and 30 m compared to prior scenarios, even though Scenario 1 still exhibits a slightly better performance. Regarding reference heights between 30 and 40 m, Scenario 4 produces unbiased estimates while other scenarios tend to underestimate canopy height. Overall, Scenario 1 exhibits the largest variability, with a tendency to consistently underestimate, especially in the [35, 40] m interval. Scenarios 2 and 3 bring notable improvements but are still outperformed by Scenario 4. Lastly, for heights greater than 40 m, all scenarios produce underestimated predictions, with Scenario 4 demonstrating the least underestimation and Scenario 1 the most pronounced.
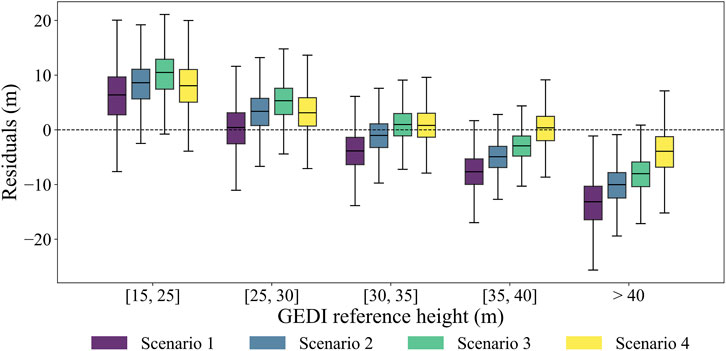
Figure 5. Residual analysis depending on canopy height class for all scenarios. Positive residuals indicate that predictions are greater than GEDI reference values. The boxplot shows the median, the quartiles, as well as the 10th and 90th percentiles.
Landscape type is another key parameter to investigate in the heterogeneous and diverse context of French Guiana’s forest ecosystems. In this perspective, we analyzed the residuals according to four main forest landscape classes: swamp, lowland, hill, and highland. The box plot in Figure 6 displays the residuals (measured in m) of CHNET estimates across these four forest landscape categories for all training scenarios. The results show that for all FLT, the residuals gradually decrease in absolute value as we progress from Scenario 1 to Scenario 4. Overall, Scenario 1 shows the biggest variability and consistently underestimates canopy height across all four landscape classes. Scenario 2 also presents a slight tendency to underestimate. A notable observation that can be drawn from these results is that the inclusion of HAND and FLT in model training (Scenario 3) results in unbiased estimates per forest class. Furthermore, the optimal approach is once again Scenario 4, which exhibits significantly lower variability in residuals. Regarding FLT in general, variability is typically larger in highland areas, which are known to present greater challenges for land remote sensing applications compared to other landscapes.
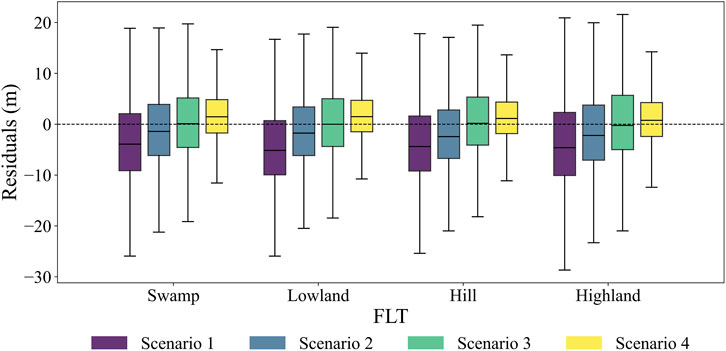
Figure 6. Residuals analysis depending on main forest landscape types (FLT) for all scenarios. Positive residuals indicate that predictions are greater than GEDI reference values. The boxplot shows the median, the quartiles, as well as the 10th and 90th percentiles.
3.2 Evaluation with independent ALS validation data
Canopy height models derived from ALS surveys were used to evaluate the performances of CHNET as well as to confirm the relevance of using GEDI height metrics as reference for canopy height. Table 3 presents, for each training scenario, the accuracy metrics between CHNET canopy height predictions and canopy heights retrieved from ALS data.
The evaluation of CHNET canopy height estimates against ALS data reveals a clear progression in accuracies across the four training scenarios. Overall, prediction models trained on GEDI data tend to underestimate canopy height, as highlighted by the negative bias values. Scenario 1, which is the one based on the least refined GEDI database in our comparative study, is characterized by a significant underestimation of canopy heights, with the strongest negative bias value of −6.6 m. The optimization of GEDI reference data implemented in Scenario 2 induces improvements, demonstrating the benefit of enhanced GEDI data integration. The most substantial improvements are observed in Scenarios 3 and 4, where the inclusion of additional environmental descriptors and the geo-correction process significantly improve the accuracy of canopy height estimates. This results in a global RMSE improvement of about 32% between Scenarios 1 and 4, along with a significant reduction in bias. The progressive rRMSE reduction from 13.2% in Scenario 1%–8.8% in Scenario 4 also underscores the effectiveness of these enhancements in providing more reliable canopy height estimates. Indeed, the geolocation correction (Scenario 4) nearly eliminates bias between CHNET estimates and ALS ground truth data. However, there is no notable enhancement observed in RMSE when performing geo-correction.
Figure 7 presents the scatter plot of Scenario 4 CHNET predictions against ALS ground truth data. Even though this configuration is the best performing model, it still exhibits noticeable difficulties at the edges, demonstrating a tendency to overestimate lower heights and to underestimate higher ones. However, it also shows robust performance within the height range of 30–40 m, which corresponds to the predominant height range observed in the forests of French Guiana. These findings are consistent with our earlier analysis using GEDI data (Figure 5), where Scenario 4 consistently provided accurate and unbiased estimates within the [30, 35] and [35, 40] m height intervals, while exhibiting tendencies towards overestimation at lower heights (lower than 30 m) and underestimation at higher heights (greater than 40 m).
3.3 Accuracies against global canopy height map
To assess our estimates relative to established models, we compared the CHNET predictions to Lang et al. high-resolution canopy height model of the Earth (Lang et al., 2023). Table 4 summarizes the comparison between the two models across the four different training scenarios. Overall, CHNET tends to underestimate canopy height when compared to Lang et al. estimates, as highlighted by the negative bias values. Enhancing the GEDI data used as reference to train CHNET (Scenario 2) induces a notable improvement with regards to Lang et al. model, achieving a minimal RMSE value of 4.3 m. However, incorporating environmental descriptors (Scenario 3) does not bring additional benefits compared to Scenario 2. The inclusion of HAND and FLT in our model does not lead to enhanced performance when assessed against Lang et al. map.
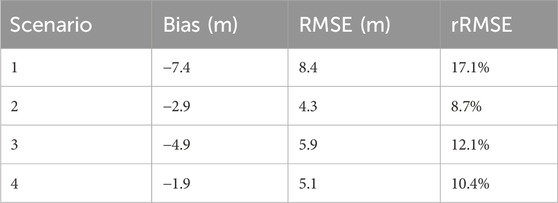
Table 4. Accuracy metrics of CHNET canopy height estimates vs. Lang et al. global canopy height model.
These observations are further refined when using ALS reference data to characterize both models together (Figure 8). In general, the residuals of Lang et al. estimates are consistently above those of CHNET, which explains the negative bias values reported in Table 4. Notably, for both Lang et al. and CHNET, the residuals increase as the ALS reference height decreases. Lang et al. overestimation tendency is particularly significant in the [15, 25] m height range. The key difference between the two models lies in the height at which the transition from overestimation to underestimation happens: Lang et al. tend to overestimate heights up to 35 m, whereas CHNET produces relatively unbiased estimates at 30 m. Conversely, for canopy heights greater than 35 m, Lang et al. outperforms CHNET, providing unbiased estimates in the [35, 40] m range and exhibiting less underestimation for canopy heights above 40 m.
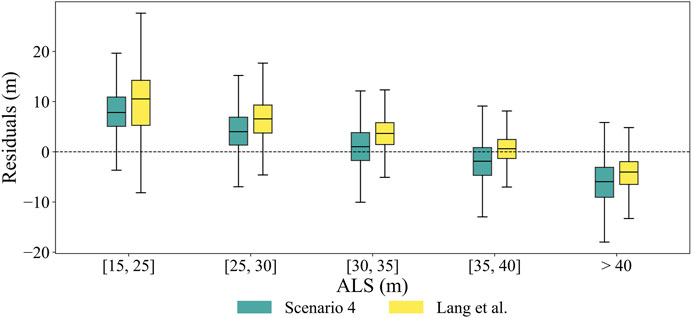
Figure 8. Residual analysis depending on ALS height class for Scenario 4 CHNET and Lang et al. map. Positive residuals indicate that predictions are greater than ALS reference values. The boxplot shows the median, the quartiles, as well as the 10th and 90th percentiles.
In assessing the performances of Lang et al. canopy height map, which has its own uncertainties like any model-based product, we compared it against the same GEDI test dataset and ALS data to understand its accuracy relative to our own map (Table 5). The analysis reveals that Lang et al. map generally tends to overestimate canopy heights in French Guiana, a pattern consistent with our previous findings where our CHNET model typically underestimates canopy height compared to Lang et al. estimates. When evaluated against ALS data, Lang et al. product exhibits a slightly better RMSE value than our CHNET framework. However, Lang et al. map also exhibits a notably higher positive bias, confirming a tendency to overestimate canopy height. In contrast, results from the GEDI test dataset are more favorable to our model. Specifically, Lang et al. map demonstrates significant error metrics, which are in line with the geographical error analysis presented in their study on held-out GEDI validation data.
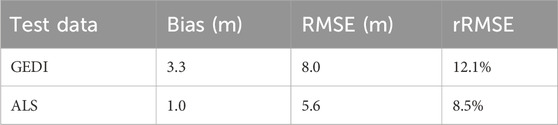
Table 5. Accuracy metrics of Lang et al. global canopy height model vs. GEDI test dataset and ALS data.
3.4 Canopy height map
We chose to implement the Scenario 4 CHNET model to the whole area of interest, as it yielded the best accuracies relative to GEDI and ALS reference data. Moreover, this approach is also the most developed as it relies on all available data and uses an optimized GEDI database for training. Figure 9 presents the results obtained and the final canopy height map.
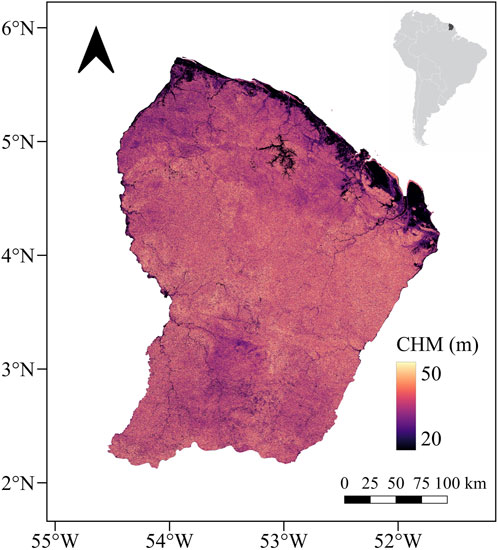
Figure 9. Canopy Height Model (CHM) of French Guiana at 10-m resolution predicted by CHNET built under the configuration of Scenario 4.
Despite CHNET being trained and validated on sparse reference data (rasterized GEDI rh_95 metric), it successfully generates a continuous canopy height map with good precision. Indeed, this map offers a comprehensive depiction of forest structures and other landscape features observable in the remote sensing data. It effectively distinguishes height differences across the study area while describing the spatial distribution and variability of canopy heights in French Guiana.
4 Discussion
In this study, a U-Net model was assessed under different training conditions to generate a canopy height map of French Guiana. Each consecutive training scenario involved optimizing and enriching the data used in the learning process. Ultimately, the model that incorporated diverse data sources, including optical, radar, and environmental parameters, along with a refiltered and geolocation-corrected GEDI database, yielded the best results. This optimized CHNET model was applied to the entire study area to produce a high-resolution 10-m map of canopy height across French Guiana (Figure 9).
4.1 On the challenges of GEDI data
To train our CHNET model, a GEDI height metric from the L2A product was used as direct indicator of canopy height. Using GEDI metrics as ground truth data and considering them as a reliable representation of canopy height is the most straightforward and the most used approach in deep learning applications (Lang et al., 2023; Schwartz et al., 2024; Fayad et al., 2024). Theoretically, rh_100 represents the top of the canopy cover, but other high-percentile RH metrics are used in practical applications because they are less sensitive to noise and contain less outliers (Li et al., 2024). We specifically chose the rh_95 metric in accordance with a previous study where this metric showed the best performance in the dense tropical context of French Guiana (Lahssini et al., 2022). Other studies have also advocated for the use of this metric (Potapov et al., 2021; Lahssini et al., 2024; Dorado-Roda et al., 2021), although metrics like rh_98 have also been used (Lang et al., 2023). Despite GEDI’s unprecedented capabilities for forest structure characterization, there are still limitations to using these data as reference ground truth for empirical model calibration. Especially in our study area, the ability of GEDI to accurately retrieve canopy height in complex, tall, and dense forest structures is still uncertain. In tropical biomes, the GEDI signal may sometimes not penetrate enough to reach the ground, leading to incorrect height estimations (Lahssini et al., 2022). Moreover, the uncertainties in the GEDI footprint locations can lead to significant errors (Roy et al., 2021). Our training scenarios in this study were specifically designed to address and mitigate these existing challenges.
The GEDI L2A metrics are based on the L2A processing algorithm, which utilizes six different sets of parameters for waveform processing. Consequently, there are six possible values for canopy height for any given footprint. We opted for the data derived from algorithm setting group number 5, as previous research indicated it delivered significantly better accuracy than other groups, including the GEDI automatic algorithm selection, and particularly in the tropical context of French Guiana (Lahssini et al., 2022; Liu et al., 2024). Algorithm setting group number five is defined by the lowest waveform signal end threshold compared to other groups, which allows for better extraction of low-intensity ground returns. However, this advantage comes with a trade-off, as algorithm setting group number five tends to overestimate lower heights. Low-height areas are generally not concerned by signal penetration challenges. Due to its greater sensitivity to noise, algorithm setting group number 5 may interpret noise below the actual ground as information on ground elevation, leading to overestimations of low canopy heights. This behavior is observed in the results of our CHNET model and is consistent with similar models in existing literature (Schneider et al., 2020; Schlund et al., 2023). In our case, using GEDI metrics computed with the parameters of algorithm setting group number five to calibrate CHNET tends to produce overestimated predictions for heights lower than 30 m. While algorithm setting group number five offers a better detection of weak ground returns, the parameters of this signal processing algorithm naturally lead to an overestimation of lower heights. Alternative approaches could be explored to mitigate this overestimation of lower heights. For instance, Potapov et al. (2021) adopted an approach in their global canopy height map where they averaged the middle four values of the RH metrics across all six algorithm configurations. Given the global scale of their study, relying on a single configuration would not be suitable to capture the diversity of forest structures and ecosystems accurately. Their method, used in their global dataset, aims to harmonize GEDI metrics across diverse biomes by avoiding extreme values (minimum and maximum of the six setting groups) and minimizing the biases introduced by a single algorithm configuration. Similarly, in their supervised machine learning approach to interpret GEDI waveforms and regress canopy top height globally, Lang et al. (2022) emphasized that, while algorithm setting group number 2 performed best over all continental areas, the performance of individual algorithm setting groups changes regionally. These findings, although obtained at global scales, suggest that local adaptations could possibly improve the reference heights used to train our model. Given the variety of forest landscapes across French Guiana, future studies could consider the use of different GEDI algorithm setting groups for different forest types. By exploiting several algorithm settings each adapted to the specificities of distinct areas, it might be possible to reduce the overestimation of lower canopy heights, a known limitation of algorithm setting group number 5, while preserving the accuracy for taller canopies.
While GEDI tends to overestimate lower canopy heights, it conversely underestimates higher canopy heights. Even though we are using the algorithm setting group with the lowest signal end threshold, a phenomenon of underestimation is present for high heights. Existing standard methods for canopy height estimation often face challenges in accurately measuring tall canopies, with height estimates typically saturating at approximately 25–30 m (Potapov et al., 2021; Healey et al., 2020). In our case, the transition appears at around 35 m. For heights greater than 35 m, our CHNET model, which is built on GEDI data, starts to underestimate canopy height. Tall canopies are generally associated with densely vegetated areas, which pose a significant challenge for GEDI laser signal penetration. This issue is particularly present with coverage beams, which are half-power beams compared to the full-power configuration. Pulses emitted with coverage lasers generally have more difficulties reaching the ground because of the density of the vegetation, causing the extracted ground peak in the waveforms to appear at a higher elevation than the actual ground level. This discrepancy leads to an overestimation of the ground height and, consequently, and underestimation of canopy heights. Several studies have highlighted the link between laser energy and vegetation penetration. For example, Fayad et al. (2022) observed that coverage lasers exhibited significantly lower performance for canopy height estimation compared to full-power signals such as GEDI power beams and NASA’s Land Vegetation and Ice Sensor (LVIS). In this context, many studies recommend using only high-power beams for better canopy height estimates (Lahssini et al., 2022; Liu et al., 2021; Wang et al., 2022). However, deep learning approaches heavily rely on a sufficient amount of reference data for training, which is why most models built on GEDI data do not filter by beam type and use all the available footprints (Lang et al., 2023; Schwartz et al., 2024; Potapov et al., 2021). Furthermore, full-power beams also face challenges when penetrating dense canopies, as laser energy is not the only parameter affecting signal penetration. According to specifications, the GEDI instrument is capable of measuring vertical canopy profiles in environments with up to 95% canopy cover for coverage beams and up to 98% for power beams (Dubayah et al., 2020). All things considered, in a region like French Guiana, GEDI inherently exhibits a tendency to underestimate tall canopy heights in densely vegetated areas, which in turn impacts the CHNET model estimates in the same way. The saturation of optical and radar data also contributes to this underestimation.
In our study, we explored various training scenarios to optimize canopy height estimation from GEDI data, and we found that the inclusion of geolocation correction for GEDI footprints produced the most accurate results. GEDI geolocation uncertainty is a major challenge that limits the benefits of the data. It is a common issue across all remote sensing instruments due to orbital dynamics, instrument calibration, and atmospheric disturbances (Roy et al., 2021). Addressing these uncertainties is crucial for any GEDI-based application and various studies proposed mitigation strategies (Hancock et al., 2019; Shannon et al., 2024; Schleich et al., 2023; Tang et al., 2023). The expected accuracy of the GEDI version 2 data product is 10 m. In a previous study, we had estimated that geolocation uncertainty might account for approximately half of the error observed in canopy height estimates (Lahssini et al., 2024). To address geolocation errors, we implemented an iterative spatial perturbation of each GEDI footprint center, leveraging previously developed models to refine footprint locations. This approach significantly improved the accuracy of canopy height estimates through CHNET, demonstrating the potential benefits of using an enhanced GEDI database. Importantly, these enhancements can be achieved in an operational context without the need for additional validation datasets, relying solely on an iterative process that consists in consecutive model development under diverse training scenarios. Nonetheless, no reduction in error was observed when comparing model performance against ALS data before and after geo-correction (Table 3). This could be explained by the nature of the correction applied. The geo-correction improves the spatial accuracy of the GEDI footprints’ locations, which is reflected in the improved performance of the model when compared against the GEDI test set (Table 2). This is expected because we are directly correcting GEDI information. However, ALS data is independent and has no direct connection to the GEDI footprints’ locations. The discrepancy between GEDI and ALS measurements could limit the impact of the geo-correction. Since the model was trained on GEDI data, it may still carry the biases inherent to GEDI, and these would persist even after geo-correction when compared to ALS reference canopy heights. In previous training scenarios (i.e., Scenarios 1–3), we introduced new data or removed irrelevant information, which gave the model a different perspective on canopy height, and improvements were seen in comparison to ALS. In Scenario 4, no new data is added or removed (only a spatial correction is performed), and it could explain why no improvement in error is observed when assessing the model against ALS data.
Overall, using GEDI RH metrics as a direct and reliable proxy for canopy height has limitations due to the characteristics of the GEDI sensor. However, when mapping large areas using an empirical approach like CHNET, it is essential to have enough reference data for training. The optimal reference data would come from in-situ measurements or high-resolution sensors such as ALS. In our case, while we do have ALS data, it is insufficient for training our model, because it covers a relatively small area compared to the entire study site (less than 2%) and it is limited to the north of French Guiana, thus lacking diversity for a robust training set. To understand the accuracy of GEDI data and its relevance as reference data, we performed a comparison between GEDI rh_95 values and the available ALS data. The results, as shown in Figure 10, indicate that GEDI is far from being a perfect ground truth, with an RMSE of 8.2 m and a tendency to underestimate canopy height, with a bias of −1.6 m. A large portion of the CHNET model’s error can therefore be attributed to GEDI’s inaccuracies. In a previous study, Lahssini et al., 2022 demonstrated that reference canopy height values could be improved by building models based on RH metrics and calibrated on actual ground truth data. While such an approach is effective at local scales, it becomes challenging at a regional scale, such as across the entirety of French Guiana, due to the limited availability of ALS data over the entire study site. When studying larger study areas using GEDI data, an iterative process of refining and enhancing the input reference data at each step proved effective. Indeed, it allowed bringing notable improvements and partly overcoming the challenges of working with GEDI data.
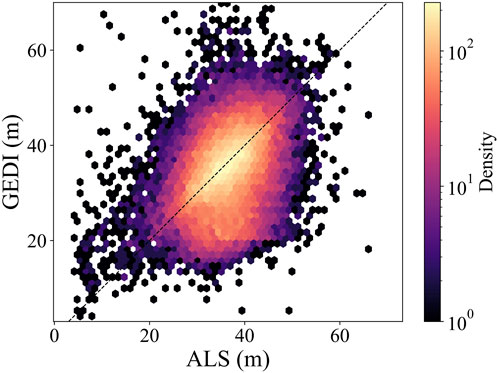
Figure 10. GEDI rh_95 (Scenario 3) as a function of ALS ground truth data. We used the Scenario 3 GEDI reference data to highlight the actual relationship between GEDI data as they are provided and ALS.
4.2 On the value of input data sources
The complementarity between optical and radar is particularly valuable to derive canopy height, as each sensor provides specific information. They are commonly used to characterize forest ecosystems, and their complementarity has been leveraged in several studies across a range of biomes, including temperate and tropical regions (Schwartz et al., 2024; Morin et al., 2023; Fagua et al., 2019). Optical data, such as that from S2, is generally known for its ability to provide information related to stand composition (Karasiak et al., 2017; Grabska et al., 2019). However, it also offers valuable features for characterizing structural parameters of the vegetation, especially through certain spectral bands (Lahssini et al., 2022). In fact, the reflectance of a pixel is primarily influenced by the characteristics of the foliage, such as its spectral properties, quantity, and orientation, rather than trunk biomass or tree height. However, the spatial arrangement of pixels, often referred to as texture, gives valuable information that can be linked to structural parameters of the forest. Deep learning techniques consider both spectral and textural aspects. In our case, the CHNET model’s architecture allows extracting and linking local spatial features to canopy height. Other studies using machine learning algorithms have demonstrated that incorporating multispectral data enhances the accuracy of forest canopy height estimation and decreases the uncertainty of these estimates, even for tall forests (García et al., 2018). However, optical data can be limited by the saturation of reflectance in tropical forests, and this saturation makes it hard to distinguish vegetation heights above a certain level (Simard et al., 2011). Sentinel-2 data alone are not the optimal source for predicting forest variables that are mainly influenced by tree and stand structure, especially canopy height.
Therefore, to build our CHNET model, we complemented these data with other sources, particularly radar information. In this study, we utilized two radar satellite platforms operating in different bands: C-band for S1 and L-band for ALOS. Different polarizations of radar data, as implemented in our CHNET model, further enhance forest characterization. HH and VV polarizations are generally associated with surface scattering and can be useful for assessing forest density and structure (Wijaya et al., 2015). Cross-polarizations like HV and VH are more sensitive to volume scattering, which can help describe canopy structure (Ulaby et al., 1990). In the future, the European Space Agency (ESA) planned BIOMASS mission, which is specifically designed for forest structure and biomass estimation, will collect P-band SAR data and provide global estimates of forest biomass and height (Quegan et al., 2019). P-band SAR has a significantly longer wavelength compared to C-band or L-band systems, which allows penetrating dense canopies more effectively. Indeed, longer wavelengths are less affected by canopy scattering and absorption, allowing for greater penetration into the canopy (Khati et al., 2018). Moreover, even L-band radar signals are known to encounter difficulties penetrating canopies when biomass levels are beyond 150 Mg/ha (Mermoz et al., 2015), a common scenario in French Guiana. Specifically for the BIOMASS mission, dual-polarization and interferometric capabilities will also bring a valuable opportunity for better discrimination of different types of vegetation and terrain, making it an ideal complement to GEDI data.
In our study, we observed that enriching the remote sensing input data with environmental descriptors HAND and FLT induced a significant enhancement in the canopy height estimates produced by CHNET. Environmental parameters generally impact both the studied environment and the data collected by the sensors acquiring measurements over that environment. Specifically, hydrological and geomorphological descriptors like HAND and FLT are directly related to forest state, structure, and dynamics. They provide valuable contextual information that complements optical and radar data. HAND gives contextual information about a pixel’s vertical proximity to the river within its watershed. Such information can relate to water table depth, access to water sources through aboveground or belowground drainage flows, and variations in vegetation species along drainage transects. For example, Schietti et al. (2014) identified a link between HAND and floristic composition changes in the Amazon. HAND captures the elevation relative to drainage networks, which influences water availability and soil moisture, critical factors affecting in turn vegetation growth, canopy height, and AGB. Indeed, some studies have established a link between HAND and forest structural parameters. For example, the link between HAND and biomass in Eucalyptus clonal plantations in Brazil indicates that the functioning and the dynamics of a same species can be very different over a gradient of vertical distance from the river (Stape and Alvares, 2023). In a region like French Guiana, characterized by complex and highly developed water networks as well as a rich variety of species, hydrological configuration therefore strongly impacts forest structure. Incorporating HAND into our CHNET model provides unique and specific information on canopy height that is not available from the other data sources we used, which explains the observed improvements in model performance.
Similarly, FLT categorizes forest types based on their landscape characteristics These characteristics include soil type, geological and geomorphological factors, terrain elevation, and vegetation types. This categorization provides key insights into the structural diversity of French Guiana’s forest ecosystems, and those factors ultimately impact the spatial arrangements of canopy height. Integrating FLT data into the inputs of CHNET helps the model gain a more detailed understanding of how different forest landscape types exhibit distinct canopy height profiles. In their study on canopy height estimation from multi-source remote sensing data using a Random Forest model, Jin et al. (2018) noted that models trained on given locations or vegetation types were not transferable to other settings. However, they also found that training their algorithm with data from diverse sites and vegetation types enabled the development of a universal model capable of accurately predicting canopy height across various locations and vegetation types. Our results also underline the importance of including environmental descriptors when implementing and applying a model to extensive areas characterized by diverse ecological conditions. HAND and FLT contain environmental information that is directly linked to ecological processes influencing canopy dynamics, and the CHNET model effectively incorporates this information alongside optical and radar inputs to identify complex relationships between environmental descriptors and canopy height. It is important to note that the FLT map utilized in this study was produced in 2015 by ONF and incorporates data on human activities to map non-forested areas, as reflected in the NA class in Figure 3. This includes areas affected by human disturbances up to 2015, such as urbanization and historical logging or mining. However, more recent disturbances, such as illegal logging and mining activities after 2015, or natural forest degradation, are not represented. While this limitation could affect canopy height predictions by potentially underestimating the impact of human activities, the conclusions of our study remain robust. Nonetheless, accounting for these disturbances could further refine the input data used by the CHNET model. The Joint Research Center’s (JRC) Tropical Moist Forest (TMF) dataset (Vancutsem et al., 2021), which provides detailed records of forest cover and land use changes in tropical regions, could be a useful resource in this regard. It has been successfully used in other studies to focus on undisturbed forested areas and facilitate consistent comparisons across datasets from different periods (Lahssini et al., 2024). Even if all data sources used in this study were obtained from relatively contemporary acquisitions, the inclusion of ancillary information about forest cover changes could still allow for an interesting refinement of the input data, by filtering out even more disturbed or non-forested areas beyond what the FLT’s NA class shows (Figure 3). Using this additional information, the CHNET model would gain a more accurate understanding of land use and forest type during its training process, which would potentially lead to better accuracy in canopy height estimations.
While the CHNET model does not allow for traditional feature importance analysis (as is possible in machine learning models like Random Forest), we assessed the contribution of environmental predictors by comparing models trained with and without these inputs. The observed improvement in canopy height predictions suggests that HAND and FLT are indeed informative because of their strong correlation with forest structural properties, which has been noted in previous studies (Rennó et al., 2008; Fayad et al., 2016). Although HAND and FLT were effective in this study, other candidate variables could also be explored to further improve the CHNET model’s canopy height estimates. Yang et al., 2016 found that climate and soil factors, such as water stress, precipitation, and soil fertility, can be significant in explaining canopy height variations at large scales. They suggested that soil and climate jointly explain a quite significant portion (about 30%) of height variability in tropical forests. Fayad et al. (2016) also highlighted the importance of environmental variables like topography, geological features, and rainfall data in mapping forest biomass in French Guiana, which correlates with forest structure and canopy height. Their study emphasized that variables capturing terrain and hydrological conditions, similar to the information given by the HAND variable, are key to explaining AGB distribution. However, it is also important to consider that not all abiotic variables will always improve model performance. For example, while Yang et al., 2016 noted that soil and climate variables can explain large-scale height variations, they might not be as directly useful for small-scale canopy height predictions, because local terrain and forest characteristics often dominate at such scales. Therefore, the inclusion of these variables was not prioritized in our study due to the specific ecological and geographical context of French Guiana, where HAND and FLT were expected to play a dominant role in forest structure variability. Future work could nonetheless explore incorporating other candidate variables, such as soil properties or climatic factors, to test whether they could add further predictive power, particularly in regions with distinct ecological characteristics. While these additional variables might be informative, they should be chosen carefully based on their relevance to canopy structure and the specific scale of the study.
4.3 On the relevance of the U-Net architecture
When dealing with heterogeneous data sources and sparse reference data, the U-Net architecture represents a particularly robust approach. It is well-suited for tasks involving complex data integration and spatial feature extraction due to its structure that combines a contracting path to capture context and an expanding path to enable precise localization (Ronneberger et al., 2015). Previous research has indeed shown that performance decreases significantly, especially for tall canopies, when models are unable to learn spatial features (Lang et al., 2019). The CHNET model’s capacity to derive and analyze features from diverse inputs, i.e., optical and radar remote sensing data alongside environmental descriptors, is a key advantage that we leveraged in this study to produce our final canopy height map. Remote sensing data inherently contains both spectral and spatial features, and the U-Net architecture is designed to efficiently extract and interpret these multi-dimensional features. The convolutional layers in U-Net neural networks are great at capturing both spectral variations and spatial patterns directly from the raw data, thus removing the need for preliminary feature engineering like computing vegetation indices or other spectral indicators. In their canopy height model of the Earth produced with an ensemble of FCNs, Lang et al., 2023 noted that while there is a good correlation between the Normalized Difference Vegetation Index (NDVI) and the estimated canopy height, the relationship learned by the model between the S2 image features and canopy height is much more complex and cannot be fully described by a straightforward index like NDVI. Therefore, the direct utilization of raw spectral bands ensures that the model can exploit the full richness of the input data.
Furthermore, the U-Net architecture is advantageous when reference data are sparse, which is the case of GEDI measurements. This capability is of paramount importance when mapping canopy height over a large region such as French Guiana, where the only reference data available in sufficient quantity for model training consists of GEDI measurements that are sparsely and unevenly distributed (Figure 1). The model’s ability to generalize from sparse reference points ensures that predictions are spatially consistent across the entire study area. This characteristic was particularly beneficial when training our CHNET model on sparse reference GEDI data and when applying it to produce our final canopy height map. Moreover, given the complexity of the factors impacting canopy height, we chose to build our CHNET model using multiple complementary data sources through a data fusion approach. Indeed, hydrological and geomorphological descriptors related to forest growth have demonstrated their ability to provide supplementary information not found in classical remote sensing data. The strategy for integrating and combining all this information together is important for maximizing its value. Some approaches process each data source separately using dedicated encoders before performing data fusion (Chen et al., 2024). In our study, the convolutional approach in the CHNET framework preserves the spatial hierarchies and relationships within the data, which is crucial for accurately mapping a variable like canopy height that varies continuously across landscapes (Lang et al., 2019). Convolutional filters allow linking multi-dimensional features with the target variable. All things considered, the U-Net model’s ability to integrate multi-source data, to identify relevant spectral and spatial features without the need for pre-computed indicators, and to perform robustly with sparse reference data makes it a relevant choice for producing a high-resolution canopy height map of French Guiana in the conditions described in this study. While more recent segmentation architectures like Transformer-based models (Fayad et al., 2024; Tolan et al., 2024) or attention-enhanced networks (Zhao et al., 2024) have shown success in forest canopy height retrieval, the U-Net’s well-established performance and efficiency in handling multimodal data, combined with its relatively lightweight architecture, made it the more practical choice for the scale and resolution of this study. Newer architectures might offer enhanced feature extraction, but they also often come with increased computational and memory requirements. Hence, the choice of U-Net allowed us to reach a balance between computational efficiency and model performance. Moreover, the study’s significant conclusions are about the data themselves, as we achieved clear and consistent improvements in our canopy height predictions with U-Net. In the end, our findings highlight that, regardless of the architecture employed, the quality and integration of the data are important factors in producing accurate canopy height estimates.
5 Conclusion
This study presents an operational deep learning application that leverages multi-source satellite data and ancillary environmental information, all of which are freely available to users on a global scale. We demonstrate the potential of a U-Net architecture trained on sparse and unevenly distributed data to generate a continuous map of canopy height at a regional scale. Our assessment reveals that GEDI can be effectively used to generate canopy height products, particularly when integrated with other data sources into a deep learning estimation model.
Our CHNET framework successfully produced a canopy height map of French Guiana at a 10-m spatial resolution with relatively good accuracy. Notably, we observe that it is essential to account for GEDI inaccuracies as well as geolocation uncertainties, and this consideration should be integrated into any GEDI-based application. The iterative geolocation correction approach presented in this study is novel in that, unlike many existing correction methods that rely on external datasets (for example, airborne LiDAR point clouds) and calibration procedures, it is highly operational and does not require additional data to be performed. Given its efficiency and simplicity, this approach could be explored for use in other regions, both tropical and non-tropical, to enhance the accuracy of GEDI-based applications. Moreover, considering ancillary data that provides complementary insights into the structural dynamics of the canopy, which classical remote sensing data cannot fully reveal, has proven to be of significant interest. Incorporating relevant environmental descriptors closely linked to forest growth contributes significantly to the model’s accuracy, as our deep learning framework CHNET was able to leverage these complex relationships to improve estimates. The integration method of multimodal data is crucial for fully exploiting the diverse and multi-level information contained in the different input layers, especially given the specificities of GEDI reference data.
Despite these advancements, challenges remain, particularly in accurately assessing tall canopies with GEDI data. Addressing these challenges is critical in tropical forest studies. For instance, improved GEDI-derived reference canopy height values could be obtained by applying linear or nonlinear models based on GEDI RH metrics and calibrated on actual ground data, such as ALS, provided that there is a sufficient amount of data available to build these models. Future improvements in the characterization of dense canopy structures are essential for better understanding tropical forest ecosystems and the Earth’s carbon cycle, with a need for both enhanced data quality and improved methodologies.
Data availability statement
The original contributions presented in the study are included in the article/supplementary material, further inquiries can be directed to the corresponding author.
Author contributions
KL: Conceptualization, Data curation, Formal Analysis, Investigation, Methodology, Software, Validation, Visualization, Writing–original draft. NB: Conceptualization, Funding acquisition, Investigation, Methodology, Supervision, Validation, Writing–review and editing. GL: Conceptualization, Investigation, Methodology, Supervision, Validation, Writing–review and editing. IF: Conceptualization, Investigation, Methodology, Software, Validation, Writing–review and editing. LV: Data curation, Writing–review and editing.
Funding
The author(s) declare that financial support was received for the research, authorship, and/or publication of this article. This research received funding from the National Center for Space Studies (CNES, TOSCA 2024 project) and the National Research Institute for Agriculture, Food and the Environment (INRAE).
Acknowledgments
The authors would like to thank the GEDI team and NASA’s LP DAAC for providing the GEDI data. The authors also wish to thank the National Forests Office (ONF) and Caroline Bedeau for processing and providing the ALS data.
Conflict of interest
Author IF was employed by Kayrros SAS.
The authors declare that the research was conducted in the absence of any commercial or financial relationships that could be construed as a potential conflict of interest.
Publisher’s note
All claims expressed in this article are solely those of the authors and do not necessarily represent those of their affiliated organizations, or those of the publisher, the editors and the reviewers. Any product that may be evaluated in this article, or claim that may be made by its manufacturer, is not guaranteed or endorsed by the publisher.
References
Adam, M., Urbazaev, M., Dubois, C., and Schmullius, C. (2020). Accuracy assessment of GEDI terrain elevation and canopy height estimates in European temperate forests: influence of environmental and acquisition parameters. Remote Sens. 12 (23), 3948. doi:10.3390/rs12233948
Asner, G. P., and Mascaro, J. (2014). Mapping tropical forest carbon: calibrating plot estimates to a simple LiDAR metric. Remote Sens. Environ. 140 (January), 614–624. doi:10.1016/j.rse.2013.09.023
Baghdadi, N., Le Maire, G., Bailly, J.-S., Ose, K., Nouvellon, Y., Zribi, M., et al. (2015). Evaluation of ALOS/PALSAR L-band data for the estimation of Eucalyptus plantations aboveground biomass in Brazil. IEEE J. Sel. Top. Appl. Earth Observations Remote Sens. 8 (8), 3802–3811. doi:10.1109/JSTARS.2014.2353661
Barenblitt, A., Fatoyinbo, L., Thomas, N., Stovall, A., De Sousa, C., Nwobi, C., et al. (2024). Invasion in the Niger delta: remote sensing of mangrove conversion to invasive Nypa fruticans from 2015 to 2020. Remote Sens. Ecol. Conservation 10 (1), 5–23. doi:10.1002/rse2.353
Barron, J. T. (2017). A general and adaptive robust loss function. arXiv. doi:10.48550/ARXIV.1701.03077
Beck, H. E., Zimmermann, N. E., McVicar, T. R., Vergopolan, N., Berg, A., and Wood, E. F. (2018). Present and future köppen-geiger climate classification maps at 1-km resolution. Sci. Data 5 (1), 180214. doi:10.1038/sdata.2018.214
Berninger, A., Lohberger, S., Stängel, M., and Siegert, F. (2018). SAR-based estimation of above-ground biomass and its changes in tropical forests of kalimantan using L- and C-band. Remote Sens. 10 (6), 831. doi:10.3390/rs10060831
Black, M. J., and Anandan, P. (1996). The robust estimation of multiple motions: parametric and piecewise-smooth flow fields. Comput. Vis. Image Underst. 63 (1), 75–104. doi:10.1006/cviu.1996.0006
Bouvet, A., Mermoz, S., Ballère, M., Koleck, T., and Toan, T.Le (2018). Use of the SAR shadowing effect for deforestation detection with sentinel-1 time series. Remote Sens. 10 (8), 1250. doi:10.3390/rs10081250
Boyd, D. S., and Danson, F. M. (2005). Satellite remote sensing of forest resources: three decades of research development. Prog. Phys. Geogr. Earth Environ. 29 (1), 1–26. doi:10.1191/0309133305pp432ra
Catalogue des habitats forestiers de Guyane. (2015). Cayenne (Guyane): direction régionale ONF Guyane.
Charbonnier, P., Blanc-Feraud, L., Aubert, G., and Barlaud, M. (1994). “Two deterministic half-quadratic regularization algorithms for computed imaging,” in Proceedings of 1st international Conference on image processing, 2 (Austin, TX, USA: IEEE Comput. Soc. Press), 168–172. doi:10.1109/ICIP.1994.413553
Chave, J., Andalo, C., Brown, S., Cairns, M. A., Chambers, J. Q., Eamus, D., et al. (2005). Tree allometry and improved estimation of carbon stocks and balance in tropical forests. Oecologia 145 (1), 87–99. doi:10.1007/s00442-005-0100-x
Chen, M., Dong, W., Hao, Yu, Woodhouse, I. H., Ryan, C. M., Liu, H., et al. (2024). Multimodal deep learning enables forest height mapping from patchy spaceborne lidar using sar and passive optical satellite data. doi:10.2139/ssrn.4898106
Corbane, C., Politis, P., Kempeneers, P., Simonetti, D., Soille, P., Burger, A., et al. (2020). A global cloud free pixel- based image composite from sentinel-2 data. Data Brief 31 (August), 105737. doi:10.1016/j.dib.2020.105737
Cresson, R., and Saint-Geours, N. (2015). Natural color satellite image mosaicking using quadratic programming in decorrelated color Space. IEEE J. Sel. Top. Appl. Earth Observations Remote Sens. 8 (8), 4151–4162. doi:10.1109/JSTARS.2015.2449233
De Thoisy, B., Richard-Hansen, B. C., Bertrand, G., Joubert, P., Obstancias, J., Winterton, P., et al. (2010). Rapid evaluation of threats to biodiversity: human footprint score and large vertebrate species responses in French Guiana. Biodivers. Conservation 19 (6), 1567–1584. doi:10.1007/s10531-010-9787-z
Dennis, J. E., and Welsch, R. E. (1978). Techniques for nonlinear least squares and robust regression. Commun. Statistics - Simul. Comput. 7 (4), 345–359. doi:10.1080/03610917808812083
Dorado-Roda, I., Pascual, A., Godinho, S., Silva, C., Botequim, B., Rodríguez-Gonzálvez, P., et al. (2021). Assessing the accuracy of GEDI data for canopy height and aboveground biomass estimates in mediterranean forests. Remote Sens. 13 (12), 2279. doi:10.3390/rs13122279
Dubayah, R., Bryan Blair, J., Scott, G., Fatoyinbo, L., Hansen, M., Healey, S., et al. (2020). The global ecosystem dynamics investigation: high-resolution laser ranging of the Earth’s forests and topography. Sci. Remote Sens. 1 (June), 100002. doi:10.1016/j.srs.2020.100002
Dubayah, R., Hofton, M., Blair, J., Armston, J., Tang, H., and Luthcke, S. (2021). “GEDI L2A elevation and height metrics data global footprint level V002,” in NASA EOSDIS land processes distributed active archive center. doi:10.5067/GEDI/GEDI02_A.002
Fagua, J. C., Jantz, P., Rodriguez-Buritica, S., Duncanson, L., and Goetz, S. J. (2019). Integrating LiDAR, multispectral and SAR data to estimate and map canopy height in tropical forests. Remote Sens. 11 (22), 2697. doi:10.3390/rs11222697
Fassnacht, F. E., Poblete-Olivares, J., Rivero, L., Lopatin, J., Ceballos-Comisso, A., and Galleguillos, M. (2021). Using sentinel-2 and canopy height models to derive a landscape-level biomass map covering multiple vegetation types. Int. J. Appl. Earth Observation Geoinformation 94 (February), 102236. doi:10.1016/j.jag.2020.102236
Fayad, I., Baghdadi, N., Alvares, C. A., Stape, J. L., Bailly, J. S., Scolforo, H. F., et al. (2021). Terrain slope effect on forest height and wood volume estimation from GEDI data. Remote Sens. 13 (11), 2136. doi:10.3390/rs13112136
Fayad, I., Baghdadi, N., Bailly, J.-S., Barbier, N., Gond, V., Hajj, M., et al. (2014). Canopy height estimation in French Guiana with LiDAR ICESat/GLAS data using principal component analysis and random forest regressions. Remote Sens. 6 (12), 11883–11914. doi:10.3390/rs61211883
Fayad, I., Baghdadi, N., Guitet, S., Bailly, J.-S., Hérault, B., Gond, V., et al. (2016). Aboveground biomass mapping in French Guiana by combining remote sensing, forest inventories and environmental data. Int. J. Appl. Earth Observation Geoinformation 52 (October), 502–514. doi:10.1016/j.jag.2016.07.015
Fayad, I., Baghdadi, N., and Lahssini, K. (2022). An assessment of the GEDI lasers’ capabilities in detecting canopy tops and their penetration in a densely vegetated, tropical area. Remote Sens. 14 (13), 2969. doi:10.3390/rs14132969
Fayad, I., Ciais, P., Schwartz, M., Wigneron, J.-P., Baghdadi, N., De Truchis, A., et al. (2024). Hy-TeC: a hybrid vision transformer model for high-resolution and large-scale mapping of canopy height. Remote Sens. Environ. 302 (March), 113945. doi:10.1016/j.rse.2023.113945
Fayad, I., Ienco, D., Baghdadi, N., Gaetano, R., Alvares, C. A., Stape, J. L., et al. (2021). A CNN-based approach for the estimation of canopy heights and wood volume from GEDI waveforms. Remote Sens. Environ. 265 (November), 112652. doi:10.1016/j.rse.2021.112652
Feldpausch, T. R., Lloyd, J., Lewis, S. L., Brienen, R. J. W., Gloor, M., Monteagudo Mendoza, A., et al. (2012). Tree height integrated into pantropical forest biomass estimates. Biogeosciences 9 (8), 3381–3403. doi:10.5194/bg-9-3381-2012
Ferry, B., Morneau, F., Bontemps, J.-D., Blanc, L., and Vincent, F. (2010). Higher treefall rates on slopes and waterlogged soils result in lower stand biomass and productivity in a tropical rain forest. J. Ecol. 98 (1), 106–116. doi:10.1111/j.1365-2745.2009.01604.x
García, M., Saatchi, S., Ustin, S., and Balzter, H. (2018). Modelling forest canopy height by integrating airborne LiDAR samples with satellite radar and multispectral imagery. Int. J. Appl. Earth Observation Geoinformation 66 (April), 159–173. doi:10.1016/j.jag.2017.11.017
Gazzea, M., Solheim, A., and Arghandeh, R. (2023). High-resolution mapping of forest structure from integrated SAR and optical images using an enhanced U-net method. Sci. Remote Sens. 8 (December), 100093. doi:10.1016/j.srs.2023.100093
Ge, S., Gu, H., Su, W., Praks, J., and Antropov, O. (2022). Improved semisupervised UNet deep learning model for forest height mapping with satellite SAR and optical data. IEEE J. Sel. Top. Appl. Earth Observations Remote Sens. 15, 5776–5787. doi:10.1109/JSTARS.2022.3188201
Geman, S., and McClure, D. E. (1985). “Bayesian image analysis: an application to single photon emission tomography,” in Proceedings of the American statistical association, 12–18.
Gond, V., Vincent, F., Molino, J.-F., Brunaux, O., Ingrassia, F., Joubert, P., et al. (2011). Broad-scale spatial pattern of forest landscape types in the Guiana shield. Int. J. Appl. Earth Observation Geoinformation 13 (3), 357–367. doi:10.1016/j.jag.2011.01.004
Grabska, E., Hostert, P., Pflugmacher, D., and Ostapowicz, K. (2019). Forest stand species mapping using the sentinel-2 time series. Remote Sens. 11 (10), 1197. doi:10.3390/rs11101197
Guitet, S., Cornu, J.-F., Brunaux, O., Betbeder, J., Carozza, J.-M., and Richard-Hansen, C. (2013). Landform and landscape mapping, French Guiana (South America). J. Maps 9 (3), 325–335. doi:10.1080/17445647.2013.785371
Guitet, S., Pélissier, R., Brunaux, O., Jaouen, G., and Sabatier, D. (2015). Geomorphological landscape features explain floristic patterns in French Guiana rainforest. Biodivers. Conservation 24 (5), 1215–1237. doi:10.1007/s10531-014-0854-8
Hancock, S., Armston, J., Hofton, M., Sun, X., Tang, H., Duncanson, L. I., et al. (2019). The GEDI simulator: a large-footprint waveform lidar simulator for calibration and validation of spaceborne missions. Earth Space Sci. 6 (2), 294–310. doi:10.1029/2018EA000506
Hansen, M. C., Potapov, P. V., Moore, R., Hancher, M., Turubanova, S. A., Tyukavina, A., et al. (2013). High-resolution global maps of 21st-century forest cover change. Science 342 (6160), 850–853. doi:10.1126/science.1244693
Healey, S. P., Yang, Z., Gorelick, N., and Simon, I. (2020). Highly local model calibration with a new GEDI LiDAR asset on Google Earth engine reduces Landsat forest height signal saturation. Remote Sens. 12 (17), 2840. doi:10.3390/rs12172840
Hilbert, C., and Schmullius, C. (2012). Influence of surface topography on ICESat/GLAS forest height estimation and waveform shape. Remote Sens. 4 (8), 2210–2235. doi:10.3390/rs4082210
Ho Tong Ming, D., Dinh, T.Le T., Rocca, F., Tebaldini, S., Villard, L., Réjou-Méchain, M., et al. (2016). SAR tomography for the retrieval of forest biomass and height: cross-validation at two tropical forest sites in French Guiana. Remote Sens. Environ. 175 (March), 138–147. doi:10.1016/j.rse.2015.12.037
Hong, D., Gao, L., Yokoya, N., Yao, J., Chanussot, J., Du, Q., et al. (2021). More diverse means better: multimodal deep learning meets remote-sensing imagery classification. IEEE Trans. Geoscience Remote Sens. 59 (5), 4340–4354. doi:10.1109/TGRS.2020.3016820
Ienco, D., Gaetano, R., Dupaquier, C., and Maurel, P. (2017). Land cover classification via multitemporal spatial data by deep recurrent neural networks. IEEE Geoscience Remote Sens. Lett. 14 (10), 1685–1689. doi:10.1109/LGRS.2017.2728698
Jin, S., Su, Y., Gao, S., Hu, T., Liu, J., and Guo, Q. (2018). The transferability of random forest in canopy height estimation from multi-source remote sensing data. Remote Sens. 10 (8), 1183. doi:10.3390/rs10081183
Joetzjer, E., Pillet, M., Ciais, P., Barbier, N., Chave, J., Schlund, M., et al. (2017). Assimilating satellite-based canopy height within an ecosystem model to estimate aboveground forest biomass. Geophys. Res. Lett. 44 (13), 6823–6832. doi:10.1002/2017GL074150
Jucker, T., Bongalov, B., Burslem, D. F. R. P., Nilus, R., Dalponte, M., Lewis, S. L., et al. (2018). “Topography shapes the structure, composition and function of tropical forest landscapes,”. Editor M. Uriarte, 21, 989–1000. doi:10.1111/ele.12964Ecol. Lett.7
Karasiak, N., Sheeren, D., Fauvel, M., Willm, J., Dejoux, J.-F., and Monteil, C. (2017). “Mapping tree species of forests in southwest France using sentinel-2 image time series,” in 2017 9th international workshop on the analysis of multitemporal remote sensing images (MultiTemp) (Brugge, Belgium: IEEE), 1–4. doi:10.1109/Multi-Temp.2017.8035215
Khati, U., Singh, G., and Kumar, S. (2018). Potential of space-borne PolInSAR for forest canopy height estimation over India—a case study using fully polarimetric L -C -and X -band SAR data. IEEE J. Sel. Top. Appl. Earth Observations Remote Sens. 11 (7), 2406–2416. doi:10.1109/JSTARS.2018.2835388
Kutchartt, E., Pedron, M., and Pirotti, F. (2022). Assessment of canopy and ground height accuracy from gedi lidar over steep mountain areas. ISPRS Ann. Photogrammetry, Remote Sens. Spatial Inf. Sci. V-3–2022, 431–438. doi:10.5194/isprs-annals-V-3-2022-431-2022
Lahssini, K., Baghdadi, N., Le Maire, G., Dupuy, S., and Fayad, I. (2024). Use of GEDI signal and environmental parameters to improve canopy height estimation over tropical forest ecosystems in Mayotte island. Can. J. Remote Sens. 50 (1), 2351004. doi:10.1080/07038992.2024.2351004
Lahssini, K., Baghdadi, N., Le Maire, G., and Fayad, I. (2022). Influence of GEDI acquisition and processing parameters on canopy height estimates over tropical forests. Remote Sens. 14 (24), 6264. doi:10.3390/rs14246264
Lahssini, K., Teste, F., Dayal, K. R., Durrieu, S., Ienco, D., and Monnet, J.-M. (2022). Combining LiDAR metrics and sentinel-2 imagery to estimate basal area and wood volume in complex forest environment via neural networks. IEEE J. Sel. Top. Appl. Earth Observations Remote Sens. 15, 4337–4348. doi:10.1109/JSTARS.2022.3175609
Lang, N., Jetz, W., Schindler, K., and Jan, D. W. (2023). A high-resolution canopy height model of the Earth. Nat. Ecol. and Evol. 7 (11), 1778–1789. doi:10.1038/s41559-023-02206-6
Lang, N., Kalischek, N., Armston, J., Schindler, K., Dubayah, R., and Wegner, J. D. (2022). Global canopy height regression and uncertainty estimation from GEDI LIDAR waveforms with deep ensembles. Remote Sens. Environ. 268 (January), 112760. doi:10.1016/j.rse.2021.112760
Lang, N., Schindler, K., and Jan, D. W. (2019). Country-wide high-resolution vegetation height mapping with sentinel-2. Remote Sens. Environ. 233 (November), 111347. doi:10.1016/j.rse.2019.111347
LeCun, Y., Bengio, Y., and Hinton, G. (2015). Deep learning. Nature 521 (7553), 436–444. doi:10.1038/nature14539
Lefsky, M. A., Harding, D. J., Keller, M., Cohen, W. B., Carabajal, C. C., Espirito-Santo, F. D. B., et al. (2005). Estimates of forest canopy height and aboveground biomass using ICESat. Geophys. Res. Lett. 32 (22), 2005GL023971. doi:10.1029/2005GL023971
Lemaire, G., Francois, C., Soudani, K., Berveiller, D., Pontailler, J., Breda, N., et al. (2008). Calibration and validation of hyperspectral indices for the estimation of broadleaved forest leaf chlorophyll content, leaf mass per area, leaf area index and leaf canopy biomass. Remote Sens. Environ. 112 (10), 3846–3864. doi:10.1016/j.rse.2008.06.005
Li, H., Li, X., Kato, T., Hayashi, M., Fu, J., and Hiroshima, T. (2024). Accuracy assessment of GEDI terrain elevation, canopy height, and aboveground biomass density estimates in Japanese artificial forests. Sci. Remote Sens. 10 (December), 100144. doi:10.1016/j.srs.2024.100144
Lima, A. J. N., Suwa, R., Gabriel Henrique Pires, De M. R., Kajimoto, T., Dos Santos, J., Pereira Da Silva, R., et al. (2012). Allometric models for estimating above- and below-ground biomass in amazonian forests at são gabriel da cachoeira in the upper rio negro, Brazil. For. Ecol. Manag. 277 (August), 163–172. doi:10.1016/j.foreco.2012.04.028
Liu, A., Cheng, X., and Chen, Z. (2021). Performance evaluation of GEDI and ICESat-2 laser altimeter data for terrain and canopy height retrievals. Remote Sens. Environ. 264 (October), 112571. doi:10.1016/j.rse.2021.112571
Liu, X., Neigh, C. S. R., Pardini, M., and Forkel, M. (2024). Estimating forest height and above-ground biomass in tropical forests using P-band TomoSAR and GEDI observations. Int. J. Remote Sens. 45 (9), 3129–3148. doi:10.1080/01431161.2024.2343134
Long, J., Shelhamer, E., and Darrell, T. (2014). Fully convolutional networks for semantic segmentation. arXiv. doi:10.48550/ARXIV.1411.4038
Main-Knorn, M., Pflug, B., Louis, J., Vincent, D., Müller-Wilm, U., and Gascon, F. (2017). “Sen2Cor for sentinel-2,”. Image and signal processing for remote sensing XXIII. Editors L. Bruzzone, F. Bovolo, and J. A. Benediktsson (Warsaw, Poland: SPIE), 3. doi:10.1117/12.2278218
McDowell, N., Barnard, H., Bond, B., Hinckley, T., Hubbard, R., Ishii, H., et al. (2002). The relationship between tree height and leaf area: sapwood area ratio. Oecologia 132 (1), 12–20. doi:10.1007/s00442-002-0904-x
Mermoz, S., Le Toan, T., Villard, L., Réjou-Méchain, M., and Seifert-Granzin, J. (2014). Biomass assessment in the Cameroon savanna using ALOS PALSAR data. Remote Sens. Environ. 155 (December), 109–119. doi:10.1016/j.rse.2014.01.029
Mermoz, S., Réjou-Méchain, M., Villard, L., Le Toan, T., Rossi, V., and Gourlet-Fleury, S. (2015). Decrease of L-band SAR backscatter with biomass of dense forests. Remote Sens. Environ. 159 (March), 307–317. doi:10.1016/j.rse.2014.12.019
Morin, D., Planells, M., Baghdadi, N., Bouvet, A., Fayad, I., Le Toan, T., et al. (2022). Improving heterogeneous forest height maps by integrating GEDI-based forest height information in a multi-sensor mapping process. Remote Sens. 14 (9), 2079. doi:10.3390/rs14092079
Morin, D., Planells, M., Guyon, D., Villard, L., Mermoz, S., Bouvet, A., et al. (2019). Estimation and mapping of forest structure parameters from open access satellite images: development of a generic method with a study case on coniferous plantation. Remote Sens. 11 (11), 1275. doi:10.3390/rs11111275
Morin, D., Planells, M., Mermoz, S., and Mouret, F. (2023). Estimation of forest height and biomass from open-access multi-sensor satellite imagery and GEDI lidar data: high-resolution maps of metropolitan France. arXiv. doi:10.48550/ARXIV.2310.14662
Muscarella, R., Kolyaie, S., Morton, D. C., Zimmerman, J. K., and Uriarte, M. (2020). “Effects of topography on tropical forest structure depend on climate context,”. Journal of ecology. Editor T. Jucker, 108, 145–159. doi:10.1111/1365-2745.132611
Ngo, Y.-N., Minh, D.Ho T., Baghdadi, N., and Fayad, I. (2023). Tropical forest top height by GEDI: from sparse coverage to continuous data. Remote Sens. 15 (4), 975. doi:10.3390/rs15040975
Pan, Y., Birdsey, R. A., Fang, J., Houghton, R., Kauppi, P. E., Kurz, W. A., et al. (2011). A large and persistent carbon sink in the world’s forests. Science 333 (6045), 988–993. doi:10.1126/science.1201609
Pimple, U., Leadprathom, K., Simonetti, D., Sitthi, A., Peters, R., Pungkul, S., et al. (2022). Assessing mangrove species diversity, zonation and functional indicators in response to natural, regenerated, and rehabilitated succession. J. Environ. Manag. 318 (September), 115507. doi:10.1016/j.jenvman.2022.115507
Potapov, P., Li, X., Hernandez-Serna, A., Tyukavina, A., Hansen, M. C., Kommareddy, A., et al. (2021). Mapping global forest canopy height through integration of GEDI and Landsat data. Remote Sens. Environ. 253 (February), 112165. doi:10.1016/j.rse.2020.112165
Pourrahmati, M. R., Baghdadi, N. N., Darvishsefat, A. A., Namiranian, M., Fayad, I., Bailly, J.-S., et al. (2015). Capability of GLAS/ICESat data to estimate forest canopy height and volume in mountainous forests of Iran. IEEE J. Sel. Top. Appl. Earth Observations Remote Sens. 8 (11), 5246–5261. doi:10.1109/JSTARS.2015.2478478
Qi, W., Armston, J., Choi, C., Stovall, A., Saarela, S., Pardini, M., et al. (2023). “Mapping large-scale pantropical forest canopy height by integrating GEDI lidar and TanDEM-X InSAR data. doi:10.21203/rs.3.rs-3306982/v1
Quegan, S., Le Toan, T., Chave, J., Dall, J., Exbrayat, J.-F., Minh, D.Ho T., et al. (2019). The European Space agency BIOMASS mission: measuring forest above-ground biomass from Space. Remote Sens. Environ. 227 (June), 44–60. doi:10.1016/j.rse.2019.03.032
Rahman, M. M., and Sumantyo, J. T. S. (2010). Mapping tropical forest cover and deforestation using synthetic aperture radar (SAR) images. Appl. Geomatics 2 (3), 113–121. doi:10.1007/s12518-010-0026-9
Rennó, C. D., Donato Nobre, A., Adriana Cuartas, L., Soares, J. V., Hodnett, M. G., Tomasella, J., et al. (2008). HAND, a new terrain descriptor using SRTM-DEM: mapping terra-firme rainforest environments in Amazonia. Remote Sens. Environ. 112 (9), 3469–3481. doi:10.1016/j.rse.2008.03.018
Robbins, H., and Monro, S. (1951). A stochastic approximation method. Ann. Math. Statistics 22 (3), 400–407. doi:10.1214/aoms/1177729586
Ronneberger, O., Fischer, P., and Brox, T. (2015). U-net: convolutional networks for biomedical image segmentation. arXiv, 234–241. doi:10.1007/978-3-319-24574-4_28
Roy, D. P., Kashongwe, H. B., and Armston, J. (2021). The impact of geolocation uncertainty on GEDI tropical forest canopy height estimation and change monitoring. Sci. Remote Sens. 4 (December), 100024. doi:10.1016/j.srs.2021.100024
Saatchi, S. S., Harris, N. L., Brown, S., Lefsky, M., Mitchard, E. T. A., Salas, W., et al. (2011). Benchmark map of forest carbon stocks in tropical regions across three continents. Proc. Natl. Acad. Sci. 108 (24), 9899–9904. doi:10.1073/pnas.1019576108
Schietti, J., Emilio, T., Rennó, C. D., Drucker, D. P., Costa, F. R. C., Nogueira, A., et al. (2014). Vertical distance from drainage drives floristic composition changes in an amazonian rainforest. Plant Ecol. and Divers. 7 (1–2), 241–253. doi:10.1080/17550874.2013.783642
Schleich, A., Durrieu, S., Soma, M., and Vega, C. (2023). Improving GEDI footprint geolocation using a high-resolution digital elevation model. IEEE J. Sel. Top. Appl. Earth Observations Remote Sens. 16, 7718–7732. doi:10.1109/JSTARS.2023.3298991
Schlund, M., Wenzel, A., Camarretta, N., Stiegler, C., and Erasmi, S. (2023). Vegetation canopy height estimation in dynamic tropical landscapes with TanDEM-X supported by GEDI data. Methods Ecol. Evol. 14 (7), 1639–1656. doi:10.1111/2041-210X.13933
Schneider, F. D., António, F., Hancock, S., Duncanson, L. I., Dubayah, R. O., Pavlick, R. P., et al. (2020). Towards mapping the diversity of canopy structure from Space with GEDI. Environ. Res. Lett. 15 (11), 115006. doi:10.1088/1748-9326/ab9e99
Schwartz, M., Ciais, P., Ottlé, C., De Truchis, A., Vega, C., Fayad, I., et al. (2024). High-resolution canopy height map in the Landes forest (France) based on GEDI, sentinel-1, and sentinel-2 data with a deep learning approach. Int. J. Appl. Earth Observation Geoinformation 128 (April), 103711. doi:10.1016/j.jag.2024.103711
Shannon, E. S., Finley, A. O., Hayes, D. J., Noralez, S. N., Weiskittel, A. R., Cook, B. D., et al. (2024). Quantifying and correcting geolocation error in spaceborne LiDAR forest canopy observations using high spatial accuracy data: a bayesian model approach. Environmetrics 35 (4), e2840. doi:10.1002/env.2840
Simard, M., Pinto, N., Fisher, J. B., and Baccini, A. (2011). Mapping forest canopy height globally with spaceborne lidar. J. Geophys. Res. 116 (G4), G04021. doi:10.1029/2011JG001708
Simonetti, D., Pimple, U., Langner, A., and Marelli, A. (2021). Pan-tropical sentinel-2 cloud-free annual composite datasets. Data Brief 39 (December), 107488. doi:10.1016/j.dib.2021.107488
Smith, L. N. (2015). Cyclical learning rates for training neural networks. arXiv. doi:10.48550/ARXIV.1506.01186
Sothe, C., Gonsamo, A., Lourenço, R. B., Kurz, W. A., and Snider, J. (2022). Spatially continuous mapping of forest canopy height in Canada by combining GEDI and ICESat-2 with PALSAR and Sentinel. Remote Sens. 14 (20), 5158. doi:10.3390/rs14205158
Stape, J. L., and Alvares, C. A. (2023). Physiographic position drives Eucalyptus productivity in mato grosso do sul, Brazil. Série Técnica IPEF 26 (48), 289–293. doi:10.18671/sertec.v26n48.057
Sun, D., Roth, S., and Black, M. J. (2010). “Secrets of optical flow estimation and their principles,” in 2010 IEEE computer society conference on computer vision and pattern recognition (San Francisco, CA, USA: IEEE), 2432–2439. doi:10.1109/CVPR.2010.5539939
Sun, M., Cui, L., Park, J., García, M., Zhou, Y., Silva, C. A., et al. (2022). Evaluation of NASA’s GEDI lidar observations for estimating biomass in temperate and tropical forests. Forests 13 (10), 1686. doi:10.3390/f13101686
Tang, H., Stoker, J., Luthcke, S., Armston, J., Lee, K., Bryan, B., et al. (2023). Evaluating and mitigating the impact of systematic geolocation error on canopy height measurement performance of GEDI. Remote Sens. Environ. 291 (June), 113571. doi:10.1016/j.rse.2023.113571
Tolan, J., Yang, H.-I., Nosarzewski, B., Couairon, G., Vo, H. V., Brandt, J., et al. (2024). Very high resolution canopy height maps from RGB imagery using self-supervised vision transformer and convolutional decoder trained on aerial lidar. Remote Sens. Environ. 300 (January), 113888. doi:10.1016/j.rse.2023.113888
Tyukavina, A., Baccini, A., Hansen, M. C., Potapov, P. V., Stehman, S. V., Houghton, R. A., et al. (2015). Aboveground carbon loss in natural and managed tropical forests from 2000 to 2012. Environ. Res. Lett. 10 (7), 074002. doi:10.1088/1748-9326/10/7/074002
Ulaby, F. T., Sarabandi, K., McDONALD, K., Whitt, M., and Craig Dobson, M. (1990). Michigan microwave canopy scattering model. Int. J. Remote Sens. 11 (7), 1223–1253. doi:10.1080/01431169008955090
Vancutsem, C., Achard, F., Pekel, J.-F., Vieilledent, G., Carboni, S., Simonetti, D., et al. (2021). Long-term (1990–2019) monitoring of forest cover changes in the humid tropics. Sci. Adv. 7 (10), eabe1603. doi:10.1126/sciadv.abe1603
Verhegghen, A., Eva, H., Ceccherini, G., Achard, F., Gond, V., Gourlet-Fleury, S., et al. (2016). The potential of Sentinel satellites for burnt area mapping and monitoring in the Congo basin forests. Remote Sens. 8 (12), 986. doi:10.3390/rs8120986
Wagner, F. H., Roberts, S., Ritz, A. L., Carter, G., Dalagnol, R., Favrichon, S., et al. (2024). Sub-meter tree height mapping of California using aerial images and LiDAR-informed U-net model. Remote Sens. Environ. 305 (May), 114099. doi:10.1016/j.rse.2024.114099
Wang, C., Elmore, A. J., Numata, I., Cochrane, M. A., Lei, S., Hakkenberg, C. R., et al. (2022). A framework for improving wall-to-wall canopy height mapping by integrating GEDI LiDAR. Remote Sens. 14 (15), 3618. doi:10.3390/rs14153618
Watanabe, M., Koyama, C. N., Hayashi, M., Nagatani, I., and Shimada, M. (2018). Early-stage deforestation detection in the tropics with L -band SAR. IEEE J. Sel. Top. Appl. Earth Observations Remote Sens. 11 (6), 2127–2133. doi:10.1109/JSTARS.2018.2810857
Wijaya, A., Liesenberg, V., Susanti, A., Karyanto, O., and Verchot, L. V. (2015). Estimation of biomass carbon stocks over peat swamp forests using multi-temporal and multi-polratizations SAR data. Int. Archives Photogrammetry, Remote Sens. Spatial Inf. Sci. XL-7/W3 (April), 551–556. doi:10.5194/isprsarchives-XL-7-W3-551-2015
Yang, Y., Saatchi, S., Xu, L., Yu, Y., Lefsky, M., White, L., et al. (2016). Abiotic controls on macroscale variations of humid tropical forest height. Remote Sens. 8 (6), 494. doi:10.3390/rs8060494
Yuan, X., Shi, J., and Gu, L. (2021). A review of deep learning methods for semantic segmentation of remote sensing imagery. Expert Syst. Appl. 169 (May), 114417. doi:10.1016/j.eswa.2020.114417
Keywords: canopy height, data fusion, deep learning, French Guiana, GEDI, lidar, tropical forests
Citation: Lahssini K, Baghdadi N, le Maire G, Fayad I and Villard L (2024) Canopy height mapping in French Guiana using multi-source satellite data and environmental information in a U-Net architecture. Front. Remote Sens. 5:1484900. doi: 10.3389/frsen.2024.1484900
Received: 22 August 2024; Accepted: 31 October 2024;
Published: 25 November 2024.
Edited by:
Celso H. L. Silva-Junior, Instituto de Pesquisa Ambiental da Amazonia (IPAM), BrazilReviewed by:
Patrick Jantz, Northern Arizona University, United StatesSugandha Arora, University of Münster, Germany
Copyright © 2024 Lahssini, Baghdadi, le Maire, Fayad and Villard. This is an open-access article distributed under the terms of the Creative Commons Attribution License (CC BY). The use, distribution or reproduction in other forums is permitted, provided the original author(s) and the copyright owner(s) are credited and that the original publication in this journal is cited, in accordance with accepted academic practice. No use, distribution or reproduction is permitted which does not comply with these terms.
*Correspondence: Kamel Lahssini, a2FtZWwubGFoc3NpbmlAaW5yYWUuZnI=