- 1Governor’s School of Engineering, Hampton, VA, United States
- 2NASA Langley Research Center, Hampton, VA, United States
With a large footprint size, multiple scattering measurements of clouds from spaceborne lidar provide useful information about cloud physical properties, such as cloud optical depths and cloud droplet size, both during daytime and nighttime. A neural network algorithm, with a subset of cloud backscatter profiles of dual-polarization and dual-wavelength Cloud-Aerosol Lidar and Infrared Pathfinder Satellite Observation (CALIPSO) lidar measurements during daytime as input variables and cloud physical properties derived from collocated Moderate Resolution Imaging Spectroradiometer (MODIS) multi-spectral measurements as output, is developed and evaluated with an independent subset of the collocated CALIPSO and MODIS measurements. The study suggests that with a receiver footprint size of 110 m, CALIPSO lidar measurements are sensitive to liquid-phase cloud optical depth variations from 0 to 25. A larger footprint size, thus more multiple scattering, is required for lidar to have sensitivities to all liquid-phase clouds. The technique can be applied to all 17 years of CALIPSO daytime and nighttime measurements and, thus, provides useful information about global distributions of cloud physical properties both during day and night.
1 Introduction
Satellite remote sensing of clouds is important for understanding Earth’s hydrological cycle and quantifying the radiative energy balance. Moderate Resolution Imaging Spectroradiometer (MODIS) and other passive sensors can provide cloud information in daytime, although nighttime information has to be from active sensors, e.g., Cloud-Aerosol Lidar and Infrared Pathfinder Satellite Observation (CALIPSO).
Lidar measurements provide unique information about clouds. The cloud thermodynamic phase determined by space-based lidars (Hu et al., 2009) is considered the gold standard (Wang et al., 2020; Wang et al., 2024). In recent studies, water cloud microphysical properties were derived from CALIPSO’s space-based lidar measurements (Hu et al., 2021).
Cloud properties, such as droplet size and optical depth, can be acquired from multi-spectral measurements (e.g., MODIS) during daytime (Platnick et al., 2003). These cloud properties can be used for training collocated lidar measurements. The knowledge from the training can be applied to lidar measurements at night to produce unique cloud properties at night.
In this study, we use MODIS daytime measurements of cloud optical depths and effective particle sizes of water clouds to train collocated CALIPSO measurements with machine learning. We then apply the algorithms on all daytime and nighttime CALIPSO measurements. The objectives of this study are to
A. evaluate the accuracy of the CALIPSO water cloud data product,
B. assess the sensitivity of CALIPSO measurements to water cloud optical depth,
C. estimate global statistics of nighttime water cloud physical properties.
2 Spaceborne lidar measurement of the water cloud optical depth and effective droplet size: information content and machine learning algorithm
CALIPSO lidar measurements are highly sensitive to cloud properties. For example, a huge portion of the cloud layer-integrated backscatter comes from multiple scattering of laser light bouncing among cloud particles. Thus, they are sensitive to cloud physical properties such as optical depths (Figure 1) and particulate sizes. The layer-integrated backscatter is inversely proportional to the so-called cloud lidar ratio, which is the ratio of extinction to the backscatter cross section. The cloud lidar ratio is a function of the effective droplet size (Mace et al., 2020; Hu et al., 2021) and the so-called “multiple scattering factor,” which is a simple function of CALIPSO depolarization ratio measurements of the cloud backscatter (Hu, 2007) (Figure 2). The cloud backscatter ratio of the infrared (1,064 nm) and green (532 nm) wavelengths provide additional information about droplet sizes (Figure 2). The path length distribution of laser-light multiple scattering inside clouds is also sensitive to the physical thickness and extinction coefficient of the cloud layer (Hu et al., 2022; Hu et al., 2023).
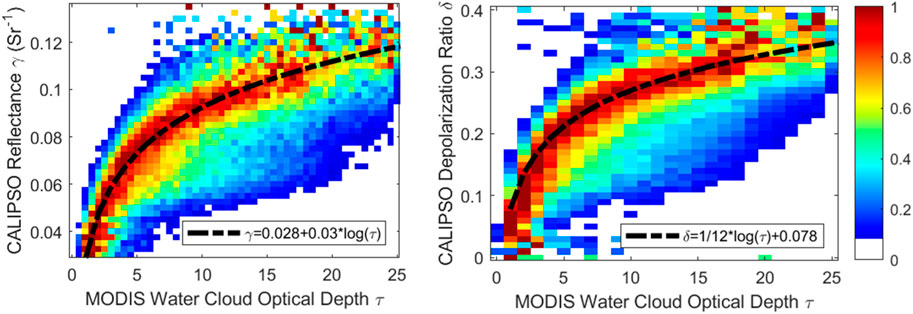
Figure 1. Left panel: CALIPSO water cloud layer-integrated backscatter (also called reflectance), γ, increases linearly with the logarithm of the MODIS optical depth, γ. Right panel: depolarization ratio, δ, of CALIPSO water cloud layer-integrated backscatter also increases linearly with the logarithm of the MODIS optical depth.
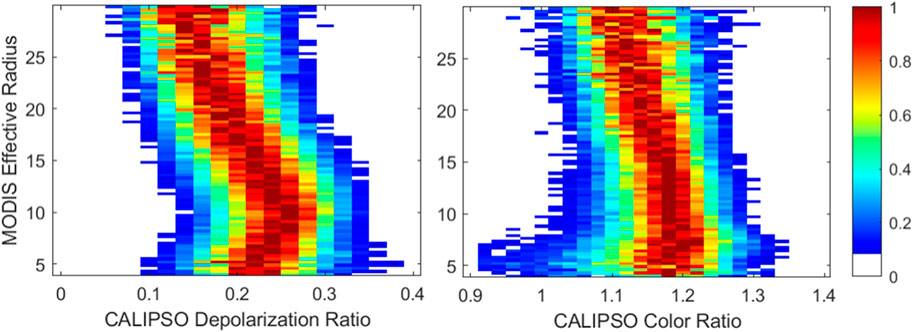
Figure 2. Sensitivity of the water cloud effective radius to the depolarization ratio (left panel) and color ratio (right panel) of CALIPSO layer-integrated lidar backscatter measurements of the water clouds.
Although cloud properties are highly sensitive to CALIPSO lidar measurements, deriving cloud properties from CALIPSO data requires good estimates of laser-light attenuation by molecules and background aerosols above clouds, which are sensitive to the CALIPSO lidar measurements of aerosol and molecular backscatter profiles.
The MODIS level-2 water cloud optical depth derived from MODIS band 6 (1.6 μm) and band 20 (3.7 μm) and the effective radius derived from MODIS band 20 are used for training the collocated CALIPSO daytime measurements. The output parameters of the machine learning algorithm, based on MATLAB neural network software fitnet with 2 hidden layers (10 neurons for the first hidden layer and 6 for the second hidden layer), are the water cloud optical depth and effective radius (Figure 3).
The input parameters of the neural network fitnet (Figure 3) are the following CALIPSO measurements.
• Three channel-integrated backscatter of the atmosphere above clouds (532-nm parallel component, 532-nm perpendicular component, and 1,064 total backscatter),
• Layer-integrated cloud backscatter of the three channels,
• Layer mean cloud backscatter of the three channels,
• Backscatter-weighted mean path lengths (<L>) of the three channels, where <L>=
• Peak backscatter intensity of the three channels,
• Cloud top height and temperatures at the centroid of the CALIPSO backscatter.
These CALIPSO properties are obtained from CALIPSO version 4 level-1 and level-2 data products.
3 Evaluating cloud properties derived from CALIPSO and machine learning
After the neural network algorithms that link CALIPSO measurements to water cloud optical depths and effective radii are trained with a limited amount (2 months: January and July in 2008) of collocated daytime CALIPSO and MODIS measurements, the algorithms are applied to daytime CALIPSO measurements from the other months of a whole year (2009) to derive water cloud optical depths and effective radii. Figure 4 shows that the biases between the CALIPSO and MODIS water cloud effective radii are nearly zero, with an averaged difference of CALIPSO/MODIS effective radii of approximately 3 μm. Similarly, there is very little bias in the CALIPSO cloud optical depths for cloud optical depths below 20 and the averaged difference of CALIPSO/MODIS optical depths is less than 2. The result suggests that the CALIPSO water cloud property can be derived reliably using the neural network algorithms.
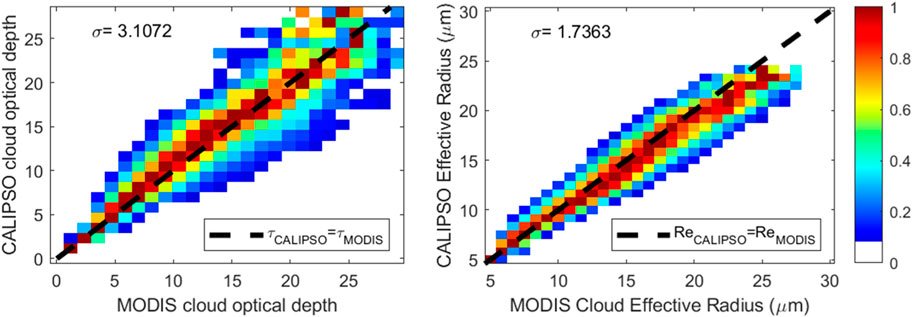
Figure 4. Comparisons of daytime water cloud optical depths (left panel) and effective radii (right panel) derived from the neural network algorithms to collocated MODIS measurements.
4 Global statistics of the nighttime water cloud optical depth and effective radius and comparison with daytime measurements
Only daytime measurements of MODIS cloud optical depths and effective radii are available for the training of the neural networks. We applied the neural network algorithm to both daytime and nighttime CALIPSO measurements, enabling us to derive cloud optical depths and effective radii for both day and night (Figures 5, 6).
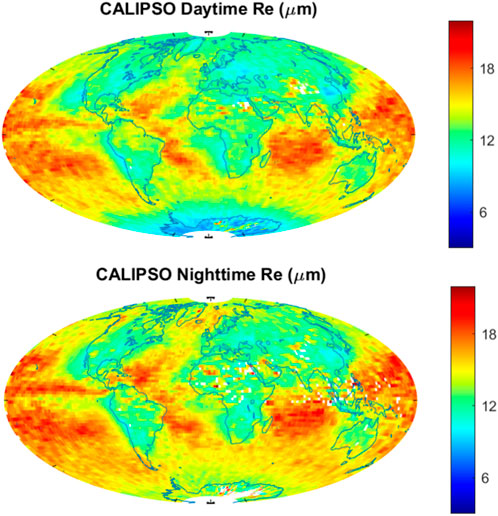
Figure 5. Global statistics of the annual mean water cloud effective droplet radii during daytime (upper panel) and nighttime (lower panel).
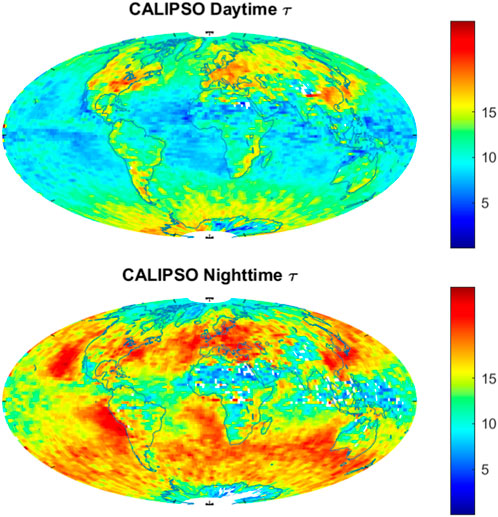
Figure 6. Global statistics of the annual mean water cloud optical depths during daytime (upper panel) and nighttime (lower panel).
It is important to measure cloud properties at night because these properties are important constraints of weather and climate models to simulate the water and energy cycle (Liu et al., 2008). Passive remote sensing, such as MODIS, mostly relies on spectral measurements of sunlight reflected by water clouds to derive their physical properties. Thus, it is difficult to obtain water cloud physical properties, such as particle size and cloud optical depth from passive remote sensing (e.g., MODIS) measurements at nighttime (Pérez et al., 2009).
CALIPSO lidar measurements can provide the same cloud information during daytime and nighttime. Thus, it is possible to acquire nighttime water cloud properties using CALIPSO measurements with the neural network algorithms developed with daytime collocated CALIPSO and MODIS measurements.
Although the effective droplet radii of water clouds at night derived from CALIPSO lidar measurements are slightly larger than those at daytime (Figure 5), nighttime water cloud optical depths derived from CALIPSO lidar measurements are significantly larger than daytime water cloud optical depths (Figure 6). As the cloud liquid water path is proportional to the product of water cloud optical depth and droplet effective radius (e.g., Li and Min, 2013), the day and night contrast in CALIPSO cloud optical depths (Figure 6) suggests that the nighttime water cloud liquid paths are significantly larger than daytime cloud liquid water paths, which is consistent with the diurnal cloud liquid water path measurements from collocated AMSR-E measurements (O’Dell et al., 2008). The nighttime and daytime differences in water cloud effective radii and optical depths are likely due to the enhanced condensation at night due to difference in the radiative cooling rate near the cloud top between day and night.
5 Summary
A neural network lidar measurement algorithm for water cloud property estimation is introduced in this study. Water cloud optical depths and effective radii from MODIS daytime measurements are used for training collocated CALIPSO lidar measurements. Applying the algorithm to daytime CALIPSO measurements different from the training dataset, the cloud properties compare very well with the cloud properties from the collocated MODIS measurements. The biases of droplet effective radii are near zero, with a standard deviation of the CALIPSO/MODIS effective radius difference of approximately 3 μm. The biases of CALIPSO water cloud optical depths are near zero for a cloud optical depth of less than 20, with a standard deviation of the CALIPSO/MODIS optical depth difference of 2.
This algorithm is applied to both daytime and nighttime CALIPSO measurements. The nighttime global statistics of the water cloud droplet effective radius are similar to that of daytime, with a slightly larger droplet size at night. The nighttime water cloud optical depths are significantly larger than daytime cloud optical depths.
Data availability statement
The original contributions presented in the study are included in the article/Supplementary Material; further inquiries can be directed to the corresponding author.
Author contributions
XL: data curation, writing–review and editing, and supervision. KH: conceptualization, writing–original draft, investigation, methodology, and software.
Funding
The author(s) declare that no financial support was received for the research, authorship, and/or publication of this article.
Acknowledgments
The authors acknowledge the NASA CALIPSO program for supporting this research. The authors thank all the colleagues who directly or indirectly contributed to the making of Neural Network Toolbox software.
Conflict of interest
The authors declare that the research was conducted in the absence of any commercial or financial relationships that could be construed as a potential conflict of interest.
Publisher’s note
All claims expressed in this article are solely those of the authors and do not necessarily represent those of their affiliated organizations, or those of the publisher, the editors, and the reviewers. Any product that may be evaluated in this article, or claim that may be made by its manufacturer, is not guaranteed or endorsed by the publisher.
References
Hu, Y. (2007). Depolarization ratio–effective lidar ratio relation: theoretical basis for space lidar cloud phase discrimination. Geophys. Res. Lett. 34, L11812. doi:10.1029/2007GL029584
Hu, Y., Lu, X., Zeng, X., Stamnes, S., Neumann, T. A., Kurtz, N. T., et al. (2022). Deriving snow depth from ICESat-2 lidar multiple scattering measurements. Front. Remote Sens. 3, accepted. doi:10.3389/frsen.2022.855159
Hu, Y., Lu, X., Zeng, X., Gatebe, C., Fu, Q., Yang, P., et al. (2023). Linking lidar multiple scattering profiles to snow depth and snow density: an analytical radiative transfer analysis and the implications for remote sensing of snow. Front. Remote Sens. 4. doi:10.3389/frsen.2023.1202234
Hu, Y., Lu, X., Zhai, P. W., Hostetler, C. A., Hair, J. W., Cairns, B., et al. (2021). Liquid phase cloud microphysical property estimates from CALIPSO measurements. Front. Remote Sens. 2, 724615. doi:10.3389/frsen.2021.724615
Hu, Y., Winker, D., Vaughan, M., Lin, B., Omar, A., Trepte, C., et al. (2009). CALIPSO/CALIOP cloud phase discrimination algorithm. J. Atmos. Ocean. Tech. 26, 2293–2309. doi:10.1175/2009JTECHA1280.1
Li, S., and Min, Q. (2013). Retrievals of vertical profiles of stratus cloud properties from combined oxygen A-band and radar observations. J. Geophys. Res. Atmos. 118 (2), 769–778. doi:10.1029/2012jd018282
Liu, C., Zipser, E. J., Mace, G. G., and Benson, S. (2008). Implications of the differences between daytime and nighttime CloudSat observations over the tropics. J. Geophys. Res. Atmos. 113 (D8). doi:10.1029/2008jd009783
Mace, G. G., Benson, S., and Hu, Y. (2020). On the frequency of occurrence of the ice phase in su-percooled Southern Ocean low clouds derived from CALIPSO and CloudSat. Geophys. Re-search Lett. 47, e2020GL087554. doi:10.1029/2020GL087554
O’Dell, C. W., Wentz, F. J., and Bennartz, R. (2008). Cloud liquid water path from satellite-based passive microwave observations: a new climatology over the global oceans. J. Clim. 21 (8), 1721–1739. doi:10.1175/2007jcli1958.1
Pérez, J. C., Cerdeña, A., González, A., and Armas, M. (2009). Nighttime cloud properties retrieval using MODIS and artificial neural networks. Adv. Space Res. 43 (5), 852–858. doi:10.1016/j.asr.2008.06.013
Platnick, S., King, M. D., Ackerman, S. A., Menzel, W. P., Baum, B. A., Riédi, J. C., et al. (2003). The MODIS cloud products: algorithms and examples from Terra. IEEE Trans. geoscience Remote Sens. 41 (2), 459–473. doi:10.1109/tgrs.2002.808301
Wang, C., Platnick, S., Meyer, K., Zhang, Z., and Zhou, Y. (2020). A machine-learning-based cloud detection and thermodynamic-phase classification algorithm using passive spectral observations. Atmos. Meas. Tech. 13, 2257–2277. doi:10.5194/amt-13-2257-2020
Keywords: cloud optical depth, cloud effective droplet size, spaceborne lidar, CALIPSO, multiple scattering
Citation: Hu K and Lu X (2024) Spaceborne lidar measurement of global cloud properties through machine learning. Front. Remote Sens. 5:1477503. doi: 10.3389/frsen.2024.1477503
Received: 07 August 2024; Accepted: 16 September 2024;
Published: 02 October 2024.
Edited by:
Zhenzhu Wang, Aiofm, CAS, ChinaReviewed by:
Peng-Wang Zhai, University of Maryland, Baltimore County, United StatesWeizhen Hou, Harvard University, United States
Copyright © 2024 Hu and Lu. This is an open-access article distributed under the terms of the Creative Commons Attribution License (CC BY). The use, distribution or reproduction in other forums is permitted, provided the original author(s) and the copyright owner(s) are credited and that the original publication in this journal is cited, in accordance with accepted academic practice. No use, distribution or reproduction is permitted which does not comply with these terms.
*Correspondence: Xiaomei Lu, eGlhb21laS5sdUBuYXNhLmdvdg==