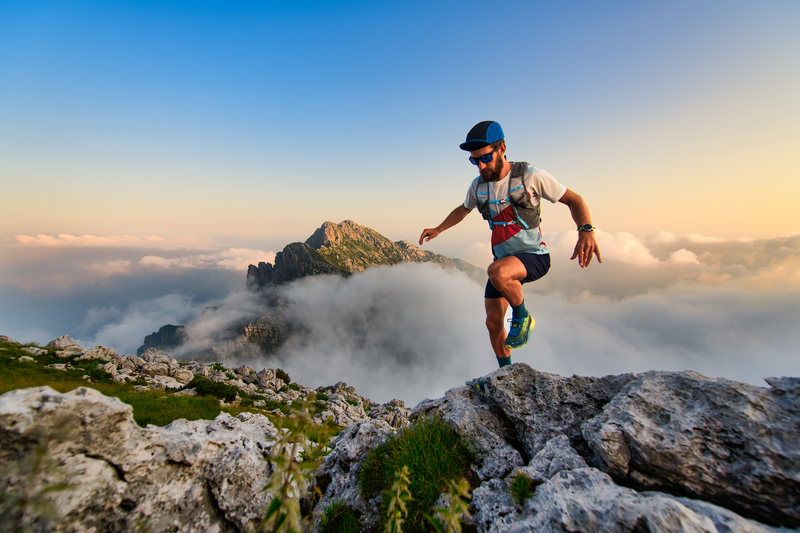
94% of researchers rate our articles as excellent or good
Learn more about the work of our research integrity team to safeguard the quality of each article we publish.
Find out more
ORIGINAL RESEARCH article
Front. Remote Sens. , 22 August 2024
Sec. Terrestrial Carbon Cycle
Volume 5 - 2024 | https://doi.org/10.3389/frsen.2024.1432577
Introduction: The knowledge about forest growth, influenced by factors such as tree species, tree age, and environmental conditions, is a key for future forest preservation. Height and age data can be combined to describe forest growth and used to infer known environmental effects.
Methods: In this study, we built 14 height growth curves for stands composed of monospecific or mixed species using ground measurements and satellite data. We built a random forest height model from tree species, age, area of disturbance, and 125 environmental parameters (climate, altitude, soil composition, geology, stand ownership, and proximity to road and urban areas). Using feature elimination and SHapley Additive exPlanations (SHAP) analysis, we identified six key features explaining the forest growth and investigated how they affect the height.
Results: The agreement between satellite and ground data justifies their simultaneous exploitation. Age and tree species are the main predictors of tree height (49% and 10%, respectively). The disturbed patch area, revealing the regeneration method, impacts post-disturbance growth at 19%. The soil pH, altitude, and climatic water budget in summer impact tree height differently depending on the age and tree species.
Discussion: Methods integrating satellite and field data show promise for analyzing future forest evolution.
Forests are critical to both the environment and human wellbeing and provide services ranging from carbon sequestration to biodiversity conservation and climate regulation (IPCC, 2019). Forest productivity is a major factor of forest resources and, thus, a concern for both forestry and climate sciences. In addition, preserving carbon stocks and maintaining a carbon sink in the forest sector are fundamental elements of the EU Forestry Strategy 2020 and the EU neutrality objectives (Korosuo et al., 2023). In the EU, most forests are managed (FOREST EUROPE, 2020), and management options include rotation ages, thinning regime, regeneration cuttings, the choice of tree species in regeneration and plantation, and forest growth rates (Pretzsch, 2009; Bontemps and Bouriaud, 2014). Natural disturbances can also lead to stand suppression in the case of severe events. The growth rate of forest stands after a management or natural disturbance is linked to the time since the disturbance or stand age, tree species, and site quality (Franklin et al., 2002; Swamy et al., 2003; D’Amato et al., 2011; Luyssaert et al., 2008; Niinemets, 2010; Chazdon et al., 2016; Poorter et al., 2016; Liang et al., 2016; Messier et al., 2019; Pugh et al., 2019; Seidl et al., 2017; Thom et al., 2017). Site quality refers to the intrinsic capacity of local conditions to facilitate the growth of vegetation, and it is influenced by factors such as climate, soil characteristics, topography, and hydrology. Stands located in areas with better site quality have higher growth rates and reach higher carbon stocks at maturity (Swamy et al., 2003; Pan et al., 2011). The importance of forest growth rates has been highlighted in the “Forest Principles” for sustainable forest management at the Rio Conference (UN Conference on Environment and Development, 1992) and confirmed in the criteria for forest management under the Montreal Process (Montreal Process Working Group, 1994) and at the Ministerial Conference on the Protection of Forests in Europe (MCPFE, 2002).
Despite being crucial, determining the growth rates of forests has consistently posed a significant challenge in the field of forestry due to the long-living nature of forest stands and the diversity of development patterns. Several studies analyzed tree height as a function of site-related environmental variables used as proxies of productivity (Bontemps and Bouriaud, 2014; Huang et al., 2017; Günlü et al., 2019; Pretzsch, 2020; Vacek et al., 2023; Viet et al., 2023). Although environmental factors affecting tree growth have been known for decades, these findings are based on a limited amount of field data collected under controlled conditions (Watt et al., 2015; Zhang et al., 2019; Barrio-Anta et al., 2020; Harvey et al., 2020). To remove these limitations, remote sensing data offer sufficient information to conduct an analysis on a large scale and under actual conditions of tree growth (Coops, 2015; Gopalakrishnan et al., 2019; Wang et al., 2022; Antón-Fernández et al., 2023; Appiah Mensah et al., 2023). Remote sensing data may contain significant systematic errors (Palahí et al., 2021; Wernick et al., 2021; Breidenbach et al., 2022). In this context, combining both field and remote sensing data on the same level enhances the accuracy of the resulting products, and some national forest inventories and studies have started to combine both data sources (Tomppo et al., 2008; Nagel et al., 2014; Venier et al., 2014; Breidenbach et al., 2020; Gustafsson et al., 2020; Lister et al., 2020; Næsset et al., 2020; Breidenbach et al., 2021; Schumacher et al., 2020; Sims, 2022).
This study presents one of the first analyses of temperate forest growth detailing tree species, previous disturbance patch areas, and a large number of environmental variables and management practices on a large scale. Our method integrates remote sensing data with inventory measurements to capitalize on the advantages of both data types: the abundance of remote sensing data and the extensive range of tree ages accessible through the inventory data. Thanks to global tree height maps (Besnard et al., 2021; Potapov et al., 2021; Lang et al., 2023), our growth monitoring analysis is applicable to any region across the globe. In this study, our primary objective is to examine the correlation between forest height growth, forest age, species, past disturbance patch size, and a combination of environmental factors to address the following key research questions: (A) can height, age, and tree species data obtained through remote sensing be effectively integrated with similar field measurements? (B) Can we categorize forest growth based on species, and what are the growth disparities between mixed-species forests and pure-species forests? (C) What is the influence of environmental factors beyond age and species on tree height? The study area is a large temperate forest region in the northern-central part of France that has a diversity of tree species and well-documented environmental variables.
We provide an overview of the data sources and processing methods in Section 2. In Section 3, we present a consistency assessment of the two data sources (question A), show the polymorphic age–height curves per species from satellite and field data (question B), and analyze the factors influencing spatial variations in height using explainable machine learning (question C). Then, Section 4 contains a discussion of the results, including the limitations and perspectives.
We combined satellite observations and national forest inventory measurements. The methodology consisted of three sequential steps (Figure 1). In the first step (see Figure 1A), we ensured the coherence of the data. This involved assessing the spatial consistency of satellite-derived parameters, tree height, tree age, and tree species, with the corresponding values acquired through field measurements at specific locations. In the second step (see Figure 1B), we modeled the relationship between tree height and age at the species level. To do so, we retrieved tree height, age, and tree species information from satellite observations and the French National Forest Inventory (NFI). These datasets were then integrated to construct empirical growth height–age curves of various tree species, with both satellite and field data. The height–age data were fitted using a Chapman–Richards equation. Additionally, we calculated simple metrics for comparing the height–age curve characteristics of different tree species. In this step, height is explained by age and species only. In the third step (see Figure 1C), we extended our analysis by considering a range of environmental variables, in addition to age and species, to better explain the variations in the height growth. This was done by building an explainable machine learning model to predict the height using the age, species, and the environmental variables as predictive features. This model is made parsimonious using recursive feature elimination to retain only the most influential environmental factors explaining the height. Finally, SHapley Additive exPlanations (SHAP) indexes (Lundberg and Lee, 2017; Lundberg et al., 2020) were used to elucidate the impact of the selected environmental variables on the prediction of tree height.
Figure 1. Flowchart. Input data (in black) are made consistent in step (A) and collocated with ground measurements, allowing us in step (B) to build an empirical height–age relationship for each species. In step (C), an explainable machine learning model is used to predict height from age, species, and environmental factors and separate the influence of each factor.
In 2009, the French metropolitan territory was divided into distinct forest regions for taking into account biogeographical factors determining forest production and the distribution of major forest habitat types. In this paper, we study the large ecological region (GRECO) B, which is in north-central France. The GRECO B (north-central semi-oceanic) has a relief of plains and plateaus not exceeding 400 m over a 149,800-km2 area (see Figure 2) (IGN, 2013). It has an oceanic climate with increasing continental influences.
Figure 2. (A) Tree species map from the National Institute of Geographic and Forest Information (IGN) (BDF). (B) French National Forest Inventory (NFI). (C) High-resolution tree height map (FORMS-H) of forests computed from GEDI, Sentinel-1, and Sentinel-2. (D) Year of disturbance of forests maps (S&S) computed from historical forest disturbances since 1986 from satellites.
Production forests are rather diverse in this region, both in terms of species and stand quality. The average forestation rate over the whole GRECO is 21% but with very important variations between sub-regions, which range from 5% to 51%. The tree species diversity within a stand is low in GRECO B: 42% of the forest stands are dominated by a single species and 33% by two species. The GRECO B region is predominantly made up of pure hardwood stands (80%), with pure conifers accounting for only 8%. A total of 90% of the forest areas have a regular structure with or without an understory (IGN, 2020). According to the NFI, 46% of the forest area is composed of oaks, the cultivated poplar covers 16%, and the other broadleaf covers 38%. The coniferous species amount to 8%. The plantations constitute only 13% of the stands in GRECO B, while the others are established by natural regeneration (IGN, 2020). The mixed stands most often combine oaks, pines, and birches. Forest management differs depending on whether the forest is privately owned (82%), state-owned (14%), or city-owned (5%) due to different legal constraints (IGN 2018b; Canopée, 2020). Public forests that are either state or city owned are subject to the Forestry Regime. In private forests larger than 25 ha, a management plan guaranteeing sustainable management is mandatory.
We used BD Forêt® version 2, henceforth referred to as BDF (see Figure 2A), to provide tree species localization in all the steps of the study (see Figure 1) (IGN 2018a). It was developed between 2007 and 2018 by the photo-interpretation of color and infrared images. A forest is described by the density of cover of the stand, its composition, and the dominant species for all patches that are more than 0.5 ha and larger than 20 m. We selected only the closed forest stands with canopy covers superior to 40%. For forest patches where at least 75% of the tree canopy consisted of the same species, the dominant species was identified among 16 common genera or species. Less frequent species were categorized under a general deciduous or coniferous classification. For the sake of simplicity, tree species refer to the 11 monospecific and 3 mixed-species categories explained in Table 1.
We used a new 10-m resolution satellite tree height map of France (Forest Multiple Satellite—Height [FORMS-H]; Schwartz et al., 2023; Schwartz et al., 2024; Fayad et al., 2024) corresponding to 2020 (see Figure 2C) to provide tree height in all steps of the study (see Figure 1). It was obtained from a deep learning model based on multi-stream remote sensing measurements (NASA’s GEDI LiDAR mission and ESA’s Copernicus Sentinel-1 and 2 satellites), and it does not use any ground measurements for calibration. The accuracy of FORMS-H has been evaluated with respect to the NFI height for 2020 over all of France (MAE = 2.94 m) and two ALS heights from high definition LiDAR measurements (MAE = 3.54 m and MAE = 4.50 m).
We used the European forest disturbance dataset at a resolution of 30 m built by Senf and Seidl (2021), hereafter S&S, derived from Landsat satellite data (see Figure 2D), to compute an age map of forests by 2020 (see Figure 1). We assumed that the selected disturbances were all severe enough to replace the previous stand (i.e., disturbance intensity greater than or equal to 0.95) and were followed by regrowth. The forest age was computed as the difference between 2020 and the year of disturbance.
We used plot data from the French NFI (see Figure 2B) (IGN, 2005) from 2017 to 2021 to provide tree height and age (see Figure 1). The French NFI consists of temporary plots distributed throughout the country on a systematic grid of 1 by 1 km surveyed over a 10-year rotation and made up of three concentric plots of radii of 6, 9, and 15 m. Dendrometric measurements are collected on the 6-, 9-, and 15-m circles for trees with diameters greater than 7.5 cm, 22.5 cm, and 47.5 cm, respectively. For each size class and tree species, a representative tree is selected for the plot, and a height measurement is taken. The dominant height of the plot is the average height of the tallest trees on the plot. Two trees chosen from the six tallest in the plot are cored at a height of 1.30 m to the pith for age measurement by counting annual rings. A general description of the stand including the vertical structure and tree species is established using a 25-m radius plot. We filtered out the trees that are not in the target population. We corrected the age values at 1.30 m to take into account the missing tree rings at heights between 0 and 1.30 m using calibrating measurements from the NFI made in 2005. In order to select the even-aged plots, we selected only the plots with a regular vertical structure with or without understory. In addition, we computed the mean and standard deviation of the distribution of the age per plot, and we selected the plots with a coefficient of variation of age smaller than 0.25 knowing that we kept the plots with single age data. The age of the plot is the average age of the trees within the plot.
We used 125 environmental variables from various datasets (see Figure 1C). Specifically, we incorporated 14 climatic features, encompassing temperature (Ninyerola et al., 2000; Bertrand et al., 2011; Richard, 2011), precipitation (Ninyerola et al., 2000; Richard, 2011), solar radiation (Lebourgeois and Piedallu, 2005; Piedallu and Gégout, 2007; 2008; Richard, 2011), evapotranspiration (Bertrand et al., 2011), climatic water budget (Piedallu and Gégout, 2007; Piedallu and Gégout, 2008), and the maximum and available water capacity (Al Majou et al., 2008; Piedallu et al., 2011; Piedallu et al., 2013). From the digital elevation model at a resolution of 25 m, we computed the aspect, the slope, the hillshade, and the roughness, leading to five altimetry features with the altitude (IGN, 2017). The 92 soil composition features include a 1-km forest soil pH map and composition data on various soil properties, texture components, essential nutrients, and minerals at a resolution of 16 km (Antoni et al., 2011). The geology of the soil is characterized by eight features (BGRM, 2017). The human impact on growth was taken into account by four features: the private or public ownership of the forest stand, whether the forest stand is in a national park or reserve, and the proximity to roads (i.e., an estimation of skidding distance) (IGN, 2022) and urban areas (IGN, 2018c).
This section makes forest attributes such as height, age, and species consistent across satellite observations and ground-based NFI measurements (see Figure 1A). The BDF tree species and the FORMS-H height were extracted at the precise localization of the NFI plots. Similarly the S&S age map was collocated with the NFI plots and compared with the associated NFI age. The NFI tree species was compared to the corresponding BDF tree species.
In this section, we explain how height–age relationships were derived for individual species (see Figure 1B). First, the FORMS-H height and S&S age pixels were assigned to a species from the corresponding BDF stand (dataset I). We then used the BDF map to assign a species to each NFI height and age combination to maintain consistency with the satellite data (dataset II).
Growth curves (height–age relationships) per species were computed from all triplets (height, age, and tree species) across the region using space-for-time substitution. For each tree species, the percentile-based growth curves denoted by
Figure 3. Conceptual figure of the growth curve processing per species (A). For each age bin, a percentile of the height distribution is computed, leading to percentile growth curves (PGCs) (shown as disks). Chapman–Richards fitting with an offset
To ensure a robust fit, we fixed the offset value
In order to check the consistency between satellite-derived and field-measured height–age relationships (see Figure 1A), a Chapman–Richards equation was fitted to the median growth curve
Using the fitted parameters, we derived three simple metrics to compare growth curve characteristics between species: the maximum height
Height variability between locations is not only dependent on age and species but also on the variability of environmental factors and management practices, which are included in a model based on interpretable machine learning (see Figure 1C). We opted for a random forest architecture due to its versatility and robustness as a machine learning algorithm (Breiman, 2001; Khajavi and Rastgoo, 2023; Zhu et al., 2023). The resilience of random forest to noisy and collinear data (Yue et al., 2023) allowed us to compute feature importance (Ishwaran and Lu, 2019), which we used to identify the key environmental variables affecting tree height.
We used the satellite-derived FORMS-H height map as a dependent variable and the S&S age map derived from forest disturbance analysis, the BDF tree species map, and 125 environmental parameters from various French datasets as predictors (see Table 2). We converted the S&S age map into aggregated patches and computed the S&S disturbed patches areas and the intensity of disturbance averaged over the patch, which were also used as predictors (see Table 2). A 10-m buffer was drawn inside the S&S disturbance patches, and the S&S age and disturbed patch area maps, along with the FORMS-H height data and the tree species map from BDF resampled at a resolution of 20 m, were collocated with the 125 environmental variables, resulting in dataset I. The tree species from the BDF and the tree height and age from the NFI were extracted using spatial consistency between the NFI plots and the BDF stands. Then, the 125 environmental variables maps were collocated with the center of the grid associated with the NFI plots, leading to dataset II. One-hot encoding was used to encode the categorical features. We included an additional feature to indicate whether the data originated from dataset I or II.
The fused dataset (dataset I + dataset II) was split into training and test subsets, ensuring that the ratios of tree species and age remained the same in each split. We built a random forest predicting height using all the features and optimized the hyperparameters. Then, using the training subset and the Python scikit-learn implementation of RandomForestRegressor with the parameters n_estimators = 100, max_depth = 15, max_features = 0.5, and criterion = “friedman_mse,” we performed a recursive feature elimination using a 3-fold cross-validation. At each iteration, the feature associated with the lowest feature importance computed is eliminated. The procedure is monitored by the computation of the coefficient of determination at each step. We computed the feature importance in two ways. First, starting from the 128 features, we computed the feature importance using Gini impurity, leading to a coefficient of determination R2 slowly increasing and then quickly decreasing with the number of features (see black data points in Figure 4A). Then, starting from the 29 features associated with the maximum of R2, we performed another recursive feature elimination while computing the feature importance using permutation with the R2 metric and five repeats (see blue data points in Figure 4A). We observed a slower decrease in R2 with the number of features when using permutation feature importance than when using Gini impurity feature importance. The subset of six features obtained by permutation feature importance was chosen as this subset corresponds to the smallest importance exceeding 5%. In order to avoid overfitting, the parameter max_depth was then set to 11 for the final model.
Figure 4. Recursive feature elimination. (A) Coefficient of determination R2 versus the number of features obtained from the recursive feature elimination. The mean values of R2 (markers) and the standard deviation (filled color) correspond to the 3-fold distribution after each step corresponding to the elimination of the least important feature. The feature importance has been computed using the Gini impurity (black) and permutation importance with the R2 metric (blue). The red dashed line corresponds to six features. (B–D) Performances of the random forest models with 128 features (B), 29 features (C), and 6 features (D). Density plots of the predicted height versus the true height, with brighter colors indicating a higher density of points. The dashed line represents the 1:1 axis. Distributions and error between the true and predicted heights. The boxplots show the variation in the error with the true height. The median value is represented by a red line, while the upper and lower quartiles are represented by the upper and lower edges, respectively. The whiskers symbolize the 5th and 95th percentiles.
In order to analyze the sensitivity of each of the six explanatory variables on the simulation of tree height, we used SHAP (Lundberg and Lee, 2017; Lundberg et al., 2020). SHAP is a game-theoretic approach that attributes the contribution of each feature to the prediction of a model for a specific instance. The SHAP values indicate the difference induced by a specific feature value to the predicted height with respect to the mean predicted height (Lundberg and Lee, 2017). Consequently, we can assess the significance of various features. The SHAP interaction values differentiate between the main effect term and the interactions of a pair of features (Lundberg et al., 2020). The remaining effects or main effects of a feature are defined as the difference between the SHAP value and the off-diagonal SHAP interaction values. For each tree species, we randomly sampled 500 rows of data, each from different BDF stands from the test subset. For the tree species with an insufficient number of stands, the number of data is the number of rows. The error metrics on this sample are MAE = 3.92 m and R2 = 0.103, which are similar to the metrics computed over the whole test subset. We computed the SHAP values and the SHAP interaction values for the 4,134 data using the SHAP Python package. The expected mean value of the model is 11.34 m. The feature importance was computed by permutation with respect to the R2 metric with 30 repeats on the same subset used for the SHAP analysis. The SHAP feature importances were obtained using the sum of the absolute SHAP values per feature scaled by the sum over the features (L1 norm).
We compared the tree species of the NFI plots and the BDF, the height derived from satellites with that observed at the NFI plots, and the age inferred from S&S with that reported for NFI plots. The BDF species classification is not very coherent with the NFI observations: the sample-weighted F1-score (i.e., harmonic mean of precision and recall) is 0.45. Hence, we used the BDF tree species in the following for the NFI data. For height consistency, the results (see Figure 5A) are very good and comparable with an error of 2.94 m obtained over the whole of France (Schwartz et al., 2023). For age (see Figure 5B), the mean absolute error on the stand age between S&S and NFI is 11 years, but it becomes 6 years (Senf and Seidl, 2021) when removing plots with at least one forest edge and a tree cover smaller than 40% (see Figure 5B). The presence of forest edges and the low tree cover density are likely to introduce bias into the age estimation for the NFI. Furthermore, given that the median patch area is 4 30-m pixels, it is likely that these errors are due to geolocalization errors and edge effects of the disturbed patches.
Figure 5. Comparison of satellite-derived height and age with those obtained from the NFI and consistency of growth curves across satellite and NFI data. (A) Distribution, error, and density plots of the satellite (FORMS-H) height versus the NFI height. (B) Distribution, error, and density plots of the satellite (S&S) age versus the NFI age. The dashed line represents the 1:1 axis. Data points likely resulting from geolocation errors and edge effects and the associated MAE are in gray. The median value is represented by a dotted line, while the upper and lower quartiles are represented by the upper and lower edges, respectively. The whiskers symbolize the 5th and 95th percentiles. Coefficient of determination R2 (C) and fitted parameters (A–F) for the 14 tree species associated with the Chapman–Richards equation fitted to the median growth curve
We compared the growth curves based on the three curve fitting methods explained in Section 2.4. While R2 is similar for M2 and M3, it is significantly lower for M1. Nevertheless, the fitted parameter values are similar between the three methods when considering the error margins (see Figures 5C–F). This result suggests that combining satellite, NFI height, and age allows us to better define the shape of the growth curves at young ages rather than using only the NFI data. The satellite data are consistent with the NFI reported values whether or not they are used together in the fit. Based on this, we adopted M3 for defining the “best” growth curves shown in Section 3.2.
The height–age patterns for five broadleaf species, six coniferous species, and other mixed forests are shown in Figure 6. While the growth of deciduous oak, mixed broadleaf, and mixed species is described by a large number of points in both datasets, species such as chestnut, locust, fir/spruce, mixed pines, and mixed conifer lack NFI data to constrain their long-term growth. From a visual assessment of the growth curves (see Figure 6), beech, Douglas, Austrian pines, and fir/spruce seem to have an almost linear growth. The growth of beech and Douglas appears to reach the largest height, larger than that of oak, locust, chestnut, and maritime and Austrian pines. Poplars stand out due to their fast initial growth, which is the opposite of oak, beech, Scotch pine, and mixed broadleaf. For Douglas fir, Austrian pine, and fir/spruce stands, the low number of measurements at ages higher than 50 years does not permit us to depict or to estimate the asymptotic value of the maximum dominant height.
Figure 6. Growth curves for different tree species. Satellite data are in color (green for pure deciduous trees, red for pure coniferous trees, and blue for mixed species), and NFI data are in black. The median (dots), interquartile range (dark filled color), and the interval between the 5th and 95th quantiles (light filled color) are shown for satellite data and deciduous oak, mixed broadleaf, and mixed species of NFI data. For the other tree species of NFI data, the black points correspond to the data points. The numbers of 10-m pixels (left number) and BDF stands (right number in parentheses) are given in black for the NFI data and in color for satellite data.
Then, we compared the growth curves after fitting the data with Eq. 1, fit parameters, and metrics from Section 2.5 (see Figure 7). The fitting yielded accurate results: R2 averaged over the five percentile growth curves (
Figure 7. Fitted growth curves, height versus age, for different tree species. The satellite data are in color (green for the pure deciduous trees, red for the pure coniferous trees, and blue for the mixed species). The NFI data have been digitized into 10-year ranges.
The growth metrics are not significantly different on average between deciduous trees, conifers, or a mixture of both (see Figures 8A–C): when comparing coniferous and deciduous species, we observe similarities in both
Figure 8. Growth metrics associated with the median growth curve PGC50. Maximum height
The growth curves (see Figure 6) suggest a first-order impact of the age and tree species in determining spatial variations in the height of forests. Using explanatory machine learning, we identified other features that might explain variations in height, confirming a dominant role for age and species that Figure 4 suggests. While the coefficient of determination R2 of the random forest model prediction decreases with the number of features (see Figure 4A), the mean absolute errors and the coefficients of determination using 128 features, 30 features, or 6 features are similar (see Figures 4B–D). The model with six features is almost as good at predicting the height as the models using more features, and it predicts height from satellite and NFI data with similar precision (MAE = 3.32 m and R2 = 0.47 for dataset I; MAE = 3.20 m and R2 = 0.53 for dataset II). On average, for different tree species, the model performs with a MAE of 3.38 m and R2 of 0.43 with standard deviations of 0.39 m and 0.11, respectively. The six selected features are mostly uncorrelated (see Figure 9A). From the permutation feature importance (see Figure 9B), the main driver to the estimated height is tree age (49%), followed by disturbed patch area, which is defined as the area of the patch within a stand impacted by a disturbance (19%), tree species (10%), and the environmental parameters such as soil pH (9%), altitude (8%), and summer climatic water budget (SCWB) (5%). The impact of the patch area also depends on the tree species (see Figure 10). For small patches, the distribution of values is shifted toward larger values and more diffused than for larger patches.
Figure 9. Impact of the six features on the predicted forest height. Correlation of the features (A). Feature importances of the six-feature model computed using permutation and the R2 metrics and SHAP values (B). Boxplot of the distributions of the disturbed patch area (C), soil pH (D), the altitude (E), and the summer climatic water budget (SCWB) (F) for each tree species. The data correspond to the data used to compute the SHAP values and interaction values. The median value is represented by a black line, while the upper and lower quartiles are represented by the upper and lower edges, respectively. The whiskers indicate the 5th and 95th percentiles. SHAP value versus feature value for the following features: age (G), disturbed patch area (H), tree species (I), soil pH (J), altitude (K), and SCWB (L). The expected height value of 11.34 m is represented by a dashed red line. Sum of the absolute value of the SHAP interaction values (M).
Figure 10. Growth curves for different tree species and disturbed patch areas. The median (markers) and interquartile range (filled color). The disturbed patch areas are categorized into small (blue), medium (purple), and large (orange).
The influence of environmental variables on height estimates is constrained by their range of variation across the study region, illustrated by the distributions of the disturbed patch area, soil pH, the altitude, and SCWB (see Figures 9C–F). Except for the patch area, the distributions of the features are different between species. We investigated the impact of the features on the model height estimates using Shapley indexes (Lundberg and Lee, 2017), hereafter referred to as SHAP. The feature importances from permutation and SHAP analysis are similar (see Figure 9B). The SHAP values correspond to the first-order impact of the features on the height (see Figures 9G–L). The effect of tree age (see Figure 9G) shows that the model reproduces well the exponential growth of the forest (Bontemps and Duplat, 2012; Pretzsch, 2020). The model predicts larger heights when the disturbed area is smaller (see Figure 9H). Except for poplars and, to a lesser extent, Douglas firs and fir/spruces, the SHAP values do not depend much on the tree species (see Figure 9I). Soil pH only has a decreasing impact if the soil pH is sufficiently basic (see Figure 9J). The altitude has a non-monotonous effect on the model output (see Figure 9K). The predicted height increases with the SCWB (see Figure 9L). The second-order impact of features on the predicted height, which is how one feature influences the importance of another, was investigated using the SHAP interaction values (Lundberg et al., 2020) (see Figure 9M). Mainly, the forest age has a strong interaction with the disturbed patch area (see Figure 11, cells 1,2 and 2,1). The growth of poplars and Douglas stands out from the growth of other tree species (see Figure 11, cell 3). For most tree species, the predicted height decreases when the disturbed patch area increases, but the effect is reversed for poplars (see Figure 11, cell 3,2).
Figure 11. SHAP interaction value between two features. SHAP interaction value versus one feature value, with the interacting feature value highlighted on the off-diagonal and remaining SHAP effect on the diagonal for the following features: age (1), disturbed patch area (2), tree species (3), soil pH (4), altitude (5), and SCWB (6). For the age and the disturbed patch area, the color map is in the logarithmic scale. The expected height value of 11.34 m is represented by a dashed line.
This study combines data obtained from field measurements and satellite observations to derive height–age growth curves and retrieve well-known drivers of height. The consistency between satellite and NFI data is to be noted as a main result. The combination of satellite and field data enhances the result accuracy (Hasenauer et al., 2012; Neumann et al., 2015; Næsset et al., 2020; Breidenbach et al., 2021).
The growth curves derived from the satellite and NFI data are in agreement with similar results found in the literature for broadleaf (Crockford and Savill, 1991; Álvarez-González et al., 2010; Bontemps et al., 2012; Rédei et al., 2014; Pretzsch et al., 2015; Viet et al., 2023), conifer (Aughanbaugh, 1960; Lemoine, 1991; Zhang et al., 1993; Bravo-Oviedo et al., 2004; Cieszewski et al., 2007; Pretzsch et al., 2010; Pretzsch et al., 2015), or mixed species (Pretzsch et al., 2010; Pretzsch et al., 2015; del Río et al., 2016). Studies of the growth of mixed tree species show a slower early growth than that of pure species, which is in line with our results (del Río et al., 2016). The similarity in height between pure and mixed forests in our results can be attributed to the irregular canopy height and low density, which is not accurately captured at a resolution of 10 m (Pretzsch and Schütze, 2016; Aldea et al., 2021). The effects of tree species on growth cannot be aggregated into a single broadleaf/coniferous/mixed species group. To date, a number of studies calculating height or biomass have used the broadleaf/coniferous distinction alone to take into account the effect of tree species, thus creating a source of uncertainty (Santoro and Cartus, 2023; Schwartz et al., 2023).
Our random forest model predicts forest height with an accuracy (MAE = 3.20 m for 128 features) comparable to the accuracy of the satellite height map FORMS-H (MAE = 2.94 m with respect to the NFI and MAE = 3.54 m or 4.50 m with respect to LiDAR HD measurements). Hence, the accuracy of the model is not reduced by the simplicity of its architecture because it is already constrained by the uncertain height values from FORMS-H.
Apart from the age and tree species features that were expected to be the main predictors of height, our feature elimination procedure selected three environmental variables (soil pH, altitude, and summer climatic water budget) and the disturbed patch area. Other features such as the difference between public and private forests and the proximity to roads and cities had a negligible impact on the height prediction. Low relief and a well-developed road network enable forest management throughout the region (Aguiar et al., 2021). Moreover, proximity to urban areas such as the Île-de-France region (12 million inhabitants for 12,000 km2) does not seem to influence forest growth much (Stone and Skelly, 1974; Pregitzer et al., 2021; Franceschi et al., 2023). The potential management differences, highlighted by the forest ownership and whether the stand is in a natural park, may not be very noticeable since private forests are subject to all types of management, from intensive cultivation to complete neglect. The ownership of the forest and the state park features were not found to be important, likely because this feature inadequately portrays management practices, such as thinning or selective logging.
The importance of the disturbed patch area on the height model may be explained by the fact that the tree age is based on the hypothesis that the forest starts to grow back immediately after the disturbance. While poplar stands are mostly planted after cutting, natural regeneration prevails for the majority of other tree species (IGN, 2020), which is in agreement with our results. In contrast, natural regeneration is impacted by the micro-climate and the preservation and dispersion of seeds, in part driven by the disturbed patch area (Parde, 1962; d Oliveira and Ribas, 2011; Hanbury-Brown et al., 2022).
Overall, our model accurately predicts the most favorable soil pH condition for the selected tree species to reach a higher height at a given age (Hjelm and Rytter, 2016; Cremer and Prietzel, 2017; Arrobas et al., 2018). Since the SCWB, which corresponds to the amount of rainfall available to plants once evaporation and transpiration needs have been met, is always negative (see Figure 9F), the trees draw on soil reserves to survive the summer. Notably, in the process of recursive feature elimination, the SCWB was chosen over summer available water capacity (SAWC), which quantifies the water available to plants. Since water supplies are rarely insufficient in the studied area, the accessibility of water to trees is primarily constrained by rainfall. The susceptibility of beech (Weber et al., 2013; Leuschner, 2020), Douglas (Sergent et al., 2014; Chauvin et al., 2019), fir/spruces (Solberg, 2004; Lévesque et al., 2013), and Scotch pines (Bigler et al., 2006; Eilmann et al., 2009; Martínez-Vilalta et al., 2012) to drought aligns with the reports of previous research. The other species demonstrate an ability to withstand drought conditions proficiently (Eilmann et al., 2009; Lévesque et al., 2013). Mixed-species categories encompass the effects of multiple species not specifically identified by the BDF and the impacts of mixed species, making it difficult to conclude.
Different biases are associated with the satellite-derived and ground measurement data: since the BDF was computed from images spanning 10 years, it is possible that the recent disturbances have led to a change in the tree species. The historical S&S disturbance map only indicates one disturbance event between 1984 and 2020, providing no insight into the age homogeneity of the resulting forest. Additionally, its 30-m resolution is coarse, potentially leading to boundary effects.
An indirect space-for-time approach was used to obtain the growth curves. Assuming that the productivity of each forest site does not vary over time, temporal dynamics were inferred by studying multiple sites that differ under abiotic conditions not directly related to stand age or time (Fukami and Wardle, 2005). The environmental variables are also supposed to be constant over the last 100 years. Consequently, any application of these models to predict changes in site productivity over time is reliant on the underlying assumption of equivalence between the stands. In the context of climate change, the invariance of the climatic and environmental variables is challenged, potentially compromising our results (Rolo et al., 2016; Wu et al., 2022; Yue et al., 2023).
The consistency of the tree height and age derived from satellite and ground measurements is promising for monitoring a forest and its evolution at larger scales, i.e., regional or country level. Here, satellite and ground-based data were found to be consistent, and thus, they were combined to produce the first growth curves per species in a large forested region (question A). The significant variations in tree growth according to tree species cannot be accurately reported by a coarse categorization into broadleaf or conifer (question B). Several environmental factors that are known to influence tree height were identified in addition to tree age and species: soil pH, altitude, and summer climatic water budget. In addition, the post-disturbance growth was shown to be highly dependent on the disturbed area due to different regeneration methods, natural or plantation (question C). At a regional level, our results did not showcase any discernible human impact on tree growth, whether from urban vicinity or different management techniques, likely due to a lack of detailed data, specifically on management types. Management types may also be correlated with other parameters, such as tree species, soil, and climate, which may make it difficult to isolate their effects on tree growth.
The datasets presented in this study can be found in online repositories. The names of the repository/repositories and accession number(s) can be found at: https://zenodo.org/doi/10.5281/zenodo.10079578.
AP-T: conceptualization, data curation, formal analysis, methodology, validation, writing–original draft, and writing–review and editing. PC: conceptualization, funding acquisition, methodology, writing–original draft, and writing–review and editing. MS: writing–review and editing. IF: methodology and writing–review and editing. YX: formal analysis and writing–review and editing. FR: writing–review and editing. AdT: writing–review and editing. J-ML: writing–review and editing.
The author(s) declare that financial support was received for the research, authorship, and/or publication of this article. This work was supported by the French German AI4Forest R-22-FAI1-0002-01. AP-T was supported by the ANR project “plan France Relance” with CEA Kayrros.
Authors IF and AdT were employed by Kayrros SAS.
The remaining authors declare that the research was conducted in the absence of any commercial or financial relationships that could be construed as a potential conflict of interest.
All claims expressed in this article are solely those of the authors and do not necessarily represent those of their affiliated organizations, or those of the publisher, the editors, and the reviewers. Any product that may be evaluated in this article, or claim that may be made by its manufacturer, is not guaranteed or endorsed by the publisher.
Aguiar, M. O., Fernandes da Silva, G., Mauri, G. R., Ribeiro de Mendonça, A., Junio de Oliveira Santana, C., Marcatti, G. E., et al. (2021). Optimizing forest road planning in a sustainable forest management area in the Brazilian Amazon. J. Environ. Manage 288, 112332. doi:10.1016/j.jenvman.2021.112332
Aldea, J., Ruiz-Peinado, R., del Río, M., Pretzsch, H., Heym, M., Brazaitis, G., et al. (2021). Species stratification and weather conditions drive tree growth in Scots pine and Norway spruce mixed stands along Europe. For Ecol Manag 481, 118697. doi:10.1016/j.foreco.2020.118697
Al Majou, H., Bruand, A., Duval, O., Le Bas, C., and Vautier, A. (2008). Prediction of soil water retention properties after stratification by combining texture, bulk density and the type of horizon. Soil Use Manag. 24, 383–391. doi:10.1111/j.1475-2743.2008.00180.x
Álvarez-González, J. G., Zingg, A., and Gadow, K. V. (2010). Estimation de la croissance dans les hêtraies: une étude basée sur des expérimentations à long terme en Suisse. Ann For Sci 67, 307. doi:10.1051/forest/2009113
Antón-Fernández, C., Hauglin, M., Breidenbach, J., and Astrup, R. (2023). Building a high-resolution site index map using boosted regression trees: the Norwegian case. Can J For Res 53, 416–429. doi:10.1139/cjfr-2022-0198
Appiah Mensah, A., Jonzén, J., Nyström, K., Wallerman, J., and Nilsson, M. (2023). Mapping site index in coniferous forests using bi-temporal airborne laser scanning data and field data from the Swedish national forest inventory. For Ecol Manag 547, 121395. doi:10.1016/j.foreco.2023.121395
Arrobas, M., Afonso, S., and Rodrigues, M. Â. (2018). Diagnosing the nutritional condition of chestnut groves by soil and leaf analyses. Sci. Hortic. 228, 113–121. doi:10.1016/j.scienta.2017.10.027
Aughanbaugh, J. (1960). Comparative growth of height conifers in a plantation in mahoning county. Ohio.
Barrio-Anta, M., Castedo-Dorado, F., Cámara-Obregón, A., and López-Sánchez, C. A. (2020). Predicting current and future suitable habitat and productivity for Atlantic populations of maritime pine (Pinus pinaster Aiton) in Spain. Ann For Sci 77, 41–19. doi:10.1007/s13595-020-00941-5
Beck, J., Wirt, B., Armston, J., et al. (2020). GLOBAL ecosystem dynamics investigation (GEDI) level 2 user guide.
Bertrand, R., Lenoir, J., Piedallu, C., Riofrío-Dillon, G., de Ruffray, P., Vidal, C., et al. (2011). Changes in plant community composition lag behind climate warming in lowland forests. Nature 479, 517–520. doi:10.1038/nature10548
Besnard, S., Koirala, S., Santoro, M., Weber, U., Nelson, J., Gütter, J., et al. (2021). Mapping global forest age from forest inventories, biomass and climate data. Earth Syst. Sci. Data 13, 4881–4896. doi:10.5194/essd-13-4881-2021
Bigler, C., Bräker, O. U., Bugmann, H., Dobbertin, M., and Rigling, A. (2006). Drought as an inciting mortality factor in Scots pine stands of the valais, Switzerland. Ecosystems 9, 330–343. doi:10.1007/s10021-005-0126-2
Bontemps, J.-D., and Bouriaud, O. (2014). Predictive approaches to forest site productivity: recent trends, challenges and future perspectives. For Int J For Res 87, 109–128. doi:10.1093/forestry/cpt034
Bontemps, J.-D., and Duplat, P. (2012). A non-asymptotic sigmoid growth curve for top height growth in forest stands. For Int J For Res 85, 353–368. doi:10.1093/forestry/cps034
Bontemps, J.-D., Herve, J.-C., Duplat, P., and Dhôte, J.-F. (2012). Shifts in the height-related competitiveness of tree species following recent climate warming and implications for tree community composition: the case of common beech and sessile oak as predominant broadleaved species in Europe. Oikos 121, 1287–1299. doi:10.1111/j.1600-0706.2011.20080.x
Bravo-Oviedo, A., Río, M. D., and Montero, G. (2004). Site index curves and growth model for Mediterranean maritime pine (Pinus pinaster Ait.) in Spain. For Ecol Manag 201, 187–197. doi:10.1016/j.foreco.2004.06.031
Breidenbach, J., Ellison, D., Petersson, H., Korhonen, K. T., Henttonen, H. M., Wallerman, J., et al. (2022). Harvested area did not increase abruptly—how advancements in satellite-based mapping led to erroneous conclusions. Ann For Sci 79, 2. doi:10.1186/s13595-022-01120-4
Breidenbach, J., Granhus, A., Hylen, G., Eriksen, R., and Astrup, R. (2020). A century of National Forest Inventory in Norway – informing past, present, and future decisions. For Ecosyst 7, 46. doi:10.1186/s40663-020-00261-0
Breidenbach, J., Ivanovs, J., Kangas, A., Nord-Larsen, T., Nilsson, M., and Astrup, R. (2021). Improving living biomass C-stock loss estimates by combining optical satellite, airborne laser scanning, and NFI data. Can J For Res 51, 1472–1485. doi:10.1139/cjfr-2020-0518
Canopée (2020). Bas carbone, hauts risques, une analyse critique des projets forestiers label bas carbone en France.
Chauvin, T., Cochard, H., Segura, V., and Rozenberg, P. (2019). Native-source climate determines the Douglas-fir potential of adaptation to drought. For Ecol Manag 444, 9–20. doi:10.1016/j.foreco.2019.03.054
Chazdon, R. L., Broadbent, E., Rozendaal, D. M. A., Bongers, F., Zambrano, A. M. A., Aide, T. M., et al. (2016). Carbon sequestration potential of second-growth forest regeneration in the Latin American tropics. Sci. Adv. 2, e1501639. doi:10.1126/sciadv.1501639
Cieszewski, C. J., Strub, M., and Zasada, M. (2007). New dynamic site equation that fits best the Schwappach data for Scots pine (Pinus sylvestris L.) in Central Europe. For Ecol Manag 243, 83–93. doi:10.1016/j.foreco.2007.02.025
Coops, N. C. (2015). Characterizing forest growth and productivity using remotely sensed data. Curr For Rep 1, 195–205. doi:10.1007/s40725-015-0020-x
Cremer, M., and Prietzel, J. (2017). Soil acidity and exchangeable base cation stocks under pure and mixed stands of European beech, Douglas fir and Norway spruce. Plant Soil 415, 393–405. doi:10.1007/s11104-017-3177-1
Crockford, K. J., and Savill, P. S. (1991). Preliminary yield tables for oak coppice. For Int J For Res 64, 29–50. doi:10.1093/forestry/64.1.29
D Amato, A. W., Palik, B. J., Bradford, J. B., and Fraver, S. (2011). Sustaining forest ecosystems: a framework for managing temperate and boreal forest landscapes in the face of global change. For Ecol Manage 261, 951–962. doi:10.1016/j.foreco.2011.01.001
del Río, M., Pretzsch, H., Alberdi, I., Bielak, K., Bravo, F., Brunner, A., et al. (2016). Characterization of the structure, dynamics, and productivity of mixed-species stands: review and perspectives. Eur J For Res 135, 23–49. doi:10.1007/s10342-015-0927-6
d Oliveira, M. V. N., and Ribas, L. A. (2011). Forest regeneration in artificial gaps twelve years after canopy opening in Acre State Western Amazon. For Ecol Manag 261, 1722–1731. doi:10.1016/j.foreco.2011.01.020
Dubayah, R., Blair, J. B., Goetz, S., Fatoyinbo, L., Hansen, M., Healey, S., et al. (2020). The global ecosystem dynamics investigation: high-resolution laser ranging of the earth’s forests and topography. Sci. Remote Sens. 1, 100002. doi:10.1016/j.srs.2020.100002
Eilmann, B., Zweifel, R., Buchmann, N., Fonti, P., and Rigling, A. (2009). Drought-induced adaptation of the xylem in Scots pine and pubescent oak. Tree Physiol. 29, 1011–1020. doi:10.1093/treephys/tpp035
Fayad, I., Ciais, P., Schwartz, M., Wigneron, J. P., Baghdadi, N., de Truchis, A., et al. (2024). Hy-TeC: a hybrid vision transformer model for high-resolution and large-scale mapping of canopy height. Remote Sens. Environ. 302, 113945. doi:10.1016/j.rse.2023.113945
Franceschi, E., Moser-Reischl, A., Honold, M., Rahman, M. A., Pretzsch, H., Pauleit, S., et al. (2023). Urban environment, drought events and climate change strongly affect the growth of common urban tree species in a temperate city. Urban For Urban Green 128083, 128083. doi:10.1016/j.ufug.2023.128083
Franklin, J. F., Spies, T. A., Van Pelt, R., Carey, A. B., Thornburgh, D. A., Berg, D. R., et al. (2002). Disturbances and structural development of natural forest ecosystems with silvicultural implications, using Douglas-fir forests as an example. For Ecol Manage 155, 399–423. doi:10.1016/S0378-1127(01)00575-8
Fukami, T., and Wardle, D. A. (2005). Long-term ecological dynamics: reciprocal insights from natural and anthropogenic gradients. Proc. R. Soc. B Biol. Sci. 272, 2105–2115. doi:10.1098/rspb.2005.3277
Gopalakrishnan, R., Kauffman, J. S., Fagan, M. E., Coulston, J. W., Thomas, V. A., Wynne, R. H., et al. (2019). Creating landscape-scale site index maps for the southeastern US is possible with airborne LiDAR and Landsat imagery. Forests 10, 234. doi:10.3390/f10030234
Günlü, A., Bulut, S., Keleş, S., and Ercanlı, İ. (2019). Evaluating different spatial interpolation methods and modeling techniques for estimating spatial forest site index in pure beech forests: a case study from Turkey. Environ. Monit. Assess. 192, 53. doi:10.1007/s10661-019-8028-5
Gustafsson, L., Bauhus, J., Asbeck, T., Augustynczik, A. L. D., Basile, M., Frey, J., et al. (2020). Retention as an integrated biodiversity conservation approach for continuous-cover forestry in Europe. Ambio 49, 85–97. doi:10.1007/s13280-019-01190-1
Hanbury-Brown, A. R., Ward, R. E., and Kueppers, L. M. (2022). Forest regeneration within Earth system models: current process representations and ways forward. New Phytol. 235, 20–40. doi:10.1111/nph.18131
Harvey, J. E., Smiljanić, M., Scharnweber, T., Buras, A., Cedro, A., Cruz-García, R., et al. (2020). Tree growth influenced by warming winter climate and summer moisture availability in northern temperate forests. Glob. Change Biol. 26, 2505–2518. doi:10.1111/gcb.14966
Hasenauer, H., Petritsch, R., Zhao, M., Boisvenue, C., and Running, S. W. (2012). Reconciling satellite with ground data to estimate forest productivity at national scales. Forest ecology and management 276, 196–208. doi:10.1016/j.foreco.2012.03.022
Hjelm, K., and Rytter, L. (2016). The influence of soil conditions, with focus on soil acidity, on the establishment of poplar (Populus spp.). New For 47, 731–750. doi:10.1007/s11056-016-9541-9
Huang, S., Ramirez, C., Conway, S., Kennedy, K., Kohler, T., and Liu, J. (2017). Mapping site index and volume increment from forest inventory, Landsat, and ecological variables in Tahoe National Forest, California, USA. Can J For Res 47, 113–124. doi:10.1139/cjfr-2016-0209
IGN (2005). National forest inventory of France raw data. Annual campaigns 2005 onwards, IGN – Inventaire For. Natl. français. Available at: https://inventaire-forestier.ign.fr/dataIFN/ (Accessed May 1, 2024).
IGN (2018b) L’IF n°41 Portrait des forêts privées avec ou sans PSG, 16 pages. Institut national de l’information géographique et forestière (IGN)
IGN (2018c). L’IF n°44 L’IGN accompagne les parcs naturels régionaux, 16 pages. Institut national de l’information géographique et forestière (IGN).
Ishwaran, H., and Lu, M. (2019). Standard errors and confidence intervals for variable importance in random forest regression, classification, and survival. Statistics in medicine 38 (4), 558–582. doi:10.1002/sim.7803
Khajavi, H., and Rastgoo, A. (2023). Predicting the carbon dioxide emission caused by road transport using a Random Forest (RF) model combined by Meta-Heuristic Algorithms. Sustain Cities Soc. 93, 104503. doi:10.1016/j.scs.2023.104503
Korosuo, A., Pilli, R., Abad Viñas, R., Blujdea, V. N. B., Colditz, R. R., Fiorese, G., et al. (2023). The role of forests in the EU climate policy: are we on the right track? Carbon Balance Manag. 18, 15. doi:10.1186/s13021-023-00234-0
Lang, N., Jetz, W., Schindler, K., and Wegner, J. D. (2023). A high-resolution canopy height model of the Earth. Nat. Ecol. Evol. 7, 1778–1789. doi:10.1038/s41559-023-02206-6
Lebourgeois, F., and Piedallu, C. (2005). Appréhender le niveau de sécheresse dans le cadre des études stationnelles et de la gestion forestière à partir d’indices bioclimatiques. Rev For Fr 57, 331. doi:10.4267/2042/5055
Lemoine, B. (1991). Growth and yield of maritime pine (Pinus pinaster Ait): the average dominant tree of the stand. Ann Sci For 48, 593–611. doi:10.1051/forest:19910508
Leuschner, C. (2020). Drought response of European beech (Fagus sylvatica L.)—a review. Perspect. Plant Ecol. Evol. Syst. 47, 125576. doi:10.1016/j.ppees.2020.125576
Lévesque, M., Saurer, M., Siegwolf, R., Eilmann, B., Brang, P., Bugmann, H., et al. (2013). Drought response of five conifer species under contrasting water availability suggests high vulnerability of Norway spruce and European larch. Glob. Change Biol. 19, 3184–3199. doi:10.1111/gcb.12268
Liang, J., Crowther, T. W., Picard, N., Wiser, S., Zhou, M., Alberti, G., et al. (2016). Positive biodiversity-productivity relationship predominant in global forests. Science 354, aaf8957. doi:10.1126/science.aaf8957
Lister, A. J., Andersen, H., Frescino, T., Gatziolis, D., Healey, S., Heath, L. S., et al. (2020). Use of remote sensing data to improve the efficiency of national forest inventories: a case study from the United States national forest inventory. Forests 11, 1364. doi:10.3390/f11121364
Lundberg, S. M., Erion, G., Chen, H., DeGrave, A., Prutkin, J. M., Nair, B., et al. (2020). From local explanations to global understanding with explainable AI for trees. Nat. Mach. Intell. 2, 56–67. doi:10.1038/s42256-019-0138-9
Lundberg, S. M., and Lee, S.-I. (2017). “A unified approach to interpreting model predictions,” in Advances in neural information processing systems, I. Guyon, U. V. Luxburg, and S. Bengio, (Curran Associates, Inc).
Luyssaert, S., Schulze, E. D., Börner, A., Knohl, A., Hessenmöller, D., Law, B. E., et al. (2008). Old-growth forests as global carbon sinks. Nature 455, 213–215. doi:10.1038/nature07276
Martínez-Vilalta, J., López, B. C., Loepfe, L., and Lloret, F. (2012). Stand- and tree-level determinants of the drought response of Scots pine radial growth. Oecologia 168, 877–888. doi:10.1007/s00442-011-2132-8
MCPFE (2002). Improved pan-European indicators for sustainable forest management. Vienna, Austria: MCPFE.
Messier, C., Bauhus, J., Doyon, F., Maure, F., Sousa-Silva, R., Nolet, P., et al. (2019). The functional complex network approach to foster forest resilience to global changes. For. Ecosyst. 6, 21. doi:10.1186/s40663-019-0166-2
Montreal Process Working Group (1994). Criteria and indicators for the conservation and sustainable management of temperate and boreal forests. Santiago declaration.
Nagel, T. A., Svoboda, M., and Kobal, M. (2014). Disturbance, life history traits, and dynamics in an old-growth forest landscape of southeastern Europe. Ecol. Appl. 24, 663–679. doi:10.1890/13-0632.1
Næsset, E., McRoberts, R. E., Pekkarinen, A., Saatchi, S., Santoro, M., Trier, Ø. D., et al. (2020). Use of local and global maps of forest canopy height and aboveground biomass to enhance local estimates of biomass in miombo woodlands in Tanzania. Int. J. Appl. Earth Obs. Geoinformation 93, 102138. doi:10.1016/j.jag.2020.102138
Neumann, M., Zhao, M., Kindermann, G., and Hasenauer, H. (2015). Comparing MODIS net primary production estimates with terrestrial national forest inventory data in Austria. Remote Sensing 7 (4), 3878–3906. doi:10.1016/j.foreco.2012.03.022
Niinemets, Ü. (2010). Responses of forest trees to single and multiple environmental stresses from seedlings to mature plants: past stress history, stress interactions, tolerance and acclimation. For Ecol Manage 260, 1623–1639. doi:10.1016/j.foreco.2010.07.054
Ninyerola, M., Pons, X., and Roure, J. M. (2000). A methodological approach of climatological modelling of air temperature and precipitation through GIS techniques. Int. J. Climatol. 20, 1823–1841. doi:10.1002/1097-0088(20001130)20:14<1823::AID-JOC566>3.0.CO;2-B
Palahí, M., Valbuena, R., Senf, C., Acil, N., Pugh, T. A. M., Sadler, J., et al. (2021). Concerns about reported harvests in European forests. Nature 592, E15–E17. doi:10.1038/s41586-021-03292-x
Pan, Y., Birdsey, R. A., Fang, J., Houghton, R., Kauppi, P. E., Kurz, W. A., et al. (2011). A large and persistent carbon sink in the world's forests. Science 333, 988–993. doi:10.1126/science.1201609
Parde, J. (1962). La régéneration du pin noir d’Autriche en Lozère. Rev For Fr 931, 931. doi:10.4267/2042/24426
Piedallu, C., and Gégout, J. (2008). Efficient assessment of topographic solar radiation to improve plant distribution models. Agric For Meteorol 148, 1696–1706. doi:10.1016/j.agrformet.2008.06.001
Piedallu, C., and Gégout, J.-C. (2007). Calcul multi-échelle du rayonnement solaire pour la modélisation prédictive de la végétation. Ann For Sci 64, 899–909. doi:10.1051/forest:2007072
Piedallu, C., Gégout, J.-C., Bruand, A., and Seynave, I. (2011). Mapping soil water holding capacity over large areas to predict potential production of forest stands. Geoderma 160, 355–366. doi:10.1016/j.geoderma.2010.10.004
Piedallu, C., Gégout, J.-C., Perez, V., and Lebourgeois, F. (2013). Soil water balance performs better than climatic water variables in tree species distribution modelling. Glob. Ecol. Biogeogr. 22, 470–482. doi:10.1111/geb.12012
Poorter, L., Rozendaal, D. M. A., Bongers, F., Almeyda Zambrano, A. M., Balvanera, P., Becknell, J. M., et al. (2016). Biomass resilience of Neotropical secondary forests. Nature 530, 211–214. doi:10.1038/nature16512
Potapov, P., Li, X., Hernandez-Serna, A., Tyukavina, A., Hansen, M. C., Kommareddy, A., et al. (2021). Mapping global forest canopy height through integration of GEDI and Landsat data. Remote Sens. Environ. 253, 112165. doi:10.1016/j.rse.2020.112165
Pregitzer, C. C., Charlop-Powers, S., and Bradford, M. A. (2021). Natural area forests in US cities: opportunities and challenges. J For 119, 141–151. doi:10.1093/jofore/fvaa055
Pretzsch, H. (2009). “Forest dynamics, growth, and yield,” in Forest dynamics, growth and yield: from measurement to model. Editor H. Pretzsch (Berlin, Heidelberg: Springer), 1–39.
Pretzsch, H. (2020). The course of tree growth. Theory and reality. For Ecol Manag 478, 118508. doi:10.1016/j.foreco.2020.118508
Pretzsch, H., Block, J., Dieler, J., Dong, P. H., Kohnle, U., Nagel, J., et al. (2010). Comparison between the productivity of pure and mixed stands of Norway spruce and European beech along an ecological gradient. Ann For Sci 67, 712. doi:10.1051/forest/2010037
Pretzsch, H., del Río, M., Ammer, Ch, Avdagic, A., Barbeito, I., Bielak, K., et al. (2015). Growth and yield of mixed versus pure stands of Scots pine (Pinus sylvestris L.) and European beech (Fagus sylvatica L.) analysed along a productivity gradient through Europe. Eur J For Res 134, 927–947. doi:10.1007/s10342-015-0900-4
Pretzsch, H., and Schütze, G. (2016). Effect of tree species mixing on the size structure, density, and yield of forest stands. Eur J For Res 135, 1–22. doi:10.1007/s10342-015-0913-z
Pugh, T. A. M., Arneth, A., Kautz, M., et al. (2019). Role of forest regrowth in global carbon sink dynamics. Nature 570, 220–224. doi:10.1038/s41586-019-1361-8
Rédei, K., Csiha, I., Keserű, Z., Rásó, J., Kamandiné Végh, Á., and Antal, B. (2014). Growth and yield of black locust (robinia pseudoacacia L.) stands in nyírség growing region (North-East Hungary). South-East Eur For SEEFOR 5, 13–22. doi:10.15177/seefor.14-04
Richard, J.-B. (2011). Caractérisation de la contrainte hydrique des sols à l’aide de cartes numériques pour prendre en compte les effets potentiels du changement climatique dans les catalogues de stations forestières: Applications aux plateaux calcaires de Lorraine. Champagne-Ardenne Bourgogne. AgroParisTech.
Rolo, V., Olivier, P. I., Guldemond, R. A. R., and van Aarde, R. J. (2016). Validating space-for-time substitution in a new-growth coastal dune forest. Appl. Veg. Sci. 19, 235–243. doi:10.1111/avsc.12210
Santoro, M., and Cartus, O. (2023). ESA biomass climate change initiative (Biomass_cci): global datasets of forest above-ground biomass for the years 2010, 2017, 2018, 2019 and 2020.
Schumacher, J., Hauglin, M., Astrup, R., and Breidenbach, J. (2020). Mapping forest age using National Forest Inventory, airborne laser scanning, and Sentinel-2 data. For Ecosyst 7, 60. doi:10.1186/s40663-020-00274-9
Schwartz, M., Ciais, P., De Truchis, A., Chave, J., Ottlé, C., Vega, C., et al. (2023). FORMS: forest Multiple Source height, wood volume, and biomass maps in France at 10 to 30 m resolution based on Sentinel-1, Sentinel-2, and GEDI data with a deep learning approach. Earth Syst. Sci. Data Discuss., 1–28. doi:10.5194/essd-2023-196
Schwartz, M., Ciais, P., Ottlé, C., De Truchis, A., Vega, C., Fayad, I., et al. (2024). High-resolution canopy height map in the Landes forest (France) based on GEDI, Sentinel-1, and Sentinel-2 data with a deep learning approach. Int. J. Appl. Earth Observation Geoinformation 128, 103711. doi:10.1016/j.jag.2024.103711
Seidl, R., Thom, D., Kautz, M., Martin-Benito, D., Peltoniemi, M., Vacchiano, G., et al. (2017). Forest disturbances under climate change. Nat. Clim. Change 7, 395–402. doi:10.1038/nclimate3303
Senf, C., and Seidl, R. (2021). Mapping the forest disturbance regimes of Europe. Nat. Sustain 4, 63–70. doi:10.1038/s41893-020-00609-y
Sergent, A.-S., Rozenberg, P., and Bréda, N. (2014). Douglas-fir is vulnerable to exceptional and recurrent drought episodes and recovers less well on less fertile sites. Ann For Sci 71, 697–708. doi:10.1007/s13595-012-0220-5
Sims, A. (2022). “Remote sensing data and methods in NFI,” in Principles of national forest inventory methods: theory, practice, and examples from Estonia. Editor A. Sims (Cham: Springer International Publishing), 97–118.
Solberg, S. (2004). Summer drought: a driver for crown condition and mortality of Norway spruce in Norway. For Pathol 34, 93–104. doi:10.1111/j.1439-0329.2004.00351.x
Stone, L. L., and Skelly, J. M. (1974) Growth of two forest tree species adjacent to a periodic source of air pollution. Phytopathol. U. S. 64. doi:10.1016/j.jag.2019.101908
Swamy, S. L., Puri, S., and Singh, A. K. (2003). Growth, biomass, carbon storage and nutrient distribution in Gmelina arborea Roxb. stands on red lateritic soils in central India. Bioresour. Technol. 90, 109–126. doi:10.1016/S0960-8524(03)00120-2
Thom, D., Rammer, W., and Seidl, R. (2017). Disturbances catalyze the adaptation of forest ecosystems to changing climate conditions. Glob. Change Biol. 23, 269–282. doi:10.1111/gcb.13506
Tomppo, E., Olsson, H., Ståhl, G., Nilsson, M., Hagner, O., and Katila, M. (2008). Combining national forest inventory field plots and remote sensing data for forest databases. Remote Sens. Environ. 112, 1982–1999. doi:10.1016/j.rse.2007.03.032
UN Conference on Environment and Development (1992). Non-legally binding authoritative statement of principles for a global consensus on the management, conservation and sustainable development of all types of forests. Rio J. Braz.
Vacek, Z., Vacek, S., and Cukor, J. (2023). European forests under global climate change: review of tree growth processes, crises and management strategies. J. Environ. Manage 332, 117353. doi:10.1016/j.jenvman.2023.117353
Venier, L. A., Thompson, I. D., Fleming, R., Malcolm, J., Aubin, I., Trofymow, J., et al. (2014). Effects of natural resource development on the terrestrial biodiversity of Canadian boreal forests. Environ. Rev. 22, 457–490. doi:10.1139/er-2013-0075
Viet, H. D. X., Tymińska-Czabańska, L., and Socha, J. (2023). Modeling the effect of stand characteristics on oak volume increment in Poland using generalized additive models. Forests 14, 123. doi:10.3390/f14010123
Wang, Y., Feng, Z., and Ma, W. (2022). Analysis of tree species suitability for plantation forests in Beijing (China) using an optimal random forest algorithm. Forests 13, 820. doi:10.3390/f13060820
Watt, M. S., Dash, J. P., Bhandari, S., and Watt, P. (2015). Comparing parametric and non-parametric methods of predicting Site Index for radiata pine using combinations of data derived from environmental surfaces, satellite imagery and airborne laser scanning. For Ecol Manag 357, 1–9. doi:10.1016/j.foreco.2015.08.001
Weber, P., Bugmann, H., Pluess, A. R., Walthert, L., and Rigling, A. (2013). Drought response and changing mean sensitivity of European beech close to the dry distribution limit. Trees 27, 171–181. doi:10.1007/s00468-012-0786-4
Wernick, I. K., Ciais, P., Fridman, J., Högberg, P., Korhonen, K. T., Nordin, A., et al. (2021). Quantifying forest change in the European Union. Nature 592, E13–E14. doi:10.1038/s41586-021-03293-w
Wu, F., Jiang, Y., Zhao, S., Wen, Y., Li, W., and Kang, M. (2022). Applying space-for-time substitution to infer the growth response to climate may lead to overestimation of tree maladaptation: evidence from the North American White Spruce Network. Glob. Change Biol. 28, 5172–5184. doi:10.1111/gcb.16304
Yue, C., Kahle, H.-P., Klädtke, J., and Kohnle, U. (2023). Forest stand-by-environment interaction invalidates the use of space-for-time substitution for site index modeling under climate change. For Ecol Manag 527, 120621. doi:10.1016/j.foreco.2022.120621
Zhang, H., Wang, K., Zeng, Z., Du, H., Zou, Z., Xu, Y., et al. (2019). Large-scale patterns in forest growth rates are mainly driven by climatic variables and stand characteristics. For Ecol Manag 435, 120–127. doi:10.1016/j.foreco.2018.12.054
Zhang, L., Moore, J. A., and Newberry, J. D. (1993). A whole-stand growth and yield model for interior douglas-fir. West J Appl For 8, 120–125. doi:10.1093/wjaf/8.4.120
Keywords: satellite imagery, forest inventory, secondary tree growth, temperate forest, tree species, random forest
Citation: Pellissier-Tanon A, Ciais P, Schwartz M, Fayad I, Xu Y, Ritter F, de Truchis A and Leban J-M (2024) Combining satellite images with national forest inventory measurements for monitoring post-disturbance forest height growth. Front. Remote Sens. 5:1432577. doi: 10.3389/frsen.2024.1432577
Received: 14 May 2024; Accepted: 19 July 2024;
Published: 22 August 2024.
Edited by:
Shengli Tao, Peking University, ChinaReviewed by:
Tianyu Hu, Chinese Academy of Sciences (CAS), ChinaCopyright © 2024 Pellissier-Tanon, Ciais, Schwartz, Fayad, Xu, Ritter, de Truchis and Leban. This is an open-access article distributed under the terms of the Creative Commons Attribution License (CC BY). The use, distribution or reproduction in other forums is permitted, provided the original author(s) and the copyright owner(s) are credited and that the original publication in this journal is cited, in accordance with accepted academic practice. No use, distribution or reproduction is permitted which does not comply with these terms.
*Correspondence: Agnès Pellissier-Tanon, YWduZXMucGVsbGlzc2llci10YW5vbkBsc2NlLmlwc2wuZnI=
Disclaimer: All claims expressed in this article are solely those of the authors and do not necessarily represent those of their affiliated organizations, or those of the publisher, the editors and the reviewers. Any product that may be evaluated in this article or claim that may be made by its manufacturer is not guaranteed or endorsed by the publisher.
Research integrity at Frontiers
Learn more about the work of our research integrity team to safeguard the quality of each article we publish.