- 1Climate and Radiation Laboratory, Goddard Space Flight Center, National Aeronautics and Space Administration, Greenbelt, MD, United States
- 2Department of Earth and Environment, College of Arts and Sciences, Boston University, Boston, MA, United States
- 3University of Maryland, Baltimore County, Baltimore, MD, United States
Current operational satellite retrievals of cloud optical and microphysical properties go back to the Nakajima-King technique developed at the end of the 1980 s. This technique is based on library calculations for plane-parallel homogeneous clouds. It often works well for overcast skies but leads to substantial errors for inhomogeneous and broken cloud fields where the plane-parallel-geometry assumption is no longer valid. The basic concept of a new technique was first introduced in nuclear physics to quantify the critical conditions of reactors. Based on the eigenvalues of the radiative transfer equation, their approach provides a powerful means to parameterize the structure of 3D media. This parameterization was later successfully applied to relate surface reflectance spectra to 3D canopy structure and is known as the spectrally-invariant approximation. The proposed approach adapts this technique to the remote sensing of cloud properties such as droplet single scattering albedo and the average number of scatterings (which are the fundamental parameters in radiative transfer theory), with an emphasis on quantifying the associated errors and uncertainties. This retrieval is free from the plane-parallel homogeneous cloud assumption.
1 Introduction
Clouds cover roughly two thirds of the globe. Reflecting and absorbing solar and thermal radiation, they strongly affect the Earth’s energy (Rossow et al., 2002). The way this budget is modulated depends, among other factors, on the cloud particle size distribution. Since clouds contribute the largest uncertainty to estimates of the Earth’s changing energy budget, accurate retrievals of particle size from satellite observations is one of the most critical goals in cloud remote sensing. Indeed, cloud particle size has not only a significant influence on cloud response to aerosol modification (Várnai and Marshak, 2002; Oreopoulos and Platnick, 2008; Spencer et al., 2019), but it is also a key parameter for understanding aerosol-cloud-precipitation interactions (e.g., Albrecht, 1989; Twomey, 2007).
The current approach to simultaneously estimating cloud optical depth τ (vertical integral of cloud extinction coefficient over cloud physical thickness) and the effective particle radius reff (the ratio of the 3rd to the 2nd moment of particle size distribution) goes back to Twomey and Seton (1980). Their method was further developed and popularized by Nakajima and King (1990). Since then, this approach has been thoroughly studied in both theory and practice (see Platnick et al., 2003; Platnick et al., 2017 and references therein) and has been applied to a variety of observations, for example to Advanced Very High Resolution Radiometer (AVHRR) and Moderate Resolution Imaging Spectroradiometer (MODIS) data. The approach uses reflectances from two narrow spectral bands: one in the visible (or near-infrared) region where cloud absorption is negligible, and the other in a near-infrared region where solar radiation is slightly absorbed by cloud particles. When the two reflectance measurements are combined, both τ and reff can be determined (Nakajima and King, 1990).
The retrievals are based on library calculations for plane-parallel homogeneous clouds (Platnick et al., 2003). The underlying assumption of plane-parallel clouds and the use of a one-dimensional (1D) radiative transfer forward model can introduce substantial errors for retrievals applied to inhomogeneous cloud scenes (e.g., Loeb and Coakley, 1998; Zuidema and Evans, 1998; Stephens and Kummerow, 2007; Evans et al., 2008; Di Girolamo et al., 2010; Wolters et al., 2010). Left and middle panels in Figure 1, reproduced from Zhang and Platnick (2011), show the global monthly means of effective radii retrieved using a visible band in combination with the 2.1 μm and 3.7 μm MODIS bands, respectively.

Figure 1. Monthly mean of reff retrieved using 2.1 μm (left) and 3.7 μm (middle) Terra MODIS observations in April 2005. The enhanced values of reff for 2.1 μm are correlated with lower cloud fraction (right) and are partly due to 3D radiative effects (from Figure 9 of Zhang and Platnick, 2011).
Figure 1 shows the dramatic difference between reff retrieved from 2.1 μm to 3.7 μm. As demonstrated in Zhang and Platnick (2011), 2.1 µm is affected more by inhomogeneous cloud structure than 3.7 μm, which remains relatively more stable. The difference is well correlated with cloud fraction (CF): lower CF leads to higher values of reff being retrieved using 2.1 µm. Moreover, the error in reff retrieved from 2.1 μm strongly increases with the cloud horizontal heterogeneity index (Hσ) introduced by Liang et al. (2009). This suggests the need for physically meaningful structural variables to account for cloud heterogeneity in new retrieval techniques (e.g., Fu et al., 2019).
The use of the plane-parallel assumption in droplet effective radius retrievals can result in substantial errors in inhomogeneous or broken cloud regions. Here we propose a new and completely different retrieval approach that has the potential of filling the gap in current retrieval capabilities for inhomogeneous clouds. This technique comes from nuclear reactor physics and was developed in 1960 s (e.g., Bell and Glasstone, 1970). We propose to adapt the technique for space-based cloud remote sensing. It is not based on the 1D assumption and can be applied to any inhomogeneous and broken cloud fields. Obviously, the proposed technique has its own strengths and weaknesses that will be also addressed here.
The outline of the paper is as follows. Section 2 discusses the operationally retrieved cloud droplet effective radius and cloud optical depth and then Section 3 examines the ratio of cloud reflectance over single scattering albedo. Next, Section 4 states the two main hypotheses for interpreting satellite observations. Section 5 discusses retrievals of single scattering albedo and number of scatterings. Finally, Sections 6, 7 summarize our theoretical research questions and provide a few concluding remarks.
2 Cloud droplet effective radius and cloud optical depth
Figure 2 illustrates the relationship between the operationally retrieved values of droplet effective radius reff and cloud optical depth τ for all 29,148 data points of water clouds in a MODIS Aqua cloud product granule over the South Pacific Ocean. After considering all data points (grey dots), we selected from them the 1794 data points with the fixed value of ∼0.48 for the ratio of 0.87 μm and 2.13 μm reflectances (blue dots). Out of those, we selected 103 data points where the scattering angle between solar and viewing directions is ∼99° (red dots). We note that the values of 0.48° and 99° were chosen arbitrarily, as representative values that occur abundantly in the sample MODIS granule shown in Figure 2. As we see, the red points lie along a curve that has a roughly hyperbolic shape, suggesting that the product of reff and τ is nearly constant.
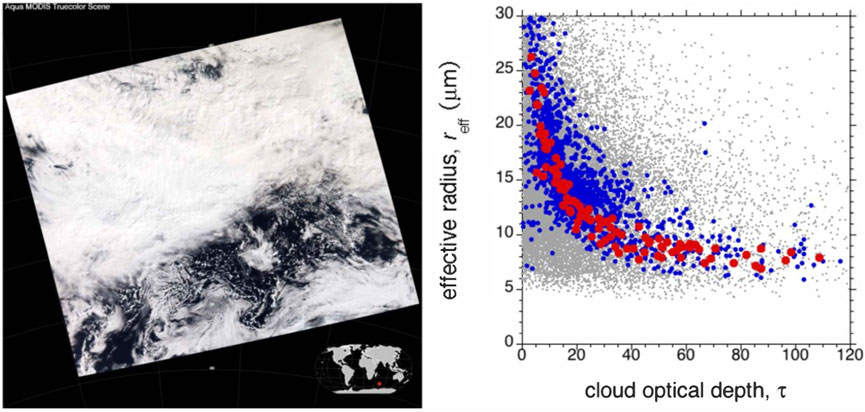
Figure 2. Left: Aqua MODIS retrieved effective radius vs. cloud optical depth for water clouds over ocean Right: The granule (MYD021KM.A2013264.0945.2013265162742) and its location showed as a red dot on a map. Grey (29,148 data points): all reff vs. τ. Blue (1794 data points): Iλ=2.13/Iλ=0.87 = 0.48 ± 0.01, all solar and viewing angles. Red (103 data points) for the fixed scattering angle of 99° ± 1°.
3 Ratio of cloud reflectance over single scattering albedo
For a sample cloudy pixel in the left panel of Figure 2, Figure 3 illustrates the ratio of the observed MODIS Top-of-Atmosphere (TOA) reflectance Iλ over the spectral single scattering albedo ω0λ (calculated based on the retrieved effective radius reff of 13 μm) plotted vs. the reflectance Iλ(Ω, Ω0) at four MODIS bands (λ = 0.86, 1.65, 2.13, and 3.75 μm). Here Ω and Ω0 are solar and viewing directions, respectively. A linear fit indicates that the relationship
holds with a slope p = 0.943 and an offset q = 0.011.
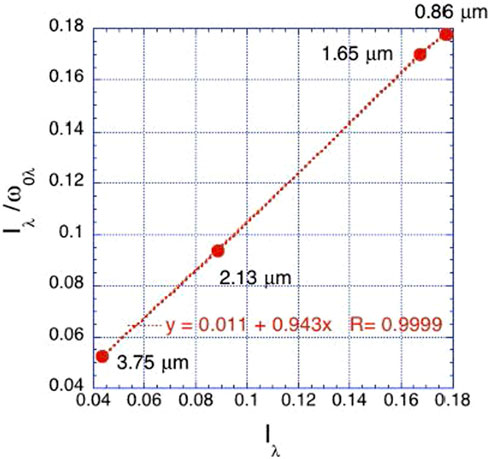
Figure 3. An example of spectral invariance in MODIS observations. MODIS retrieved reff = 13 μm. Values of spectral single scattering albedo ω0λ were calculated from Mie theory using the refwat code included in the publicly available LibRadtran software package (Mayer and Kylling, 2005; Emde et al., 2016). The refwat code is based on the results in Segelstein (1981). The ratio Iλ/ω0λ vs. Iλ is plotted for four MODIS bands: 0.86, 1.65, 2.13, and 3.75 μm. We can see that the dots follow a strong linear relationship with a slope of p = 0.943 (q = 0.011); the regression coefficient R is 0.9999.
Equation 1 can be rearranged as
Analyses of successive order of scattering suggest that Eq. 2 represents the exact solution of the 3D radiative transfer equation with non-reflecting boundaries such as a black underlying surface (Huang et al., 2007; Knyazikhin et al., 2011; Yang et al., 2017). Here p is the recollision probability, defined as the probability that a photon scattered in the medium will collide in the medium again. The variable
interactions (Marshak et al., 2011).
Indeed, in the literature of vegetation canopy remote sensing, Eq. 2 is usually written as (e.g., see Knyazikhin et al., 2013; Yang et al., 2017)
Where DASF(Ω,Ω0) = ρ(Ω)i0(Ω0)/(1
4 Two hypotheses for interpreting the observations
4.1 Hypothesis 1
This hypothesis helps interpreting Figure 2 by relating the observed behavior to cloud absorption. It reads:
All red points in Figure 2 are impacted by the same level of absorption, which can be estimated as a product of the average number of scatterings and the single scattering co-albedo averaged along all photon paths contributing to the observed reflectances.
For an “intuitive explanation,” we neglect dependency of the phase functions on wavelength. This implies that photons follow the same path (trajectory) in both absorbing and non-absorbing spectral channels. In this case, the relative effect of absorption can be estimated as the product of the (wavelength-dependent) average number of scatterings Nscat per intercepted photon and the (also wavelength-dependent) single scattering co-albedo 1-ω0:
Note that the absorption (coefficient) A = 1–Wλ, i.e. sum of absorption and scattering coefficients is equal to 1. Figure 4 schematically illustrates the above relationship on a 1-ω0 vs. Nscat plane for different level of absorption.
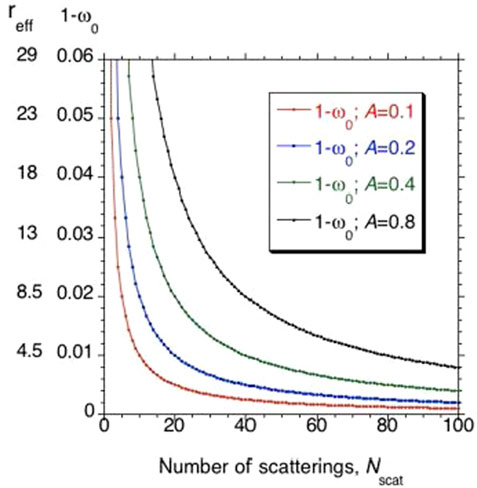
Figure 4. A plot of single scattering co-albedo vs. number of scatterings. The four lines correspond to different levels of absorption. The absorption coefficient A = 1-Iabs/Inonabs lines are calculated as the products of 1-ω0 and Nscat. For illustration the effective radius reff that corresponds to ω0 for wavelength λ = 2.13 is also indicated.
Of course, MODIS provides neither the total number of scatterings nor the single scattering co-albedo. However, cloud optical depth can be related to the number of scatterings. For example, for conservative scattering in a plane-parallel homogeneous layer, the diffusion approximation relates (see, Marshak et al., 1995; Davis and Marshak, 2002) cloud optical depth to the average number of scatterings as
and
where the tilde sign (∼) indicates proportionality. The coefficients of proportionality in Eqs 6, 7 depend on the “extrapolation length” (expressed in transport mean free paths as 1/[(1-g)σ] where g is the asymmetry factor of scattering particles (typically ≈0.85) and σ is the extinction coefficient); it is around 2 for reflected photons and 0.5 for transmitted ones (e.g., Marshak and Davis, 2005, pg. 556).
On the other hand, the single scattering co-albedo is proportional to the effective radius (Twomey and Bohren, 1980):
where κλ is the bulk absorption coefficient (4π multiplied by the ratio of the imaginary part of the refractive index to wavelength λ). As shown in Figure 5, coefficient c in front of κλ can be well approximated by 2/3 (Frank Evans, personal communication).
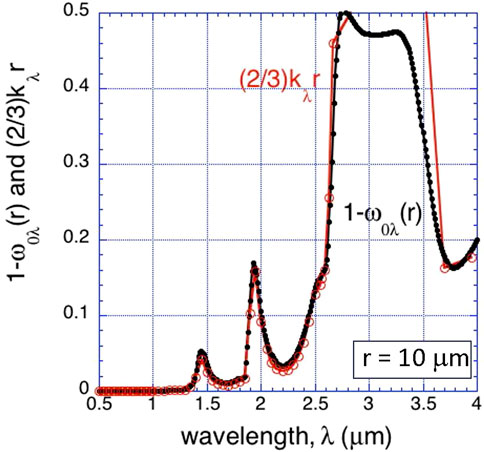
Figure 5. Single scattering co-albedo 1-ω0λ and its approximation as 2/3 κλ reff. Water droplets with reff = 10 μm are used.
Following Eqs 5–8, we can state that for a given level of absorption and solar and viewing geometry, the product of cloud optical depth τ and droplet effective radius reff can be well approximated by a constant. Thus a plot of reff vs. τ will have a hyperbolic shape as we observed in Figure 2. Naturally, dynamical/microphysical processes are important in shaping the specific (hyperbolic-shaped) reff vs. τ relationship.
4.2 Hypothesis 2
The hypothesis interprets Figure 2 as the spectrally invariant approximation. It reads:
For cloudy atmospheres, the ratio of spectral radiance over spectral single scattering albedo vs. spectral radiance is wavelength independent.
Using the ratio Iabs/Inonabs alone is not sufficient to find both ω0 and Nscat from Eq. 3. One more piece of information is needed. This can come from the spectrally invariant assumption (Knyazikhin et al., 2005; Marshak et al., 2011) expressed as Eq. 1, where Iλ and ω0λ are the wavelength-dependent radiance and single scattering albedo, while p and q are the spectrally-invariant (wavelength-independent) recollision and directional escape probabilities, respectively.
While ω0λ is a well-known parameter in atmospheric radiation, p and q are less known and thus require some explanation. They were first introduced and developed in nuclear reactor physics (Bell and Glasstone, 1970, p. 115–125). The reciprocal of the product of ω0λ and p describes the criticality condition, i.e., a situation when more than one neutron is emitted per collision (Case and Zweifel, 1967). In photon transport, p becomes the conditional probability that a scattered photon will interact with the medium again (recollision probability) and q is the conditional probability that a scattered photon will leave the medium in a given direction without further scattering (directional escape probability). The product of the recollision probability p and the single scattering albedo ω0λ approximates the maximum eigenvalue of the radiative transfer equation (see the Appendix in Marshak et al., 2011; Knyazikhin et al., 2005, pg. 634) and can be calculated as a function of reff using methods developed earlier in reactor physics.
To illustrate the validity of Eq. 1, we used the Santa Barbara DISORT Atmospheric Radiative Transfer (SBDART) code (Ricchiazzi et al., 1998). Figure 6 shows the results of SBDART calculations with a spectral resolution of 0.01 μm for a cloudy atmosphere with a cloud optical depth of 10 and an aerosol optical depth of 0.2. The three highlighted data points correspond to MODIS channels at 0.87, 1.64, and 2.13 μm.
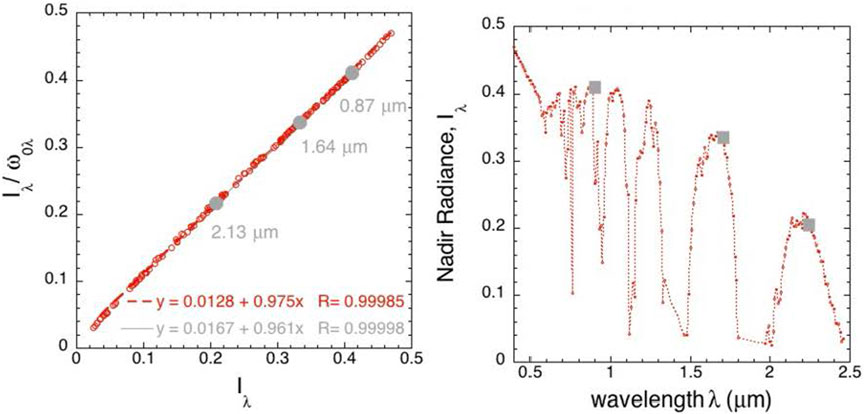
Figure 6. Illustration of spectrally-invariant approximation. (left) The ratio of spectral nadir radiance over ω0 as a function of nadir radiance. Radiances at three MODIS channels are highlighted. Slopes p for all wavelengths (red) and just for three MODIS channels: 0.87, 1.64, and 2.13 μm (grey) are provided (modified from Figure 7 of Marshak et al., 2011). (right) SBDART-calculated spectral nadir radiance between 0.4 μm and 2.4 μm, plotted at 0.01 μm resolution. Cloud properties: τcloud = 10, reff = 16 μm. Aerosol properties: rural aerosol, τaer = 0.2, relative humidity is 80%. Solar Zenith Angle (SZA) is 45°. Strong water vapor absorption bands are excluded and replaced by “doggy legs”.
In addition to the above plots, Dr. Zhibo Zhang tested the spectral invariant assumption with a Large-Eddy Simulation (LES) model (e.g., Stevens et al., 1999) and 3D radiative transfer calculations of cloud reflectance at four wavelengths (0.87, 1.64, 2.13, and 3.7 μm) and at a variety of viewing directions. Testing the validity of Eq. 1, he found a good fit by a straight line with a regression coefficient close to unity (http://userpages.umbc.edu/∼zzbatmos/research/I3RC_test_3.html).
5 Retrieval of single scattering albedo and number of scatterings
To complete our retrieval approach, we need to estimate the slope p from Eq. 1. For this, we combine radiances at MODIS non-absorbing (say, 0.87 μm) and water absorbing channels (1.64, 2.13, and 3.75 μm) using Twomey and Bohren’s (1980) approximation (4), which relates 1-ω0λ at these channels to reff as shown in Figure 5. For any given p, the number of scatterings Nscat is related to the single scattering albedo ω0 through Eq. 3 (see also Eq. 9 in Marshak et al., 2011). If we then add to Figure 4 the curve of Nscat as a function of ω0, the two independent sets of curves will intersect (see Figure 7). For each spectral reflectance Iλ of a cloudy pixel, this intersection provides the two fundamental radiative transfer parameters: the number of scatterings Nscat and the single scattering albedo ω0 averaged over all photon paths contributing to the observations. Naturally, both Nscat and ω0 are functions of wavelength λ. Note that using ω0 instead of reff will allow a more flexible interpretation of droplet size if we need to adjust the shape of the particle size distribution (whereas reff is tied to a specific distribution-shape).
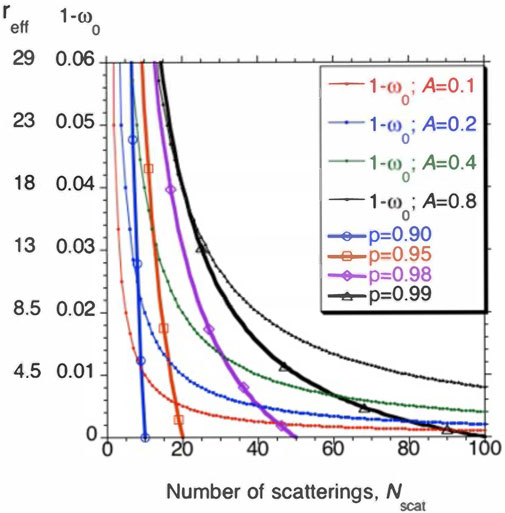
Figure 7. A plot of single scattering co-albedo, 1− ω0, vs. number of scatterings, Nscat, with curves of the recollision probability p added. The plot is the same as in Figure 4, but with four more curves from Eq. 3, corresponding to four different slopes p. The plot is for λ = 2.13 μm.
Here we briefly summarize Figure 7, which is the key plot explaining the proposed approach. For each cloudy pixel that is associated with a fixed solar-viewing geometry, the applicable thin line is selected based on the ratio as A = 1 − Iλ=2.13/Iλ=0.87 (see Eq. 5), while the applicable thick line is specified by Eq. 1 for the spectrally-invariant slope p (the recollision probability) obtained by performing a regression based on Eq. 2 to determine what p and q values work best for a set of radiances observed at multiple wavelengths at the same location, with the ω0 values at different wavelengths being related through Eq. 8.
6 Discussion and theoretical research questions
First, let us point out that the plane-parallel assumption has been neither stated nor used in the above approach. Indeed, the fundamental radiative transfer theory behind this approach is based on Eqs 1, 2, which are valid for any 3D scattering and absorbing medium (Várnai and Marshak, 2002; Várnai and Marshak, 2009; Cahalan et al., 2005; Marshak et al., 2006). Even so, both the number of scatterings Nscat and the single scattering albedo ω0 (averaged over the photon path) can be obtained for each wavelength λ (note that passive 1D reflectance-based cloud retrievals are fundamentally retrieving a 1D ω0). Our main assumption here is that the “absorbing wavelength photons” follow the same path as the non-absorbing ones. In other words, we assumed that the solution of the radiative transfer equation, Iλ, depends on {τλ, Pλ} (where Pλ is the spectral phase function) in a way that does not change with wavelength, and so the wavelength dependence of Iλ comes only from the ω0λ spectra (Marshak et al., 2012).
We note that a such separation of variables is natural for radiative transfer in leaf canopies (Knyazikhin et al., 2011), where the scattering objects are much larger than the wavelength of solar radiation—and the dependence on τ and p is determined by canopy structure (Schull et al., 2007), while the dependence on ω0 comes entirely from leaf physiology (Knyazikhin et al., 2013). For atmospheric radiative transfer this assumption is not met, since the size of scattering objects (air molecules, aerosol and cloud particles) is comparable to (or smaller than) the wavelength of solar radiation. However, in cloudy atmospheres these assumptions can be met approximately for a wide range of wavelengths (Marshak et al., 2011).
As a proof of concept, Figure 8 illustrates for several cloud optical depths the impact of neglecting the wavelength-dependence of scattering phase functions. As expected, the impact decreases as the optical depth increases: For example, for λ = 2.13 μm and τ = 5, the errors are about 8%–12%, while for τ = 40 they are reduced to 3%–8%, depending on the Solar Zenith Angle (SZA).
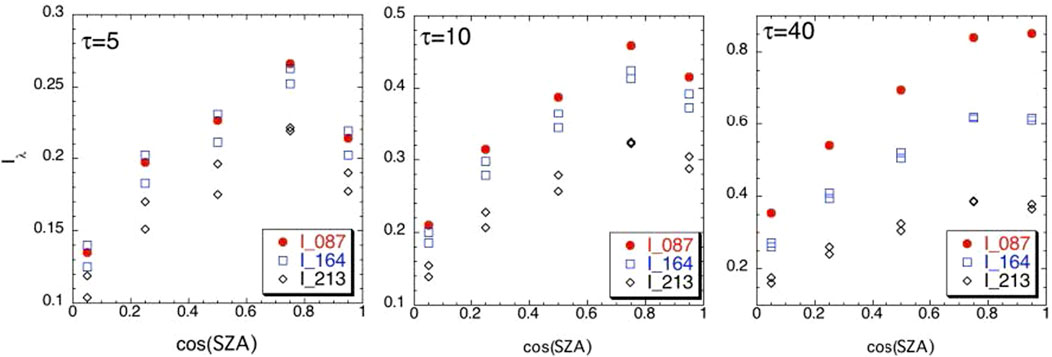
Figure 8. DISORT-calculated nadir reflectance as a function of SZA and cloud optical depth τ for 0.87, 1.64, and 2.13 μm wavelengths. For the water absorbing wavelengths (1.64 and 2.13 µm), two results are shown: one calculated using the correct phase function and the other using the 0.87 μm phase function. For each SZA, the difference between the two blue squares (1.64 μm) or between the two black diamonds (2.13 μm) shows the effect of using the 0.87 μm phase function for 1.64 and 2.13 μm. The importance of using the correct phase function decreases with increasing cloud optical depth.
Finally, we note that 1D radiative transfer theory predicts that the reflectance functionally depends on the scaled optical depth τ (1-g) (e.g., van de Hulst, 1980). Assuming that g is known a priori, the impact of phase function variations can be mitigated and the assumption of wavelength-independent phase functions can be relaxed to a certain degree. Quick preliminary calculations showed that the difference between the pair of diamond symbols for τ = 5 in the left panel of Figure 8 corresponds to a roughly 2 μm change in reff. The difference is even smaller in case of larger optical depths (middle and right panels in Figure 8). This bias can be much smaller than the one due to the assumption of plane-parallel geometry in case of strongly inhomogeneous clouds.
The above approach has been partially developed only for a black surface. However, if we know the reflectivity of the underlying surface, the surface albedo effects can be adequately removed (Stephens and Kummerow, 2007). We are familiar with the decomposition of the reflected radiation into the “black surface” problem and the additional radiative field due to the interaction between the underlying surface and the medium (Knyazikhin and Marshak, 2000; Knyazikhin et al., 2005).
While the value of retrieving ω0 (and hence reff) is clear and may warrant using the proposed approach even for just this purpose, one may wonder, what information about cloud properties does the retrieved number of scatterings convey? First of all, for non-absorbing scattering (ω0 = 1) in a plane-parallel medium, one can relate the average number of scatterings Nscat to cloud optical depth τ (and scattering asymmetry factor g) using Monte Carlo simulations or the diffusion approximation (Eqs 6, 7; see also Figure 3 in Kato and Marshak (2009), which relates Nscat to τ for different viewing zenith angles). For absorbing wavelengths (ω0 < 1), the more complex formulae also include the single scattering albedo ω0 (Platnick, 2001a; Marshak and Davis, 2005, pg. 559). Thus, under the common plane-parallel assumption, we get cloud optical depth τ and, combining τ with reff, we can also get liquid water path as done by current operational retrievals of, for example, MODIS (e.g., Platnick et al., 2003). Second, the average number of scatterings Nscat helps estimating horizontal photon transport; as horizontal transport can be expressed as a function of Nscat, ω0 and g (Platnick, 2001b). Indeed, the root-mean-square displacement of reflected and transmitted photons can be estimated from diffusion theory if the average number of scatterings is known (Platnick, 2001a; Platnick, 2001b; Marshak and Davis, 2005, pg. 560–561). We note that inconsistencies between the retrieved Nscat values and the optical depths retrieved independently using the 1D Nakajima-King approach may help identify the cases where the current operational cloud products are significantly impacted by heterogeneity effects. Finally, the average number of scatterings is closely related to the average photon path length thus it is a useful quantity in its own right. We note that the average number of scatterings obey reciprocity (Platnick, 2001b), thus all results are valid upon exchange of solar and viewing directions.
7 Summary
In this paper we propose to retrieve two fundamental radiative transfer parameters: single scattering albedo, ω0, and number of scatterings, Nscat. This retrieval does not require the assumption of plane-parallel geometry. The traditional cloud microphysical properties like optical depth, τ, and droplet size, reff, can be easily obtained from these parameters.
Eliminating the 1D assumption does not come for free here. We assume that the photons’ trajectory is the same in absorbing and non-absorbing channels. This assumption is strong and creates a bias in τ and reff. However, in many cases, the bias appears likely to be (much) smaller than the biases that the assumption of 1D geometry brings for strongly inhomogeneous clouds.
In addition, the retrieved number of scatterings and single scattering albedo convey broad information about cloud properties. For example, ω0 allows a more flexible interpretation of droplet size in cases of different shapes of particle size distribution, while Nscat allows to infer the impact of photon horizontal transport.
Data availability statement
The original contributions presented in the study are included in the article, further inquiries can be directed to the corresponding author.
Author contributions
AM: Writing–original draft, Writing–review and editing. YK: Writing–review and editing. TV: Writing–review and editing.
Funding
The author(s) declare financial support was received for the research, authorship, and/or publication of this article. This research was supported in part by the NASA Remote Sensing Theory program (through a grant awarded ensuing a solicitation in ROSES-2018).
Acknowledgments
We are grateful to Dr. Steven Platnick for helpful advice that played an important role in this study.
Conflict of interest
The authors declare that the research was conducted in the absence of any commercial or financial relationships that could be construed as a potential conflict of interest.
Publisher’s note
All claims expressed in this article are solely those of the authors and do not necessarily represent those of their affiliated organizations, or those of the publisher, the editors and the reviewers. Any product that may be evaluated in this article, or claim that may be made by its manufacturer, is not guaranteed or endorsed by the publisher.
References
Albrecht, B. A. (1989). Aerosols, cloud microphysics, and fractional cloudiness. Science 245 (4923), 1227–1230. doi:10.1126/science.245.4923.1227
Cahalan, R. F., Oreopoulos, L., Marshak, A., Evans, K. F., Davis, A. B., Pincus, R., et al. (2005). The I3RC: bringing together the most advanced radiative transfer tools for cloudy atmospheres. Bull. Am. Meteorol. Soc. 86 (9), 1275–1294. doi:10.1175/BAMS-86-9-1275
Case, K. M., and Zweifel, P. F. (1967) Linear transport theory. Reading, MA: Addison-Wesley Pub. Co, 342.
Davis, A. B., and Marshak, A. (2002). Space-time characteristics of light transmitted through dense clouds. J. Atmos. Sci. 59, 2714–2728. doi:10.1175/1520-0469(2002)059<2713:STCOLT>2.0.CO;2
Di Girolamo, L., Liang, L., and Platnick, S. (2010). A global view of one-dimensional solar radiative transfer through oceanic water clouds. Geophys. Res. Lett. 37, L18809. doi:10.1029/2010GL044094
Emde, C., Buras-Schnell, R., Kylling, A., Mayer, B., Gasteiger, J., Hamann, U., et al. (2016). The libRadtran software package for radiative transfer calculations (version 2.0.1). Geosci. Model Dev. 9, 1647–1672. doi:10.5194/gmd-9-1647-2016
Evans, K. F., Marshak, A., and Varnai, T. (2008). The potential for improved boundary layer cloud optical depth retrievals from the multiple directions of MISR. J. Atmos. Sci. 65, 3179–3196. doi:10.1175/2008jas2627.1
Fu, D., Di Girolamo, L., Liang, L., and Zhao, G. (2019). Regional biases in MODIS marine liquid water cloud drop effective radius deduced through fusion with MISR. J. Geophys. Res. Atmos. 124, 13,182–13,196. doi:10.1029/2019JD031063
Huang, D., Knyazikhin, Y., Dickinson, R. E., Rautiainen, M., Stenberg, P., Disney, M., et al. (2007). Canopy spectral invariants for remote sensing and model applications. Remote Sens. Env. 106 (1), 106–122. doi:10.1016/j.rse.2006.08.001
Kato, S., and Marshak, A. (2009). Solar zenith and viewing geometry-dependent errors in satellite retrieved cloud optical thickness: marine stratocumulus case. J. Geophys. Res. 114, D01202. doi:10.1029/2008JD010579
Knyazikhin, Yu., and Marshak, A. (2000). Mathematical aspects of BRDF modeling: adjoint problem and Green’s function. Remote Sens. Rev. 18, 263–280. doi:10.1080/02757250009532392
Knyazikhin, Y., Marshak, A., and Myneni, R. B. (2005). “3D radiative transfer in vegetation canopies,” in Three-dimensional radiative transfer in cloudy atmospheres. Editors A. Marshak, and A. B. Davis (Springer), 617–652.
Knyazikhin, Y., Schull, M. A., Stenberg, P., Mõttus, M., Rautiainen, M., Yang, Y., et al. (2013). Hyperspectral remote sensing of foliar nitrogen content. Proc. Nat. Acad. Sci. 110, E185–E192. doi:10.1073/pnas.1210196109
Knyazikhin, Y., Schull, M. A., Xu, L., Myneni, R. B., and Samanta, A. (2011). Canopy spectral invariants. Part 1: a new concept in remote sensing of vegetation. J. Quant. Spectrosc. Radiat. Transf. 112, 727–735. doi:10.1016/j.jqsrt.2010.06.014
Liang, L., Di Girolamo, L., and Platnick, S. (2009). View-angle consistency in reflectance, optical thickness and spherical albedo of marine water-clouds over the northeastern Pacific through MISR-MODIS fusion. Geophys. Res. Lett. 36, L09811. doi:10.1029/2008GL037124
Loeb, N. G., and Coakley, J. A. (1998). Inference of marine stratus cloud optical depths from satellite measurements: does 1D theory apply? J. Clim. 11, 215–233. doi:10.1175/1520-0442(1998)011<0215:iomsco>2.0.co;2
Marshak, A., Davis, A., Wiscombe, W., and Cahalan, R. (1995). Radiative smoothing in fractal clouds. J. Geophys. Res. 100, 26247–26261. doi:10.1029/95jd02895
Marshak, A., and Davis, A. B. (2005). “Horizontal fluxes and radiative smoothing,” in Three-dimensional radiative transfer in cloudy atmospheres. Editors A. Marshak, and A. B. Davis (Springer), 543–586.
Marshak, A., Knyazikhin, Y., Chiu, J. C., and Wiscombe, W. J. (2011). Spectrally-invariant approximation within atmospheric radiative transfer. J. Atmos. Sci. 68 (12), 3094–3111. doi:10.1175/jas-d-11-060.1
Marshak, A., Knyazikhin, Y., Chiu, J. C., and Wiscombe, W. J. (2012). On spectral invariance of single scattering albedo for water droplets and ice crystals at weakly absorbing wavelengths. J. Quant. Spec. Rad. Trans. 113, 715–720. doi:10.1016/j.jqsrt.2012.02.021
Marshak, A., Platnick, S., Várnai, T., Wen, G., and Cahalan, R. F. (2006). Impact of three-dimensional radiative effects on satellite retrievals of cloud droplet sizes. J. Geophys. Res. 111, D09207. doi:10.1029/2005JD006686
Mayer, B., and Kylling, A. (2005). Technical note: the libRadtran software package for radiative transfer calculations—description and examples of use. Atmos. Chem. Phys. 5, 1855–1877. doi:10.5194/acp-5-1855-2005
Nakajima, T., and King, M. D. (1990). Determination of the optical thickness and effective particle radius of clouds from reflected solar radiation measurements. Part I: theory. J. Atmos. Sci. 47 (15), 1878–1893. doi:10.1175/1520-0469(1990)047<1878:dotota>2.0.co;2
Oreopoulos, L., and Platnick, S. (2008). Radiative susceptibility of cloudy atmospheres to droplet number perturbations: 2. Global analysis from MODIS. J. Geophys. Res. 113, D14S21. doi:10.1029/2007JD009655
Platnick, S. (2001a). Approximations for horizontal photon transport in cloud remote sensing problems. J. Quant. Spectrosc. Radiat. Transf. 68 (1), 75–99. doi:10.1016/s0022-4073(00)00016-9
Platnick, S. (2001b). A superposition technique for deriving mean photon scattering statistics in plane-parallel cloudy atmospheres. J. Quant. Spectrosc. Radiat. Transf. 68 (1), 57–73. doi:10.1016/s0022-4073(00)00015-7
Platnick, S. E., King, M. D., Ackerman, S. A., Menzel, W. P., Baum, B. A., Riédi, J. C., et al. (2003). The MODIS cloud products: algorithms and examples from Terra. IEEE Trans. Geosci. Remote Sens. 41, 459–473. doi:10.1109/tgrs.2002.808301
Platnick, S. E., Meyer, K. G., King, M. D., Wind, G., Amarasinghe, N., Marchant, B., et al. (2017). The MODIS cloud optical and microphysical products: collection 6 updates and examples from Terra and Aqua. IEEE Trans. Geosci. Remote Sens. 55, 502–525. doi:10.1109/tgrs.2016.2610522
Ricchiazzi, P., Yang, S. R., Gautier, C., and Sowle, D. (1998). SBDART: a research and teaching software tool for planeparallel radiative transfer in the earth’s atmosphere. Bull. Amer. Meteor. Soc. 79, 2101–2114. doi:10.1175/1520-0477(1998)079<2101:sarats>2.0.co;2
Rossow, W. B., Delo, C., and Cairns S, B. (2002). Implications of the observed mesoscale variations of clouds for the Earth’s radiation budget. J. Clim. 15 (6), 557–585. doi:10.1175/1520-0442(2002)015<0557:iotomv>2.0.co;2
Schull, M. A., Ganguly, S., Samanta, A., Huang, D., Shabanov, N. V., Jenkins, J. P., et al. (2007). Physical interpretation of the correlation between multi-angle spectral data and canopy height. Geophys, Res. Lett. 34, L18405. doi:10.1029/2007gl031143
Segelstein, D. (1981) The complex refractive index of water. M.S. Thesis. Kansas City: University of Missouri—Kansas City.
Spencer, R. S., Levy, R. C., Remer, L. A., Mattoo, S., Hlavka, D., Arnold, G., et al. (2019). Exploring aerosols near clouds with high-spatial-resolution aircraft remote sensing during SEAC4RS. J. Geophys. Res. 124, 2148–2173. doi:10.1029/2018JD028989
Stephens, G. L., and Kummerow, C. D. (2007). The remote sensing of clouds and precipitation from space: a review. J. Atmos. Sci. 64, 3742–3765. doi:10.1175/2006jas2375.1
Stevens, B., Moeng, C.-H., and Sullivan, P. P. (1999). Large-eddy simulations of radiatively driven convection: sensitivities to the representation of small scales. J. Atmos. Sci. 56, 3963–3984. doi:10.1175/1520-0469(1999)056<3963:lesord>2.0.co;2
Twomey, S. (2007). Pollution and the planetary albedo. Atmos. Environ. 41, 120–125. doi:10.1016/j.atmosenv.2007.10.062
Twomey, S., and Bohren, C. F. (1980). Simple approximations for calculations of absorption in clouds. J. Atmos. Sci. 37, 2086–2095. doi:10.1175/1520-0469(1980)037<2086:safcoa>2.0.co;2
Twomey, S., and Seton, K. J. (1980). Inferences of gross microphysical properties of clouds from spectral reflectance measurements. J. Atmos. Sci. 37, 1065–1069. doi:10.1175/1520-0469(1980)037<1065:iogmpo>2.0.co;2
van de Hulst, H. C. (1980) Multiple light scattering: tables, formulas, and applications, 1. Academic Press, 317.
Várnai, T., and Marshak, A. (2002). Observations of three-dimensional radiative effects that influence MODIS cloud optical thickness retrievals. J. Atmos. Sci. 59, 1607–1618. doi:10.1175/1520-0469(2002)059<1607:ootdre>2.0.co;2
Várnai, T., and Marshak, A. (2009). MODIS observations of enhanced clear sky reflectance near clouds. Geophys. Res. Lett. 36, L06807. doi:10.1029/2008GL037089
Wolters, E. L. A., Deneke, H. M., van den Hurk, B. J. J. M., Meirink, J. F., and Roebeling, R. A. (2010). Broken and inhomogeneous cloud impact on satellite cloud particle effective radius and cloud-phase retrievals. J. Geophys. Res. 115, D10214. doi:10.1029/2009JD012205
Yang, B., Knyazikhin, Y., Mottus, M., Rautiainen, M., Stenberg, P., Yan, L., et al. (2017). Estimation of leaf area index and its sunlit portion from DSCOVR EPIC data: theoretical basis. Remote Sens. Env. 198, 69–84. doi:10.1016/j.rse.2017.05.033
Zhang, Z., and Platnick, S. (2011). An assessment of differences between cloud effective particle radius retrievals for marine water clouds from three MODIS spectral bands. J. Geophys. Res. 116, D20215. doi:10.1029/2011JD016216
Keywords: clouds, remote sensing, number of scatterings, single scattering albedo, spectral-invariant
Citation: Marshak A, Knyazikhin Y and Várnai T (2024) A new spectrally-invariant approach to the remote sensing of inhomogeneous clouds. Front. Remote Sens. 5:1392596. doi: 10.3389/frsen.2024.1392596
Received: 27 February 2024; Accepted: 17 April 2024;
Published: 16 May 2024.
Edited by:
Suhyb Salama, University of Twente, NetherlandsReviewed by:
Yevgeny Derimian, Univ. of Lille/CNRS, FranceWeizhen Hou, Harvard University, United States
Copyright © 2024 Marshak, Knyazikhin and Várnai. This is an open-access article distributed under the terms of the Creative Commons Attribution License (CC BY). The use, distribution or reproduction in other forums is permitted, provided the original author(s) and the copyright owner(s) are credited and that the original publication in this journal is cited, in accordance with accepted academic practice. No use, distribution or reproduction is permitted which does not comply with these terms.
*Correspondence: Alexander Marshak, alexander.marshak@nasa.gov