- 1Satellite Services and Research Group, Arctic Space Center, Space and Earth Observation Department, Finnish Meteorological Institute, Helsinki, Finland
- 2Metsäteho Oy, Vantaa, Finland
Soil wetness forecasts on a local level are needed to ensure sustainable forestry operations during summer when the soil is neither frozen nor covered with snow. Training gradient boosting models has been successful in predicting satellite observation-based products into the future using Numerical Weather Prediction (NWP) and Earth Observation (EO) climate data as inputs. The Copernicus Global Land Monitoring Service’s Soil Water Index (SWI) satellite-based observations from 2015 to 2023 at 10,000 locations in Europe were used as the predictand (target parameter) to train an artificial intelligence (AI) model to predict soil wetness with XGBoost (eXtreme Gradient Boosting) and LightGBM (Light Gradient Boosting Machine) implementations of gradient boosting algorithms. The locations were selected as a representative set of points from the Land Use/Cover Area Frame Survey (LUCAS) sites, which helped evaluate the characteristics of distinct locations used in fitting to represent diverse landscapes across Europe. Over 40 predictors, mainly from ERA5-Land reanalysis, were used in the final model. Over 70 predictors were tested, including the climatology of EO based predictors like SWI and Leaf-Area Index (LAI). The final model achieved a mean absolute error of 5.5% and a root mean square error of 7% for variable values ranging from 0% to 100%, an accuracy sufficient for forestry use case. To further validate the model, SWI prediction was made using the 215-day seasonal forecast ensemble from April 2021, consisting of 51 members. With this, the quality could also be demonstrated in the way our forestry climate service (HarvesterSeasons.com) would use the forecasts. As soil wetness is not changing as rapidly as many weather parameters, the forecast skill appears to last longer for it than for the weather variables. The technology demonstration and machine learning work were conducted as a part of the HarvesterDestinE project, supported by European Union Destination Earth funding managed by the European Center for Medium-Range Weather Forecasts (ECMWF) contract DE_370d_FMI. The authors wish to acknowledge CSC – IT Center for Science, Finland, for computational resources. The code for the machine learning work and the predictions are available as open source at https://github.com/fmidev/ml-harvesterseasons (see README-SWI2). The training data and ML models are at https://destine.data.lit.fmi.fi/soilwater/. All data used for predictions are accessible from the SmartMet server at https://desm.harvesterseasons.com/grid-gui and the work flow is available in the script https://github.com/fmidev/harvesterseasons-smartmet/blob/master/bin/get-seasonal.sh Everything is made available for ensuring reproducibility. One will need to register and use their own https://cds.climate.copernicus.eu credentials for doing so.
Introduction
Forest harvesting is nowadays a year-round activity in the bioeconomy value chain. It is both affordable and environmentally sustainable on frozen soil or deep snow, conditions that are constantly diminishing due to climate change (Lehtonen et al., 2019). This creates pressure to perform harvesting more during the summer when the soil is unfrozen. Forest soil can endure forestry operations when conditions are dry enough, avoiding disturbances to the soil that could make the land less productive. Sustainable forestry requires a more efficient use of optimal conditions for forest lands that need to be harvested in winter and good predictions for sustainable summer conditions. Harvesting and forwarding timber to roadsides typically takes only a few days per site but transporting 20-ton machines to the site and onwards requires longer planning horizons to ensure cost efficiency. Currently, decisions are often made a week in advance but with better long-term forecasts, longer planning will become more common. A combination of seasonal predictions months ahead and 10-day weather forecasts is optimal for forestry operations planning.
The trafficability (i.e., vehicle bearing capacity) of forest soil for harvesting during summer conditions is driven by the amount of water present in the soil. It would be particularly important to know the soil moisture down to a depth of 30 cm, as this has the most significant effect on the soil’s bearing capacity when considering forestry operations. The soil wetness product used in the HarvesterSeasons service since 2020 has been deemed, based on user feedback, too static and model soil-type dependent to capture changes in soil wetness caused by weather events. It also tends to underestimate systematic changes associated with seasonal transitions. As a result, the service has provided, e.g., unrealistically optimistic long-term predictions about terrain trafficability during a rainy fall period. The current service relied purely on the ECMWF Integrated Forecast System (IFS), meaning both the forecasts and reanalysis data, which provided bias adjustment and downscaling, represented the same model. Without independent observations, model errors remain undetected. Therefore, updating the base information with the satellite-based product Soil Water Index (SWI) should improve many aspects, enabling also a more meaningful verification of forecasts. Although the SWI product combines data from two different satellite instruments, its 1 km resolution makes it the best available product for this study. SWI data has been available daily since 2015 (with two Sentinel-1 satellites until end of 2021), providing sufficient training data for a machine learning (ML) exercise.
The aim of this study is to use machine learning to predict new seasonal and weather forecast based soil wetness SWI forecast products, using the satellite-based observation product SWI as the target parameter (predictand) in fitting. The strength of our service is that the end user can themselves compare how well the SWI2 forecasts matched observations in the past weeks. This has proven to be a successful approach in weather forecasts, convincing the end users of the services’ usefulness.
For machine learning, we use gradient boosting methods and their implementations in Python. For tabular data fitting, gradient boosting has won most of the machine learning competitions in recent years. The information skill for higher resolution stems from using 1 km or finer land cover and orography predictors, along with high-resolution data from Earth Observation satellites for fitting the model and predicting with it. Higher resolution Extreme Digital Twin predictions will improve the weather-forecast time window of the product. For seasonal forecasts at coarser resolution the forecast ensemble allows us to assess probabilities of future predictions. Machine learning (ML) downscaling incorporates sophisticated climatology-based bias adjustment and distinguishing the local distribution of wetness, but it is not forecasting weather, which does have the greatest impact on changing conditions. One needs to combine ML with numerical weather and seasonal predictions for optimal information about the future on a local scale.
This study presents the new soil wetness ML prediction product. The following sections describe the data and methods used to develop the soil wetness model, including complete tables of location data, ML training input data sets, and ML prediction input/output datasets in Supplementary Material S1–S3. Additionally, the codes used both in training and prediction are detailed. Finally, we present the results of soil wetness model training and prediction for the forestry service. The discussion and conclusions address both the forecasting skill of the resulting product and the technology readiness for training and deploying ML models.
Data used for machine learning
In machine learning, much of a model’s quality and capabilities depend on the data used for training. The predictand must have a representative time series in both spatial and temporal extent, and all predictors need to be available for most data points. In our case, using an EO predictand reduces some capability as SWI data is not available in all seasons due to snow cover and is unavailable in mountainous regions, where observation retrieval is difficult. The quality of improvements achieved in predicting soil wetness will need to outweigh the limitations introduced by using a restricted predictand.
Soil water index ML target variable (predictand)
The Soil Water Index is a Copernicus Land Monitoring Service (CLMS) product from the global land services. It is based on a combination of the Sentinel-1 surface soil moisture product from CLMS and the EUMETSAT Hydrological Satellite Application Facility (H-SAF) Metop mission ASCAT surface soil moisture product. -Europe in a 1 km grid resolution, with data available daily over several years, suitable for ML applications. At 12.5 km resolution the product is also available globally, based only on ASCAT data. The product adds to the 0–5 cm surface soil moistures observed from satellites a two-layer water balance model developed by Wagner et al. (1999) which, put simply, relates deeper layer soil wetness to an accumulation of surface conditions over several days. For a complete description of the product, refer to Bauer-Marschallinger et al. (2018).
The SWI describes soil wetness within a 0%–100% range and includes quality flags for eight soil depths based on the number of days of accumulated surface moisture. For the use of SWI in relation to the IFS model soil moisture variables, the SWI T5 is considered to match with IFS soil layer 1 (0–7 cm), T15 matches with soil layer 2 (7–28 cm), T60 with soil layer 3 (28–100 cm) and T100 to layer 4 (100–255 cm). We refer to these here as SWI1, SWI2, SWI3 and SWI4, respectively. SWI is more detailed locally and in its range than the volumetric soil water layer variable, which the IFS model is forecasting, and which was our initial summer condition indicator.
For the soil wetness ML model, the SWI product at soil layer 2 (SWI2) at 1 km resolution over Europe is used as the machine learning target variable (predictand). This enables the prediction of a superior downscaled soil wetness product based on seasonal and weather forecasts. The daily data were acquired from the Copernicus Global Land Service (land.copernicus.eu) and set up to the Destination Earth SmartMet-server.
Locations for training
Our EO predictand and the EO or reanalysis model predictors are available as gridded data covering nearly all of Europe, with some grids containing even several million points. Training on all grid points is not required to achieve a good-quality model. For a sufficiently representative dataset to fit the model, we utilize points from the LUCAS survey (d’Andrimont et al., 2020), totaling 63,287 points with extended land cover analysis and site photos available in Europe (Figure 1). To compute a model reasonably fast, we selected a representative subset of 10,000 points to cover Europe’s various land types (Supplementary Material S1) and ensure a well-distributed sample for training (Figure 2).
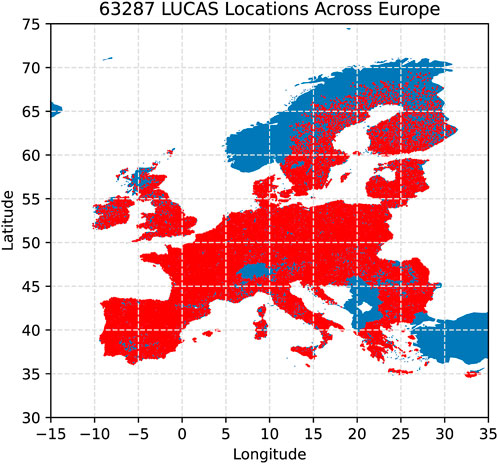
Figure 1. Distribution of the 63,287 LUCAS point locations over Europe. Red points represent LUCAS locations, while the blue area represents the base map of Europe, showing that the LUCAS is not available in all countries. Kosovo and Bosnia-Herzegovina are unfortunately white.
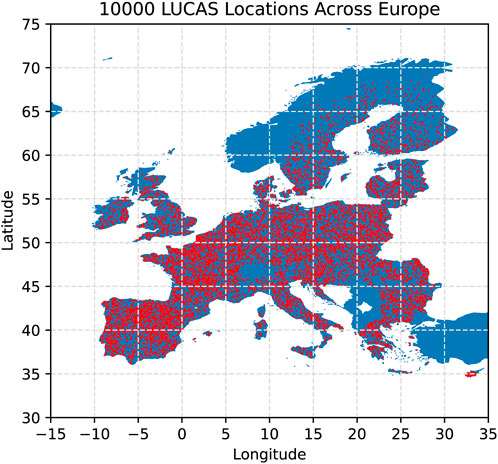
Figure 2. The subset of 10,000 LUCAS points over Europe for training the soil wetness model. Red points represent LUCAS locations used, while the blue area represents the base map of Europe. See land cover classes representation in Supplementary Material S1 for information on how well this subset matches the complete set of locations.
Predictors for fitting the model
The predictors used in our model training include terrain statistics from the Copernicus Digital Elevation Model (DEM) (European Space Agency, 2020), SoilGrids 2.0 (Poggio et al., 2021) soil composition data, IFS model parameters for terrain statistics, and weather information from the ERA5-Land reanalysis (Muñoz Sabater, 2019), and corresponding seasonal forecast variables and EO parameters for predicting soil wetness. For some ERA5-Land parameters used as predictors, such as total precipitation, rolling cumulative daily sums over 5-day, 15-day, 60-day and 100-day intervals were calculated, like the surface moisture accumulation method used in the SWI products, which integrates satellite observations across comparable time windows. Since ERA5-Land also has the IFS model as its engine, training with it enables compatibility with Digital Twin Engine (DTE) and ECMWF seasonal forecast outputs. Additionally, an SWI climatology predictor was created by averaging daily SWI values for each day of the year based on 2015–2023 observations. In total, we tested 107 parameters of which 47 were eventually chosen as predictors for the final model to predict SWI2. Detailed tables of the predictand and predictors datasets, including data sources and links, are provided in Supplementary Material S2.
Time series data for ML training, covering the period 2015–2022 for all 63,287 LUCAS locations, were retrieved from the Destination Earth SmartMet-server using its Timeseries API. Most datasets have been ingested to the server, data and metadata browsing is available via Smartmet-server grid-gui interface (https://desm.harvesterseasons.com/grid-gui) and downloading through the new OGC Environmental Data Retrieval (EDR) interface (https://desm.harvesterseasons.com/edr/collections). The time series query replaces some of the missing values in the SWI target parameter using linear interpolation with the two nearest values within a four-day time interval. The processed fitting data are stored as CSV files on our development server, ml-harvesterseasons, where ML training was conducted.
Machine learning methods and training process
Gradient boosting
Gradient Boosting is an ensemble learning method that combines predictions from multiple weak learners (decision trees) that form a boosted ensemble, for a stronger final mean (median) prediction model from all trees. It samples both time and predictor dimensions of the fitting data randomly and fits trees for each random sample one by one such that each new tree minimizes the prediction error of the previous tree. For all the ML models developed within HarvesterDestinE, we apply the extreme gradient boosting method through two widely-used Python implementations: XGBoost (Chen and Guestrin, 2016) and LightGBM (Ke et al., 2017). XGBoost was for several years the most probable winner in machine learning competitions, but last year LightGBM outperformed it in some contests. We tested both methods to find the best fit.
Hyperparameter tuning and validation metrics
To find the optimized hyperparameters for each ML model, we used an automatic hyperparameter optimization software Optuna (Akiba et al., 2019). Its Optuna Dashboard also offers a convenient way to store the optimization history with all fitting experiments and get more information about the related hyperparameters. K-fold cross-validation is used to find the optimal teaching/validation data split from the training time series by sampling years. Model performance is assessed through root mean square error (RMSE) and mean absolute error (MAE) as measures of model accuracy.
Feature selection and predictor importance
The cross correlation (Pearson correlation) matrix between all 108 parameters (predictand and predictors) was computed with Scikit-Learn (Buitinck et al., 2013) feature selection module’s r_regression class. Predictor importance was evaluated with readily available XGBoost/LightGBM package metrics, such as gain (XGBoost’s F-score) which shows each predictor’s relative contribution to the trained model, and LightGBM’s plot_importance. Both methods were utilized for optimized training set feature selection by eliminating low-importance predictors and selecting only the most relevant predictors from the highly cross-correlated ones. For instance, as the 2 m temperatures at 00 and 12 UTC were highly correlated, the 12 UTC 2 m temperature was dropped from the training set. Using an optimized subset of predictors both improves the model skill and decreases the fitting execution time as well as yields a smaller model file with lesser memory needs.
Machine learning prediction data and validation methods
All results and trained models are stored on our ml-server. The final model is used to predict a downscaled target variable from seasonal and Destination Earth (DestinE) Extremes Digital Twin forecast data as new predictions become available. Currently, once a month a new seasonal forecast (Johnson et al., 2019) and daily an ECMWF Extremes Digital Twin (EDTE) prediction (Randriamampianina, 2023) is automatically processed and uploaded to the SmartMet-server, to be used in our service. This procedure will also be implemented for the DestinE climate adaptation digital twin. Input data specifics for prediction with ECMWF seasonal forecast (51 members, 50 perturbed forecasts plus a control forecast) are detailed in Supplementary Material S3. The same parameters are used also for the DestinE extremes predictions as the IFS model is close to the same version and the same parameters are available from both. In addition to the ML forecasts, all the input data is available from our SmartMet-server as well. Links to the seasonal forecast variables are in Supplementary Material S3, for the extremes forecast variables and our AI product from this source are available from the EDTE producer.
For the extremes forecast, we only have one prediction and no other model to compare to. Validation is presented therefore for two ensemble systems. It is also more fitting for analyzing the quality of a climate service that is based on probability to exceed a threshold to use ensembles. To validate the results and study the skill of the ensemble forecasts, the continuous ranked probability score (CRPS; Hersbach, 2000) is measured for the XGBoost downscaled ECXSF SWI2 seasonal forecast product and, to compare to our previous product, the ERA5-Land statistically bias-adjusted ECBSF Volumetric soil water layer 2 product. CRPS evaluates forecast accuracy by measuring the difference between the forecast and the reference cumulative distribution functions (CDFs). For deterministic forecasts, the CRPS can be interpreted as mean absolute error (MAE). The reference can be climatology, observation, or reanalysis. The CRPS can be decomposed into reliability and resolution/uncertainty parts, see Equations 1, 2 below. We use the Climate Data Operators (CDO; Schulzweida, 2023) enscrps operator to determine CRPS, CRPS potential (resolution/uncertainty), and CRPS reliability. These are calculated over Finland, Germany, Poland, Spain, France, Romania, Sweden, and for the full European domain. According to Hersbach, reliability measures the statistical properties of the forecast system and “the resolution/uncertainty part can be related to the average spread within the ensemble and the behavior of its outliers.”
Code description
Our machine learning application requires two sets of scripts: one for training and another for predictions. The training phase includes tools for preparing tabular data files needed for training, as well as scripts for XGBoost and LightGBM fitting to test both methods. These scripts are available in the Finnish Meteorological Institute’s (FMI) Github repository ml-harvesterseasons. The other scripts are needed for preparing the input data sets for prediction and the production scripts. These are part of the FMI Github repository harvesterseasons-smartmet, which contains the entire backend system supporting the harvesterseasons.com service, including data fetching, reformatting for ingestion, and production of value-added datasets including the new ML prediction workflows.
To prepare training data, we have several Python get-timeseries scripts that use the requests module to make HTTP requests to our SmartMet-server Timeseries API. These scripts retrieve for all LUCAS locations timeseries from ERA5-Land, SWI and climatology for SWI, and leaf area index (LAI) for each day of year from 2015 to 2022. Additionally, static variables such as different land covers or inland-water fractions must be formatted as timeseries. All these predictors and the predicant SWI are described in Supplementary Material S2. The timeseries API allows querying thousands of locations in one request for our time window. For optimal efficiency, we found that querying 5000 locations per request worked best within the SmartMet-server’s memory limit. Larger requests extend the responses from the server more than asking the 63,000 locations across 13 sequential queries.
For training, the script xgb-fit-optuna-swi2.py conducts Optuna hyperparameter tuning, and xgb-fit-swi2.py reruns the training using the best hyperparameter settings. Equivalent scripts for LightGBM are prefixed lgbm-fit-. These scripts are remarkably like each other since both XGBoost and LightGBM integrate with scikit-learn. They use the same input training file and differ mainly in function calls and hyperparameter attributes. Additional scripts cover the K-Fold analysis, cross-correlation, and scripts to plot figures for the location maps and feature importance.
SWI2 prediction generation involves two steps. The first step is integrated into get-seasonal.sh and get-edte.sh bash scripts. Here, all input data is regirded to match the output grid specifications: for seasonal forecasts, the ERA5-Land grid for Europe (from −30° W to 50° E and 25° N to 75° N at 0.1° increments) is used; for EDTE, a 0.04° grid within the same bounds is required. This step relies heavily on GNU parallel (Tange, 2018) and Climate Data Operators (CDO) (Schulzweida, 2023).
The second step is carried out in our self-developed xgb-predict-swi2-era5l.py and -edte.py scripts. These python scripts use xarray (Hoyer and Hamman, 2017) to merge the different input grids into a single data frame that includes all time steps for each input within the target grid. This data frame is then used by XGBoost to calculate the grid with the predicted SWI2 values.
Machine learning results
More than 30 model optimization runs for Soil Water Index level 2 (SWI2) as the target parameter were performed using subsets of 200 to 42,000 LUCAS points with XGBoost, LightGBM, or Optuna for both methods. Each Optuna study was run for 100 trials, meaning up to 100 models were trained within each study to find the best hyperparameters. For different training runs, the predictors, number of training locations (LUCAS), and/or hyperparameters were varied to assess their impact on model skill. The final XGBoost ML SWI2 model used time series data from 10,000 LUCAS locations (Supplementary Material S2) and reached MAE 5.5%. The details and results for the model are presented here, with input data specifications provided in Supplementary Material S2. K-fold cross validation showed that splitting the time series data (from 2015 to 2022) using 2019 and 2021 as validation years and the remaining years for training produced the best results.
Hyperparameter tuning, feature engineering, and validation results
Figures 3, 4 display the Optuna Dashboard views for hyperparameter optimization (with up to 100 study trials) for XGBoost and LightGBM, showing the best trial RMSEs. Table 1 presents the best parameters from Optuna hyperparameter optimization for both XGBoost and LightGBM, and evaluation metrics for model fitting with tuned parameters. XGBoost reached RMSE 7.04% (MAE 5.5%) and LightGBM RMSE 7.03% (MAE 5.5%). Although LightGBM ML training is faster, it tends toward overfitting. Therefore, given the similar validation results, we chose to use XGBoost.
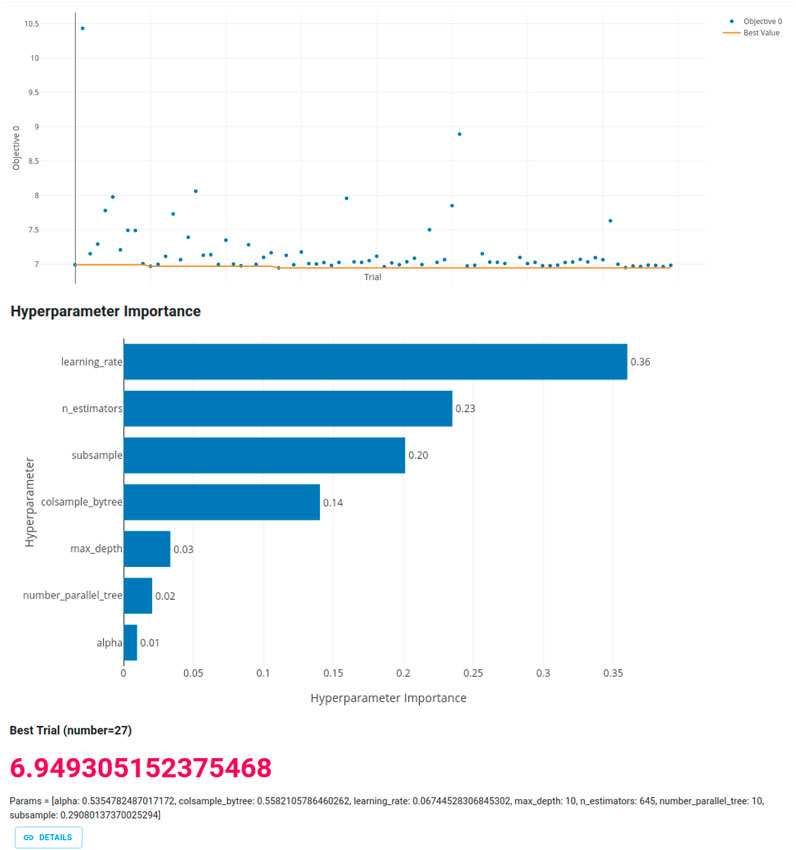
Figure 3. Optuna Dashboard view of hyperparameter optimization for XGBoost with best trial values. Upper: trials and their objectives (minimized RMSE). Middle: hyperparameter importance. Lower: best trial and its tuned hyperparameters. Optuna-run was stopped at trial number 80 for results were not improving significantly.
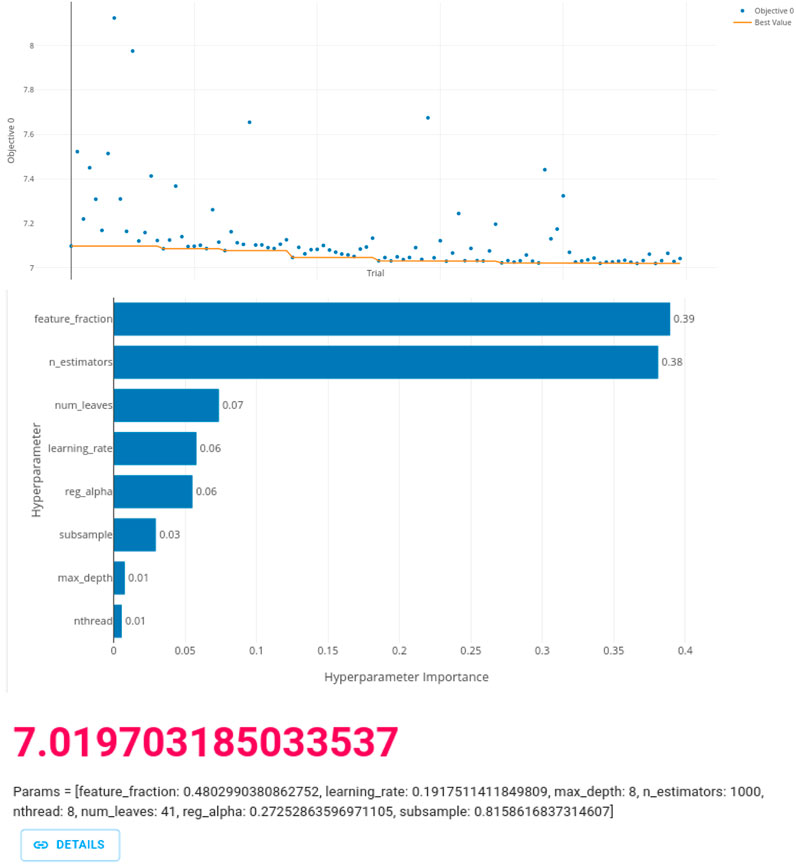
Figure 4. Optuna Dashboard view of hyperparameter optimization for LightGBM with best trial values. Similar views as Figure 3.
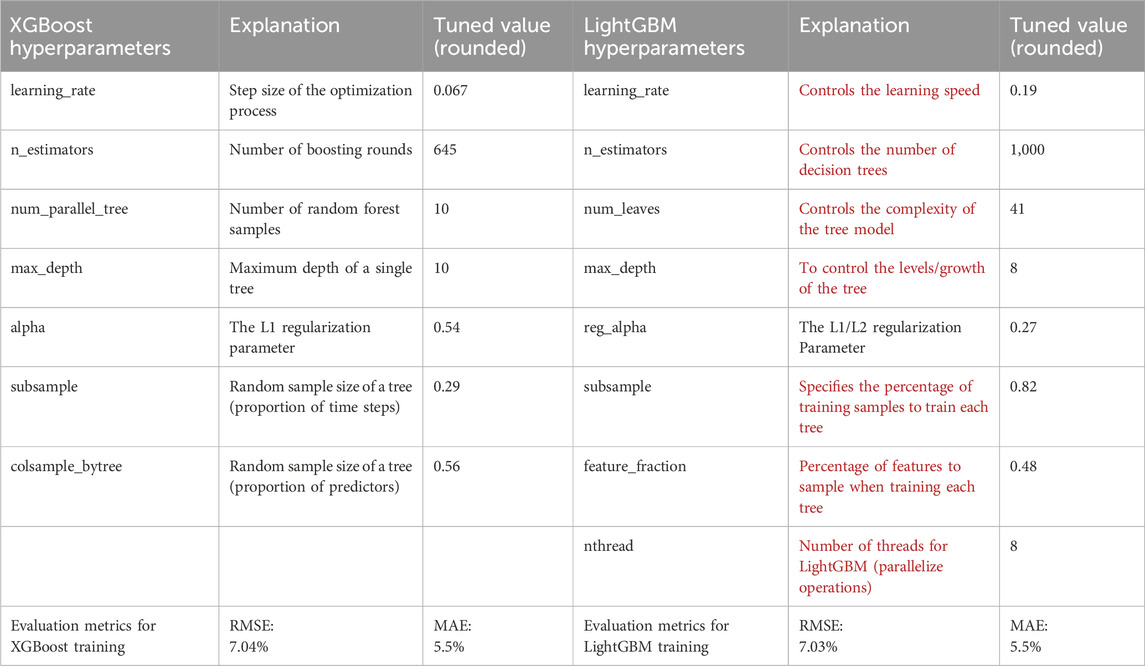
Table 1. Optuna hyperparameter optimization best parameters (trials = 100) for XGBoost and LightGBM, and validation metrics (RMSE, MAE) for fitting an XGBoost/LightGBM model with tuned parameters.
Feature selection and predictor importance
The cross-correlation matrix for all 108 parameters (predictand and predictors) is shown in Figure 5, while Figure 6 highlights the correlation between the predictand (swi2) and each of the 107 predictors (17 EO derivatives, while rest are reanalysis/IFS variables). From Figure 5 we see that there are highly correlated parameters in the training set. To optimize the model, we dropped half of the predictors, leaving only the most relevant, and avoiding using many of the highly cross-correlated ones. For instance, Volumetric Soil Water Layers 1–4 at 00 and 12 UTC (swvl1-4) are highly correlated, and we ended up using swvl2-00 as predictor as it corresponds to soil moisture at similar depth to SWI2 (7–28 cm). Similarly, for soil grids predictors, we selected parameters at 0–30 cm depths for the training set. From the running cumulative daily sum predictors, only the 15-day sums were used, as the satellite-based observation product SWI2 predictand is produced combining satellite surface soil moisture observations over this time window.
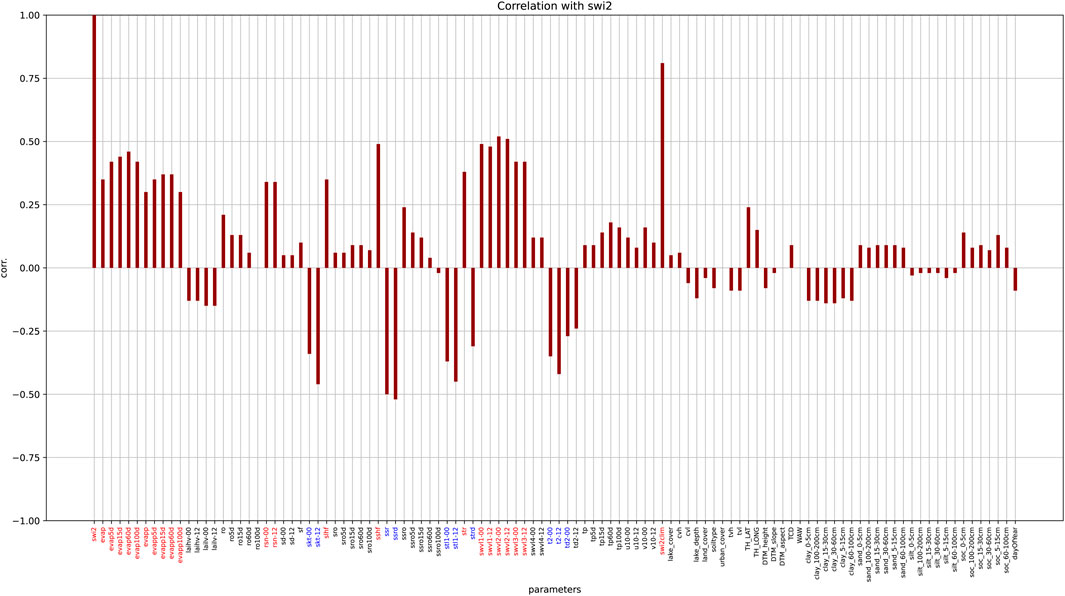
Figure 6. Correlation between swi2 and each predictor. Predictors with correlation > |0.25| highlighted with red/blue.
Figure 7 illustrates the F-score (gain) of the XGBoost model, indicating predictor importance based on usage frequency and tree placement. Predictors with the lowest F-scores from previous model runs (e.g., wind variables) were removed, resulting in a final model with 47 predictors (Supplementary Material S2). The Soil Wetness Index layer 2 climate (swi2clim) was the most important predictor, underscoring the effect of local conditions that are not captured by ERA5-Land data. Swi2clim also showed the highest correlation with the SWI2 target (see Figure 6). Volumetric Soil Water Layer 2 (swvl2-00) was the second most important predictor, both highest ranked predictors affirming model’s focus on relevant soil moisture dynamics. These were followed by Soil type (affects the amount of water in the soil), surface solar radiation downwards, day of year, and latitude as additional influential predictors. In comparison, Figure 8 shows the LightGBM model’s importance plot, where day of year, latitude, longitude, and runoff ranked the highest, only then followed by swvl2-00 and swi2clim.
ML prediction results
XGBoost downscaling is performed for SWI2 across Europe as the new ECXSF product for seasonal forecasts and set up to the SmartMet-server, and as a part of the EDTE products for the extremes digital twin predictions. Seasonal forecast production workflow is performed in 9 km resolution (best for comparing to the ERA5-Land statistically bias-adjusted product). The ML prediction input data set must have comparable parameters with the ML training input data set, detailed in Supplementary Material S3 alongside output product details. Figure 9 displays the SmartMet-server grid-gui view for the seasonal forecast ECXSF SWI2 product for September 2023, providing daily data for over 200 days ahead (approximately 6 months).
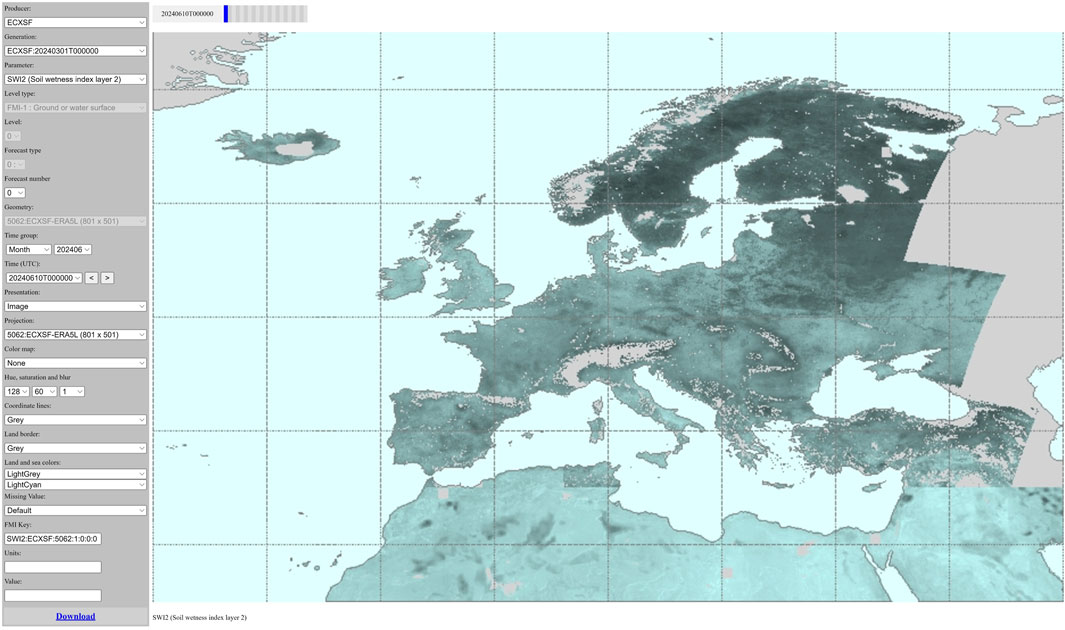
Figure 9. Seasonal forecast ECXSF SWI2 product displayed in SmartMet-server grid-gui plugin for HarvesterSeasons DestinE. Link for a better view here.
To further validate our results, we tested forecasts against data that was not used in training. Specifically, we ran the prediction model for April 2021 ECMWF seasonal forecast (SEAS5; Johnson et al., 2019) for downscaling the AI ECXSF SWI2, and compared it to the ERA5-Land climatologically bias adjusted seasonal forecast ECBSF Volumetric soil moisture (swvl2) product. Figure 10 shows the CRPS, CRPS Reliability, and CRPS Potential for ECXSF SWI2 seasonal forecast compared to SWI2 observations, remapped to a 9 km grid from the original 1 km resolution for a fair comparison. We applied a distance-weighted area fraction method from the Climate Data Operators (CDO) software (Schulzweida, 2023). Figure 11 shows the CRPS and its decomposition for the ECXSF SWI2 seasonal forecast against SWI2 climate remapped to the 9 km resolution, while Figure 12 presents the CRPS for the ECBSF SWVL2 seasonal forecast compared to ERA5-Land SWVL2 reanalysis. In these figures, the x-axis represents time from 2021-04-10 to 2021-10-27 the graph colors are red for Germany, bright green for Spain, blue for Finland, yellow for France, light blue for Poland, pink for Romania, green for Sweden, black for Europe as a whole.
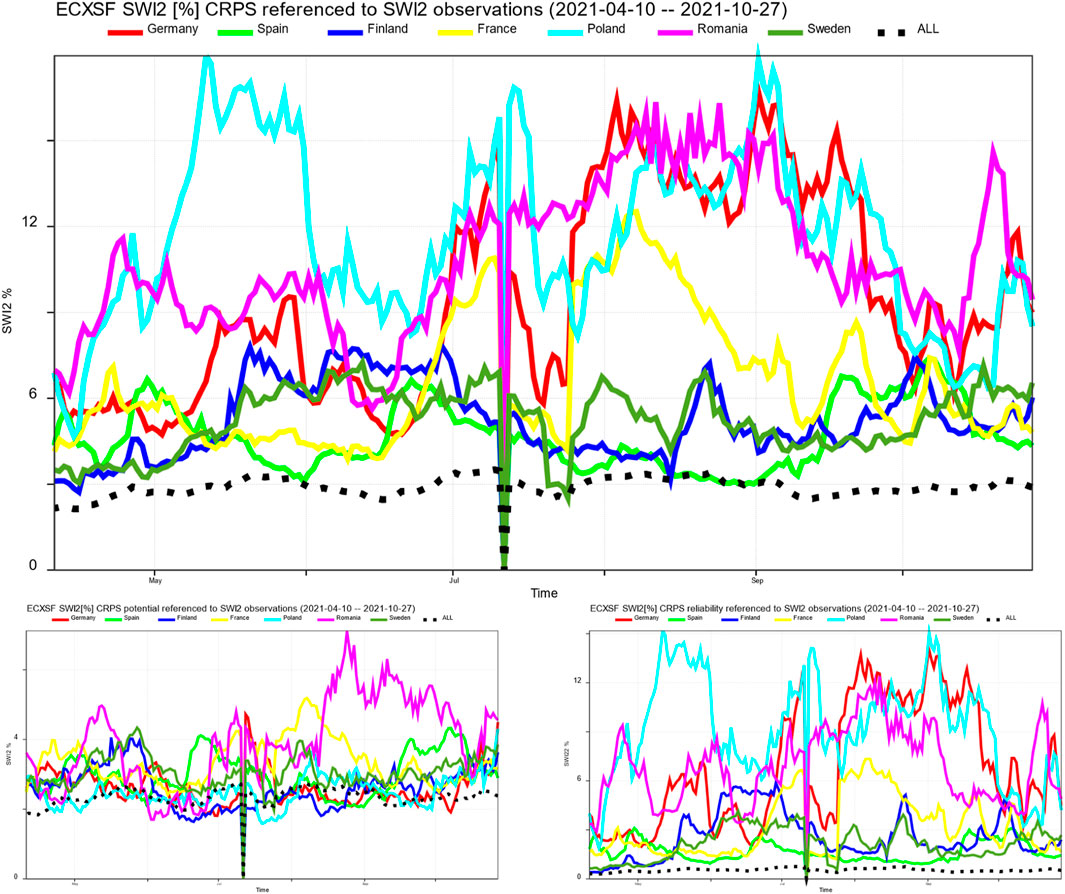
Figure 10. ECXSF SWI2 against SWI2 observations as reference with decomposition. Top: CRPS. Bottom left: Potential (resolution/uncertainty). Bottom right: Reliability. Units %.
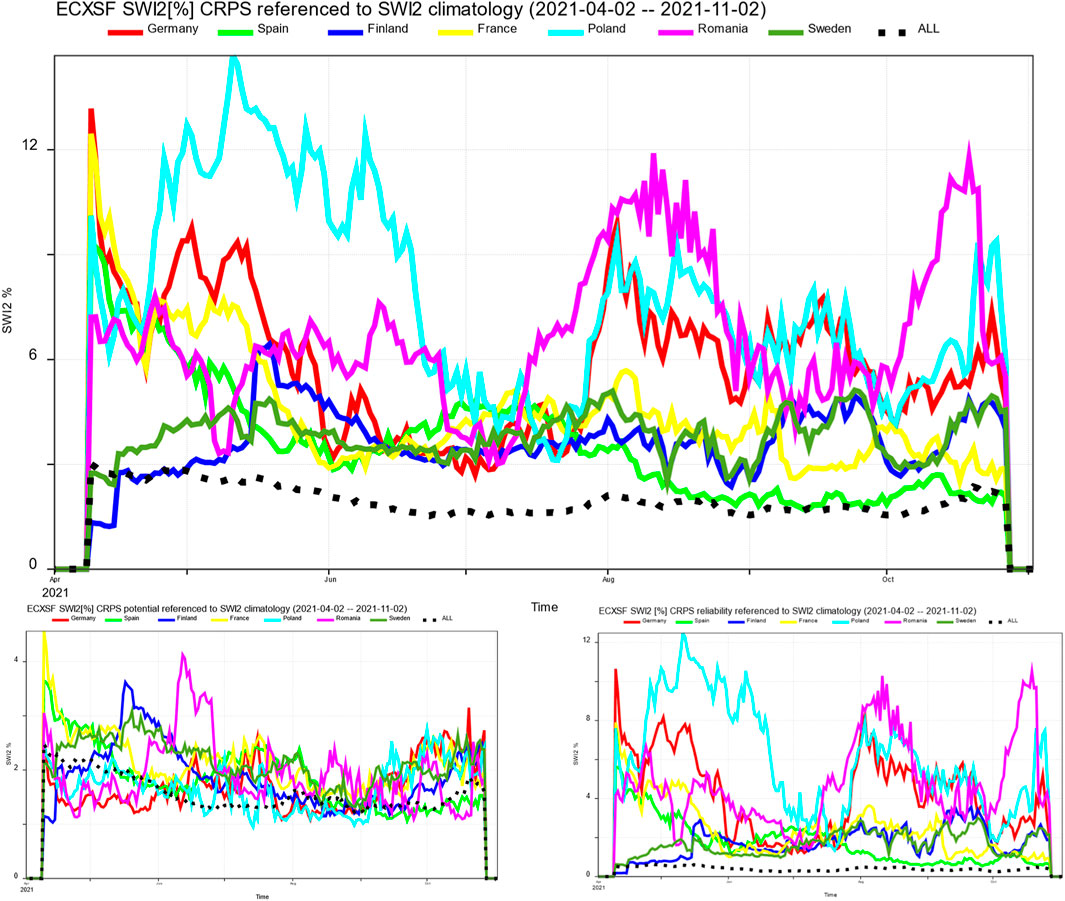
Figure 11. ECXSF SWI2 against SWI2 climate as reference with decomposition. Top: CRPS. Bottom left: Potential (resolution/uncertainty). Bottom right: Reliability-. Units %.
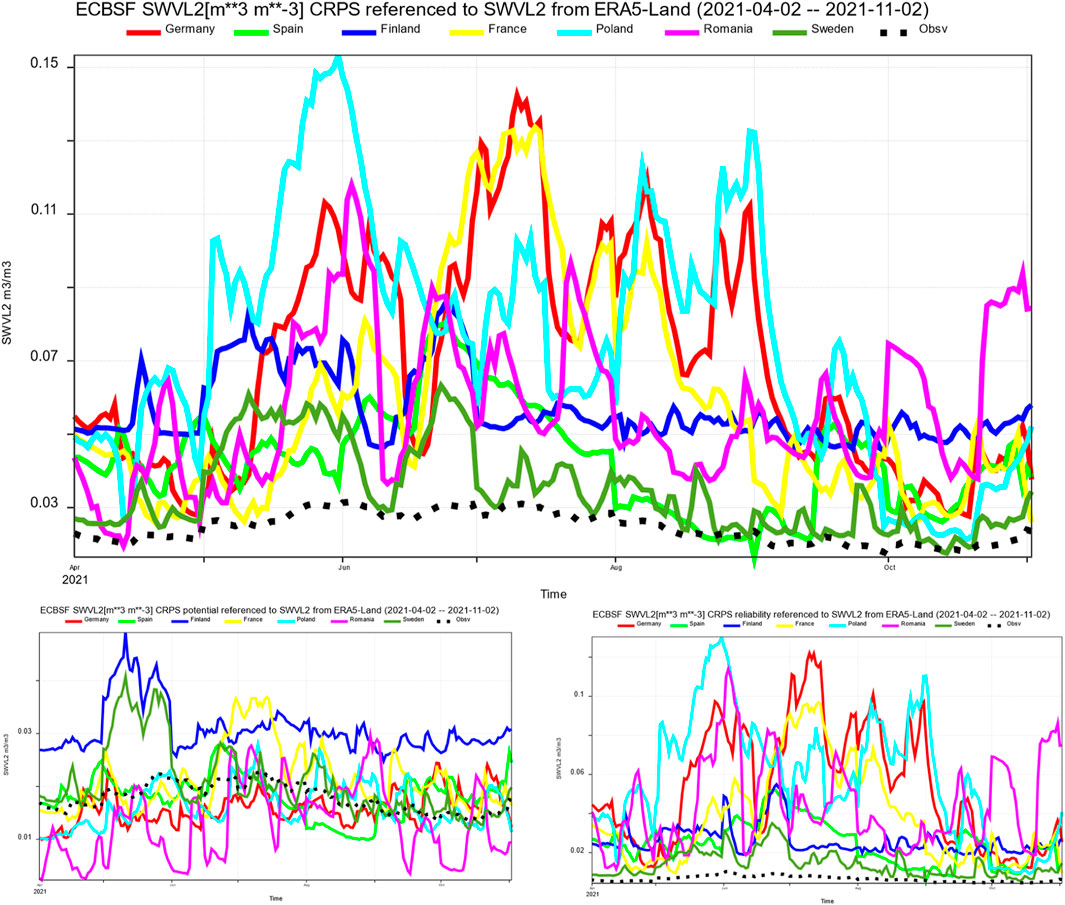
Figure 12. CRPS for ECBSF SWVL2 against ERA5-Land reanalysis as reference with decomposition. Top: CRPS. Bottom left: Potential (resolution/uncertainty). Bottom right: Reliability. Units m3/m3.
These results indicate that the ECXSF climate has a better CRPS performance than observations. Forecasting upcoming weather with seasonal forecasts is difficult, but representing climatic trends should be achievable. Since the CRPS, which for an ensemble forecast represents the same as MAE for a deterministic forecast, is lower than our model’s validation scores, the model demonstrates skill. Furthermore, CRPS Potential scores surpass those for Reliability, suggesting that climate serves as a strong predictor for soil wetness. Notably, skill levels vary a lot across different countries and forecast lengths. Most countries have difficulties across the forecast range without a clear deterioration of skill with longer forecast lead times. This also suggests that climate overall is a good predictor for soil wetness. Why certain countries like Poland consistently exhibit issues over the whole forecast length has likely to do with the unusual weather during the summer of 2021, making climate-based predictions challenging. As neighboring country Germany is similarly a bit less difficult to predict, unusual local weather is a plausible explanation. Further verifications across different seasons and the year 2019 could provide deeper insights, though time constraints limited our analysis in this project.
In Figure 12, the ECBSF and ERA5-Land based bias adjustment appears to outperform SWI2; however, this comparison may be biased, as it essentially measures the model against itself, questioning only the seasonal prediction system’s forecasting skills. An objective assessment of soil wetness representation skill is therefore absent. However, the findings indicate that the seasonal forecasts are gradually improving and approaching climate. Nonetheless, end-users should be aware when predictions diverge from climate, especially when such variations are substantial.
Figure 13 examines details for a specific location in central Finland. The predicted ensemble members, shown in blue, exhibit over 10% spread, with outliers naturally deviating from the ensemble median. Compared to SWI2 observations, only a few observations fall outside the ensemble spread. When compared to SWI2 climatology (monthly averages from 2015-2022), the ensemble generally spreads around the observations/climatology (red lines) but deviates clearly more to wetter or drier conditions at times. This indicator represents the key information the service aims to convey to the end-user, successfully predicting a wetter summer than usual and drier autumn for this location. Supplementary Material S4 contains similar figures for six additional locations (one in each CRPS-assessed country), with results from Sweden, Germany, and France comparable to those in Finland, while forecasts failed for Romania and Poland. Spain displayed limited ensemble spread in late summer but performed well in spring forecasting. The challenges in Romania and Poland are due to atypical extreme summer weather in 2021, which is difficult to predict with climate-based skill. Supplementary Material S4 also includes SWVL2 forecasts based on the bias adjusted seasonal forecast for the same period compared to the SWI2 climate. It is noticeably clear that this product lacks sufficiently strong seasonal signal or ensemble spread.
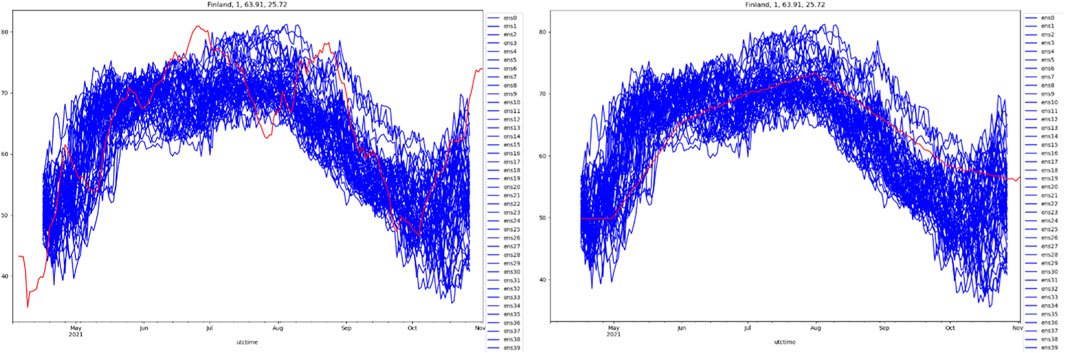
Figure 13. Location in Finland ECXSF SWI2 ensemble members timeseries (blue) plot against SWI2 observations (red, left) and SWI2 climate (red, right). Units %.
Discussion
The results indicate that transitioning from reanalysis-based bias adjusted model data to an Earth Observation-based approach will answer to our service’s key shortcoming experienced by users related to summer bearing capacity. The model in its current state is too close to climate and lacks sufficient spread, seldom signaling the probability for extremes that are crucial for climate adaptation. The ML model predicting an EO product offers a more refined and accurate downscaling, but a service more beneficial to end-users needs also to be able to indicate extremes without being too tied to climatological boundaries. The latter cannot yet be concluded in more statistics, but the location-based figures strongly indicate a useful product, notably much better than bias adjusted seasonal forecasts. Supplementary Material S4 verification time series show that ECBSF SWVL2 is a weak product and requires an update. Broader analysis across additional locations and forecasts is recommended for a more comprehensive evaluation of the ECXSF SWI2 product’s quality.
The Extremes Digital Twin SWI2 forecasts have been operational only for a brief period. With them verification can be performed that will also become practical for end-users, providing them with insights into the forecast’s performance in the past weeks. In Finland, where the current users are located, SWI2 observations are only available outside of the snow season, so with winter having begun early this year, this verification will become useful for the next summer season.
Applying ML to downscale model outputs to match EO products has proven to be feasible. The success of XGBoost was explainable by logical physical relationships by its feature importance compared to a similar quality result for LightGBM. LightGBM’S reliance on features such as dayOfYear, latitude, and longitude suggests a limited understanding of the physical processes affecting soil wetness, whereas XGBoost’s key predictors - SWI climatology, reanalysis soil moisture, soil type, and soil temperature – offer more physically interpretable results. In the latter case, the final model is also easier for end-users to understand. This is a principal factor when applying AI solutions, particularly when the training dataset must be a subset of the entire available data. When all available data are used for training, one can assume that the ML model will fit optimally to the full dataset. However, when only a subset is used, concerns arise regarding whether this subset is sufficiently representative of the full dataset. We limited the training locations in Europe to sites with known land cover characteristics, derived from LUCAS surveys. While a fully randomized subset could have worked too, interpretability would be compromised.
Soil wetness emerges as a robust candidate for climate applications that use EO data to match high resolution observations with coarse models for refined forecasts. Soil wetness captures the cumulative effects of weather over time, which often helps bridge the gap between spatially differing observations and model scales. This is particularly true for soil wetness in-situ measurements, which are highly localized, so a single measurement is seldom well correlated to model estimates that are describing the wetness for larger grid areas. The Soil Water Index at a one-square-kilometer resolution is a bridge between reliable observations and well-performing models on their respective scales. Focusing on soil wetness at a depth of 7–28 cm improves the predictability of our target variable, as changes in subsurface soil are an accumulation of surface condition variations over multiple days. Climate is defined as the long-term statistical pattern of a variable’s states over many years. Combining short periods of measurements helps align the model with the EO products, as indicated by the feature importance of both 15-day accumulated evaporation and precipitation in Figures 7, 8.
Feature engineering of predictors in this ML exercise involved recognizing the production characteristics of the SWI predictand and incorporating its seasonal climatology. According to the SWI documentation, our SWI2 layer should correspond to either the original SWI10 or SWI15 datasets, where the number signifies the days over which surface soil moisture observations are aggregated. We selected SWI15, expanding predictors for precipitation, evaporation, and runoff to include 15-day running cumulative sums in addition to daily, 5-day, 60-day and 100-day sums (5 = SWI1, 60 = SWI3 and 100 = SWI4). Testing with 107 predictors revealed that the 15-day sums held higher feature importance compared to other sums. Ultimately, SWI climatology, calculated as the monthly mean of the years 2015–2022 and interpolated for each day of the year to ensure a smooth transition throughout the year, emerged as the most important predictor. Using the average seemed better than using minimum and maximum bounds, as it allows the model to project extreme events beyond recorded data. The nature of decision trees as the underlying AI logic might limit this capability when relying on minimum and maximum values.
On the technical side, the ability to retrieve data across various model grids or EO product rasters has been essential for assembling the training dataset. The FMI open-source data dissemination system SmartMet-server has proven effective, with most training data fetched directly from it in tabular form. Its Timeseries interface handles interpolation to precise locations automatically, based on the variable’s configuration. While some high-resolution EO data was retrieved from cloud-optimized GeoTiff files using the gdallocationinfo program (GDAL/OGR contributors, 2023), the comparison of these two methods illustrates the strength of APIs like SmartMet-server’s Timeseries for EO data dissemination. GDAL allows retrieving data only one file at a time with just two different output formats. Therefore, depending on timesteps and variables present in the files, the query must be adapted accordingly. Unlike GDAL, SmartMet-server’s Timeseries query can include multiple producers and timesteps and allows data aggregation and simple math operations between variables, simplifying ML data preparation significantly.
Conclusion
The key conclusion from our application is positive: an EO-based AI model can significantly improve the prediction of soil wetness in Europe. The model is driven by IFS data, combining the predictive skill of numerical weather prediction and the ability to distinguish local conditions from satellite-based EO data. AI, and in our case gradient boosting methodologies, serves as an effective bridge between these two datasets. A minimum of 5 years of data is required for the predictand, with ERA5 or ERA5-Land reanalysis consistently available, offering a broad variety of variables relevant to the predictand. Since the reanalysis is based on the ECMWF IFS model system, predictions can be produced across weather, sub-seasonal, seasonal, and climate periods. The IFS is employed for all these applications, with data openly available for seasonal and climate, and with some limitations for weather forecasts. Applications can be developed globally and for various uses. In our forestry example, the weather period is directly related to actual harvesting operations, the seasonal timeframe addresses long-term operational planning, and the climate considerations assist in planning investments throughout the forestry production chain–from forest owners to bio factories.
From a technological standpoint, attention in machine learning must focus on the representativeness of training data and feature engineering. In our case, incorporating climatology for SWI observations was important for success. Eight years of SWI observations effectively represented both the climate and sufficient variability across Europe for different soil wetness events in varying seasons. Utilizing locations with prior knowledge of their characteristics facilitates the evaluation of representativeness, and the LUCAS survey locations in Europe enabled us to achieve this conveniently. Reanalysis data supports complex feature engineering by combining variables and generating temporal statistics.
There are excellent software tools available for AI development, making machine learning a user-friendly experience. Gradient boosting methods are effective when training locations and predictors can be limited based on the memory available in the computing system. The challenge in our case will be to expand the prediction area to grids with higher resolution; however, we still managed to operate on a system with 225 GB of memory and 64 CPUs for both training and prediction. For more flexible production, it is essential to enable XGBoost to support predictions in chunks, rather than requiring the entire grid at once.
Integrating climate adaptation into day-to-day decision making is challenging, as new predictions are produced many years apart and the significant changes within one’s domain often seem distant. Seasonal forecasts provide a monthly evaluation of whether an application domain faces risks of extraordinary conditions. This helps to prepare and gradually adapt to a changing climate in a more tactical manner. Encouraging end-users to utilize this information is challenging, as most domains, such as forestry, typically plan only within the period of weather forecasts. Our service, harvesterseasons.com, has gradually gained more attention, but for broader success, the information provided must be of high quality. The outlook for soil wetness appears promising, and the upcoming summer season will be revealing.
Data availability statement
The datasets presented in this study can be found in online repositories. The names of the repository/repositories and accession number(s) can be found below: https://desm.harvesterseasons.com/grid-gui (producers ECXSF and EDTE for output; ECSF, EDTE, ERA5L, SWI, ECC and SG for input) https://destine.data.lit.fmi.fi/soilwater/swi2_training_10000lucasPoints_2015-2022_all_soils_swi2clim_ecc.csv.gz (training data).
Author contributions
MS: Conceptualization, Data curation, Funding acquisition, Methodology, Project administration, Resources, Supervision, Writing–original draft, Writing–review and editing. AK: Formal Analysis, Investigation, Methodology, Software, Validation, Visualization, Writing–original draft, Writing–review and editing. GP: Formal Analysis, Writing–original draft. MK: Writing–review and editing. MM: Writing–review and editing. HO: Writing–review and editing. AP: Writing–review and editing.
Funding
The author(s) declare that financial support was received for the research, authorship, and/or publication of this article. The technology demonstration and machine learning work were carried out as a part of the HarvesterDestinE project, supported by European Union Destination Earth funding managed by the European Center for Medium-Range Weather Forecasts (ECMWF) contract DE_370d_FMI. In addition to funding ECMWF also guided and approved project reports, which form a large basis of this paper.
Acknowledgments
The authors wish to acknowledge CSC – IT Center for Science, Finland, for computational resources.
Conflict of interest
Authors HO and AP were employed by Metsäteho Oy.
The remaining authors declare that the research was conducted in the absence of any commercial or financial relationships that could be construed as a potential conflict of interest.
Publisher’s note
All claims expressed in this article are solely those of the authors and do not necessarily represent those of their affiliated organizations, or those of the publisher, the editors and the reviewers. Any product that may be evaluated in this article, or claim that may be made by its manufacturer, is not guaranteed or endorsed by the publisher.
Supplementary material
The Supplementary Material for this article can be found online at: https://www.frontiersin.org/articles/10.3389/frsen.2024.1360572/full#supplementary-material
Abbreviations
SWI, Soil Water Index; EO, Earth Observation; IFS, Integrated Forecasting System; XGBoost, Extreme Gradient Boosting; LightGBM, Light Gradient Boosting Machine; ERA5-Land, ERA5-Land reanalysis; LUCAS, Land Use/Cover Area frame Survey; ECBSF, bias-adjusted ECMWF seasonal forecast; EDTE, ECMWF Digital Twin Extremes forecast.; ECXSF, XGBoost products from ECMWF seasonal forecast; CRPS, Continuous Ranked Probability Score; MAE, mean absolute error.
References
Akiba, T., Sano, S., Yanase, T., Ohta, T., and Koyama, M. (2019). “Optuna: a next-generation hyperparameter optimization framework,” in Proceedings of the 25th {ACM} {SIGKDD} international conference on knowledge discovery and data mining. Available at: https://optuna.org.
Bauer-Marschallinger, B., Paulik, C., Hochstöger, S., Mistelbauer, T., Modanesi, S., Ciabatta, L., et al. (2018). Soil moisture from fusion of scatterometer and SAR: closing the scale gap with temporal filtering. Remote Sens. 1019, 1030. doi:10.3390/rs10071030
Buitinck, L., Louppe, G., Blondel, M., Fabian, P., Mueller, A., Grisel, O., et al. (2013). “API design for machine learning software: experiences from the scikit-learn project,” in ECML PKDD workshop: languages for data mining and machine learning, 108–122. Available at: https://scikit-learn.org.
Chen, T., and Guestrin, C. (2016). “XGBoost: a scalable tree boosting system,” in Proceedings of the 22nd ACM SIGKDD international conference on knowledge discovery and data mining (New York, NY, USA: ACM), 785–794. doi:10.1145/2939672.2939785
d’Andrimont, R., Yordanov, M., Martinez-Sanchez, L., Eiselt, B., Palmieri, A., Dominici, P., et al. (2020). Harmonised LUCAS in-situ land cover and use database for field surveys from 2006 to 2018 in the European Union. Sci. Data 7, 352. doi:10.1038/s41597-020-00675-z
GDAL/OGR contributors (2023). GDAL/OGR geospatial data abstraction software library. Open-Source Geospatial Found. doi:10.5281/zenodo.5884351
Hersbach, H. (2000). Decomposition of the continuous ranked probability score for ensemble prediction systems. Weather Forecast. 15 (5), 559–570. doi:10.1175/1520-0434(2000)015<0559:dotcrp>2.0.co;2
Hoyer, S., and Hamman, J. (2017). xarray: N-D labeled Arrays and Datasets in Python. J. Open Res. Softw. 5 (1), 10. doi:10.5334/jors.148
Johnson, S. J., Stockdale, T. N., Ferranti, L., Balmaseda, M. A., Molteni, F., Magnusson, L., et al. (2019). SEAS5: the new ECMWF seasonal forecast system. Geosci. Model Dev. 12, 1087–1117. doi:10.5194/gmd-12-1087-2019
Ke, G., Meng, Q., Finley, T., Wang, T., Chen, W., Ma, W., et al. (2017). Lightgbm: a highly efficient gradient boosting decision tree. Adv. neural Inf. Process. Syst. 30, 3146–3154. doi:10.5555/3294996.3295074
Lehtonen, I., Venäläinen, A., Kämäräinen, M., Asikainen, A., Laitila, J., Anttila, P., et al. (2019). Projected decrease in wintertime bearing capacity on different forest and soil types in Finland under a warming climate. Hydrol. Earth Syst. Sci. 23, 1611–1631. doi:10.5194/hess-23-1611-2019
Muñoz Sabater, J. (2019). ERA5-Land hourly data from 1950 to present. Copernic. Clim. Change Serv. (C3S) Clim. Data Store (CDS). doi:10.24381/cds.e2161bac
Poggio, L., de Sousa, L. M., Batjes, N. H., Heuvelink, G. B. M., Kempen, B., Ribeiro, E., et al. (2021). SoilGrids 2.0: producing soil information for the globe with quantified spatial uncertainty. SOIL 7, 217–240. doi:10.5194/soil-7-217-2021
Randriamampianina, R. (2023). And the on-demand extremes digital twin team: destination Earth on-demand extremes digital twin. EGU General Assem. doi:10.5194/egusphere-egu23-6122
Keywords: soil wetness, forestry operations, seasonal forecast quality, XGBoost (Extreme gradient boosting), lightGBM, ERA5-Land, soil water index (SWI), SoilGrids
Citation: Strahlendorff M, Kröger A, Prakasam G, Kosmale M, Moisander M, Ovaskainen H and Poikela A (2024) Forestry climate adaptation with HarvesterSeasons service—a gradient boosting model to forecast soil water index SWI from a comprehensive set of predictors in Destination Earth. Front. Remote Sens. 5:1360572. doi: 10.3389/frsen.2024.1360572
Received: 23 December 2023; Accepted: 13 November 2024;
Published: 20 December 2024.
Edited by:
Yongxiang Hu, National Aeronautics and Space Administration, United StatesReviewed by:
Nazario Tartaglione, Istituto Superiore per la Protezione e la Ricerca Ambientale (ISPRA), ItalyAbhishek Lodh, Swedish Meteorological and Hydrological Institute, Sweden
Copyright © 2024 Strahlendorff, Kröger, Prakasam, Kosmale, Moisander, Ovaskainen and Poikela. This is an open-access article distributed under the terms of the Creative Commons Attribution License (CC BY). The use, distribution or reproduction in other forums is permitted, provided the original author(s) and the copyright owner(s) are credited and that the original publication in this journal is cited, in accordance with accepted academic practice. No use, distribution or reproduction is permitted which does not comply with these terms.
*Correspondence: Mikko Strahlendorff, bWlra28uc3RyYWhsZW5kb3JmZkBmbWkuZmk=
†ORCID: Mikko Moisander, orcid.org/0000-0003-1435-6465; Heikki Ovaskainen, orcid.org/0000-0001-5063-6662