- 1Centre for Geography and Environmental Science, University of Exeter, Penryn, United Kingdom
- 2NASA Goddard Space Flight Center (USRA), Greenbelt, MD, United States
- 3Seafari Marine Services, Stony Brook, NY, United States
- 4Plymouth Marine Laboratory, Plymouth, United Kingdom
Visible spectral radiometry of seawater, often referred to as ocean colour, from space, provides a synoptic view of surface phytoplankton, and other optically-active constituents, at high temporal resolution, that is unsurpassed by any other technology. Yet, in-situ observations of ocean colour are critical to the success of the satellite, tracking the calibration of the radiometers and validating atmospheric correction algorithms. Owing to the high cost of commercial field radiometers, as well as the high costs associated with ocean-based field work, ocean colour scientists are plagued by a sparsity of high quality in-situ radiometric observations, particularly in remote regions. In this perspective article, we highlight potential to increase the number of in-situ observations of ocean colour by harnessing superyachts. Using openly-available data processing software, we show that automated ocean colour data collected using a superyacht can be used for the validation of an ocean colour satellite, with comparable results to traditional validation studies. Reaching out to wealthy citizen scientists may help fill gaps in our ability to monitor the colour of the ocean.
1 Introduction
Ocean colour is defined as spectral variations in water leaving radiance, or reflectance, in visible bands of the electromagnetic spectrum. A primary application of ocean-colour data is to observe the dynamics of phytoplankton in the ocean. The optical signal is processed to retrieve information on the major phytoplankton pigment, chlorophyll-a. Methods are also available to infer phytoplankton community structure from ocean-colour data, by exploiting spectral differences in the optical signatures of different types of phytoplankton, as well as other important optically-active constituents, such as the types of coloured dissolved organic matter (CDOM) in the water and non-algal particles. Recognised as an Essential Climate Variable, ocean-colour data helps to address many important issues, such as changing ocean biogeochemical cycles, collapsing fisheries, climate change, and shifts in waterborne diseases (Sathyendranath et al., 2023).
Ocean-colour sensors mounted on satellites, such as NASA’s Moderate Resolution Imaging Spectroradiometer (MODIS) sensors, provide ocean-colour data on a daily basis (clouds permitting) at global scale, with a resolution of a few kilometres. Continual satellite observations since the late 1980’s mean that these products provide an excellent platform for monitoring and interpreting regional, seasonal and interannual variations in marine plankton populations and their activity, over multiple decades (Sathyendranath et al., 2019). Ocean-colour data can be formally combined with numerical models of ocean circulation and ecosystems to provide state estimates of the system (i.e., using the models as an interpolation tool) and these data can be used to constrain parameters of the models which can then be used in prognostic mode (IOCCG, 2020).
A critical component of successful satellite ocean colour remote sensing, is the validation and calibration of the satellite data using in-situ measurements, collected at the ocean surface (Mélin, 2022). Despite large efforts by the scientific community to collect and archive in-situ measurements of ocean colour (Valente et al., 2022), a paucity of in-situ data limits our capability to validate and calibrate satellite ocean-colour data, meaning the satellite data cannot be harnessed to their full extent. This is driven by the high cost of commercial in-situ radiometers, as well as the costs associated with ocean-based field work, particularly in remote regions (Lauro et al., 2014). Solutions to this issue include harnessing optical sensors mounted on autonomous platforms (Demeaux and Boss, 2022), mounting sensors on ships-of-opportunity (Simis and Olsson, 2013; Raitsos et al., 2014; Brando et al., 2016; Wang and Costa, 2022) and using citizen science (Lauro et al., 2014; Vanhellemont et al., 2022). Citizen science (or participatory science), requires allocating a task, usually conducted by professionals, to a network of voluntary citizens. If successfully administered, it can improve public understanding of science by disseminating knowledge through different social classes, and tackle expensive, demanding and intractable scientific data collection (Bresnahan et al., 2022). Typically, use of citizen science for evaluating ocean colour data has focused on low cost options, tapping into mobile phones, either for logging data using low cost tools (Novoa et al., 2014; Busch et al., 2016; Brewin et al., 2019; George et al., 2021), or harnessing mobile phone camera sensors (Leeuw and Boss, 2018; Burggraaff et al., 2022). Although these low cost options have proven remarkably robust (e.g., see Brewin et al., 2023), they can lack the quality required for accurate validation and calibration (Lin et al., 2022).
In this perspective article, we discuss opportunities to expand in-situ measurements of ocean colour by engaging wealthier citizen scientists who own superyachts, who can afford to purchase commercial in-situ radiometers, who have an active interest in environmental monitoring, and who often sail to remote areas that are not routinely covered by research vessels or ships-of-opportunity. We begin by demonstrating results from a pilot study, where automated ocean colour data were collected on a superyacht and used for the validation of an ocean colour satellite, and finish by discussing challenges that need to be addressed for this strategy to be successful.
2 Pilot study on the Archimedes yacht
2.1 Archimedes yacht setup and data collection
A Surface Acquisition System (SAS) Solar Tracker Aiming unit was acquired from Sea-Bird Scientific (https://www.seabird.com/), equipped with two HyperOCR radiance sensors (with a field of view of 11.5° in air), measuring hyperspectral total water radiance (Lt) and sky radiance (Li) at an angle of 40° from nadir and zenith respectively, a HyperOCR irradiance sensor, measuring downwelling irradiance (Es), and a infrared radiation pyrometer, for measuring sea surface temperature (SST, not used in this study). The system is designed to maintain the correct pointing angle with respect to the Sun, permitting autonomous operation (Figure 1A). The SAS Solar Tracker Aiming unit was mounted to the bow of the Archimedes Yacht (Figure 1B), following Sea-Bird Scientific (2017) assembly instructions, and aligned to point forward, offering the best placement for viewing total water radiance (Lt) at angles relative to the yacht’s center line. The placement of the SAS Solar Tracker Aiming unit at the bow minimised errors from shading and reflection due to the yacht, and ensures that the water sampled is undisturbed by the yacht’s wake. This placement also considered the position of the Es sensor, being far from the yacht’s bridge and cabins, minimising shading and reflection effects, with the exception of a thin telescopic removable mast which was occasionally erected near the bow of the yacht (see Figure 1B for image when erected) and may have impacted Es data collected (see discussion of this aspect in Section 3). The system was deployed on Archimedes Yacht in calm seas at various periods over 3 years (2018–2020), when stationary and moving, and sampled a wide range of geographical locations and optical water types, including: the east coast of the United States, the Caribbean, the northern and equatorial Atlantic, coastal regions of the United Kingdom and Ireland, the Mediterranean Sea, the South Pacific, and the Antarctic Peninsular. By the end of 2020, the system had begun to degrade following extensive environmental exposure and required repair (see Figure 1D).
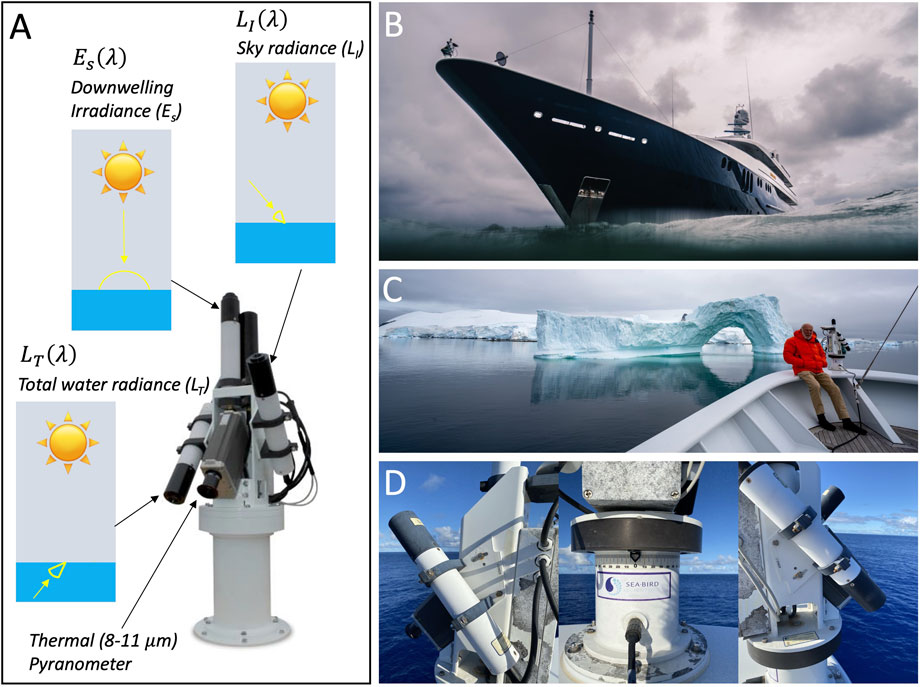
FIGURE 1. (A) Sea-Bird Scientific Solar Tracker Aiming System measuring total water radiance and sky radiance at a solid angle, and downwelling irradiance, which are used to compute remote sensing reflectance. Reproduced with permission from Sea-Bird Scientific (2017). (B) Position of the Sea-Bird Scientific Solar Tracker on the bow of the Archimedes Yacht. (C) Jim Simons, owner of the Archimedes Yacht alongside the Sea-Bird Scientific Solar Tracker in Antarctica, a remote region where few satellite validation studies have been performed. (D) Images showing degradation of the Sea-Bird Scientific Solar Tracker after 3 years of operation (note the corrosion of the instrumentation on the base and junction box of the Solar Tracker).
2.2 In-situ data processing
Data were collected using SatView software, Sea-Bird Scientific/Satlantic’s real time data logging and display software for Microsoft Windows, with “.raw” files logged on an hourly interval. Data were processed using NASA’s open source HyperInSPACE software (https://github.com/nasa/HyperCP). Originally developed to provide hyperspectral in-situ support for NASA’s Plankton, Aerosol, Cloud, ocean Ecosystem (PACE) mission, HyperInSPACE is specifically designed to process automated, above-water, hyperspectral ocean color radiometry data, and supports data collected using the Sea-Bird Scientific SAS Tracker Aiming unit. HyperInSPACE offers the user a range of processing options, among which include various quality assurance methods and protocols, uncertainty estimation, sky and sunglint correction, and convolution of data to satellite wavebands. The software also outputs files formatted to text files for submission to NASA’s SeaBASS database.
For this pilot study, the Archimedes data were processed as follows: 1) SatView Level 0 “.raw” files were periodically transferred from the yacht via File Transfer Protocol (FTP) and processed to Level 1A (Hierarchical Data Format 5 “.hdf”) using a solar zenith angle filter that discarded data greater than 60° (Brewin et al., 2016); 2) Level 1A files were processed to Level 1AQC by removing data with absolute rotator angles greater than 40° and less than −40°, removing data with azimuth angles less than 90° and greater than 135° (Mobley, 1999; Zhang et al., 2017), and deglitching the data using default HyperInSPACE settings; 3) Level 1AQC files were processed to Level 1B, correcting for dark counts, applying instrument calibration files, and interpolating to common set of timestamps and a common set of wavebands (at 3.3 nm resolution); 4) Level 1B files were processed to Level 1BQC, using the Zhang et al. (2017) glint correction, which required downloading GMAO MERRA2 ancillary data (see https://oceancolor.gsfc.nasa.gov/resources/docs/ancillary/) through HyperInSPACE, removing data with wind speed
In total, 2,402 hyperspectral Rrs spectra made it through the processing chain, 314 collected in 2018, 1,605 in 2019, and 483 in 2020 (Figures 2A–D). Data were outputted as NASA SeaBASS formatted text files and are openly available through the Simons Collaborative Marine Atlas Project (https://simonscmap.com) and NASA SeaBASS website (https://seabass.gsfc.nasa.gov/experiment/SUPERYACHT_SCIENCE). This perspective article is focused on demonstrating the potential of augmenting superyachts with automated ocean colour radiometers, rather than testing all the potential combinations of settings in HyperInSPACE, and hence we have used a standard set of settings we think sensible for this dataset. However, all the Level 0 “.raw” data and instrument calibration files are openly available in the NASA SeaBASS archive, for future work testing and comparing different settings, or for reprocessing as and when new updates to HyperInSPACE are introduced.
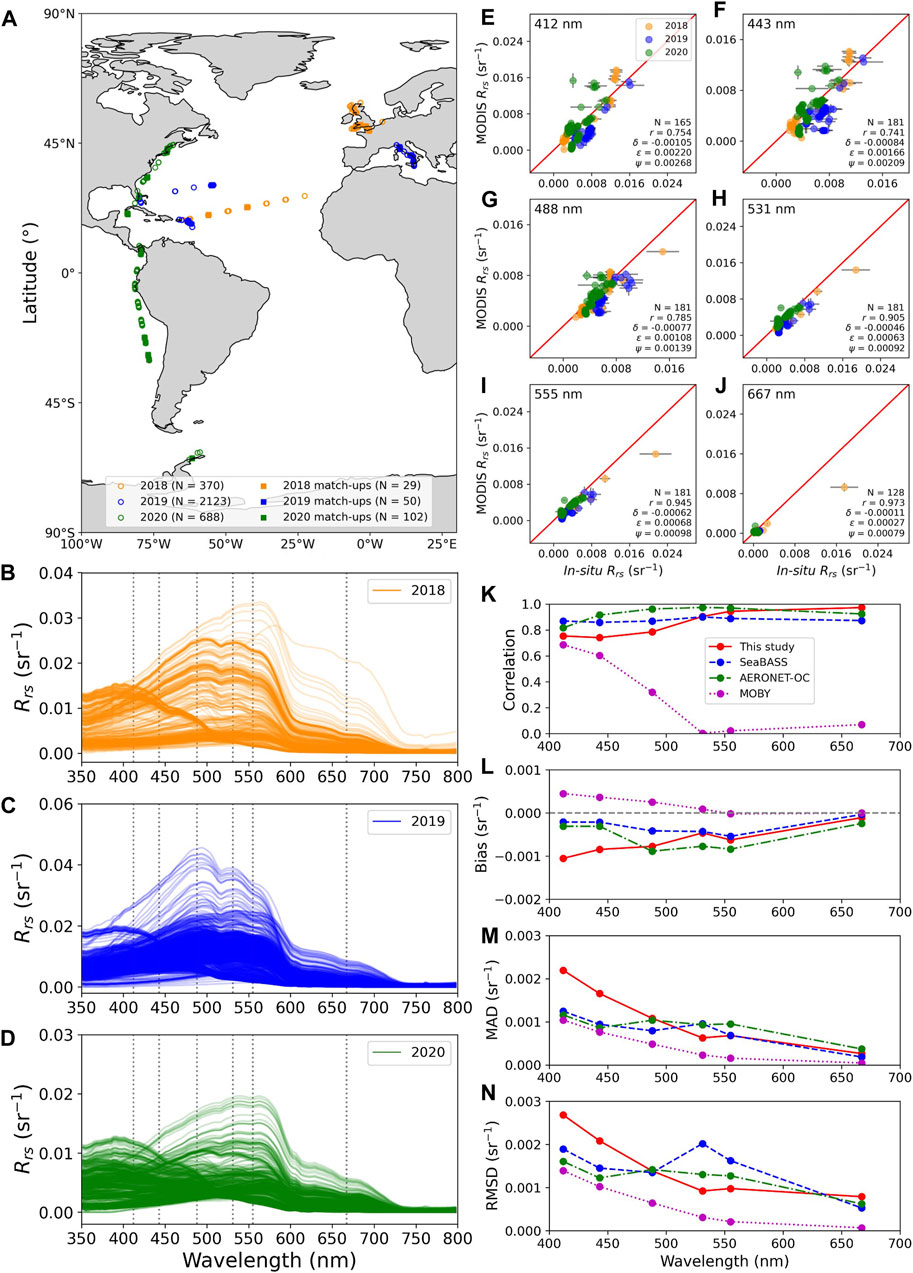
FIGURE 2. (A) Map showing locations of Archimedes remote-sensing reflectance data (Rrs) at 443 nm, processed using HyperInSPACE and the locations of MODIS-Aqua match-ups (N refers to the number of spectra). (B–D) Rrs as a function of wavelength, for Archimedes data collected in 2018, 2019 and 2020, respectively. The dotted lines represent the six wavelengths (412, 443, 488, 531, 555, 667 nm) of MODIS-Aqua satellite data used in the satellite validation. (E–J) Comparison of in-situ (after BRDF correction) and MODIS-Aqua-derived Rrs at six wavelengths (412, 443, 488, 531, 555, 667 nm respectively). The error bar on the x-axis direction is the uncertainty in the in-situ measurements, the error bar on the y-axis direction is the standard deviation of MODIS-Aqua-derived data. The statistical tests include correlation (r), bias (δ), mean absolute difference (MAD, denoted ϵ), and root mean squared difference (RMSD, denoted Ψ). (K–N) MODIS-Aqua validation statistics (correlation, bias, MAD, and RMSD, respectively) from this study as a function of wavelength compared with validation results obtained from NASA SeaBASS, including SeaBASS, AERONET-OC, and MOBY.
2.3 Satellite MODIS-Aqua match-ups
In-situ Rrs spectra at six wavebands (412, 443, 488, 531, 555, 667 nm, convolved to the spectral bands of MODIS-Aqua), and their corresponding uncertainties, were used for comparison with Level 2 (L2) MODIS-Aqua data. To compare the in-situ Rrs data more directly with MODIS-Aqua Rrs, we performed a bidirectional reflectance distribution function (BRDF) correction following the method of Lee et al. (2011), inputting information on sun and viewing angles, and environmental conditions. In-situ data were matched with MODIS-Aqua L2 data through a python tool available through NASA SeaBASS (fd_matchup.py, https://seabass.gsfc.nasa.gov/wiki/validation_matchup_tools#Satellite%20Match-Up%20Extractor). Each in-situ sample was matched in time (within the ±4 h window) and space (closest latitude and longitude) with a MODIS-Aqua L2 image. From 3,181 in-situ data, 2,402 had matching MODIS-Aqua L2 images (R2022 reprocessing), resulting in a total of 228 images, which were downloaded automatically from NASA’s ocean colour website (https://oceandata.sci.gsfc.nasa.gov/directdataaccess/) on 2 October 2023.
Each in-situ sample was paired with its corresponding MODIS-Aqua L2 image in space by extracting a 3 × 3 pixel window centred on the location of the in-situ sample. The mean and standard deviation of satellite-derived Rrs was calculated from nine pixels, and was retained if the following conditions were met: 1) at least five valid pixels were available from the nine, and 2) the closest satellite pixel contained a valid value. In total, 181 samples fulfilled these criteria, with 29, 50, and 102 samples in 2018, 2019 and 2020 respectively (Figure 2A). To compare the Archimedes in-situ data with MODIS-Aqua, we computed four statistical metrics, commonly used in validation exercises (e.g., Brewin et al., 2015), including the correlation coefficient (r), mean difference (bias, denoted δ), mean absolute difference (MAD, denoted ϵ), and root mean squared difference (RMSD, denoted Ψ), all computed in linear space.
To compare the validation results from Archimedes with those from more traditional validation studies conducted by ocean colour scientists, we computed the same statistical metrics, for MODIS-Aqua derived Rrs (R2022 processing), using in-situ Rrs match-ups available in the NASA SeaBASS website (https://seabass.gsfc.nasa.gov/search#val, accessed on 3 October 2023, using standard settings in the tool). These included results from SeaBASS, AERONET-OC, and MOBY. The SeaBASS, AERONET-OC, and MOBY datasets contain a maximum (wavelength-depended) of 730, 6663, and 776 samples, respectively.
3 Augmenting superyachts with ocean colour radiometers
Archimedes in-situ Rrs data compare well with MODIS-Aqua Rrs at the six wavelengths studied (Figures 2E–J). Statistical metrics obtained in the validation exercise using Archimedes are in good agreement with those using other data collected by scientists (Figures 2K, L). Overall, Archimedes Rrs biases, MAD and RMSD are slightly higher for blue wavelengths (412 and 443 nm), but lower than, or comparable to, other validation studies, between 488 and 665 nm (Figures 2L–N). Considering MODIS-Aqua blue bands are sensitive to temporal degradation (Lee et al., 2019), poorer statistics in blue wavelengths for Archimedes likely reflects that this validation was conducted in more recent years (2018–2020), in comparison with the other validation studies that include data from an earlier time period (see also recent study by Pardo et al., 2023). These result demonstrate that automated Rrs data collection on superyachts can provide very useful data for the validation of satellite ocean colour measurements. When considering superyachts visit remote regions (e.g., in the case of Archimedes, the Antarctic peninsular, see Figure 1C), this may help provide quality in-situ radiometric observations in regions rarely sampled. Furthermore, with increasing numbers of ocean-colour satellites being launched, long-term investments in sustaining the time-series of ocean colour data (e.g., through the ESA’s Sentinel 3 program), and new exciting hyperspectral ocean-colour satellites being developed (e.g., NASA’s PACE mission), it is critical that the international community continues investing in the collection of Rrs in-situ data and explore new ways to expand the temporal and spatial coverage of these datasets. Augmenting superyachts with ocean colour radiometers could be one opportunity to do this. Nonetheless, for this strategy to be successful, certain challenges need to be considered:
• The scientific community needs to find ways to engage with superyacht communities, to highlight the value and benefits of collecting in-situ data. We may be better positioned to do this if we team up with other marine scientists, who are developing automated tools for collecting other useful oceanography data (e.g., microplastsics, see Setälä et al., 2022), possible bringing these instruments together into a simple data collection package that would benefit a broader range of marine scientists. Scientists should consider ways in which they could motivate superyacht owners to collect in situ data, building on citizen-science based principles (Garcia-Soto et al., 2021) and existing initiatives (e.g., https://yachtsforscience.com), promoting financial investment that adds to existing funding for validation. Satellite validation work could also extend beyond ocean colour data, for example, collecting SST data for validation of satellite thermal radiometers, by utilising state-of-the-art in-situ methods (e.g., Donlon et al., 2014). Ocean colour scientists may also benefit from integration of radiometric systems with other automated systems for monitoring the inherent optical properties of the water (e.g., Slade et al., 2010).
• Availability of automotive radiometric systems is a potential issue for ocean colour validation on superyachts. Unfortunately, a few years after the Sea-Bird Scientific SAS Solar Tracker Aiming unit was purchased for Archimedes, the manufacturer decommissioned the system. However, similar systems are now being developed. For example, the EU Monocle project saw the development of a Solar-tracking Radiometry platform (So-Rad) system (see https://www.monocle-h2020.eu/Sensors_and_platforms/Solar_tracking_radiometry_platform_en) designed to be operated with commercial radiometers (TriOS RAMSEs), with a high degree of automation and low-power consumption. The So-Rad designs are open-source and the system can be installed on non-stationary platforms like superyachts.
• It is essential there is a close dialog between the crew of the superyacht and scientists, so as to ensure these systems are set-up appropriately and for support during operation. This was key to the success of the pilot study on the Archimedes yacht. Sea-Bird Scientific SAS Solar Tracker Aiming unit was placed right at the bow of Archimedes, and carefully set-up by the vessels caption and crew, and removed and replaced during periods of rough and calm weather. In hindsight, it would have been useful to document when the telescopic mast was erected (see Figure 1B) so as to quantity any effect it may have had on the Es data. Future efforts may benefit from placing the Es elsewhere on the vessel (e.g., at top of the telescopic mast). Such a dialog requires a good relationship between scientists and crew.
• For the pilot study on the Archimedes yacht, data were periodically transferred via FTP. This worked well, but meant data were not processed in near real time. Adopting a near real time data transfer/upload system could be useful for operational applications and identifying any issues with the data that could be resolved quickly (both in situ and satellite).
• The pilot study on the Archimedes yacht benefited from the development of HyperInSPACE software, allowing for the rapid processing of radiometric data, with full traceability, building on community developed and supported protocols (IOCCG, 2019). The software is also designed to ingest new developments in radiometric data processing, as and when they appear. Increasing efforts are being placed on quantifying uncertainties with full SI traceability (Ruddick et al., 2019; Banks et al., 2020; Tilstone et al., 2020; Lin et al., 2022; Pardo et al., 2023), that should be incorporated in these software in the future, bringing further rigor to the quality of the measurements.
• It is essential that appropriate data archiving systems are maintained and available to support initiatives like this. These need to be open source, to maximise data usage, and must be designed to cater for the raw radiometric measurements, the processed data, with full traceability between the two, so users can reprocess the data if needed, using different settings, or future reprocessing can be made, should new knowledge come to light. The NASA SeaBASS archive is ideally suited for this. Additionally, these data should also be ingested into other archives or data complications, to broaden the user community and allow for integration with other data streams. For the pilot study on the Archimedes yacht, we also submitted the dataset to the Collaborative Marine Atlas Project (CMAP), an open-source data portal designed for retrieving, visualizing, and analysing diverse ocean datasets (see https://simonscmap.com). It strives to enable scientists and the public to dive into the vast ocean datasets without going through the time-consuming process of finding and harmonizing data from different sources (Ashkezari et al., 2021). Such an atlas maybe useful for the development of future ocean colour algorithms and for widening the use of radiometric datasets.
4 Summary and outlook
Due to the high cost of ocean-based field work and commercial field radiometers, there is a paucity of high quality in-situ radiometric observations required for satellite validation of ocean colour data. In this perspective article, we have highlighted potential to increase in-situ data collection by harnessing superyachts. Utilising openly-available data processing and archiving software, we demonstrate that automated ocean colour data collected from a superyacht can be used for the validation of an ocean colour satellite, with comparable results to more traditional validation studies. We discuss challenges and future work needed for this strategy to be successful.
Data availability statement
The datasets presented in this study can be found in online repositories. The names of the repository/repositories and accession number(s) can be found below: https://seabass.gsfc.nasa.gov/experiment/SUPERYACHT_SCIENCE; and https://oceandata.sci.gsfc.nasa.gov/directdataaccess/.
Ethics statement
Written informed consent was obtained from the individual(s) for the publication of any potentially identifiable images or data included in this article.
Author contributions
RB: Conceptualization, Formal Analysis, Funding acquisition, Investigation, Methodology, Project administration, Resources, Software, Validation, Visualization, Writing–original draft, Writing–review and editing. XS: Formal Analysis, Investigation, Methodology, Writing–review and editing. DA: Formal Analysis, Methodology, Resources, Software, Writing–review and editing. JV: Writing–review and editing. CW: Data curation, Resources, Writing–review and editing. SS: Conceptualization, Resources, Writing–review and editing.
Funding
The author(s) declare financial support was received for the research, authorship, and/or publication of this article. RB is funded by a UKRI Future Leader Fellowship (MR/V022792/1) and by the Simons Foundation Project “Processing and distribution of hyperspectral radiometer data from ships of opportunity for monitoring phytoplankton in the ocean” (654879). SS is supported by the Simons Foundation Project Collaboration on Computational Biogeochemical Modeling of Marine Ecosystems (CBIOMES; 549947, SS).
Acknowledgments
The authors would like to thank all the crew of the Archimedes yacht who assisted in the collection of in-situ data. We thank Jim Simons, his family, Marian Carlson and the Simons Foundation, for supporting this work. We thank all those who have supported the development of the HyperInSPACE software and NASA for providing MODIS-Aqua data used in the work.
Conflict of interest
Author CW was employed by Seafari Marine Services.
The remaining authors declare that the research was conducted in the absence of any commercial or financial relationships that could be construed as a potential conflict of interest.
Publisher’s note
All claims expressed in this article are solely those of the authors and do not necessarily represent those of their affiliated organizations, or those of the publisher, the editors and the reviewers. Any product that may be evaluated in this article, or claim that may be made by its manufacturer, is not guaranteed or endorsed by the publisher.
References
Ashkezari, M. D., Hagen, N. R., Denholtz, M., Neang, A., Burns, T. C., Morales, R. L., et al. (2021). Simons Collaborative Marine Atlas Project (Simons CMAP): an open-source portal to share, visualize, and analyze ocean data. Limnol. Oceanogr. Methods 19, 488–496. doi:10.1002/lom3.10439
Banks, A. C., Vendt, R., Alikas, K., Bialek, A., Kuusk, J., Lerebourg, C., et al. (2020). Fiducial reference measurements for satellite ocean colour (FRM4SOC). Remote Sens. 12, 1322. doi:10.3390/rs12081322
Brando, V. E., Lovell, J. L., King, E. A., Boadle, D., Scott, R., and Schroeder, T. (2016). The potential of autonomous ship-borne hyperspectral radiometers for the validation of ocean color radiometry data. Remote Sens. 8, 150. doi:10.3390/rs8020150
Bresnahan, P., Cyronak, T., Brewin, R. J. W., Andersson, A., Wirth, T., Martz, T., et al. (2022). A high-tech, low-cost, internet of things surfboard fin for coastal citizen science, outreach, and education. Cont. Shelf Res. 242, 104748. doi:10.1016/j.csr.2022.104748
Brewin, R. J. W., Brewin, T. G., Phillips, J., Rose, S., Abdulaziz, A., Wimmer, W., et al. (2019). A printable device for measuring clarity and colour in lake and nearshore waters. Sensors 9, 936–1026. doi:10.3390/s19040936
Brewin, R. J. W., Dall’Olmo, G., Pardo, S., van Dongen-Vogel, V., and Boss, E. S. (2016). Underway spectrophotometry along the Atlantic Meridional Transect reveals high performance in satellite chlorophyll retrievals. Remote Sens. Environ. 183, 82–97. doi:10.1016/j.rse.2016.05.005
Brewin, R. J. W., Pitarch, J., Dall’Olmo, G., van der Woerd, H. J., Lin, J., Sun, X., et al. (2023). Evaluating historic and modern optical techniques for monitoring phytoplankton biomass in the Atlantic Ocean. Front. Mar. Sci. 10. doi:10.3389/fmars.2023.1111416
Brewin, R. J. W., Sathyendranath, S., Müller, D., Brockmann, C., Deschamps, P.-Y., Devred, E., et al. (2015). The Ocean colour climate change initiative: III. A round-robin comparison on in-water bio-optical algorithms. Remote Sens. Environ. 162, 271–294. doi:10.1016/j.rse.2013.09.016
Burggraaff, O., Werther, M., Boss, E. S., Simis, S. G. H., and Snik, F. (2022). Accuracy and reproducibility of above-water radiometry with calibrated smartphone cameras using RAW data. Front. Remote Sens. 3. doi:10.3389/frsen.2022.940096
Busch, J. A., Bardaji, R., Ceccaroni, L., Friedrichs, A., Piera, J., Simon, C., et al. (2016). Citizen bio-optical observations from coast-and ocean and their compatibility with ocean colour satellite measurements. Remote Sens. 8, 879–919. doi:10.3390/rs8110879
Demeaux, C. B., and Boss, E. (2022). Validation of remote-sensing algorithms for diffuse attenuation of downward irradiance using BGC-Argo floats. Remotre Sens. 14, 4500. doi:10.3390/rs14184500
Donlon, C. J., Wimmer, W., Robinson, I., Fisher, G., Ferlet, M., Nightingale, T., et al. (2014). A second-generation blackbody system for the calibration and verification of seagoing infrared radiometers. J. Atmos. Ocean. Technol. 31, 1104–1127. doi:10.1175/JTECH-D-13-00151.1
Garaba, S. P., Schulz, J., Wernand, M. R., and Zielinski, O. (2012). Sunglint detection for unmanned and automated platforms. Sensors 12, 12545–12561. doi:10.3390/s120912545
Garcia-Soto, C., Seys, J. J. C., Zielinski, O., Busch, J. A., Luna, S. I., Baez, J. C., et al. (2021). Marine citizen science: current state in Europe and new technological developments. Front. Mar. Sci. 8. doi:10.3389/fmars.2021.621472
George, G., Menon, N. N., Abdulaziz, A., Brewin, R. J. W., Pranav, P., Gopalakrishnan, A., et al. (2021). Citizen scientists contribute to real-time monitoring of lake water quality using 3D printed mini Secchi disks. Front. Water 3. doi:10.3389/frwa.2021.662142
IOCCG (2019). Ocean optics and biogeochemistry protocols for satellite Ocean colour sensor validation, volume 3.0: protocols for satellite Ocean colour data validation: In Situ optical radiometry. Tech. Rep dartmouth, NS, Canada, International Ocean-Colour Coordinating Group (IOCCG), 67pp. (IOCCG protocol series), China, IEEE, G. Zibordi, K. J. Voss, B. C. Johnson, and J. L. Mueller
IOCCG (2020). “Synergy between ocean colour and biogeochemical/ecosystem models,” in IOCCG report series, No. 19. Editors T. rep., and S. Dutkiewicz (Dartmouth, Canada: International Ocean Colour Coordinating Group).
Lauro, F. M., Senstius, S. J., Cullen, J., Neches, R., Jensen, R. M., Brown, M. V., et al. (2014). The common oceanographer: crowdsourcing the collection of oceanographic data. PLoS Biol. 12, e1001947. doi:10.1371/journal.pbio.1001947
Lee, S., Meister, G., and Franz, B. (2019). MODIS Aqua reflective solar band calibration for NASA’s r2018 ocean color products. Remote Sens. 11, 2187. doi:10.3390/rs11192187
Lee, Z. P., Du, K., Voss, K. J., Zibordi, G., Lubac, B., Arnone, R., et al. (2011). An inherent-optical-property-centered approach to correct the angular effects in water-leaving radiance. Appl. Opt. 50, 3155–3167. doi:10.1364/AO.50.003155
Leeuw, T., and Boss, E. (2018). The HydroColor App: above water measurements of remote sensing reflectance and turbidity using a smartphone camera. Sensors 18, 256. doi:10.3390/s18010256
Lin, J., Dall’Olmo, G., Tilstone, G. H., Brewin, R. J. W., Vabson, V., Ansko, I., et al. (2022). Derivation of uncertainty budgets for continuous above-water radiometric measurements along an Atlantic Meridional Transect. Opt. Express 30, 45648–45675. doi:10.1364/OE.470994
Mélin, F. (2022). Validation of ocean color remote sensing reflectance data: analysis of results at European coastal sites. Remote Sens. Environ. 280, 113153. doi:10.1016/j.rse.2022.113153
Mobley, C. D. (1999). Estimation of the remote-sensing reflectance from above-surface measurements. Appl. Opt. 38, 7442–7455. doi:10.1364/ao.38.007442
Mueller, J. L., Morel, A., Frouin, R., Davis, C. O., Arnone, R., Carder, K. L., et al. (2003). Ocean optics protocols for satellite Ocean color sensor validation, revision 4, Vol. III. Greenbelt, MD: NASA Goddard Space Flight Center.
Novoa, S., Wernand, M. R., and van der Woerd, H. J. (2014). The modern Forel-Ule scale: a ‘do-it-yourself’ colour comparator for water monitoring. J. Eur. Opt. Soc. - Rapid Publ. 9, 1–10. doi:10.2971/jeos.2014.14025
Pardo, S., Tilstone, G. T., Brewin, R. J. W., Dall’Olmo, G., Lin, J., Nencioli, F., et al. (2023). Radiometric assessment of OLCI, VIIRS, and MODIS using fiducial reference measurements along the atlantic meridional transect. Remote Sens. Environ. 299, 113844. doi:10.1016/j.rse.2023.113844
Raitsos, D. E., Pradhan, Y., Lavender, S. J., Hoteit, I., McQuatters-Gollop, A., Reid, P. C., et al. (2014). From silk to satellite: half a century of ocean colour anomalies in the Northeast Atlantic. Glob. Change Biol. Press 20, 2117–2123. doi:10.1111/gcb.12457
Ruddick, K. G., De Cauwer, V., Park, Y.-J., and Moore, G. (2006). Seaborne measurements of near infrared water-leaving reflectance: the similarity spectrum for turbid waters. Limnol. Oceanogr. 51, 1167–1179. doi:10.4319/lo.2006.51.2.1167
Ruddick, K. G., Voss, K., Boss, E., Castagna, A., Frouin, R., Gilerson, A., et al. (2019). A review of protocols for Fiducial Reference Measurements of water-leaving radiance for validation of satellite remote-sensing data over water. Remote Sens. 11, 2198. doi:10.3390/rs11192198
Sathyendranath, S., Brewin, R. J. W., Brockmann, C., Brotas, V., Calton, B., Chuprin, A., et al. (2019). An ocean-colour time series for use in climate studies: the experience of the Ocean-Colour Climate Change Initiative (OC-CCI). Sensors 19, 4285. doi:10.3390/s19194285
Sathyendranath, S., Brewin, R. J. W., Ciavatta, S., Jackson, T., Kulk, G., Jönsson, B., et al. (2023). Ocean biology studied from space. Surv. Geophys. 44, 1287–1308. doi:10.1007/s10712-023-09805-9
Sea-Bird Scientific (2017). Product manual SAS solar tracker (document: SAT-DN-635). Tech. Rep. Sea-Bird Scientific.
Setälä, O., Tirroniemi, J., and Lehtiniemi, M. (2022). Testing citizen science as a tool for monitoring surface water microplastics. Environ. Monit. Assess. 194, 851. doi:10.1007/s10661-022-10487-w
Simis, S. G., and Olsson, J. (2013). Unattended processing of shipborne hyperspectral reflectance measurements. Remote Sens. Environ. 135, 202–212. doi:10.1016/j.rse.2013.04.001
Slade, W. H., Boss, E., Dall’Olmo, G., Langner, M. R., Loftin, J., Behrenfeld, M. J., et al. (2010). Underway and moored methods for improving accuracy in measurement of spectral particulate absorption and attenuation. J. Atmos. Ocean. Technol. 27, 1733–1746. doi:10.1175/2010JTECHO755.1
Tilstone, G., Dall’Olmo, G., Hieronymi, M., Ruddick, K., Beck, M., Ligi, M., et al. (2020). Field intercomparison of radiometer measurements for ocean colour validation. Remote Sens. 12, 1587. doi:10.3390/rs12101587
Valente, A., Sathyendranath, S., Brotas, V., Groom, S., Grant, M., Jackson, T., et al. (2022). A compilation of global bio-optical in situ data for ocean colour satellite applications – version three. Earth Syst. Sci. Data 14, 5737–5770. doi:10.5194/essd-14-5737-2022
Vanhellemont, Q., Brewin, R. J. W., Bresnahan, P. J., and Cyronak, T. (2022). Validation of Landsat 8 high resolution sea surface temperature using surfers. Estuar. Coast. Shelf Sci. 265, 107650. doi:10.1016/j.ecss.2021.107650
Wang, Z., and Costa, M. (2022). Autonomous shipborne in situ reflectance data in optically complex coastal waters: a case study of the Salish sea, Canada. Front. Remote Sens. 3. doi:10.3389/frsen.2022.867570
Wernand, M. R. (2002). “Guidelines for (ship bourne) auto-monitoring of coastal and ocean color,” in Ocean optics XVI. Editors S. Ackleson, and C. Trees (Santa Fe, NM, USA: Ocean).
Keywords: satellite, validation, ocean colour, superyacht, remote sensing
Citation: Brewin RJW, Sun X, Aurin DA, Viljoen JJ, Walsh C and Sathyendranath S (2024) Superyachts could support satellite ocean colour validation. Front. Remote Sens. 5:1336494. doi: 10.3389/frsen.2024.1336494
Received: 10 November 2023; Accepted: 08 January 2024;
Published: 24 January 2024.
Edited by:
Vittorio Ernesto Brando, National Research Council (CNR), ItalyReviewed by:
Tristan Harmel, Magellium, FranceCopyright © 2024 Brewin, Sun, Aurin, Viljoen, Walsh and Sathyendranath. This is an open-access article distributed under the terms of the Creative Commons Attribution License (CC BY). The use, distribution or reproduction in other forums is permitted, provided the original author(s) and the copyright owner(s) are credited and that the original publication in this journal is cited, in accordance with accepted academic practice. No use, distribution or reproduction is permitted which does not comply with these terms.
*Correspondence: Robert J. W. Brewin, ci5icmV3aW5AZXhldGVyLmFjLnVr