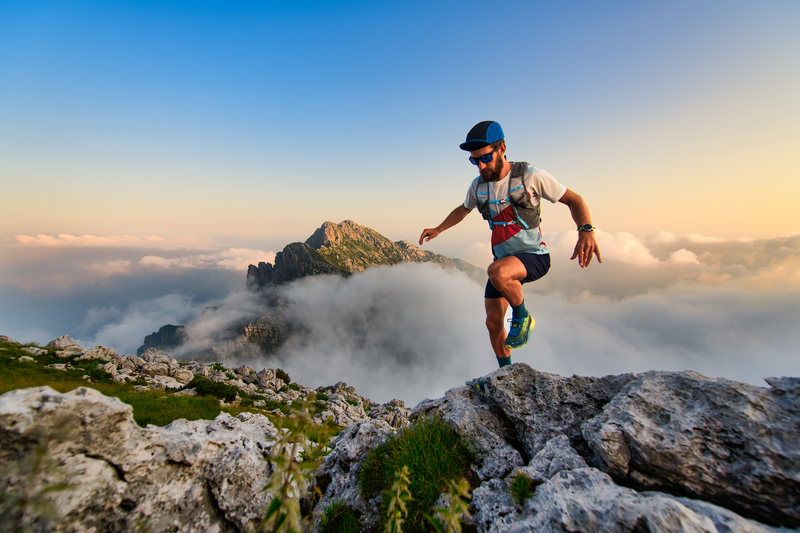
94% of researchers rate our articles as excellent or good
Learn more about the work of our research integrity team to safeguard the quality of each article we publish.
Find out more
MINI REVIEW article
Front. Remote Sens. , 14 March 2024
Sec. Terrestrial Carbon Cycle
Volume 5 - 2024 | https://doi.org/10.3389/frsen.2024.1332728
Tropical moist forests have been severely affected by natural and anthropogenic disturbances, leading to substantial changes in global carbon cycle and climate. These effects have received great attention in scientific research and debates. Here we review recent progress on drivers and ecological impacts of tropical moist forest disturbances, and their monitoring and modeling methods. Disturbances in tropical moist forests are primarily driven by clearcutting, selective logging, fire, extreme drought, and edge effects. Compound disturbances such as fire and edge effects aggravate degradation in the edge forests. Drought can result in terrestrial carbon loss via physiological impacts. These disturbances lead to direct carbon loss, biophysical warming and microclimate change. Remote sensing observations are promising for monitoring forest disturbances and revealing mechanisms, which will be useful for implementing disturbance processes in dynamic vegetation models. Yet, constrained spatiotemporal coverages and resolutions limit the application of these data in process-based models. It is also challenging to represent physical processes derived from fine-resolution remote sensing data in coarse-resolution models. We highlight the need to continuously integrate new datasets and physical processes in forest disturbance modeling to advance understanding of disturbance patterns and impacts. Interactions and impacts of climate change and anthropogenic activities should also be considered for modeling and assessing feedbacks of tropical moist forest disturbances.
Tropical moist forests are one of the world’s largest and most productive ecosystems and an important component of the global carbon cycle, containing 44% of the global aboveground biomass (Liu et al., 2015; Xu et al., 2021). Over the past decades, they have been affected by severe natural (e.g., drought, fire) and anthropogenic (e.g., deforestation, shifting cultivation, mining) disturbances, leading to substantial decreases in forest area, aboveground biomass, and soil carbon (Pugh et al., 2019a; Hansen et al., 2020; Pyles et al., 2022). Deforestation and forest degradation induced by these disturbances can destroy vegetation and reduce aboveground carbon stock, resulting in significant carbon emissions (Molinario et al., 2017; Wigneron et al., 2020; Ahmed et al., 2021). Nevertheless, carbon sequestration from post-disturbance ecosystem recovery remains limited in tropical moist forests at a regional scale due to soil carbon loss (Kleinschroth et al., 2015; Heinrich et al., 2023). Under intensifying climate change and human activities, disturbance-induced emissions will likely increase in the future (Dai, 2013; Li et al., 2017). Additionally, disturbances can harm vegetation health and vitality, lead to tree mortality, and threaten ecosystem services in the tropics (FAO, 2020). Studying disturbances in tropical moist forests is thus of great value in exploring physical mechanisms of disturbances, designing management and conservation policies, and mitigating climate change in the future.
Forest disturbances can cause forest loss and degradation. The extent, type, and impact of forest disturbances can be measured from field experiments, forest inventory data, and remote sensing observations. Field experiments such as the Biological Dynamics of Forest Fragments Project and the throughfall exclusion experiment were designed to study the effects of a particular disturbance type (Fisher et al., 2007; Laurance et al., 2011). Forest inventory data from the Food and Agriculture Organization of the United Nations (FAO) indicated a decrease in tropical deforestation rate from 15.8 Mha yr−1 during 1990–2000 to 10.2 Mha yr−1 during 2015–2020 (FAO, 2020). Recently, remote sensing data have been commonly used to investigate forest disturbances as multi-year products at moderate-to high-resolutions became available. For example, global forest loss data at 30 m resolution revealed that deforestation decreased in Amazon but increased in Indonesia during 2000–2012 (Hansen et al., 2013). Multi-year optical and microwave data also indicated that the total forest degradation area exceeded the deforested forests in the Amazon since the 1990s (Matricardi et al., 2020; Qin et al., 2021). However, there is still difficulty in monitoring forest degradation and its ecological impacts, preventing full understanding of its driving mechanisms and modeling development of the physical processes.
This review aims to present key findings from recent studies about the magnitudes, mechanisms, and impacts of disturbances in tropical moist forests. We first summarized the disturbances and their underlying drivers that lead to deforestation and degradation in pantropical moist forests, specifically focusing on the physical processes and mechanisms. We then evaluated the impacts of the disturbances on the carbon cycle, surface energy budget, and microclimate. We further discussed the data and modeling needs for studying these disturbances. In particular, we emphasized the integration of remote sensing data for representing related processes in dynamic global vegetation models (DGVMs).
In ecology, disturbance is commonly defined as regular or irregular events that disrupt the structure of an ecosystem, community, or population and alter the physical environment or resource availability (White and Pickett, 1985). Here we focus on the most common disturbance types in tropical moist forests (Figure 1). For anthropogenic types, we consider clear-cutting, selective logging, forestry, shifting agriculture (and related fire use), and edge effects induced by forest fragmentation. Wildfire and extreme drought are the major natural disturbance types reviewed in this article. Driven by natural climate variability and anthropogenic activities, forest disturbances typically result in substantial deforestation and forest degradation in the tropics. Deforestation refers to the transition of forests to other land cover types, such as forest clear-cutting for cropland (Curtis et al., 2018). Unlike deforestation, forest degradation leads to a loss of forest attributes (e.g., canopy cover, biomass) and a decrease in ecosystem functioning and services (e.g., carbon sequestration, soil protection, biodiversity), but the land cover type remains unchanged (Ghazoul et al., 2015; Zhu et al., 2023).
FIGURE 1. Types, underlying drivers, and biogeochemical and biophysical impacts of major disturbances in pantropical moist forests discussed in this review. The dashed arrows highlight potential interactions of compound disturbances. Forestry is included as a driver to represent natural forest loss induced by forestry commodities or plantations (e.g., oil palm, rubber). Here ET refers to evapotranspiration.
Natural and anthropogenic drivers of forest disturbances vary across the tropics (Andoh et al., 2022). Long-term and permanent deforestation is typically caused by commodity production, urbanization, and mining, with forests converted into other land cover types such as agriculture, plantation, or infrastructure (Curtis et al., 2018; Giljum et al., 2022). Meanwhile, short-term forest loss is commonly associated with shifting cultivation, forestry, and fire. Forest degradation is mainly caused by forest fragmentation resulting from deforestation, forestry, overgrazing, uncontrolled fire, and extreme climatic events like drought (Kissinger et al., 2012; Zhu et al., 2023).
In Africa, increasing food and energy demands due to socioeconomic development and rapid population growth lead to deforestation and degradation (Rudel, 2013; Heinimann et al., 2017; Curtis et al., 2018; Tyukavina et al., 2018). Two major types of human activities have resulted in substantial forest loss: 1) widespread selective logging and the following constructions of roads and log landings (Kleinschroth et al., 2016; Umunay et al., 2019) and 2) fires for forest clearing induced by shifting cultivation or land management (Barlow and Peres, 2008; Dwomoh et al., 2019; Zubkova et al., 2019). Impacts of industrial mining in Africa are relatively minor except for Ghana, where direct forest loss from mining was 213 km2 during 2000–2019. Charcoal collection as a local energy resource is another driver of deforestation and degradation in low-income regions (Balomba et al., 2018). In addition to human activities, extreme floods, droughts, and megaherbivores (e.g., elephant, hippo, rhino) have led to forest damages and become common natural disturbances in Africa in recent years (Wieczkowski, 2009; Parolin and Wittmann, 2010; Cromsigt and te Beest, 2014; Schmitz et al., 2018; Xu et al., 2019; Hyvarinen et al., 2021).
Commodity-driven clear cutting is a main driver of deforestation in South America. Lost forest area was likely converted to cropland or pastureland (Curtis et al., 2018). Over the past 2 decades, massive cropland (e.g., soybean, corn, sugarcane) expansion strongly drive deforestation in Brazil (Zalles et al., 2019; Song et al., 2021). Between 2005 and 2015, mining also led to 9% of forest loss directly and indirectly in Brazilian Amazon (Sonter et al., 2017). Edge effects from deforestation-driven fragmentation, fire, and extreme droughts are the main drivers of forest degradation in South America (Lapola et al., 2023). In particular, about 60% of the degraded forests in the Brazilian Amazon can be attributed to edge areas and isolated fragments of forests from 1992 to 2014 (Matricardi et al., 2020). During this period, a notable increasing fraction of the degraded forests is from the logged and burned area. The humid forests in South America have also suffered from severe droughts that lead to tree mortality and threaten the carbon sink (Lapola et al., 2023). Additionally, flooding has become a new disturbance agent that leads to tree mortality and decreased resilience in Amazon forests (Resende et al., 2019; Boulton et al., 2022; Bredin et al., 2022).
Southeast Asia (SEA) has one of the highest rates of deforestation and biodiversity loss globally (Felbab-Brown, 2013), primarily due to human activities such as palm oil production, agriculture, and logging (Wilcove et al., 2013; Curtis et al., 2018; Zeng et al., 2018). Oil palm and rubber plantation expansion are the principal drivers of deforestation in the insular SEA and mainland SEA, respectively (Jamaludin et al., 2022). Land use for oil palm plantations has quadrupled since 1980, and approximately 50% of oil palm expansion occurs in the forests in Indonesia and Malaysia (Koh and Wilcove, 2008). Over 2001–2015, oil palm plantations led to about 50.2 TgC yr−1 biomass loss in these two countries (Xu et al., 2022). Forests with high biomass density were particularly encroached by small-scale plantations after 2007 (Xu et al., 2022). Additionally, Indonesia has experienced 1,901 km2 area of deforestation directly from industrial mining, which is the most affected country in the tropics (Giljum et al., 2022).
The natural and anthropogenic drivers of deforestation and forest degradation affect tropical moist forests through various physical processes. Compared to deforestation, forest degradation involves more complex processes and mechanisms (Bullock et al., 2020a; Pandey et al., 2020). In recent decades, small-scale deforestation (e.g., shifting cultivation and road construction) has led to severe forest fragmentation and a large amount of edge forests (Taubert et al., 2018; Umunay et al., 2019). Edge forests become degraded over time through edge effects: local circulation transfers moist air in edge forests to adjacent non-forest areas, resulting in higher temperature, stronger wind, and more severe drought in edge forests. Influences of edge effects can extend from forest patch edges to interior forests by about 110 m in tropical moist regions, and the forests experiencing the most severe degradation are typically located near the edges of forest patches (Broadbent et al., 2008; Laurance, 2008).
Fire is also an important driver of degradation in tropical moist forests, where trees are typically not resistant to fires. Compared with forests that have never suffered a fire, burned forests show reduced biomass and divergent vertical structures dominated by pioneers and saplings (Barlow and Peres, 2008). A positive feedback loop may exist between fire and edge effects in Africa: in edge forests with low humidity, it is easy for fires to intrude and burn the forests; fires also enhance the degradation of edges in tropical moist forests (Dwomoh et al., 2019; Zhao et al., 2021). However, under severe fires, tropical forests could experience substantial tree cover loss, become prone to burning, and eventually transform into savannas (D’Onofrio et al., 2018; Hoffmann et al., 2012). Fires can further interact with forest edge effects via two possible mechanisms: directly burning into forest patches and indirectly enhancing local circulation between edge forests and non-forest areas (Zhao et al., 2021). Such interactions of these compound disturbances will further worsen the degradation of edge forests in the tropics.
Widespread drought directly reduces terrestrial carbon storage via physiological impacts (Reichstein et al., 2013; Xu et al., 2019). Drought-driven soil water depletion and heat stress cause water deficit for plant uptake and affect tree growth and photosynthesis. Tree mortality induced by extreme drought then reduces terrestrial carbon sink (Brienen et al., 2015). During the 2010 drought, the Amazonian rainforest lost about 2.2 PgC carbon, even reversing it from a carbon sink to a source (Phillips et al., 2009; Lewis et al., 2011). Drought also has complex impacts on vegetation evapotranspiration. Decreased soil moisture and transpiration from stomatal closure under drought typically lead to suppressed evapotranspiration in tropical moist forests (Corlett, 2016). On the other hand, increased atmospheric moisture demand under drought may promote evapotranspiration, which can cause flash droughts and exert ecosystem stresses (Zhao et al., 2022). Additionally, drought indirectly impacts tropical forests by increasing the frequency and intensity of other disturbances, such as fire and edge effects (Staal et al., 2020; Numata et al., 2021). With more defoliation and litter accumulation, and drier vegetation components, it increases the vulnerability of moist forests to fires in Amazon (Asner and Alencar, 2010; Brando et al., 2014). The long-lasting legacy effects of drought cause continuous carbon loss and accelerate widespread forest degradation (Bullock et al., 2020a; Qin et al., 2021).
Disturbances substantially impact forest structure, vegetation composition, and biogeochemical cycles (carbon, nitrogen, phosphorus, etc.) in tropical moist forests (Ciais, 2013; Longo et al., 2020). Specifically, they cause direct loss of carbon storage via emissions and affect carbon sequestration capability, which may change the ecosystems from carbon sinks to sources (McLauchlan et al., 2014; Kranabetter et al., 2016). The changes in forest structure and carbon cycle, in return, can affect ecosystem resilience and disturbance regime. Here we only focus on the disturbance impacts on the carbon cycle in tropical moist forests. According to satellite data, carbon loss due to tropical deforestation was about 0.57–1.22 PgC yr−1 during 2000–2005, and South America, Africa, and Asia accounted for 54%, 32%, and 14% of the total loss, respectively (Harris et al., 2012). Another study found a similar magnitude of carbon loss (0.60–1.24 PgC yr−1) in the 2010s by integrating field samples and satellite imagery (Achard et al., 2014). In comparison, estimations based on forest inventory data and a bookkeeping model delivered a considerably higher carbon loss of 2.94 PgC yr−1 during 1990–2007 from tropical deforestation (Pan et al., 2011). This difference is likely because forest inventory data are often collected from undisturbed forests with higher carbon densities. Also, bookkeeping models ignore natural disturbances and the impacts of climate and CO2 on biomass, likely causing different results from satellite-based analyses. Compared to deforestation, carbon loss from forest degradation is relatively difficult to quantify since large-scale degradation monitoring is challenging. With commonly used data fusion methods and bookkeeping models, degradation-induced carbon loss was estimated to be 32%–69% of the total amount in tropical forests (Asner et al., 2010a; Baccini et al., 2017).
Tropical forest disturbances also result in biophysical warming by altering the surface energy budget. Deforested and degraded areas in tropical moist forests generally have lower evapotranspiration and surface roughness, thus having higher local surface temperature than the undisturbed forests (Li et al., 2015; Chen et al., 2020; Zhu et al., 2023). Although the albedo of deforested areas is higher than that of intact forests, the resultant cooling effect is more obvious in high-latitude regions with snow cover in winter (Lee et al., 2011; Peng et al., 2014). However, this effect is non-negligible in the tropics: complete tree cover loss from deforestation will increase the local land surface temperature by about 1.53°C from biophysical impacts (Alkama and Cescatti, 2016). In tropical moist forests, degradation could have a daytime warming effect of 0.78°C on the local land surface temperature, which is about 18% of that from deforestation (Zhu et al., 2023). Although the biophysical impacts of forest disturbances were found equally important as the biogeochemical effects, the underlying mechanisms are still unclear, and the biophysical effects are commonly overlooked by climate mitigation policies (Bala et al., 2007; Windisch et al., 2021).
Moreover, disturbance-induced deforestation and degradation can modify vegetation structure, species density, and distance to forest edge, leading to changes in microclimate conditions in tropical moist forests. Functioning as thermal insulators, forest canopies can adjust understory temperature and constrain extreme values of the microclimate (Jucker et al., 2018). Post-disturbance canopy loss drives temperature increase and air desiccation locally. In Malaysia, understory air temperatures in oil palm plantations and logged forests were about 6.5°C and 2.5°C warmer than those in primary forests (Hardwick et al., 2015). In addition to modifying forest structure, fragmentation in Amazonian rainforests can elevate tree-community dynamics (mortality, damage, turnover) near forest edges, further exacerbating edge effects on disturbance-sensitive species (Laurance et al., 1998; Ewers and Banks-Leite, 2013). Such impacts on microclimate can substantially affect the carbon balance, ecosystem functioning, and biodiversity in tropical moist forests (Vourlitis et al., 2004; Jucker et al., 2020; Sanczuk et al., 2023). Warmer and drier microclimate and leaf area reduction after deforestation may potentially convert transitional forests between tropical moist forests and savanna in Brazilian Amazon to be CO2 sources in the future, by affecting forest respiration and canopy photosynthesis (Vourlitis et al., 2004). During extreme drought, the disruption of microclimate effects further intensifies forest mortality, impact evapotranspiration, and trigger a positive feedback loop that accelerates climate change (Au et al., 2022).
In recent years, compound disturbances have become an emerging issue for the degraded forests in the tropics. They exert multiplicative impacts on forest ecosystems from two or more combined disturbances, often by affecting the resilience to the subsequent disturbance during the recovery processes (Buma, 2015; Kleinman et al., 2019). Remote sensing observations reveal that the interactions between fire and edge effects aggravate degradation in the edge forests across Africa through fire intruding into forests (direct impact) and enhanced local atmospheric circulations (indirect impact) (Zhao et al., 2021). The aggravated degradation is mainly controlled by the direct impact in dry forests but mutually by both the direct and indirect impact in moist forests (Zhao et al., 2021). In Brazilian Amazon, the 2015 drought has led to increased fire occurrence in the degraded forests, resulting in substantial carbon emissions (Aragão et al., 2018). Compound disturbances from repeated fire, drought, and windstorms also result in prolonged forest degradation by impeding the recovery of carbon stocks and tree cover in southeast Amazon, according to multi-year field measurements (Brando et al., 2019). Nevertheless, our understanding of the ecological impacts from compound disturbances is still limited compared to individual disturbances (Graham et al., 2021).
Recovery of secondary and degraded forests after disturbances is critical in ecosystem restoration, biodiversity conservation, and climate mitigation (Anderson-Teixeira et al., 2013; Poorter et al., 2021). Secondary forests (
Forest recovery and resilience to disturbances can be quantified with forest attributes from multiple aspects, including soil properties, plant functions, and forest structures (Walker et al., 2010; Martin et al., 2013; Jamaludin et al., 2022). Chronosequence studies are the major approach to exploring forest recovery and resilience by reestablishing fire disturbance history and tracking the long-term forest properties (Cole et al., 2014; Poorter et al., 2016; Poorter et al., 2021). Site observations over the past 2 decades suggest high resilience of tropical forests to low-intensity land use change, with the forest attributes recovering to 78% of the old-growth states over 20 years (Poorter et al., 2021). In particular, biomass and species composition tend to recover much more slowly than other forest components, including structure, plant functioning, and soil. Recently, finer resolution and frequent satellite products provided a new opportunity for spatially-explicit representation of the recovery pace using multi-temporal observations (Wigneron et al., 2020; Xu et al., 2021) or a space-for-time method (Heinrich et al., 2021; Heinrich et al., 2023).
Recent studies have identified non-structural carbohydrates (NSC) as a crucial characteristic that controls the carbon balance and hydraulic processes of trees, further affecting forest resistance and resilience to disturbances (Hartmann and Trumbore, 2016; Fatichi et al., 2019). Its allocation strategies influence the growth and mortality of trees (Blumstein et al., 2022). For example, allocation to light-harvesting organs enables sufficient photosynthate, whereas more NSC allocation to storage can improve survivorship by providing a buffer against resource shortage when suffering disturbances but at the expense of growth. Conservative-growing species with larger NSC reserves and structural carbon inputs, therefore, are more likely to survive during extreme climatic events (Piper and Paula, 2020; DAndrea et al., 2021). Moreover, to maintain respiration and other metabolic functions, plants may eventually deplete stored NSC during the long-term disturbances (e.g., drought), resulting in increased mortality risk (Doughty et al., 2015).
Satellite remote sensing is a primary tool to continuously monitor forest disturbances and quantify their impacts at a large scale in forest management and conservation practices (Lechner et al., 2020). Passive optical and microwave data have been widely adopted to map disturbances by tracking temporal changes in spectral information (Hirschmugl et al., 2017). Active sensors like Light Detection and Ranging (LiDAR) or synthetic aperture radar (SAR) provide high-quality data for assessing forest structure, canopy composition, and carbon sequestration potential (Mitchell et al., 2017).
However, limitations exist in forest disturbance research with remote sensing data. First, applications of such data are largely constrained by their spatiotemporal resolutions and coverages. Until recently, detecting small-scale disturbances or individual tree-level changes at a continental scale became possible by applying artificial intelligence (AI) to very high resolution (VHR) imagery (Brandt et al., 2020; Li et al., 2023). Yet, the low temporal resolution of the VHR data limits long-term monitoring of disturbances with time series analysis (Wang et al., 2019; Hethcoat et al., 2021). The effectiveness of optical products is also constrained by frequent cloud cover in the tropics (Hethcoat et al., 2021). Second, it is challenging to determine the causes of the disturbances and differentiate between natural or anthropogenic events purely based on remote sensing data (Stahl et al., 2023) such as lightning- or human-ignited fires. Third, primary and secondary forests may respond differently to disturbances regarding carbon sequestration abilities and ecological services (Gurevitch, 2022; Hua et al., 2022). Distinguishing their distributions and responses to disturbances is challenging at a large scale due to the complex information from heterogeneous canopy structures, species compositions, and within-canopy shadows (da Silva et al., 2014). Additionally, gradual forest changes induced by degradation may not be easily detectable using typical remote sensing techniques, making it difficult to monitor the legacy effects of degradation (Gao et al., 2020).
Satellite-based sensors have been newly launched or planned for data collection in recent years (Hwang et al., 2023). The combination of new optical, LiDAR, and SAR data has the potential to improve the characterization of forest disturbances and enhance our understanding of forest responses to disturbances. For example, observations from Global Ecosystem Dynamics Investigation (GEDI), the first spaceborne LiDAR sensor for monitoring global ecosystem structure, are utilized to quantify canopy structure and biomass and support monitoring of disturbances and their impacts on the carbon cycle (Dubayah et al., 2020). Though the GEDI data itself has limited spatiotemporal coverage, its integration with optical sensors (e.g., Landsat, Sentinel-2) provides wall-to-wall mapping of additional forest structure metrics (Potapov et al., 2021; Dwiputra et al., 2023). European Space Agency plans to launch the new “biomass” satellite carrying P-band SAR in 2024, which can capture the forest structure and help improve biomass and canopy height measurement. Meanwhile, newly developed products based on existing satellite data provide insights into disturbances and their impacts (Brandt et al., 2018; Fan et al., 2019; Xu et al., 2021; Santoro et al., 2021). Very promising AI approaches have been developed very recently, bypassing the problems of optical data saturation, by analyzing image texture in all spectral bands. Integrated with deep learning algorithms, recent studies have identified a surprisingly high quantity of trees and developed new benchmark datasets for tree cover and biomass in Africa utilizing multispectral VHR imagery from DigitalGlobe satellites (Brandt et al., 2020; Mugabowindekwe et al., 2023).
Development of remote sensing data can substantially support model development of tropical forest disturbances in several aspects. First, a major usage of remote sensing in DGVMs is to provide plenty of high-quality data for calibration and validation. Compared to field observations, remote sensing provides a wealth of continuous data in space and time. Products of aboveground biomass, gross primary productivity (GPP), leaf area index (LAI), and burned area have been widely adopted for calibrating and evaluating important model ouputs (Jung et al., 2011; Santoro et al., 2021). Second, satellite-based observations provide key forcing data for model simulation. Vegetation and land cover data provide plant functional type (PFT) maps and spatiotemporal deforestation information for DGVMs (Li et al., 2018). Burned area products are also critical for forcing historical forest disturbances induced by wildfires (Yue et al., 2014). Nevertheless, current application of remote sensing-based forcing data is limited to the contemporary period. Backcasting data before the satellite period can be improved by historical reconstruction (e.g., fire activity) based on contemporary remote sensing observations (Mouillot and Field, 2005; Yang et al., 2014) and machine learning methods. Third, abundant remote sensing data can be used to derive quantitative representations of physical and ecological processes related to forest disturbances (Figure 2). Currently terrestrial ecosystem models typically have very few representations of forest disturbances developed from limited field or experimental data for deriving the processes. With large spatial-temporal coverage, remote sensing data can summarize the general principles of the physical processes and thus improve the modeling capability of forest disturbance and the following recovery in DGVMs. For example, moderate-resolution (30 m) forest cover maps developed from Landsat data have been utilized to derive forest fragmentation metrics and analyze the fragmentation dynamics across the tropics (Taubert et al., 2018; Fischer et al., 2021). These results were further used to optimize the fragmentation model FRAG-B for simulating future patterns of tropical moist forests under different scenarios (Fischer et al., 2021).
FIGURE 2. Remote sensing data that support the development of major forest disturbance modules in DGVMs. Here PFT, GPP, and LAI refer to plant functional type, gross primary productivity, and leaf area index, respectively. Each line represents a forest disturbance module developed in existing DGVMs. Each square represents a certain variable with satellite-based products that have been adopted in disturbance modeling.
Oil palm plantation is a typical example of deforestation and forest degradation in Southeast Asia (Vijay et al., 2016). Oil palm modeling has been implemented in DGVMs to simulate the carbon and climate impacts of land use change induced by oil palm expansion (Fan et al., 2015; Xu et al., 2021). For example, Xu et al. (2021) introduced an oil palm module in ORCHIDEE with specific morphology, phenology, and harvest processes for oil palm. They utilized 100 m global oil palm plantation maps developed from moderate-resolution (25 m) PALSAR observations to generate PFT forcing data integrating oil palm distribution. Satellite-observed GPP values were also used in model calibration due to the lack of site observations (Xu et al., 2021). Fan et al. (2019) reconciled a canopy interception scheme of oil palm into the Community Land Model. They found 18%–27% higher transpiration and 15%–20% higher evapotranspiration in oil palm plantations than in tropical forests. By monitoring oil palm plantations at higher spatial resolution (e.g., 10 m), it is possible to improve the simulation results by distinguishing industrial and small-holder oil palm plantations with different biophysical impacts (Meijide et al., 2017). The availability of high-resolution remote sensing products will thus improve our scientific understanding and modeling capability of tropical moist forest disturbances.
However, applying medium- and high-resolution (e.g., 30 m) remote sensing data for applications in coarse-resolution (typically 0.5°) DGVMs is challenging due to the mismatch in spatial resolutions and lack of sub-grid processes. Representing physical processes of small-scale disturbances in these models may lose substantial spatial details. One common solution is to resample the remote sensing data to the resolution of DGVMs. Yet, a lot of information from remote sensing data is lost. Another potential reason is the lack of sub-grid processes like forest demography representation in these models (Yue et al., 2018). Have proposed adding sub-grid variables and processes for estimating forest age cohorts to simulate net and gross land use change in a DGVM (ORCHIDEE). Therefore, it is necessary to address how to upscale variables and mechanisms derived from remote sensing data for applications in DGVMs. More work is needed to integrate sub-grid information in modeling small-scale forest disturbances such as degradation from forest fragmentation.
Forest disturbance monitoring in the tropics can be improved in several aspects with the development of remote sensing. Near real-time monitoring can speed up the detection of forest disturbances and is now promising through the integration of multi-source time series data or VHR imagery. For example, the fusion of optical and SAR data from Landsat, Sentinel-2, and Sentinel-1 data has been found to be fast and effective in capturing tree losses in Amazon Basin (Tang et al., 2023). With a revisited time of 1-day, the VHR imagery (3 m resolution) from the PlanetScope nano-satellite constellation shows its capability to quickly identify forest disturbance activites (Francini et al., 2020). Active fire detections from the Visible Infrared Imaging Radiometer Suite (VIIRS) also support near real-time tracking and attribution of fire activities in the Amazon forests (Andela et al., 2022). Additionally, compared to abrupt forest loss, monitoring gradual forest changes due to degradation and recovery is still challenging due to their weak spectral signals (Gao et al., 2020). Spectral unmixing analysis with dense time series Landsat data has the capability to detect subtle forest changes from degradation in tropical rainforests in Rondonia, Brazil (Bullock et al., 2020b). More efforts should be made to develop suitable methods to accurately quantifying degradation extent at a large spatial scale. Monitoring forest disturbances with state-of-the-art datasets and methods can support the development of early warning systems for future research and decision-making.
Future work on developing satellite data products and physical processes is also critical to improve the modeling capability of tropical forest disturbances and enhance our understanding of the related mechanisms. Classification of forest disturbance agents at a large spatiotemporal scale is a prerequisite for developing disturbance models and quantifying ecological impacts. Though existing work has elaborated on classifying drivers of tree cover loss across the pantropical regions (Curtis et al., 2018; Laso Bayas et al., 2022), types and distributions of degradation drivers remain unknown. Continuous development of additional forcing data for contemporary and historical periods will also benefit disturbance modeling in DGVMs. Integrating NSC-related processes in DGVMs, such as species-based carbon allocation strategies and interactions between NSC, mortality and drought, may help address whether plants die of carbon starvation or hydraulic failure and support future projection of forest resilience under disturbances (Fatichi et al., 2019). Development of these processes also requires more field observations to understand their seasonal variations and unravel the underlying mechanisms of NSC. Moreover, although the compound disturbances may become more prevalent under climate change, explicit modeling of compound disturbances and forest degradation processes are still lacking in existing DGVMs.
Climate change has significantly altered the spatiotemporal patterns of extreme climatic events, affecting forest disturbances in the tropics. In recent decades, increasing frequency, severity, and duration of fire weather and drought have led to substantial carbon emissions in tropical moist forests (Dai, 2013; Jones et al., 2022). On the other hand, increasing atmospheric CO2 may promote vegetation regrowth and forest recovery in the tropics (i.e., fertilization effects) via increases in water-use efficiency or biomass production, and contribute to carbon uptakes (Walker et al., 2021). Forests also respond divergently to extreme climatic events and fertilization effects as they differ in tree functional traits and species compositions (Anderegg et al., 2020). Tropical forest degradation has induced non-negligible biogeochemical and biophysical feedbacks on climate (Li et al., 2015; Liu et al., 2019; Zhu et al., 2023). Thus, future impacts of climate change and natural disturbances on tropical moist forests are still uncertain and should be further explored.
Moreover, anthropogenic disturbances in tropical moist forests are mainly driven by socioeconomic conditions and forest management policies (Rudel, 2013; Archibald, 2016; Zubkova et al., 2019). Combined with climate warming, human-induced deforestation (e.g., intensive agriculture, industrial logging) will severely degrade the tropical moist forests and reduce the resilience of vegetation and biodiversity in the future (Gardner et al., 2009; Asner et al., 2010b; Lewis et al., 2015). Over 2001–2018, such disturbances have led to more fragmented landscapes in tropical moist forests, with higher accessibility for further resource extraction (Hansen et al., 2020). Additional deforestation will severely increase the total number of forest fragments by 2050 (Taubert et al., 2018). Under a low mitigation scenario, burned area in Amazon forests is also likely to increase by 4–28 times by 2,100 (Le Page et al., 2017). Preventing new deforestation in Amazonia could reduce fire emissions by 36%–58% and avoid escaped fires into protected forests (Brando et al., 2020). Nevertheless, forest restoration and protection have the capability to mitigate these consequences and accelerate the carbon recovery in the tropics (Philipson et al., 2020; Koch and Kaplan, 2022). Social-economic development scenarios should also be incorporated in future research to understand human-climate interactions and their impacts on forest disturbances.
JH: Formal Analysis, Investigation, Resources, Visualization, Writing–original draft. WL: Conceptualization, Funding acquisition, Supervision, Writing–review and editing. ZZ: Formal Analysis, Investigation, Resources, Writing–original draft. LZ: Formal Analysis, Investigation, Resources, Writing–original draft. XD: Formal Analysis, Investigation, Resources, Writing–original draft. YX: Formal Analysis, Investigation, Resources, Writing–original draft. MS: Formal Analysis, Investigation, Resources, Writing–original draft. JZ: Formal Analysis, Investigation, Resources, Writing–original draft. PC: Writing–review and editing. J-PW: Writing–review and editing. RL: Writing–review and editing. GL: Writing–review and editing. LF: Writing–review and editing.
The author(s) declare financial support was received for the research, authorship, and/or publication of this article. This study was funded by the Yunnan Provincial Science and Technology Project at Southwest United Graduate School (grant number: 202302AO370001), the Hainan Institute of National Park grant (grant number: KY-23ZK01), the National Key R&D Program of China (grant number: 2019YFA0606604), and the National Natural Science Foundation of China (grant numbers: 72348001 and 42175169). This work is a contribution to the CALIPSO (Carbon Loss In Plants, Soils and Oceans) project supported by the Schmidt Sciences.
The authors declare that the research was conducted in the absence of any commercial or financial relationships that could be construed as a potential conflict of interest.
The author(s) declared that they were an editorial board member of Frontiers, at the time of submission. This had no impact on the peer review process and the final decision.
All claims expressed in this article are solely those of the authors and do not necessarily represent those of their affiliated organizations, or those of the publisher, the editors and the reviewers. Any product that may be evaluated in this article, or claim that may be made by its manufacturer, is not guaranteed or endorsed by the publisher.
Achard, F., Beuchle, R., Mayaux, P., Stibig, H., Bodart, C., Brink, A., et al. (2014). Determination of tropical deforestation rates and related carbon losses from 1990 to 2010. Glob. Change Biol. 20, 2540–2554. doi:10.1111/gcb.12605
Ahmed, A. I., Bryant, R. G., and Edwards, D. P. (2021). Where are mines located in sub Saharan Africa and how have they expanded overtime? Land Degrad. Dev. 32, 112–122. doi:10.1002/ldr.3706
Alkama, R., and Cescatti, A. (2016). Biophysical climate impacts of recent changes in global forest cover. Science 351, 600–604. doi:10.1126/science.aac8083
Andela, N., Morton, D. C., Schroeder, W., Chen, Y., Brando, P. M., and Randerson, J. T. (2022). Tracking and classifying Amazon fire events in near real time. Sci. Adv. 8, eabd2713. doi:10.1126/sciadv.abd2713
Anderegg, W. R. L., Trugman, A. T., Badgley, G., Konings, A. G., and Shaw, J. (2020). Divergent forest sensitivity to repeated extreme droughts. Nat. Clim. Change 10, 1091–1095. doi:10.1038/s41558-020-00919-1
Anderson-Teixeira, K. J., Miller, A. D., Mohan, J. E., Hudiburg, T. W., Duval, B. D., and DeLucia, E. H. (2013). Altered dynamics of forest recovery under a changing climate. Glob. Change Biol. 19, 2001–2021. doi:10.1111/gcb.12194
Andoh, J., Oduro, K. A., Park, J., and Lee, Y. (2022). Towards REDD+ implementation: deforestation and forest degradation drivers, REDD+ financing, and readiness activities in participant countries. Front. For. Glob. Change 5, 957550. doi:10.3389/ffgc.2022.957550
Aragão, L. E. O. C., Anderson, L. O., Fonseca, M. G., Rosan, T. M., Vedovato, L. B., Wagner, F. H., et al. (2018). 21st Century drought-related fires counteract the decline of Amazon deforestation carbon emissions. Nat. Commun. 9, 536. doi:10.1038/s41467-017-02771-y
Archibald, S. (2016). Managing the human component of fire regimes: lessons from Africa. Philosophical Trans. R. Soc. B Biol. Sci. 371, 20150346. doi:10.1098/rstb.2015.0346
Asner, G. P., and Alencar, A. (2010). Drought impacts on the Amazon forest: the remote sensing perspective. New Phytol. 187, 569–578. doi:10.1111/j.1469-8137.2010.03310.x
Asner, G. P., Loarie, S. R., and Heyder, U. (2010b). Combined effects of climate and land-use change on the future of humid tropical forests. Conserv. Lett. 3, 395–403. doi:10.1111/j.1755-263x.2010.00133.x
Asner, G. P., Powell, G. V. N., Mascaro, J., Knapp, D. E., Clark, J. K., Jacobson, J., et al. (2010a). High-resolution forest carbon stocks and emissions in the Amazon. Proc. Natl. Acad. Sci. 107, 16738–16742. doi:10.1073/pnas.1004875107
Au, T. F., Maxwell, J. T., Robeson, S. M., Li, J., Siani, S. M. O., Novick, K. A., et al. (2022). Younger trees in the upper canopy are more sensitive but also more resilient to drought. Nat. Clim. Change 12, 1168–1174. doi:10.1038/s41558-022-01528-w
Baccini, A., Walker, W., Carvalho, L., Farina, M., Sulla-Menashe, D., and Houghton, R. A. (2017). Tropical forests are a net carbon source based on aboveground measurements of gain and loss. Science 358, 230–234. doi:10.1126/science.aam5962
Bala, G., Caldeira, K., Wickett, M., Phillips, T. J., Lobell, D. B., Delire, C., et al. (2007). Combined climate and carbon-cycle effects of large-scale deforestation. Proc. Natl. Acad. Sci. 104, 6550–6555. doi:10.1073/pnas.0608998104
Balomba, P., Nsakala, H., and Bokombola, P. (2018). Forests, exploitation and consumption of wood energy in the democratic republic of the Congo: case of kinshasa, kwango and kongo central provinces. Tropicultura 36, 553–564.
Barlow, J., and Peres, C. A. (2008). Fire-mediated dieback and compositional cascade in an Amazonian forest. Philosophical Trans. R. Soc. B Biol. Sci. 363, 1787–1794. doi:10.1098/rstb.2007.0013
Bennett, A. C., Rodrigues de Sousa, T., Monteagudo-Mendoza, A., Esquivel-Muelbert, A., Morandi, P. S., Coelho de Souza, F., et al. (2023). Sensitivity of South American tropical forests to an extreme climate anomaly. Nat. Clim. Change 13, 967–974. doi:10.1038/s41558-023-01776-4
Blumstein, M., Sala, A., Weston, D. J., Holbrook, N. M., and Hopkins, R. (2022). Plant carbohydrate storage: intra- and inter-specific trade-offs reveal a major life history trait. New Phytol. 235, 2211–2222. doi:10.1111/nph.18213
Boulton, C. A., Lenton, T. M., and Boers, N. (2022). Pronounced loss of Amazon rainforest resilience since the early 2000s. Nat. Clim. Change 12, 271–278. doi:10.1038/s41558-022-01287-8
Brando, P. M., Balch, J. K., Nepstad, D. C., Morton, D. C., Putz, F. E., Coe, M. T., et al. (2014). Abrupt increases in Amazonian tree mortality due to drought–fire interactions. Proc. Natl. Acad. Sci. 111, 6347–6352. doi:10.1073/pnas.1305499111
Brando, P. M., Silvério, D., Maracahipes-Santos, L., Oliveira-Santos, C., Levick, S. R., Coe, M. T., et al. (2019). Prolonged tropical forest degradation due to compounding disturbances: implications for CO2 and H2O fluxes. Glob. Change Biol. 25, 2855–2868. doi:10.1111/gcb.14659
Brando, P. M., Soares-Filho, B., Rodrigues, L., Assunção, A., Morton, D., Tuchschneider, D., et al. (2020). The gathering firestorm in southern Amazonia. Sci. Adv. 6, eaay1632. doi:10.1126/sciadv.aay1632
Brandt, M., Rasmussen, K., Hiernaux, P., Herrmann, S., Tucker, C. J., Tong, X., et al. (2018). Reduction of tree cover in West African woodlands and promotion in semi-arid farmlands. Nat. Geosci. 11, 328–333. doi:10.1038/s41561-018-0092-x
Brandt, M., Tucker, C. J., Kariryaa, A., Rasmussen, K., Abel, C., Small, J., et al. (2020). An unexpectedly large count of trees in the West African Sahara and Sahel. Nature 587, 78–82. doi:10.1038/s41586-020-2824-5
Bredin, Y. K., Hess, L. L., Scabin, A. B., Dunthorn, M., Haugaasen, T., Peres, C. A., et al. (2022). Above- and below-ground biodiversity responses to the prolonged flood pulse in central-western Amazonia, Brazil. Environ. DNA 4, 533–548. doi:10.1002/edn3.268
Brienen, R. J. W., Phillips, O. L., Feldpausch, T. R., Gloor, E., Baker, T. R., Lloyd, J., et al. (2015). Long-term decline of the Amazon carbon sink. Nature 519, 344–348. doi:10.1038/nature14283
Broadbent, E. N., Asner, G. P., Keller, M., Knapp, D. E., Oliveira, P. J., and Silva, J. N. (2008). Forest fragmentation and edge effects from deforestation and selective logging in the Brazilian Amazon. Biol. Conserv. 141, 1745–1757. doi:10.1016/j.biocon.2008.04.024
Bullock, E. L., Woodcock, C. E., and Olofsson, P. (2020b). Monitoring tropical forest degradation using spectral unmixing and Landsat time series analysis. Remote Sens. Environ. 238, 110968. doi:10.1016/j.rse.2018.11.011
Bullock, E. L., Woodcock, C. E., Souza, C., and Olofsson, P. (2020a). Satellite-based estimates reveal widespread forest degradation in the Amazon. Glob. Change Biol. 26, 2956–2969. doi:10.1111/gcb.15029
Buma, B. (2015). Disturbance interactions: characterization, prediction, and the potential for cascading effects. Ecosphere 6, 1–15. doi:10.1890/ES15-00058.1
Chazdon, R. L., Broadbent, E. N., Rozendaal, D. M. A., Bongers, F., Zambrano, A. M. A., Aide, T. M., et al. (2016). Carbon sequestration potential of second-growth forest regeneration in the Latin American tropics. Sci. Adv. 2, e1501639. doi:10.1126/sciadv.1501639
Chen, C., Li, Y., Piao, S., Wang, X., and Huang, M. (2020). Biophysical impacts of Earth greening largely controlled by aerodynamic resistance. Sci. Adv. 6, eabb1981. doi:10.1126/sciadv.abb1981
Ciais, P., Sabine, C., Bala, G., Bopp, L., Brovkin, V., Canadell, J., et al. (2013). “Carbon and other biogeochemical cycles,” in Climate change 2013: the physical science basis. contribution of working group I to the fifth assessment report of the intergovernmental panel on climate change Editors T. F. Stocker, D. Qin, G.-K. Plattner, M. Tignor, S. K. Allen, J. Boschunget al. Cambridge University Press, Cambridge, United Kingdom and New York, NY, USA, 465–570.
Cole, L. E. S., Bhagwat, S. A., and Willis, K. J. (2014). Recovery and resilience of tropical forests after disturbance. Nat. Commun. 5, 3906. doi:10.1038/ncomms4906
Corlett, R. T. (2016). The impacts of droughts in tropical forests. Trends Plant Sci. 21, 584–593. doi:10.1016/j.tplants.2016.02.003
Cromsigt, J. P. G. M., and te Beest, M. (2014). Restoration of a megaherbivore: landscape-level impacts of white rhinoceros in Kruger National Park, South Africa. J. Ecol. 102, 566–575. doi:10.1111/1365-2745.12218
Curtis, P. G., Slay, C. M., Harris, N. L., Tyukavina, A., and Hansen, M. C. (2018). Classifying drivers of global forest loss. Science 361, 1108–1111. doi:10.1126/science.aau3445
Dai, A. (2013). Increasing drought under global warming in observations and models. Nat. Clim. Change 3, 52–58. doi:10.1038/nclimate1633
D’Andrea, E., Scartazza, A., Battistelli, A., Collalti, A., Proietti, S., Rezaie, N., et al. (2021). Unravelling resilience mechanisms in forests: role of non-structural carbohydrates in responding to extreme weather events. Tree Physiol. 41, 1808–1818. doi:10.1093/treephys/tpab044
da Silva, R. D., Galvão, L. S., dos Santos, J. R., de J. Silva, C. V., and de Moura, Y. M. (2014). Spectral/textural attributes from ALI/EO-1 for mapping primary and secondary tropical forests and studying the relationships with biophysical parameters. GIScience Remote Sens. 51, 677–694. doi:10.1080/15481603.2014.972866
D’Onofrio, D., von Hardenberg, J., and Baudena, M. (2018). Not only trees: grasses determine African tropical biome distributions via water limitation and fire. Glob. Ecol. Biogeogr. 27, 714–725. doi:10.1111/geb.12735
Doughty, C. E., Metcalfe, D. B., Girardin, C. A. J., Amézquita, F. F., Cabrera, D. G., Huasco, W. H., et al. (2015). Drought impact on forest carbon dynamics and fluxes in Amazonia. Nature 519, 78–82. doi:10.1038/nature14213
Dubayah, R., Blair, J. B., Goetz, S., Fatoyinbo, L., Hansen, M., Healey, S., et al. (2020). The global ecosystem dynamics investigation: high-resolution laser ranging of the earth’s forests and topography. Sci. Remote Sens. 1, 100002. doi:10.1016/j.srs.2020.100002
Dwiputra, A., Coops, N. C., and Schwartz, N. B. (2023). GEDI waveform metrics in vegetation mapping—a case study from a heterogeneous tropical forest landscape. Environ. Res. Lett. 18, 015007. doi:10.1088/1748-9326/acad8d
Dwomoh, F. K., Wimberly, M. C., Cochrane, M. A., and Numata, I. (2019). Forest degradation promotes fire during drought in moist tropical forests of Ghana. For. Ecol. Manag. 440, 158–168. doi:10.1016/j.foreco.2019.03.014
Ewers, R. M., and Banks-Leite, C. (2013). Fragmentation impairs the microclimate buffering effect of tropical forests. PLOS ONE 8, e58093. doi:10.1371/journal.pone.0058093
Fan, L., Wigneron, J. P., Ciais, P., Chave, J., Brandt, M., Fensholt, R., et al. (2019a). Satellite-observed pantropical carbon dynamics. Nat. Plants 5, 944–951. doi:10.1038/s41477-019-0478-9
Fan, Y., Meijide, A., Lawrence, D. M., Roupsard, O., Carlson, K. M., Chen, H., et al. (2019b). Reconciling canopy interception parameterization and rainfall forcing frequency in the community land model for simulating evapotranspiration of rainforests and oil palm plantations in Indonesia. J. Adv. Model. Earth Syst. 11, 732–751. doi:10.1029/2018MS001490
Fan, Y., Roupsard, O., Bernoux, M., Le Maire, G., Panferov, O., Kotowska, M. M., et al. (2015). A sub-canopy structure for simulating oil palm in the Community Land Model (CLM-Palm): phenology, allocation and yield. Geosci. Model Dev. 8, 3785–3800. doi:10.5194/gmd-8-3785-2015
FAO (2020). Global forest resources assessment 2020: main report. Rome, Italy: Food and Agriculture Organization of the UN. doi:10.4060/ca9825en
Fatichi, S., Pappas, C., Zscheischler, J., and Leuzinger, S. (2019). Modelling carbon sources and sinks in terrestrial vegetation. New Phytol. 221, 652–668. doi:10.1111/nph.15451
Felbab-Brown, V. (2013). “The jagged edge: illegal logging in Southeast Asia,” in An atlas of trafficking in Southeast Asia (New York: I.B. Tauris & Co., Ltd.) 113–136.
Fischer, R., Taubert, F., Müller, M. S., Groeneveld, J., Lehmann, S., Wiegand, T., et al. (2021). Accelerated forest fragmentation leads to critical increase in tropical forest edge area. Sci. Adv. 7, eabg7012. doi:10.1126/sciadv.abg7012
Fisher, R. A., Williams, M., Da Costa, A. L., Malhi, Y., Da Costa, R. F., Almeida, S., et al. (2007). The response of an Eastern Amazonian rain forest to drought stress: results and modelling analyses from a throughfall exclusion experiment. Glob. Change Biol. 13, 2361–2378. doi:10.1111/j.1365-2486.2007.01417.x
Francini, S., McRoberts, R. E., Giannetti, F., Mencucci, M., Marchetti, M., Scarascia Mugnozza, G., et al. (2020). Near-real time forest change detection using PlanetScope imagery. Eur. J. Remote Sens. 53, 233–244. doi:10.1080/22797254.2020.1806734
Gao, Y., Skutsch, M., Paneque-Gálvez, J., and Ghilardi, A. (2020). Remote sensing of forest degradation: a review. Environ. Res. Lett. 15, 103001. doi:10.1088/1748-9326/abaad7
Gardner, T. A., Barlow, J., Chazdon, R., Ewers, R. M., Harvey, C. A., Peres, C. A., et al. (2009). Prospects for tropical forest biodiversity in a human-modified world. Ecol. Lett. 12, 561–582. doi:10.1111/j.1461-0248.2009.01294.x
Ghazoul, J., Burivalova, Z., Garcia-Ulloa, J., and King, L. A. (2015). Conceptualizing forest degradation. Trends Ecol. Evol. 30, 622–632. doi:10.1016/j.tree.2015.08.001
Giljum, S., Maus, V., Kuschnig, N., Luckeneder, S., Tost, M., Sonter, L. J., et al. (2022). A pantropical assessment of deforestation caused by industrial mining. Proc. Natl. Acad. Sci. 119, e2118273119. doi:10.1073/pnas.2118273119
Graham, E. B., Averill, C., Bond-Lamberty, B., Knelman, J. E., Krause, S., Peralta, A. L., et al. (2021). Toward a generalizable framework of disturbance ecology through crowdsourced science. Front. Ecol. Evol. 9. doi:10.3389/fevo.2021.588940
Gurevitch, J. (2022). Managing forests for competing goals. Science 376, 792–793. doi:10.1126/science.abp8463
Hansen, M. C., Potapov, P. V., Moore, R., Hancher, M., Turubanova, S. A., Tyukavina, A., et al. (2013). High-resolution global maps of 21st-century forest cover change. Science 342, 850–853. doi:10.1126/science.1244693
Hansen, M. C., Wang, L., Song, X. P., Tyukavina, A., Turubanova, S., Potapov, P. V., et al. (2020). The fate of tropical forest fragments. Sci. Adv. 6, eaax8574. doi:10.1126/sciadv.aax8574
Hardwick, S. R., Toumi, R., Pfeifer, M., Turner, E. C., Nilus, R., and Ewers, R. M. (2015). The relationship between leaf area index and microclimate in tropical forest and oil palm plantation: forest disturbance drives changes in microclimate. Agric. For. Meteorology 201, 187–195. doi:10.1016/j.agrformet.2014.11.010
Harris, N. L., Brown, S., Hagen, S. C., Saatchi, S. S., Petrova, S., Salas, W., et al. (2012). Baseline map of carbon emissions from deforestation in tropical regions. Science 336, 1573–1576. doi:10.1126/science.1217962
Hartmann, H., and Trumbore, S. (2016). Understanding the roles of nonstructural carbohydrates in forest trees – from what we can measure to what we want to know. New Phytol. 211, 386–403. doi:10.1111/nph.13955
Heinimann, A., Mertz, O., Frolking, S., Egelund Christensen, A., Hurni, K., Sedano, F., et al. (2017). A global view of shifting cultivation: recent, current, and future extent. PLOS ONE 12, e0184479. doi:10.1371/journal.pone.0184479
Heinrich, V. H. A., Dalagnol, R., Cassol, H. L. G., Rosan, T. M., de Almeida, C. T., Silva Junior, C. H. L., et al. (2021). Large carbon sink potential of secondary forests in the Brazilian Amazon to mitigate climate change. Nat. Commun. 12, 1785. doi:10.1038/s41467-021-22050-1
Heinrich, V. H. A., Vancutsem, C., Dalagnol, R., Rosan, T. M., Fawcett, D., Silva-Junior, C. H. L., et al. (2023). The carbon sink of secondary and degraded humid tropical forests. Nature 615, 436–442. doi:10.1038/s41586-022-05679-w
Hethcoat, M. G., Carreiras, J. M. B., Edwards, D. P., Bryant, R. G., and Quegan, S. (2021). Detecting tropical selective logging with C-band SAR data may require a time series approach. Remote Sens. Environ. 259, 112411. doi:10.1016/j.rse.2021.112411
Hirschmugl, M., Gallaun, H., Dees, M., Datta, P., Deutscher, J., Koutsias, N., et al. (2017). Methods for mapping forest disturbance and degradation from optical earth observation data: a review. Curr. For. Rep. 3, 32–45. doi:10.1007/s40725-017-0047-2
Hoffmann, W. A., Geiger, E. L., Gotsch, S. G., Rossatto, D. R., Silva, L. C. R., Lau, O. L., et al. (2012). Ecological thresholds at the savanna-forest boundary: how plant traits, resources and fire govern the distribution of tropical biomes. Ecol. Lett. 15, 759–768. doi:10.1111/j.1461-0248.2012.01789.x
Hua, F., Bruijnzeel, L. A., Meli, P., Martin, P. A., Zhang, J., Nakagawa, S., et al. (2022). The biodiversity and ecosystem service contributions and trade-offs of forest restoration approaches. Science 376, 839–844. doi:10.1126/science.abl4649
Hwang, K., Harpold, A. A., Tague, C. L., Lowman, L., Boisramé, G. F. S., Lininger, K. B., et al. (2023). Seeing the disturbed forest for the trees: remote sensing is underutilized to quantify critical zone response to unprecedented disturbance. Earth's Future 11, e2022EF003314. doi:10.1029/2022EF003314
Hyvarinen, O., Te Beest, M., le Roux, E., Kerley, G., de Groot, E., Vinita, R., et al. (2021). Megaherbivore impacts on ecosystem and Earth system functioning: the current state of the science. Ecography 44, 1579–1594. doi:10.1111/ecog.05703
Jamaludin, J., De Alban, J. D. T., Carrasco, L. R., and Webb, E. L. (2022). Spatiotemporal analysis of deforestation patterns and drivers reveals emergent threats to tropical forest landscapes. Environ. Res. Lett. 17, 054046. doi:10.1088/1748-9326/ac68fa
Jones, M. W., Abatzoglou, J. T., Veraverbeke, S., Andela, N., Lasslop, G., Forkel, M., et al. (2022). Global and regional trends and drivers of fire under climate change. Rev. Geophys. 60, e2020RG000726. doi:10.1029/2020rg000726
Jucker, T., Hardwick, S. R., Both, S., Elias, D. M., Ewers, R. M., Milodowski, D. T., et al. (2018). Canopy structure and topography jointly constrain the microclimate of human-modified tropical landscapes. Glob. Change Biol. 24, 5243–5258. doi:10.1111/gcb.14415
Jucker, T., Jackson, T. D., Zellweger, F., Swinfield, T., Gregory, N., Williamson, J., et al. (2020). A research agenda for microclimate ecology in human-modified tropical forests. Front. For. Glob. Change 2. doi:10.3389/ffgc.2019.00092
Jung, M., Reichstein, M., Margolis, H. A., Cescatti, A., Richardson, A. D., Arain, M. A., et al. (2011). Global patterns of land-atmosphere fluxes of carbon dioxide, latent heat, and sensible heat derived from eddy covariance, satellite, and meteorological observations. J. Geophys. Res. Biogeosciences 116, G00J07. doi:10.1029/2010jg001566
Kissinger, G., Herold, M., and De Sy, V. (2012). Drivers of deforestation and forest degradation: a synthesis report for REDD+ policymakers. Vancouver, Canada, Lexeme Consulting.
Kleinman, J. S., Goode, J. D., Fries, A. C., and Hart, J. L. (2019). Ecological consequences of compound disturbances in forest ecosystems: a systematic review. Ecosphere 10, e02962. doi:10.1002/ecs2.2962
Kleinschroth, F., Gourlet-Fleury, S., Sist, P., Mortier, F., and Healey, J. R. (2015). Legacy of logging roads in the Congo Basin: how persistent are the scars in forest cover? Ecosphere 6, 1–17. doi:10.1890/es14-00488.1
Kleinschroth, F., Healey, J. R., Sist, P., Mortier, F., and Gourlet-Fleury, S. (2016). How persistent are the impacts of logging roads on Central African forest vegetation? J. Appl. Ecol. 53, 1127–1137. doi:10.1111/1365-2664.12661
Koch, A., and Kaplan, J. O. (2022). Tropical forest restoration under future climate change. Nat. Clim. Change 12, 279–283. doi:10.1038/s41558-022-01289-6
Koh, L. P., and Wilcove, D. S. (2008). Is oil palm agriculture really destroying tropical biodiversity? Conserv. Lett. 1, 60–64. doi:10.1111/j.1755-263x.2008.00011.x
Kranabetter, J. M., McLauchlan, K. K., Enders, S. K., Fraterrigo, J. M., Higuera, P. E., Morris, J. L., et al. (2016). A framework to assess biogeochemical response to ecosystem disturbance using nutrient partitioning ratios. Ecosystems 19, 387–395. doi:10.1007/s10021-015-9934-1
Lapola, D. M., Pinho, P., Barlow, J., Aragão, L. E. O. C., Berenguer, E., Carmenta, R., et al. (2023). The drivers and impacts of Amazon forest degradation. Science 379, eabp8622. doi:10.1126/science.abp8622
Laso Bayas, J. C., See, L., Georgieva, I., Schepaschenko, D., Danylo, O., Dürauer, M., et al. (2022). Drivers of tropical forest loss between 2008 and 2019. Sci. Data 9, 146. doi:10.1038/s41597-022-01227-3
Laurance, W. F. (2008). Theory meets reality: how habitat fragmentation research has transcended island biogeographic theory. Biol. Conserv. 141, 1731–1744. doi:10.1016/j.biocon.2008.05.011
Laurance, W. F., Camargo, J. L., Luizão, R. C., Laurance, S. G., Pimm, S. L., Bruna, E. M., et al. (2011). The fate of Amazonian forest fragments: a 32-year investigation. Biol. Conserv. 144, 56–67. doi:10.1016/j.biocon.2010.09.021
Laurance, W. F., Ferreira, L. V., Rankin-de Merona, J. M., and Laurance, S. G. (1998). RAIN FOREST FRAGMENTATION AND THE DYNAMICS OF AMAZONIAN TREE COMMUNITIES. Ecology 79, 2032–2040. doi:10.2307/176707
Lechner, A. M., Foody, G. M., and Boyd, D. S. (2020). Applications in remote sensing to forest ecology and management. One Earth 2, 405–412. doi:10.1016/j.oneear.2020.05.001
Lee, X., Goulden, M. L., Hollinger, D. Y., Barr, A., Black, T. A., Bohrer, G., et al. (2011). Observed increase in local cooling effect of deforestation at higher latitudes. Nature 479, 384–387. doi:10.1038/nature10588
Le Page, Y., Morton, D., Hartin, C., Bond-Lamberty, B., Pereira, J. M. C., Hurtt, G., et al. (2017). Synergy between land use and climate change increases future fire risk in Amazon forests. Earth Syst. Dynam. 8, 1237–1246. doi:10.5194/esd-8-1237-2017
Lewis, S. L., Brando, P. M., Phillips, O. L., van der Heijden, G. M. F., and Nepstad, D. (2011). The 2010 Amazon drought. Science 331, 554. doi:10.1126/science.1200807
Lewis, S. L., Edwards, D. P., and Galbraith, D. (2015). Increasing human dominance of tropical forests. Science 349, 827–832. doi:10.1126/science.aaa9932
Li, S., Brandt, M., Fensholt, R., Kariryaa, A., Igel, C., Gieseke, F., et al. (2023). Deep learning enables image-based tree counting, crown segmentation, and height prediction at national scale. PNAS Nexus 2, pgad076. doi:10.1093/pnasnexus/pgad076
Li, W., Ciais, P., Peng, S., Yue, C., Wang, Y., Thurner, M., et al. (2017). Land-use and land-cover change carbon emissions between 1901 and 2012 constrained by biomass observations. Biogeosciences 14, 5053–5067. doi:10.5194/bg-14-5053-2017
Li, W., MacBean, N., Ciais, P., Defourny, P., Lamarche, C., Bontemps, S., et al. (2018). Gross and net land cover changes in the main plant functional types derived from the annual ESA CCI land cover maps (1992–2015). Earth Syst. Sci. Data 10, 219–234. doi:10.5194/essd-10-219-2018
Li, Y., Zhao, M., Motesharrei, S., Mu, Q., Kalnay, E., and Li, S. (2015). Local cooling and warming effects of forests based on satellite observations. Nat. Commun. 6, 6603. doi:10.1038/ncomms7603
Liu, Y. Y., van Dijk, A. I. J. M., de Jeu, R. A. M., Canadell, J. G., McCabe, M. F., Evans, J. P., et al. (2015). Recent reversal in loss of global terrestrial biomass. Nat. Clim. Change 5, 470–474. doi:10.1038/nclimate2581
Liu, Z., Ballantyne, A. P., and Cooper, L. A. (2019). Biophysical feedback of global forest fires on surface temperature. Nat. Commun. 10, 214. doi:10.1038/s41467-018-08237-z
Longo, M., Saatchi, S., Keller, M., Bowman, K., Ferraz, A., Moorcroft, P. R., et al. (2020). Impacts of degradation on water, energy, and carbon cycling of the Amazon tropical forests. J. Geophys. Res. Biogeosciences 125, e2020JG005677. doi:10.1029/2020JG005677
Martin, P. A., Newton, A. C., and Bullock, J. M. (2013). Carbon pools recover more quickly than plant biodiversity in tropical secondary forests. Proc. R. Soc. B Biol. Sci. 280, 20132236. doi:10.1098/rspb.2013.2236
Matricardi, E. A. T., Skole, D. L., Costa, O. B., Pedlowski, M. A., Samek, J. H., and Miguel, E. P. (2020). Long-term forest degradation surpasses deforestation in the Brazilian Amazon. Science 369, 1378–1382. doi:10.1126/science.abb3021
McLauchlan, K. K., Higuera, P. E., Gavin, D. G., Perakis, S. S., Mack, M. C., Alexander, H., et al. (2014). Reconstructing disturbances and their biogeochemical consequences over multiple timescales. BioScience 64, 105–116. doi:10.1093/biosci/bit017
Meijide, A., Röll, A., Fan, Y., Herbst, M., Niu, F., Tiedemann, F., et al. (2017). Controls of water and energy fluxes in oil palm plantations: environmental variables and oil palm age. Agric. For. Meteorology 239, 71–85. doi:10.1016/j.agrformet.2017.02.034
Mitchell, A. L., Rosenqvist, A., and Mora, B. (2017). Current remote sensing approaches to monitoring forest degradation in support of countries measurement, reporting and verification (MRV) systems for REDD+. Carbon Balance Manag. 12, 9. doi:10.1186/s13021-017-0078-9
Molinario, G., Hansen, M. C., Potapov, P. V., Tyukavina, A., Stehman, S., Barker, B., et al. (2017). Quantification of land cover and land use within the rural complex of the Democratic Republic of Congo. Environ. Res. Lett. 12, 104001. doi:10.1088/1748-9326/aa8680
Mouillot, F., and Field, C. B. (2005). Fire history and the global carbon budget: a 1°× 1° fire history reconstruction for the 20th century. Glob. Change Biol. 11, 398–420. doi:10.1111/j.1365-2486.2005.00920.x
Mugabowindekwe, M., Brandt, M., Chave, J., Reiner, F., Skole, D. L., Kariryaa, A., et al. (2023). Nation-wide mapping of tree-level aboveground carbon stocks in Rwanda. Nat. Clim. Change 13, 91–97. doi:10.1038/s41558-022-01544-w
Numata, I., Khand, K., Kjaersgaard, J., Cochrane, M. A., and Silva, S. S. (2021). Forest evapotranspiration dynamics over a fragmented forest landscape under drought in southwestern Amazonia. Agric. For. Meteorology 306, 108446. doi:10.1016/j.agrformet.2021.108446
Pan, Y., Birdsey, R. A., Fang, J., Houghton, R., Kauppi, P. E., Kurz, W. A., et al. (2011). A large and persistent carbon sink in the world’s forests. Science 333, 988–993. doi:10.1126/science.1201609
Pandey, A., Arunachalam, K., Thadani, R., and Singh, V. (2020). Forest degradation impacts on carbon stocks, tree density and regeneration status in banj oak forests of Central Himalaya. Ecol. Res. 35, 208–218. doi:10.1111/1440-1703.12078
Parolin, P., and Wittmann, F. (2010). Struggle in the flood: tree responses to flooding stress in four tropical floodplain systems. AoB PLANTS 2010, plq003. doi:10.1093/aobpla/plq003
Peng, S.-S., Piao, S., Zeng, Z., Ciais, P., Zhou, L., Li, L. Z. X., et al. (2014). Afforestation in China cools local land surface temperature. Proc. Natl. Acad. Sci. 111, 2915–2919. doi:10.1073/pnas.1315126111
Philipson, C. D., Cutler, M. E. J., Brodrick, P. G., Asner, G. P., Boyd, D. S., Moura Costa, P., et al. (2020). Active restoration accelerates the carbon recovery of human-modified tropical forests. Science 369, 838–841. doi:10.1126/science.aay4490
Phillips, O. L., Aragao, L. E. O. C., Lewis, S. L., Fisher, J. B., Lloyd, J., Lopez-Gonzalez, G., et al. (2009). Drought sensitivity of the Amazon rainforest. Science 323, 1344–1347. doi:10.1126/science.1164033
Piper, F. I., and Paula, S. (2020). The role of nonstructural carbohydrates storage in forest resilience under climate change. Curr. For. Rep. 6, 1–13. doi:10.1007/s40725-019-00109-z
Poorter, L., Bongers, F., Aide, T. M., Almeyda Zambrano, A. M., Balvanera, P., Becknell, J. M., et al. (2016). Biomass resilience of Neotropical secondary forests. Nature 530, 211–214. doi:10.1038/nature16512
Poorter, L., Craven, D., Jakovac, C. C., van der Sande, M. T., Amissah, L., Bongers, F., et al. (2021). Multidimensional tropical forest recovery. Science 374, 1370–1376. doi:10.1126/science.abh3629
Potapov, P., Li, X., Hernandez-Serna, A., Tyukavina, A., Hansen, M. C., Kommareddy, A., et al. (2021). Mapping global forest canopy height through integration of GEDI and Landsat data. Remote Sens. Environ. 253, 112165. doi:10.1016/j.rse.2020.112165
Pugh, T. A. M., Arneth, A., Kautz, M., Poulter, B., and Smith, B. (2019a). Important role of forest disturbances in the global biomass turnover and carbon sinks. Nat. Geosci. 12, 730–735. doi:10.1038/s41561-019-0427-2
Pugh, T. A. M., Lindeskog, M., Smith, B., Poulter, B., Arneth, A., Haverd, V., et al. (2019b). Role of forest regrowth in global carbon sink dynamics. Proc. Natl. Acad. Sci. 116, 4382–4387. doi:10.1073/pnas.1810512116
Pyles, M. V., Magnago, L. F. S., Maia, V. A., Pinho, B. X., Pitta, G., de Gasper, A. L., et al. (2022). Human impacts as the main driver of tropical forest carbon. Sci. Adv. 8, eabl7968. doi:10.1126/sciadv.abl7968
Qin, Y., Xiao, X., Wigneron, J. P., Ciais, P., Brandt, M., Fan, L., et al. (2021). Carbon loss from forest degradation exceeds that from deforestation in the Brazilian Amazon. Nat. Clim. Change 11, 442–448. doi:10.1038/s41558-021-01026-5
Reichstein, M., Bahn, M., Ciais, P., Frank, D., Mahecha, M. D., Seneviratne, S. I., et al. (2013). Climate extremes and the carbon cycle. Nature 500, 287–295. doi:10.1038/nature12350
Resende, A. F. D., Schöngart, J., Streher, A. S., Ferreira-Ferreira, J., Piedade, M. T. F., and Silva, T. S. F. (2019). Massive tree mortality from flood pulse disturbances in Amazonian floodplain forests: the collateral effects of hydropower production. Sci. Total Environ. 659, 587–598. doi:10.1016/j.scitotenv.2018.12.208
Rudel, T. K. (2013). The national determinants of deforestation in sub-Saharan Africa. Philosophical Trans. R. Soc. B Biol. Sci. 368, 20120405. doi:10.1098/rstb.2012.0405
Sanczuk, P., De Pauw, K., De Lombaerde, E., Luoto, M., Meeussen, C., Govaert, S., et al. (2023). Microclimate and forest density drive plant population dynamics under climate change. Nat. Clim. Change 13, 840–847. doi:10.1038/s41558-023-01744-y
Santoro, M., Cartus, O., Carvalhais, N., Rozendaal, D. M. A., Avitabile, V., Araza, A., et al. (2021). The global forest above-ground biomass pool for 2010 estimated from high-resolution satellite observations. Earth Syst. Sci. Data 13, 3927–3950. doi:10.5194/essd-13-3927-2021
Schmitz, O. J., Wilmers, C. C., Leroux, S. J., Doughty, C. E., Atwood, T. B., Galetti, M., et al. (2018). Animals and the zoogeochemistry of the carbon cycle. Science 362, eaar3213. doi:10.1126/science.aar3213
Song, X.-P., Hansen, M. C., Potapov, P., Adusei, B., Pickering, J., Adami, M., et al. (2021). Massive soybean expansion in South America since 2000 and implications for conservation. Nat. Sustain. 4, 784–792. doi:10.1038/s41893-021-00729-z
Sonter, L. J., Herrera, D., Barrett, D. J., Galford, G. L., Moran, C. J., and Soares-Filho, B. S. (2017). Mining drives extensive deforestation in the Brazilian Amazon. Nat. Commun. 8, 1013. doi:10.1038/s41467-017-00557-w
Staal, A., Flores, B. M., Aguiar, A. P. D., Bosmans, J. H. C., Fetzer, I., and Tuinenburg, O. A. (2020). Feedback between drought and deforestation in the Amazon. Environ. Res. Lett. 15, 044024. doi:10.1088/1748-9326/ab738e
Stahl, A. T., Andrus, R., Hicke, J. A., Hudak, A. T., Bright, B. C., and Meddens, A. J. (2023). Automated attribution of forest disturbance types from remote sensing data: a synthesis. Remote Sens. Environ. 285, 113416. doi:10.1016/j.rse.2022.113416
Tang, X., Bratley, K. H., Cho, K., Bullock, E. L., Olofsson, P., and Woodcock, C. E. (2023). Near real-time monitoring of tropical forest disturbance by fusion of Landsat, Sentinel-2, and Sentinel-1 data. Remote Sens. Environ. 294, 113626. doi:10.1016/j.rse.2023.113626
Tao, S., Chave, J., Frison, P. L., Le Toan, T., Ciais, P., Fang, J., et al. (2022). Increasing and widespread vulnerability of intact tropical rainforests to repeated droughts. Proc. Natl. Acad. Sci. 119, e2116626119. doi:10.1073/pnas.2116626119
Taubert, F., Fischer, R., Groeneveld, J., Lehmann, S., Müller, M. S., Rödig, E., et al. (2018). Global patterns of tropical forest fragmentation. Nature 554, 519–522. doi:10.1038/nature25508
Tyukavina, A., Hansen, M. C., Potapov, P., Parker, D., Okpa, C., Stehman, S. V., et al. (2018). Congo Basin forest loss dominated by increasing smallholder clearing. Sci. Adv. 4, eaat2993. doi:10.1126/sciadv.aat2993
Umunay, P. M., Gregoire, T. G., Gopalakrishna, T., Ellis, P. W., and Putz, F. E. (2019). Selective logging emissions and potential emission reductions from reduced-impact logging in the Congo Basin. For. Ecol. Manag. 437, 360–371. doi:10.1016/j.foreco.2019.01.049
Vancutsem, C., Achard, F., Pekel, J. F., Vieilledent, G., Carboni, S., Simonetti, D., et al. (2021). Long-term (1990-2019) monitoring of forest cover changes in the humid tropics. Sci. Adv. 7, eabe1603. doi:10.1126/sciadv.abe1603
Vijay, V., Pimm, S. L., Jenkins, C. N., and Smith, S. J. (2016). The impacts of oil palm on recent deforestation and biodiversity loss. PLOS ONE 11, e0159668. doi:10.1371/journal.pone.0159668
Vourlitis, G. L., Priante Filho, N., Hayashi, M. M. S., Nogueira, J. d. S., Raiter, F., Hoegel, W., et al. (2004). EFFECTS OF METEOROLOGICAL VARIATIONS ON THE CO2 EXCHANGE OF A BRAZILIAN TRANSITIONAL TROPICAL FOREST. Ecol. Appl. 14, 89–100. doi:10.1890/01-6005
Walker, A. P., De Kauwe, M. G., Bastos, A., Belmecheri, S., Georgiou, K., Keeling, R. F., et al. (2021). Integrating the evidence for a terrestrial carbon sink caused by increasing atmospheric CO2. New Phytol. 229, 2413–2445. doi:10.1111/nph.16866
Walker, L. R., Wardle, D. A., Bardgett, R. D., and Clarkson, B. D. (2010). The use of chronosequences in studies of ecological succession and soil development. J. Ecol. 98, 725–736. doi:10.1111/j.1365-2745.2010.01664.x
Wang, Y., Ziv, G., Adami, M., Mitchard, E., Batterman, S. A., Buermann, W., et al. (2019). Mapping tropical disturbed forests using multi-decadal 30 m optical satellite imagery. Remote Sens. Environ. 221, 474–488. doi:10.1016/j.rse.2018.11.028
White, P. S., and Pickett, S. T. A. (1985). The ecology of natural disturbance and patch dynamics. Academic Press, 3–13.
Wieczkowski, J. (2009). Tree mortality due to an El Niño flood along the lower tana river, Kenya. Afr. J. Ecol. 47, 56–62. doi:10.1111/j.1365-2028.2007.00918.x
Wigneron, J.-P., Fan, L., Ciais, P., Bastos, A., Brandt, M., Chave, J., et al. (2020). Tropical forests did not recover from the strong 2015-2016 El Niño event. Sci. Adv. 6, eaay4603. doi:10.1126/sciadv.aay4603
Wilcove, D. S., Giam, X., Edwards, D. P., Fisher, B., and Koh, L. P. (2013). Navjot's nightmare revisited: logging, agriculture, and biodiversity in Southeast Asia. Trends Ecol. Evol. 28, 531–540. doi:10.1016/j.tree.2013.04.005
Windisch, M. G., Davin, E. L., and Seneviratne, S. I. (2021). Prioritizing forestation based on biogeochemical and local biogeophysical impacts. Nat. Clim. Change 11, 867–871. doi:10.1038/s41558-021-01161-z
Xu, C., McDowell, N. G., Fisher, R. A., Wei, L., Sevanto, S., Christoffersen, B. O., et al. (2019). Increasing impacts of extreme droughts on vegetation productivity under climate change. Nat. Clim. Change 9, 948–953. doi:10.1038/s41558-019-0630-6
Xu, L., Saatchi, S. S., Yang, Y., Yu, Y., Pongratz, J., Bloom, A. A., et al. (2021a). Changes in global terrestrial live biomass over the 21st century. Sci. Adv. 7, eabe9829. doi:10.1126/sciadv.abe9829
Xu, Y., Ciais, P., Yu, L., Li, W., Chen, X., Zhang, H., et al. (2021b). Oil palm modelling in the global land surface model ORCHIDEE-MICT. Geosci. Model Dev. 14, 4573–4592. doi:10.5194/gmd-14-4573-2021
Xu, Y., Yu, L., Ciais, P., Li, W., Santoro, M., Yang, H., et al. (2022). Recent expansion of oil palm plantations into carbon-rich forests. Nat. Sustain. 5, 574–577. doi:10.1038/s41893-022-00872-1
Yang, J., Tian, H., Tao, B., Ren, W., Kush, J., Liu, Y., et al. (2014). Spatial and temporal patterns of global burned area in response to anthropogenic and environmental factors: reconstructing global fire history for the 20th and early 21st centuries. J. Geophys. Res. Biogeosciences 119, 249–263. doi:10.1002/2013JG002532
Yue, C., Ciais, P., Cadule, P., Thonicke, K., Archibald, S., Poulter, B., et al. (2014). Modelling the role of fires in the terrestrial carbon balance by incorporating SPITFIRE into the global vegetation model ORCHIDEE – Part 1: simulating historical global burned area and fire regimes. Geosci. Model Dev. 7, 2747–2767. doi:10.5194/gmd-7-2747-2014
Yue, C., Ciais, P., Luyssaert, S., Li, W., McGrath, M. J., Chang, J., et al. (2018). Representing anthropogenic gross land use change, wood harvest, and forest age dynamics in a global vegetation model ORCHIDEE-MICT v8.4.2. Geosci. Model Dev. 11, 409–428. doi:10.5194/gmd-11-409-2018
Zalles, V., Hansen, M. C., Potapov, P. V., Stehman, S. V., Tyukavina, A., Pickens, A., et al. (2019). Near doubling of Brazil’s intensive row crop area since 2000. Proc. Natl. Acad. Sci. 116, 428–435. doi:10.1073/pnas.1810301115
Zeng, Z., Estes, L., Ziegler, A. D., Chen, A., Searchinger, T., Hua, F., et al. (2018). Highland cropland expansion and forest loss in Southeast Asia in the twenty-first century. Nat. Geosci. 11, 556–562. doi:10.1038/s41561-018-0166-9
Zhao, M., Liu, Y., and Konings, A. G. (2022). Evapotranspiration frequently increases during droughts. Nat. Clim. Change 12, 1024–1030. doi:10.1038/s41558-022-01505-3
Zhao, Z., Li, W., Ciais, P., Santoro, M., Cartus, O., Peng, S., et al. (2021). Fire enhances forest degradation within forest edge zones in Africa. Nat. Geosci. 14, 479–483. doi:10.1038/s41561-021-00763-8
Zhu, L., Li, W., Ciais, P., He, J., Cescatti, A., Santoro, M., et al. (2023). Comparable biophysical and biogeochemical feedbacks on warming from tropical moist forest degradation. Nat. Geosci. 16, 244–249. doi:10.1038/s41561-023-01137-y
Keywords: disturbances, tropical moist forests, deforestation, forest degradation, remote sensing monitoring, process-based models
Citation: He J, Li W, Zhao Z, Zhu L, Du X, Xu Y, Sun M, Zhou J, Ciais P, Wigneron J-P, Liu R, Lin G and Fan L (2024) Recent advances and challenges in monitoring and modeling of disturbances in tropical moist forests. Front. Remote Sens. 5:1332728. doi: 10.3389/frsen.2024.1332728
Received: 03 November 2023; Accepted: 26 February 2024;
Published: 14 March 2024.
Edited by:
Xing Li, Seoul National University, Republic of KoreaReviewed by:
Su Ye, Zhejiang University, ChinaCopyright © 2024 He, Li, Zhao, Zhu, Du, Xu, Sun, Zhou, Ciais, Wigneron, Liu, Lin and Fan. This is an open-access article distributed under the terms of the Creative Commons Attribution License (CC BY). The use, distribution or reproduction in other forums is permitted, provided the original author(s) and the copyright owner(s) are credited and that the original publication in this journal is cited, in accordance with accepted academic practice. No use, distribution or reproduction is permitted which does not comply with these terms.
*Correspondence: Wei Li, d2xpMjAxOUB0c2luZ2h1YS5lZHUuY24=
Disclaimer: All claims expressed in this article are solely those of the authors and do not necessarily represent those of their affiliated organizations, or those of the publisher, the editors and the reviewers. Any product that may be evaluated in this article or claim that may be made by its manufacturer is not guaranteed or endorsed by the publisher.
Research integrity at Frontiers
Learn more about the work of our research integrity team to safeguard the quality of each article we publish.