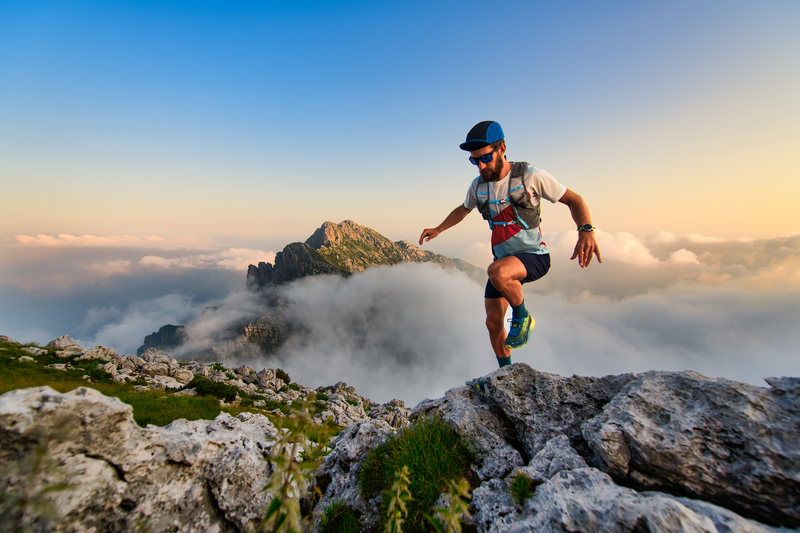
94% of researchers rate our articles as excellent or good
Learn more about the work of our research integrity team to safeguard the quality of each article we publish.
Find out more
ORIGINAL RESEARCH article
Front. Remote Sens. , 13 July 2023
Sec. Image Analysis and Classification
Volume 4 - 2023 | https://doi.org/10.3389/frsen.2023.1197523
This article is part of the Research Topic Women in Remote Sensing: 2022 View all 16 articles
The Southeastern United States has high landscape heterogeneity, with heavily managed forestlands, developed agriculture, and multiple metropolitan areas. The spatial pattern of land use is dynamic. Expansion of urban areas convert forested and agricultural land, scrub forests are converted to citrus groves, and some croplands transition to pine plantations. Previous studies have recognized that forest management is the predominant factor in structural and functional changes forests, but little is known about how forest management practices interact with surrounding land uses at the regional scale. The first step in studying the spatial relationships of forest management with surrounding landscapes is to be able to map management practices and describe their proximity to various land uses. There are two major difficulties in generating land use and land management maps at the regional scale by any method: the necessity of large training data sets and expensive computation. The combination of crowdsourced, citizen-science mapping and cloud-based computing may help overcome those difficulties. In this study, OpenStreetMap is incorporated into mapping land use and shows great potential for justifying and monitoring land use at a regional scale. Google Earth Engine enables large-scale spatial analysis and imagery processing by providing a variety of Earth observation datasets and computational resources. By incorporating the OpenStreetMap dataset into Earth observation images to map forest land management practices and determine the distribution of other nearby land uses, we develop a robust regional land-use mapping approach and describe the patterns of how different land uses may affect forest management and vice versa. We find that cropland is more likely to be near ecological forest management patches; few close spatial relationships exist between land uses and preservation forest management, which fulfills the preservation management strategy of sustaining the forests, and production forests have the strongest spatial relationships with croplands. This approach leads to increased understanding of land-use patterns and management practices at local to regional scales.
Land-use change is greatly altering terrestrial ecosystems (Lambin and Meyfroidt, 2011; Forman, 2014). In the Southeastern United States (SEUS), land-use changes are responses to a wide array of socio-economic, environmental, and climate drivers. Not only land conversions but also land management changes alter large proportions of land over time (Wear and Greis, 2013). Land-use change, which is a local-scale land practice, has regional, continental, and global ecosystem consequences. Forest ecosystems are strongly affected by anthropogenic factors both inside and outside the forest, such as timber extraction and suburban growth and cultivation (Radeloff et al., 2010; Wear and Greis, 2012). Forest management practices, which are forest land uses, are one of the major drivers of changes in forest structure and function (Becknell et al., 2015; Marsik et al., 2018).
Forests play an important role in the ecological and social conditions of the SEUS. They provide critical habitat for a wide variety of plant and animal species, including many that are threatened or endangered. Forests also regulate local and regional water cycles, help control flooding and soil erosion, and contribute to the overall health of the landscape (Riitters et al., 2002). In addition to their ecological importance, forests are also an important part of the region’s social and economic fabric (Marsik et al., 2018). They provide recreation opportunities for residents and tourists alike, support a thriving timber industry, and offer a host of other economic benefits. With all these benefits, it is clear that forests are a vital part of the SEUS. However, they are also under threat from a variety of sources, including development, pollution, and climate change (Becknell et al., 2015). It is important to work to protect and restore forests in the region so that they can continue to provide these important benefits for generations to come.
The interactions among different land-use types may be forces of landscape-wide and even global importance. Land-use transitions from one type to another are more likely in areas already close to the second land uses (Fischlin et al., 2007). For example, in the SEUS significant expansions of urban areas often convert forested land to urban uses, especially as urban land spreads outwards from the already urbanized areas, and cropland has been converted to pine plantations in areas where plantations are nearby (Wear and Greis, 2002; 2013). These kinds of landscape transitions may represent macrosystem changes depending on their scale and extent and can have immediate local social and ecological implications for landowners and their management practices (Schulte et al., 2007; Wassenaar et al., 2007). As such, understanding the relationships between various land-use patterns and forest management at a macrosystem level is of utmost importance. The current body of knowledge regarding the influence of different land-use patterns’ proximity on forest management practices, and vice versa, remains limited. To address this gap, we undertake an investigation that will contribute to the study of effective forest management strategies and to understanding of the influence of the proximity of various land-use types on land-use and land-cover transitions.
One way to protect and restore forests is to understand better the land-use patterns around them. In many cases, human activities such as cultivation, housing developments, and industrial development, can influence forest ecosystems (Kramer and Doran, 2010; Wear and Greis, 2012; Wear and Greis, 2013). However, if we study these land uses and their effects on forest ecosystems, we can develop practices for mitigating the impacts. This will help to ensure that forests in the SEUS are healthy and thriving. Also, differently managed forests can spur developments and land-use changes in different ways (Kramer and Doran, 2010; Wear and Greis, 2012; Wear and Greis, 2013). For example, landscapes in proximity to preserved forested regions have more potential to be converted to residential areas (Kramer and Doran, 2010) and residential property close to protected areas is usually more valuable.
As implied by the material and energy flows and organism and gene exchanges that occur between them, forest ecosystems and the land use that surround them have an intricate relationship. While the interactions between protected forests and adjacent land uses have been extensively studied, our understanding of the effects of non-protected forest land conversion on adjacent land uses remains limited. Despite the fact that a number of theoretical interactions have been proposed (Groenveld et al., 2017; Briassoulis, 2020), the lack of generalizability in case studies has contributed to a paucity of knowledge regarding the mechanisms underlying these interactions. Effective land management strategies require a comprehensive and nuanced understanding of the relationship between non-protected forest land conversion and nearby land uses. Hansen and Ruth (2007) review on the ecological mechanisms linking protected areas to surrounding lands provides a valuable starting point for such investigations.
Changes in land use over time are also of critical importance. Land-use changes are associated with social and biophysical system changes (Turner et al., 1996). For example, land-use change can lead to the fragmentation of forests which can then impact ecosystem function (Riitters et al., 2002). Additionally, land-use change can also alter hydrological regimes and water quality. As such, it is important to consider land-use change when planning for the future of forest ecosystems in the SEUS. Changes in land-use intensity and land-use types influence nearby and even distant forest ecosystems (Dunford and Freemark, 2005; Fahrig, 2013). Most land change studies focus only on the transitions among the land covers at the class level across the landscape (e.g., the conversion between forest and cropland) (Verburg et al., 2002; Sohl and Sayler, 2008; Verburg et al., 2009), rather than the within-class transitions. e.g., an area stays forested but may change use from a natural forest area to silviculture or an agricultural cropping system, i.e., agroforestry. Researchers also focus on projecting land-use changes and trajectories based on past patterns (Lawler et al., 2014). In addition, ground-reference data related to land-use temporal changes are often omitted, most often due to lack of availability (Hurskainen et al., 2019).
The principles of mapping land use do not share the same assumptions as those of mapping land cover. Land cover refers to the physical land cover on the Earth’s surface, including both natural and man-made features (Comber, 2008). Land cover is the physical land cover type, such as buildings, roads, forests, grassland, or wetland. It can be monitored using satellite remote-sensing techniques. Forest cover is one type of land cover that is of particular interest for many reasons, including the support it provides for biodiversity, ecohydrological processes, other environmental services, and combating climate change. By monitoring forest cover, we can better understand the health of these ecosystems and take action to protect them (Becknell et al., 2015).
Land use is the human adoption of land cover to meet specific needs. Land use refers to the way humans use land cover, such as for agriculture, housing, or recreation (Comber, 2008). Land-use features are decided and driven by people’s land-management behaviors. We can monitor land-use change by looking at both land-cover and land-use information. There are many ways to monitor land-use changes. One common method is remote sensing, which uses satellites or aircraft to collect images of the Earth’s surface. This can be used to track changes in land cover over time (DeFries et al., 2007). Another common method is ground-reference data collection, which involves physically visiting sites on the ground to observe and document changes. Ground-reference data are often used in combination with remote sensing to verify and interpret satellite data (Sanchez-Azofeifa, 1996; Marsik et al., 2018). It can be difficult to distinguish land use from land cover using remote sensing alone, so ground-reference data are an important part of land-use monitoring.
There are many challenges to monitoring land-use change. One challenge is that land use can be hidden by land cover. For example, a forest may still be a forest even if it will soon be logged or is part of a protected area. Another challenge is that land use can vary greatly over short distances. For example, a field may be used for agriculture on one side and housing on large parcels on the other. This can make it difficult to create accurate maps of land use. Despite these challenges, it is important to monitor land-use change. Land use has a major impact on the environment and understanding how it is changing is essential for effective environmental management (Turner, 1994; Schulte et al., 2007).
In the phrase “social-ecological Earth observation dataset”, the term “social” relates to information about human social systems and includes citizen-contributed data, satellite-collected Lights at Night data (Sutton et al., 2009; Li et al., 2017) and land ownership data. To map regional land management practices, we utilized ground data such as ownership information, long-term phenological patterns and their changes, and the structure of the surrounding landscape to infer forest management classes (Marsik et al., 2018). We use the Earth observation datasets, which have close relationships with human activities, such as the night light (VIIRS Stray Light Corrected Nighttime Day/Night) and land ownership (Marsik et al., 2018) databases. Citizen science is a term used for scientific research that is conducted by members of the public, rather than professional scientists (Goodchild, 2007). Citizen science projects can be used to collect data on a wide variety of topics, from environmental conditions to astronomical events (Goodchild and Glennon, 2010; Haklay, 2010). Citizen science has been used in land-use mapping for many years (Goodchild, 2007; Antoniou et al., 2016). These maps are important tools for conservation planning, as they can help decision-makers understand where different types of ecosystems are located. Citizen science projects can contribute to the creation of these maps by collecting data on the location and extent of different types of land cover (Yang et al., 2017). Recent advances in technology have made it possible for citizen science projects to collect data more efficiently and accurately than ever before (Haklay and Weber, 2008; Neis and Zielstra, 2014; Yang et al., 2017). For example, the use of GPS devices and smartphones can allow citizen scientists to quickly and easily record the location of different types of land cover and use. In addition, online mapping tools can be used to share data with other project participants and mapmakers. Citizen science projects like these are important and useful for creating accurate and up-to-date land-use maps. Citizen science can increase our understanding of land-use patterns and management practices while engaging the public at local, regional, and global scales to study their environment (Goodchild, 2007; Haklay and Weber, 2008; Goodchild and Glennon, 2010; Theobald et al., 2015). For example, citizen-contributed data from New York Breeding Bird Atlas detected the potential for colonization, extinction, and the absence of bird species due to forest fragmentation (Zuckerberg and Porter, 2010). Involving citizen science to inform land-use management and conservation practices can usually lead to a more effective outcome of research success and land management practices, because it raises awareness and garners support for the project among the public (Dickinson et al., 2012; Yang et al., 2017).
Open Street Map® (OSM) (www.OpenStreetMap.org) is a collaborative project to create a free, editable map of the world. The maps are created by volunteer contributors using GPS devices, aerial photography, and other sources. OSM is used by individuals and organizations for a variety of purposes, including land-use planning, disaster response, and route planning (Haklay and Weber, 2008; Neis and Zielstra, 2014; Yang et al., 2017). OSM is one example of a citizen science project that relies on the voluntary contributions of its participants to create a useful resource for the community. Organizations such as the Red Cross and the United Nations Office for Coordination of Humanitarian Affairs use OSM data to plan relief efforts and respond to natural disasters. Businesses such as Foursquare and Craigslist use OSM data to provide location-based services. Non-profit groups such as Mapbox and OpenStreetMap US use OSM data to create maps and apps that are available to the public. The success of OSM depends on the continued participation of its contributors.
In this research, we collect historical crowdsourced data from OSM to map regional land use and then generate a land-use change map over a 5-year period to determine how land use and thus land management have changed. We then examine the proximity of different land uses to areas with four different forest management approaches to determine the spatial relationships of forest management with land uses. We focus on the SEUS region, which has high landscape heterogeneity, heavily managed forestlands, developed agriculture, and multiple metropolitan areas.
There are two main types of forest ownership in the southeastern United States: public and private. Public forests are owned by governments and are managed for the benefit of the public. Private forests are owned by individuals or companies and are managed for their own benefit. In SEUS, we define forest management types in four categories: production forestry, ecological forestry, wilderness or preservation, and passive forestry (Becknell et al., 2015; Marsik et al., 2018). Ecological management involves managing the forest in such a way as to not only realize gains from timber harvest but also maintain or improve the forest’s ecological health (Franklin et al., 2018). This may include activities such as planting native species, maintaining an uneven age structure so that forest structure will be more diverse, thinning non-native or invasive species, and reducing pollution. Passive management is a hands-off approach that generally involves leaving the forest to its own devices. This approach is often used in areas where the forest is not actively threatened by human activity or natural disasters, is in soils too wet, such as riparian areas, to support harvesting machinery, or belongs to owners who simply want to have an intact forest. Wilderness or preservation management, in contrast, seeks to protect the forest in its natural state and may be used in certain public forests where conservation is the primary goal. Each of these management approaches comes with its unique set of consequences and complexities on the landscape, affecting not only the biodiversity within the forests but also the patterns of land use in surrounding regions. Recent developments in cloud computing, collaborative mapping, and user-generated content platforms, such as volunteered geographic information (VGI), have spawned a new era in geographic visualization (geo-visualization or mapping and visualizing the world), such that the combination of crowdsourced mapping and cloud-based computing may overcome these difficulties (Southworth and Muir, 2021).
In this study, OSM is incorporated into mapping land use to identify and monitor land use at a regional scale. We use Google Earth Engine (GEE) to enable large-scale spatial analysis and image processing by providing a variety of Earth observation datasets and powerful computational resources (Haklay and Weber, 2008; Southworth and Muir., 2021). By focusing our prime objective on the mapping of land management practices, a robust, automated regional land-use mapping approach is developed by incorporating the OSM dataset with GEE’s available Earth observation imagery. Since the SEUS is heavily forested, the diverse land-use characteristics are often hidden under the canopy, which results in the land-use patterns of the SEUS not being visible with routine remote sensing methods. In this analysis, we incorporate anthropogenic Earth observation datasets (such as nightlight and ownership) with the crowdsourced OSM database to first create land-use maps for two dates: 2013 and 2018, across our study area, and to then create a land-use change analysis or transition image from these products. We then use these individual land-use maps for 2013 and 2018 to identify all forest patches, indicating forest management type as part of the patch type record, and highlight the fragmented nature of forest cover across this region as a function of extensive road networks (Reed et al., 1996; Heilman et al., 2002; Riitters et al., 2002). Finally, we then study the spatial relationships among these managed forest patches and the land use surrounding these patches as we increase in distance from the forest patch edge. By proposing a land-use change mapping framework, we aim to increase the accuracy of mapping land use and expand the ability to map land use at the regional scale. This analysis examines how the four dominant forest management types interact with the surrounding land use in the SEUS. In the heavily forested SEUS, we examine the spatial patterns of land uses surrounding forest ecosystem patches by asking the research question: “What are the land-use patterns extending outwards from differently managed forestlands and how do these change over time?”
The methods used within this study are highlighted in the flowchart (Figure 1) indicating the multiple input data and steps taken as part of this research. The specific data and details on the analysis are outlined below.
As a case for classifying land use and monitoring land-use change around public and private forests, we chose an area of the Southeastern U.S. Coastal Plain corresponding approximately with the Worldwide Reference Systems II (WRS-2) path 17 row 39 (P17 R39), with bounding latitude and longitude coordinates of approximately 31°13′N, 83°10′W (northwest corner), and 29°20′N, 81°41′W (southeast corner) (Figure 2). The study area covers an area of about 34,000 km2. The study area has a good representation of all types of land uses and forest management practices in the SEUS. This heterogeneous landscape consists of a mixture of natural and plantation forests, large and small wetlands, several rivers with extensive riparian areas, urban centers, urban and rural residential areas, and commercial and small-scale agricultural operations. This diversity of land-cover types is spatially heterogeneous, and patch sizes within the vegetation classes vary across a wide range of scales.
FIGURE 2. The map outlining the study region and the spatial distribution of forest-management types within the study region. The light gray part represents non-forest regions. Forest management classes were mapped by Marsik et al. (2018).
The integration of remote sensing and GIS presents a robust tool to monitor, quantify, and characterize landscape features in both time and space (Reed et al., 1996; Heilman et al., 2002; Riitters et al., 2002; Hawbaker and Radeloff, 2004; Espirito-Santo et al., 2014), making it a promising approach to land-use change mapping strategy design. The advent of Volunteered Geographic Information (VGI) platforms has ushered in a new era of mapping and visualizing land systems (Neis et al., 2011; Neils and Zielstra, 2014; Hakley, 2010). Notably, OpenStreetMap (OSM) is an excellent example of a VGI platform that facilitates the rapid expansion of big data and cloud-based computing while providing a more extensive range of applications than official geographic road databases (Zielstra and Hochmair, 2013). The use of OSM allows for the creation of more current and comprehensive maps that reflect temporal changes (Girres and Touya, 2010; Estima and Painho, 2013). Our land-use change mapping strategy design focuses on creating multi-functional management units, with urban areas being excluded from the study using urban boundaries from the TIGER database (TIGER, 2015).
There are several definitions made by OSM ODbL that need to be clarified, namely,: objects, tags, and keys. The attributes of OSM are called “tags”, and the major features stored in OSM are called “keys”. An OSM “object”, e.g., a building, a road, or a parcel, is composed of geographic location information and a set of “tags”. Each object in the OSM must have at least one tag, but there is no limit to the number of tags a specified object can have. The official list of OSM tags is available on the map features wiki page: https://wiki.OpenStreetMap.org/wiki/Map_Features. The tag taxonomy has been agreed upon over years of experience and is still being updated, which reflects a folksonomy approach based on a negotiation process among OSM contributors (Ballatore and Mooney, 2015). The “tags” can be reorganized, combined, and grouped in various semantic ways to highlight the geospatial distribution of different topics, and as such, they can be utilized in different research projects.
The full historical OSM database is available at https://planet.osm.org. In this study, historical OSM was accessed through the OSM API (https://wiki.OpenStreetMap.org/wiki/API), which allows us to fetch, save, and analyze the raw data from OSM over time. In our study area in 2013, there were 1,199 objects representing buildings on the OSM in our study area, the number changed to 66,488 by October 2018. By 2018, there were more than 486 contributors who updated and edited the roads feature in the study area, 230 contributors fixed the building features OSM and 262 contributors to amenities features.
In OSM the land-use tags are often marked with different understandings of the land, e.g., for rangeland, it was marked as “yellow field” or “farm” and even “grass”. The relationship between OSM tags and labels was built based on the land-use classification strategy with a framed dictionary (Table 1). To achieve this, we converted OSM tags to five land-use classes: cropland/rangeland, commercial/industrial, managed forest, residential areas and water body. We then regrouped the OSM tags into those targeted classes based on the framed dictionary (Table 1).
The OSM condensed land use definitions in this study are:
1) Cropland/rangeland—A land-use category, which is used to produce crops or has the potential for sustainable grazing (native grasses, grass-like vegetation, shrubs).
2) Commercial/Industrial—A land-use category consisting of industrial, commercial, and institutional land, construction sites, public administrative sites, railroad yards, cemeteries, airports, golf courses, quarries, water control structures (Wear and Greis, 2013).
3) Managed Forests—A land-use category that is covered with forest. The categories of forest management are generally consistent with the forest management type map produced by the MANDIFORE group (Marsik et al., 2018). We also included the areas that showed evidence of the natural regeneration of trees and not currently developed for non-forest use.
4) Residential—A land-use category consisting of single- or multi-family residential, apartment buildings, and small parks within the urban and built-up areas.
5) Waterbody—Open water
Several different Earth observation datasets were used in the analysis, including.
1. We used Landsat data for 1/1/2013 and 8/1/2018, available on the GEE API (Google Earth engine ID: LANDSAT/LC8_L1T_ANNUAL_GREENEST_TOA). These Collection 2 Landsat 8 Operational Land Imager (OLI) data have a spatial resolution of 30-m and are Top-of-atmosphere (TOA) and Landsat 5 TOA reflectances (Chander et al., 2009).
2. We used global forest canopy height, version 2005 (Simard et al., 2011): This dataset represents the canopy height at a global level by incorporating the Geoscience Laser Altimeter System (GLAS) and ancillary data (Google Earth engine ID: NASA/JPL/global_forest_canopy_height_2005)
3. Nighttime satellite imagery was used as proxies of mapping human wellbeing and urban development (Sutton et al., 2009; Li et al., 2017) (Google Earth engine ID: NOAA/VIIRS/DNB/MONTHLY_V1/VCMSLCFG). VIIRS Stray Light Corrected Nighttime Day/Night Band Composites Version 1 (Miller et al., 2013): The dataset of nighttime has the global monthly aggregated nightlight time data.
4. Land ownership is one of the key factors delineating land use and forest management, especially under the currently ongoing rapid urbanization and increasing rural development, which may affect and alter the forest management patterns. Landowners are classified as public and private. There are six sub-types of public ownership, which are federally protected, federal, state protected, state, military, and local. Also, there are four sub-types of private ownership: non-government organization, private, family, and corporate. The ownership classification strategy is made based on different management objectives, as well as landowner skills, budgets, and interests. The Protected Area Database for the United States (PAD-US) is the primary data source to identify public ownership (USGS-PADUS-2.0).
We built a Random Forest (RF) classifier by incorporating multiple remote sensing datasets as covariates, using GEE as the mapping platform, and crowdsourcing-derived geotags as training sample databases. We used OSM derived data as training points to extract spectral statistics for use in the RF classifier (Breiman, 2001). The efficiency and accuracy of the RF classifier have been widely tested and recorded throughout regional landscape mapping. The principle of RF is to apply a bootstrapping aggregated sampling technique to build a series of individual decision trees for the classifier. The major advantages of the RF classifier are the capability of handling a large number of training samples, its efficiency in dealing with the large regional database, and its robustness to outliers and noise. To remove the noise from the classification outputs, we used a 3 × 3 cell majority filter for all land-use classes, except for waterbodies for both the 2013 and 2018 images. All images were resampled to 30 m spatial resolution using the nearest-neighbor filter algorithm.
The total number of OSM-derived training samples are 3,150 and 3,870 for 2013 and 2018, respectively. We specified two sets of 10-fold cross internal validations for the RF classifier and assessed the individual contribution from each land-use type to the overall accuracy of the land-use pattern maps. To optimize the RF algorithm, we utilized 500 trees, a 70/30 split for training/validation, and modified other parameters. These parameters were carefully selected to balance model complexity and accuracy and to avoid overfitting. Our results demonstrate the effectiveness of the RF algorithm in accurately classifying land use from remote sensing data. Furthermore, we present a novel approach for generating training data using existing land use features, which enhances the accuracy and efficiency of the classification process. Overall, it highlights the potential of the RF algorithm for land use classification in complex and diverse landscapes.
First the forest patches, defined as groups of contiguous pixels of the same management type separated from other groups by other management types or non-forest pixels, were identified spatially, and the size characteristics of each patch were determined for both 2013 and 2018, as follows: For each forest patch the forest management type (ecological, passive, preservation or production) was recorded. To test the land-use variations around each type of managed forest, a series of spatial analyses were conducted. We created nine buffers at increasing distances from the edge of the forest patches, from 500 m to 5,000 m at an interval of 500 m. The reason to pick a buffer size ranging from 500 m to 5,000 m is based on the average patch size from the management map (Figure 2; Table 2). In the study area, the overall average management patch size is 26.6 ha, which leads to the appropriate minimum buffer rings starting at around 360 m, according to the methods in Defries et al. (2007). However, from Table 2, as the size range of management patches is variable, we set nine other increasing buffers to allow for an improved analysis of the landscape. The land-use combinations of 2013 and 2018 of cropland, residential areas, and commercial/industrial areas were compared by plotting the proportion of each land-use type in the different buffer distances. At larger scales of analysis, buffer overlap could influence the independence of samples; however, after calculating the maximum buffer overlap with other forested patches, which was 6.8% of the total study areas, we assume a minimal impact of buffer overlap regionally.
Land use maps for 2013 (Figure 3) and 2018 (Figure 4) are given here. Since the urban areas were masked, residential areas represent the exurban and rural residential areas. There are significant differences between the two study dates, despite their only being 5 years apart. The proportion of the landscape in the residential area increased from 4.93% to 8.87%. For the commercial/industrial class, an increase from 0.59% to 2.97% was found, and a similar trend was found with the cropland/rangeland class with an increase of 6.22%–10.32% (Table 3).
FIGURE 3. Land-Use Composition of the study area in 2013. Because the urban areas were subtracted, the residential and commercial/industrial areas are those located in the suburban and rural residential areas.
FIGURE 4. Land-Use Composition of the study area in 2018. Because the urban areas were subtracted, the residential and commercial/industrial areas are those located in the suburban and rural residential areas.
TABLE 3. Land-Use Change of Residential, commercial/industrial, and cropland/rangeland from 2013 to 2018.
From Figure 5 and Table 3, we found a strong trend of deforestation and rural residential/suburban development, which increased by 80%. There was also a 66%increase in cropland area and a 407% increase in commercial/industrial area in the study area from 2013 to 2018.
FIGURE 5. Land-Use Change of Residential, commercial/industrial, and cropland/rangeland from 2013 to 2018.
Building the RF classifier is the starting point of our landscape analysis; the results of the accuracy of land-use classification for both 2013 and 2018 are shown in Table 4. The land-use classification out-of-bag (OOB) overall accuracy was close to 97%, with corresponding Kappa values of 0.97 for both 2013 and 2018. Considering that land-use patterns of 2013 and 2018 were extracted using the same classification method and original image collections, we assumed that the classification accuracies of this land-use change dataset are comparable.
TABLE 4. Out-Of-Bag (OOB) error matrices for five land-uses classes in 2013 and 2018 in the study area.
At the class level, the precision, or comparison of the true and false classifications, ranges from 94% to 99%. Overall, the RF classification rules, when coupled with crowd-sourced land-use training samples show a strong potential to classify land-use type well. In land-use mosaics from 2013, it was shown that the most complex land-use changes occur at cropland/rangeland and residential areas. This reflects the existence of rural residential areas as well as their continued development. The residential regions and the commercial/industrial areas are the classes in the 2018 land-use mosaics that have the most confusion. This indicates that it is difficult to differentiate between those two classes due to the high similarity of spectral signals in remote sensing imagery.
The percentage coverage of the five land uses adjacent to and within different buffers for each type of forest management approach from 2013 to 2018 is individually shown in Figures 3, 4 and is compared across the buffered region. We set the distance zones surrounding patches of each management from 500 m to 5,000 m with a 500 m interval, as those distances will cover enough spatial information to show the subtle land-use change at a landscape level (Sanchez Azofeifa, 1996; Carey et al., 2011). Adjacent land-uses surrounding each forest management type were analyzed. The results gave us an indication of the distribution of each land-use class immediately around different managed forest patches.
For the landscapes in proximity to ecological forests, there is not a strong spatial relationship between the ecologically managed forest and the surrounding lands, although there is more residential land near ecological forests and that cropland is more likely to be near ecological forest management patches (Figure 6). The proportions of cropland, commercial/industrial and residential land uses decrease within 1,500 m and then are stable out to 5,000 m. For landscapes in proximity to passively managed forests, the surrounding land uses are quite stable surrounding the passive management forests, except for the croplands.
FIGURE 6. The percentage of different land use based on the distances from the nearest forest patches under different management types (ecological, passive, preservation, and production forest).
In Figure 6, few spatial relationships between land uses and management are found within 500 m of preservation forestry, which fulfills the preservation management strategy of sustaining the forests. From 2013 to 2018, cropland and residential area proportions increased with increasing distance from the preservation forests. Among all four types of management approaches, production forests have the strongest spatial relationships with croplands (Figure 6). The proportion of the croplands surrounding production forests reached a peak of 20% at a distance of 1,000 m, and then decreased rapidly. When the distance from production forests is about 3,500 m, the residential proportion did not change from 2013 to 2018.
As evident in Figure 6, there tends to be a sharp increase in land-use proportion among different types of land use adjacent to each management forest patch (except for cropland in 4,000–5,000 m proximity of production forests). In 2013, cropland land uses showed the strongest spatial relationships with production forest, followed by passively managed forest, ecological forest, and finally, preservation forest. However, in 2018, the order changed significantly with the strongest spatial relationships with production forests, followed by ecological forest, and then passively managed forest and preservation forests. In 2013, the proportion of cropland surrounding production forest patches increased from 3.3% to 8.6%. However, the trend in 2018 shows an exponential decrease with increasing distance, particularly from 1,000 m to 3,500 m.
Land use transitions are important to map and understand (Figure 7). Table 5 shows the different transitions possible across the study area with the associated land change. Notably, the dominant land transitions were identified into seven major categories: stability (no change (stability), commercialization, afforestation, cultivation, and rural residential and suburban growth. Table 5 also shows the land-use conversion matrix used by the spatial allocation procedure by determining the possible land-use transition sequences. Just four types from the land-use patterns map were used in this analysis: cropland, commercial/industrial, managed forest, and residential.
TABLE 5. Land-use changes in the study area and the associated land change are delineated. These categories include changes that may rarely or never occur (e.g., residential to managed forest or commercial/industrial or residential to croplands).
We created a land transition analysis by combining the OSM derived land-use maps for 2013 and 2018 to produce the land-use transition patterns over the study area with a 30 m resolution. This regional land-use pattern analysis seeks to identify the dominant patterns of land use change. Across the study area, several major change patterns are recognized, together comprising a majority of suburban growth, commercialization, and cultivation.
OSM was incorporated into mapping land use and showed great potential for justifying and monitoring land use at a regional scale. The results identified the spatial land-use patterns and the variations around each type of managed forest with buffers ranging from 500 m to 5,000 m for 2013 and 2018 (Figures 3, 4). Over the 34,000 km2 study area, there has been a large amount of land-use conversion from 2013 to 2018 (Figure 7). Deforestation is a clear trend in the study area, despite economically valuable managed forests.
The nightlight remotely sensed images do a relatively better job representing and mapping human footprints (Li et al., 2017) when compared to using only the spectral imagery alone. The results of this study support the use of nightlight imagery when mapping land use. For example, Yang et al., 2017 used a variety of physical features from Earth observation datasets (i.e., forest canopy height, DEM, and EVI) to map regional land use in the same study area with an accuracy of 95% (internal validation) and 74% (external validation).
In this study, land-use classifiers’ training sample sets were extracted from historical OSM and resulted in high internal validation accuracy (97% for 2013 and 97% for 2018). OSM provides different data collection mechanisms than the traditional authoritative geographic information obtained from official or governmental institutions, agencies, or Earth observations. The results demonstrate that OSM and citizen science data have potential for mapping regional human footprints (represented as land use in this study). In this study, the training samples extracted from OSM were randomly selected. The error matrices are based on internal validation. Based on our results, the classification methods presented in this study are recommended mainly for mapping broad land-use classes. Further accuracy testing through external validation requires a large amount of historical land-use documentary data, which can be a project for further study.
The data quality of OSM and its public participatory geospatial database has always been recognized as a major concern by researchers (Antoniou and Skopeliti., 2015; Mobasheri et al., 2018). The contribution of OSM mappers is often based on perceptions rather than scientific measurements, which makes it complex to measure the mapping quality and positional accuracy. However, there are some strategies to overcome the credibility challenges of those participatory mapping databases. Firstly, there are always “superusers” in VGI mapping projects. Those “superusers” make tremendous contributions by providing a large amount of near-real time accurate information. In addition, the quality control of OSM itself is also a multi-user environmental validation process. Based on its “wiki” principle, the community of OSM mappers can act as quality filters, which means the dataset is self-validated by the other contributors’ numerous times. In this analysis, we applied this strategy to the point of self-validation. Finally, because of the vast amount of OSM data, mapping effects are mostly aggregated based on the ground truth data provided by OSM mappers.
Due to the lack of precision in delineating boundaries that surround various ecosystems, there is much that cannot be clearly understood from the perspective of land management or the management of natural resources (Duncker et al., 2012). Our results show that there are similar trends in management for lands around ecological forests and passively managed forest, i.e., no significant increase or decrease of different land-use types in surrounding landscapes. We also found that for passively and ecologically managed forests, as the distances of specific land-use types from the nearest forest patches increase, the variance of the proportion of that specific land use to the total land becomes smaller, and finally levels off. Preservation forestry covers over thirty percent of the total forest in the study area (Marsik et al., 2018). For surrounding land uses of production forests, we found patterns that imply interactions between production forests and cropland in the 1,500 m buffer. Land policy may contribute to this phenomenon as production forests, pasture, cropland, and citrus all belong to the same tax code (i.e., Commercial Agriculture Uses) in Florida, which lowers the property tax rate. There are several hypotheses to explain this phenomenon: 1) the landscapes are built out over a distance greater than 3,500 m; and 2) those areas belong to urban areas and are thus not included in the analysis. Moreover, the State of Florida does not have a minimum land area requirement for agricultural and timberland classification, which increases the potential for cropland—timberland two-way flows.
Residential developments also show a strong effect of land-use transitions on the surrounding lands, such as forests transformed into croplands or rangeland areas. As we found the increasing trend on both residential and commercial/industrial lands, nearly 45.9% of new residential lands in the study area were created from forests, with most of the rest resulting from the conversion of agricultural land. From 2013 to 2018, one of the most influential drivers of forest area change was the expansion and contraction of agricultural land. These patterns also point out how fast the rural residential areas are being developed in the heavily forested study area, especially near passive and production management forest patches (Figure 6).
The study area also experienced rapid population growth, based on the gridded population of the world v4/population count, there was a 170,130 increase from 2010 to 2020 (GPWv4). Most of the time this led to deforestation (the transfer of forest lands to developed lands). As the population increased, the potential need for residential areas, roads, commercial and industrial sites increased. The development of rural residential developments significantly contributed to land-use dynamics. The increasing rural residential areas show a strong signal of urbanization and deforestation, as most newly developed residential areas are developed on forested land. For the rural/suburban residential areas surrounding ecological forests, preservation forests, and passively managed forests, the trends of rural/suburban coverage all increased from 2013 to 2018.
The land uses in SEUS have been heavily transformed according to a variety of factors, such as population growth and economic growth. The forest ecosystems in the SEUS have been largely influenced by these land-use changes. The results of this study show that land-use patterns in the vicinity of forest under different management strategies vary substantially with the occurrences of forest patch isolation due to the proximity of agricultural development, rural residential development, and commercialization. Such land-use transitions alter the SEUS landscapes and may affect ecosystem functions. We infer the land-use information at the regional scales by using VGI from a diverse array of stand-level studies and other ancillary information.
By developing a crowdsourcing-based land-use change mapping framework over the SEUS we measured and calculated the proportion of land area that was located within nine increasing distance buffers from the nearest managed forest patches of any type of forest management, and mapped the results reclassified by land-use transitions. For lands surrounding preservation forests, we found the effectiveness of preservation in maintaining the forest cover in the first 500–1,000 m boundary buffers. However, rural residential developments are changing the lands surrounding the preservation areas. The lands surrounding production forestry, comprising important spatial relationships with croplands, show the strongest potential for land conversion between forests and croplands. For the passively managed forest surrounding landscapes, the land-use patterns represent a relatively neutral status because of the low management intensity with little interaction with croplands, which is the opposite for the production forest surroundings. For the lands surrounding ecologically managed forests, there are the least variances of land-use composition based on distances. This also supports the idea that the major principle of ecological forestry is to maintain the social-ecological functions.
Citizen science is contributing to land-change science, in ways that increase the magnitude of observations far beyond those that can be done by individual scientific projects. From a mapping land-use perspective, citizen science can be used to extend the training sample database, which is considered a huge challenge for large scale landscape classification processes. The proposed strategies seek contributions that demonstrate the application of citizen science projects supporting human-environment related research by complementing satellite observations and discussing novel methods for the collection of land management data.
The challenge of the work is that the rapid growth of OSM only started in 2013, and as such, the database has improved from both user numbers and quality perspectives, across the study period. From 2013 to 2018 is a relatively short time, only 5 years, to make a significant conclusion or assessment about any types of longer-term changes or drivers. Despite this limitation due to the short time-duration of this data source, however, this study shows a strong potential for mapping land change and human footprints at the regional scale by using VGI derived datasets as land-use indicators and proxies.
The datasets presented in this study can be found in online repositories. The names of the repository/repositories and accession number(s) can be found below: https://doi.org/10.6084/m9.figshare.11406612.v1.
Conceptualization: DY and C-SF Methodology and software: DY Validation: C-SF Formal analysis: DY and C-SF Materials: DY Writing—original draft preparation: DY Writing—review and editing: DY, C-SF, HH, JS, MB Visualization: DY, C-SF, and MB Supervision: JS, and MB Funding acquisition: DY led the conceptualization of the research study and was primarily responsible for developing the methodology and software used in the study. DY wrote the initial draft of the manuscript and was heavily involved in the writing and editing process throughout. DY was also involved in visualization of the data. C-SF contributed to the conceptualization of the study, led the validation of the results, and provided overall supervision and guidance throughout the research process. They were involved in writing and editing the manuscript and securing funding for the research. HH and JS contributed to the writing and editing of the manuscript. MB contributed to the supervision of the research study, provided overall guidance and leadership throughout the research process. He also involved in writing and editing of the manuscript. All authors contributed to the article and approved the submitted version.
The National Science Foundation (NSF #EF-1231860, and #EF-1702835, NSF-2305683) for the generous support of this project.
We extend our deepest gratitude to the contributors of OpenStreetMap, whose tireless efforts have provided invaluable data for our geographical analyses. We also wish to express our appreciation to Google Earth Engine for their innovative platform that has significantly enhanced our research capabilities. Special thanks are due to Peter Waylen and Greg Glass for their insightful reviews and constructive feedback.
The authors declare that the research was conducted in the absence of any commercial or financial relationships that could be construed as a potential conflict of interest.
All claims expressed in this article are solely those of the authors and do not necessarily represent those of their affiliated organizations, or those of the publisher, the editors and the reviewers. Any product that may be evaluated in this article, or claim that may be made by its manufacturer, is not guaranteed or endorsed by the publisher.
Antoniou, V., Costa Fonte, C., See, L., Estima, J., Arsanjani, J., Lupia, F., et al. (2016). Investigating the feasibility of geo-tagged photographs as sources of land cover input data. ISPRS Int. J. Geo-Information 5 (5), 64. doi:10.3390/ijgi5050064
Antoniou, V., and Skopeliti, A. (2015). Measures and indicators of vgi quality: An overview. ISPRS Ann. Photogrammetry, Remote Sens. Spatial Inf. Sci. 2, 345–351. doi:10.5194/isprsannals-ii-3-w5-345-2015
Ballatore, A., and Mooney, P. (2015). Conceptualising the geographic world: The dimensions of negotiation in crowdsourced cartography. Int. J. Geogr. Inf. Sci. 29 (12), 2310–2327.
Becknell, J. M., Desai, A. R., Dietze, M. C., Schultz, C. A., Starr, G., Duffy, P. A., et al. (2015). Assessing interactions among changing climate, management, and disturbance in forests: A macrosystems approach. BioScience 65 (3), 263–274. doi:10.1093/biosci/biu234
Briassoulis, H. (2020). Analysis of land use change: Theoretical and modeling approaches. 2nd edn. Edited by Scott Loveridge and Randall Jackson. WVU Research Repository.
Carey, R. O., Migliaccio, K. W., Li, Y., Schaffer, B., Kiker, G. A., and Brown, M. T. (2011). Land use disturbance indicators and water quality variability in the Biscayne Bay Watershed, Florida. Ecol. Indic. 11 (5), 1093–1104. doi:10.1016/j.ecolind.2010.12.009
Chander, G., Markham, B. L., and Helder, D. L. (2009). Summary of current radiometric calibration coefficients for Landsat MSS, TM, ETM+, and EO-1 ALI sensors. Remote Sens. Environ. 113 (5), 893–903. doi:10.1016/j.rse.2009.01.007
Comber, A. J. (2008). The separation of land cover from land use using data primitives. J. Land Use Sci. 3 (4), 215–229.
DeFries, R., Hansen, A., Turner, B. L., Reid, R., and Liu, J. (2007). Land use change around protected areas: Management to balance human needs and ecological function. Ecol. Appl. 17 (4), 1031–1038. doi:10.1890/05-1111
Dickinson, J. L., Shirk, J., Bonter, D., Bonney, R., Crain, R. L., Martin, J., et al. (2012). The current state of citizen science as a tool for ecological research and public engagement. Front. Ecol. Environ. 10 (6), 291–297.
Duncker, P. S., Barreiro, S. M., Hengeveld, G. M., Lind, T., Mason, W. L., Ambrozy, S., et al. (2012). Classification of forest management approaches: A new conceptual framework and its applicability to European forestry. Ecol. Soc. 17 (4).
Dunford, W., and Freemark, K. (2005). Matrix matters: Effects of surrounding land uses on forest birds near ottawa, Canada. Landsc. Ecol. 20 (5), 497–511. doi:10.1007/s10980-004-5650-5
Espírito-Santo, F. D., Gloor, M., Keller, M., Malhi, Y., Saatchi, S., Nelson, B., et al. (2014). Size and frequency of natural forest disturbances and the Amazon forest carbon balance. Nat. Commun. 5, 3434. doi:10.1038/ncomms4434
Estima, J., and Painho, M. (2013). “Exploratory analysis of openStreetMap for land use classification,” in Paper presented at The Second ACM SIGSPATIAL International Workshop on Crowdsourced and Volunteered Geographic Information, GEOCROWD’13, Orlando, FL, United States, November 5–8, 39–46.
Fahrig, L. (2013). Rethinking patch size and isolation effects: The habitat amount hypothesis. J. Biogeogr. 40 (9), 1649–1663. doi:10.1111/jbi.12130
Fischlin, A., Midgley, G. F., Price, J. T., Leemans, R., Gopal, B., Turley, C., et al. (2007). Ecosystems, their properties, goods and services.
Forman, R. T. T. (2014). Land mosaics: The ecology of landscapes and regions 1995. Island Press, 217.
Franklin, J. F., Norman Johnson, K., and Johnson, D. L. (2018). Ecological forest management. Waveland Press.
Girres, J. F., and Touya, G. (2010). Quality assessment of the French OpenStreetMap Dataset. Trans. GIS. 14 (4), 435–459.
Goodchild, M. F. (2007). Citizens as sensors: The world of volunteered geography. GeoJournal 69 (4), 211–221. doi:10.1007/s10708-007-9111-y
Goodchild, M. F., and Glennon, J. A. (2010). Crowdsourcing geographic information for disaster response: A research frontier. Int. J. Digital Earth 3 (3), 231–241. doi:10.1080/17538941003759255
Groeneveld, J., Müller, B., Buchmann, C. M., Dressler, G., Guo, C., Hase, N., et al. (2017). Theoretical foundations of human decision-making in agent-based land use models – a review. Environ. Model. Softw. 87, 39–48. doi:10.1016/j.envsoft.2016.10.008
Haklay, M. (2010). How good is volunteered geographical information? A comparative study of OpenStreetMap and ordnance survey datasets. Environ. Plan. B Plan. Des. 37 (4), 682–703. doi:10.1068/b35097
Haklay, M., and Weber, P. (2008). Openstreetmap: User-generated street maps. Ieee Pervas Comput. 7 (4), 12–18. doi:10.1109/mprv.2008.80
Hansen, A. J., and Ruth, D. F. (2007). Ecological mechanisms linking protected areas to surrounding lands. Ecol. Appl. 17 (4), 974–988. doi:10.1890/05-1098
Hawbaker, T. J., and Radeloff, V. C. (2004). Roads and landscape pattern in northern Wisconsin based on a comparison of four road data sources. Conserv. Biol. 18 (5), 1233–1244. doi:10.1111/j.1523-1739.2004.00231.x
Heilman, G. E., Strittholt, J. R., Slosser, N. C., and Dellasala, D. A. (2002). Forest fragmentation of the conterminous United States: Assessing forest intactness through road density and spatial characteristics. AIBS Bull. 52 (5), 411–422. doi:10.1641/0006-3568(2002)052[0411:ffotcu]2.0.co;2
Hurskainen, P., Adhikari, H., Siljander, M., Pellikka, P. K. E., and Hemp, A. (2019). Auxiliary datasets improve accuracy of object-based land use/land cover classification in heterogeneous savanna landscapes. Remote Sens. Environ. 233, 111354. doi:10.1016/j.rse.2019.111354
Kramer, D. B., and Doran, P. J. (2010). Land conversion at the protected area's edge. Conserv. Lett. 3 (5), 349–358. doi:10.1111/j.1755-263x.2010.00122.x
Lambin, E. F., and Meyfroidt, P. (2011). Global land use change, economic globalization, and the looming land scarcity. Proc. Natl. Acad. Sci. 108 (9), 3465–3472. doi:10.1073/pnas.1100480108
Lawler, J. J., Lewis, D. J., Nelson, E., Plantinga, A. J., Polasky, S., Withey, J. C., et al. (2014). Projected land-use change impacts on ecosystem services in the United States. Proc. Natl. Acad. Sci. 111 (20), 7492–7497. doi:10.1073/pnas.1405557111
Li, X., Elvidge, C., Zhou, Y., Cao, C., and Warner, T. (2017). Remote sensing of night-time light. Int. J. Remote Sens. 38 (21), 5855–5859. doi:10.1080/01431161.2017.1351784
Marsik, M., Staub, C. G., Kleindl, W. J., Hall, J. M., Fu, C. S., Yang, D., et al. (2018). Regional-scale management maps for forested areas of the Southeastern United States and the US Pacific Northwest. Sci. data 5, 180165. doi:10.1038/sdata.2018.165
Miller, S. D., Straka, W., Mills, S. P., Elvidge, C. D., Lee, T. F., Solbrig, J., et al. (2013). Illuminating the capabilities of the suomi national polar-orbiting partnership (NPP) visible infrared imaging radiometer suite (VIIRS) day/night band. Remote Sens. 5 (12), 6717–6766. doi:10.3390/rs5126717
Mobasheri, A., Zipf, A., and Francis, L. (2018). OpenStreetMap data quality enrichment through awareness raising and collective action tools—Experiences from a European project. Geo-spatial Inf. Sci. 21 (3), 234–246. doi:10.1080/10095020.2018.1493817
Neis, P., and Zielstra, D. (2014). Recent developments and future trends in volunteered geographic information research: The case of OpenStreetMap. Future Internet 6 (1), 76–106. doi:10.3390/fi6010076
Neis, P., Zielstra, D., and Zipf, A. (2011). The street network evolution of crowdsourced maps: OpenStreetMap in Germany 2007–2011. Future Internet 4 (1), 1–21.
Radeloff, V. C., Stewart, S. I., Hawbaker, T. J., Gimmi, U., Pidgeon, A. M., Flather, C. H., et al. (2010). Housing growth in and near United States protected areas limits their conservation value. Proc. Natl. Acad. Sci. 107 (2), 940–945. doi:10.1073/pnas.0911131107
Reed, R. A., Johnson Barnard, J., and Baker, W. L. (1996). Contribution of roads to forest fragmentation in the Rocky Mountains. Conserv. Biol. 10 (4), 1098–1106. doi:10.1046/j.1523-1739.1996.10041098.x
Riitters, K. H., Wickham, J. D., O'neill, R. V., Jones, K. B., Smith, E. R., Coulston, J. W., et al. (2002). Fragmentation of continental United States forests. Ecosystems 5 (8), 0815–0822.
Sanchez-Azofeifa, G. A. (1996). Assessing land use/cover change in Costa Rica. University of New Hampshire.
Schulte, L. A., Mladenoff, D. J., Crow, T. R., Merrick, L. C., and Cleland, D. T. (2007). Homogenization of northern US Great Lakes forests due to land use. Landsc. Ecol. 22 (7), 1089–1103. doi:10.1007/s10980-007-9095-5
Simard, M., Pinto, N., Fisher, J. B., and Baccini, A. (2011). Mapping forest canopy height globally with spaceborne lidar. J. Geophys. Res. Biogeosciences 116 (G4), G04021. doi:10.1029/2011jg001708
Sohl, T., and Sayler, K. (2008). Using the FORE-SCE model to project land-cover change in the southeastern United States. Ecol. Model. 219 (1-2), 49–65. doi:10.1016/j.ecolmodel.2008.08.003
Southworth, J., and Muir, C. (2021). Specialty grand challenge: Remote sensing time series analysis. Front. Remote Sens. 2. doi:10.3389/frsen.2021.770431
Sutton, P. C., Anderson, S. J., Elvidge, C. D., Tuttle, B. T., and Ghosh, T. (2009). Paving the planet: Impervious surface as proxy measure of the human ecological footprint. Prog. Phys. Geogr. 33 (4), 510–527.
Theobald, E. J., Ettinger, A. K., Burgess, H. K., DeBey, L. B., Schmidt, N. R., Froehlich, H. E., et al. (2015). Global change and local solutions: Tapping the unrealized potential of citizen science for biodiversity research. Biol. Conserv. 181, 236–244. doi:10.1016/j.biocon.2014.10.021
TIGER (2015). Cartographic boundary – urban areas. prepared by the U.S. Census Bureau. Available from https://www.census.gov/geo/maps-data/data/kml/kml_ua.html.
Turner, B. L. (1994). Local faces, global flows: The role of land use and land cover in global environmental change. Land Degrad. Dev. 5 (2), 71–78. doi:10.1002/ldr.3400050204
Turner, M. G., Wear, D. N., and Flamm, R. O. (1996). Land ownership and land-cover change in the southern Appalachian highlands and the Olympic Peninsula. Ecol. Appl. 6 (4), 1150–1172. doi:10.2307/2269599
Verburg, P. H., Soepboer, W., Veldkamp, A., Limpiada, R., Espaldon, V., and Mastura, S. S. (2002). Modeling the spatial dynamics of regional land use: The CLUE-S model. Environ. Manag. 30 (3), 391–405. doi:10.1007/s00267-002-2630-x
Verburg, P. H., Van De Steeg, J., Veldkamp, A., and Willemen, L. (2009). From land cover change to land function dynamics: A major challenge to improve land characterization. J. Environ. Manag. 90 (3), 1327–1335. doi:10.1016/j.jenvman.2008.08.005
Wassenaar, T., Gerber, P., Verburg, P. H., Rosales, M., Ibrahim, M., and Steinfeld, H. (2007). Projecting land use changes in the Neotropics: The geography of pasture expansion into forest. Glob. Environ. Change 17 (1), 86–104. doi:10.1016/j.gloenvcha.2006.03.007
Wear, D. N., and Greis, J. G. (2002). Southern forest resource assessment: Summary of findings. J. For. 100 (7), 6–14.
Wear, D. N., and Greis, J. G. (2012). “The southern forest futures project: Summary report,” in Gen. Tech. Rep. SRS-GTR-168 (Asheville, NC: USDA-Forest Service, Southern Research Station), 168, 1–54.
Wear, D. N., and Greis, J. G. (2013). “The southern forest futures project: Technical report,” in Gen. Tech. Rep. SRS-GTR-178 (Asheville, NC: USDA-Forest Service, Southern Research Station), 178, 1–542.
Yang, D., Fu, C. S., Smith, A. C., and Yu, Q. (2017). Open land-use map: A regional land-use mapping strategy for incorporating OpenStreetMap with Earth observations. Geo-spatial Inf. Sci. 20 (3), 269–281. doi:10.1080/10095020.2017.1371385
Zielstra, D., and Hochmair, H. H. (2011). Digital street data: Free versus proprietary. GIM Int. 25, 29–33.
Keywords: citizen science, machine learning, land use, land cover change, google earth engine (GEE), VGI (volunteered geographic information), forest management, landscape ecology
Citation: Yang D, Fu C-S, Herrero HV, Southworth J and Binford M (2023) Linking forest management to surrounding lands: a citizen-based approach towards the regional understanding of land-use transitions. Front. Remote Sens. 4:1197523. doi: 10.3389/frsen.2023.1197523
Received: 31 March 2023; Accepted: 04 July 2023;
Published: 13 July 2023.
Edited by:
Rajesh Bahadur Thapa, International Centre for Integrated Mountain Development, NepalReviewed by:
Chengcheng Gang, Chinese Academy of Sciences (CAS), ChinaCopyright © 2023 Yang, Fu, Herrero, Southworth and Binford. This is an open-access article distributed under the terms of the Creative Commons Attribution License (CC BY). The use, distribution or reproduction in other forums is permitted, provided the original author(s) and the copyright owner(s) are credited and that the original publication in this journal is cited, in accordance with accepted academic practice. No use, distribution or reproduction is permitted which does not comply with these terms.
*Correspondence: Di Yang, ZHlhbmcxQHV3eW8uZWR1
Disclaimer: All claims expressed in this article are solely those of the authors and do not necessarily represent those of their affiliated organizations, or those of the publisher, the editors and the reviewers. Any product that may be evaluated in this article or claim that may be made by its manufacturer is not guaranteed or endorsed by the publisher.
Research integrity at Frontiers
Learn more about the work of our research integrity team to safeguard the quality of each article we publish.