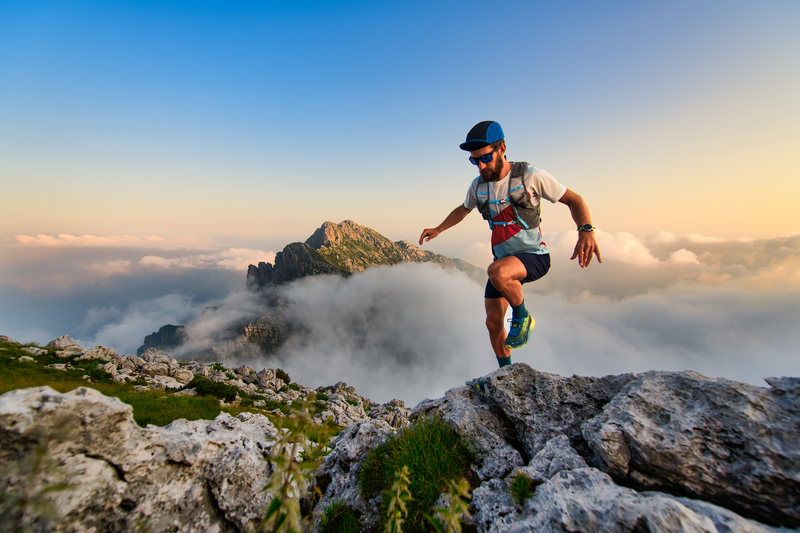
94% of researchers rate our articles as excellent or good
Learn more about the work of our research integrity team to safeguard the quality of each article we publish.
Find out more
ORIGINAL RESEARCH article
Front. Remote Sens. , 27 July 2023
Sec. Lidar Sensing
Volume 4 - 2023 | https://doi.org/10.3389/frsen.2023.1194580
This article is part of the Research Topic Lidar and Ocean Color Remote Sensing for Marine Ecology View all 5 articles
Introduction: Space-borne lidar measurements from sensors such as CALIOP were recently used to retrieve the particulate back-scattering coefficient, bbp, in the upper ocean layers at a global scale and those observations have a strong potential for the future of ocean color with depth-resolved observations thereby complementing the conventional ocean color remote sensed observations as well as overcoming for some of its limitations. It is critical to evaluate and validate the space-borne lidar measurements for ocean applications as CALIOP was not originally designed for ocean applications. Few validation exercises of CALIOP were published and each exercise designed its own validation protocol. We propose here an objective validation protocol that could be applied to any current and future space-borne lidars for ocean applications.
Methods: We, first, evaluated published validation protocols for CALIOP bbp product. Two published validation schemes were evaluated in our study, by using in-situ measurements from the BGC-Argo floats. These studies were either limited to day- or nighttime, or by the years used or by the geographical extent. We extended the match-up exercise to day-and nighttime observations and for the period 2010–2017 globally. We studied the impact of the time and distance differences between the in-situ measurements and the CALIOP footprint through a sensitivities study. Twenty combinations of distance (from 9-km to 50-km) and time (from 9 h to 16 days) differences were tested.
Results & Discussion: A statistical score was used to objectively selecting the best optimal timedistance windows, leading to the best compromise in term of number of matchups and low errors in the CALIOP product. We propose to use either a 24 h/9 km or 24 h/15 km window for the evaluation of space-borne lidar oceanic products.
Satellite ocean color observations are used successfully by the scientific community for studying the ecosystem of the oceans and to document temporal changes induced by anthropogenic activities as well as climatic conditions. Passive ocean color remote sensing has been continuously operational for more than two decades (McClain, 2009; Groom et al., 2019) and provides a synoptic understanding of various ocean processes such as primary production (Huang et al., 2021), monitoring of phytoplankton (Bracher et al., 2017), harmful algal blooms (Ghatkar et al., 2019), changes in ocean productivity (Westberry et al., 2023), and coastal water quality (Zheng and DiGiacomo, 2017). Sustained observations can also be beneficial for studies on global carbon cycle, marine biodiversity and function (Canonico et al., 2019), biogeochemical models, data assimilation, assessing impact and adaptation of marine ecosystems to climate change (Dutkiewicz et al., 2019), understanding Earth System bio-feedback mechanisms, flow of material through marine food webs (Racault et al., 2015), implications for marine resources, marine coastal hazards (Melet et al., 2020) and marine pollution (Seo et al., 2020). These benefits are critical for understanding the health of marine ecosystems, protecting human health and the environment, managing fisheries, and promoting sustainable ocean policies (Groom et al., 2019).
The conventional remote sensing of ocean color relies on measurements of radiances coming out of the water surface reaching the top-of-atmosphere using passive sensors. These observations have changed our view of the global distribution of the phytoplankton. However, this technology has some fundamental limitations (Hostetler et al., 2018; Jamet et al., 2019). Passive ocean color images are impacted by the contribution of the aerosols to the top-of-atmosphere signal which is removed through atmospheric correction and this leads to uncertainties in the ocean color products (IOCCG, 2010; Goyens et al., 2013). Unaccounted contributions from bubbles, foam, and surface reflection can further impact accuracy. Retrieval attempts may fail under challenging conditions such as Sun glint, aerosols, and clouds (Ilori et al., 2019). The ocean color signal is mainly confined to the surface, causing significant errors in water-column-integrated ocean properties such as chlorophyll concentration and net primary production. The retrieved water leaving radiance signal in ocean color remote sensing is influenced by factors such as colored dissolved matter (Tavora et al., 2020), phytoplankton pigments, non-algal particles, and backscattering by suspended particles, leading to uncertainty in retrieving fundamental properties and geophysical parameters requiring additional information to improve accuracy (Werdell et al., 2018). Ocean color global sampling is significantly limited by atmospheric interferences, Sun angle, and cloud cover. On average, more than 70% of the Earth’s ocean area is under sufficient cloud cover making passive ocean color retrievals impossible, and side-scatter from nearby clouds can compromise ocean retrievals from otherwise clear sky pixels (Hostetler et al., 2018). Strongly absorbing aerosol layers can also compromise ocean color monitoring for extended periods. Polar regions have low Sun angles and cloud conditions, which can eliminate ocean color sampling for a significant fraction of the year (Behrenfeld et al., 2017). This can undermine the understanding of plankton annual cycles and biogeochemistry. The conventional ocean color technology provides no information about the water quality parameters at night (Hostetler et al., 2018; Jamet et al., 2019; Behrenfeld et al., 2022).
Space-borne ocean color observations using lidar have a great potential to provide complementary information to existing passive ocean color sensors (Churnside, 2014; Hostetler et al., 2018; Jamet et al., 2019). Lidar is an active remote sensing technology and has been extensively used for atmospheric applications. However, it did not get a lot of attention from the ocean color community. Lidar technique can overcome some limitations of the passive ocean color observations: it can provide an enhanced temporal coverage including nighttime observations and increased spatial range including polar regions which offers a great potential expansion of the available datasets and possibility of furthering our knowledge of the polar oceans and its processes. In addition, the lidar enables to obtain bio-optical and biogeochemical parameters over the vertical in the first tens of meters, contrary to passive observations.
The potential of airborne lidar has been demonstrated for accurately estimating scattering layers and phytoplankton biomass (Chen et al., 2021; Churnside, 2014; Churnside et al., 2021; Yuan et al., 2022) or for fishery surveys (Roddewig et al., 2018). Recent developments of shipborne lidar confirmed the potential of lidar to monitor the scattering and phytoplankton layers over the first tens of meters (Collister et al., 2018; Zimmerman et al., 2020; Shen et al., 2022; Zhang et al., 2022). Although airborne and shipborne lidar sensors were shown to have great potential for measuring the optical properties of the water column for various applications (Steinvall and Björck, 2020; Zhou et al., 2022), there are currently no space-borne lidars designed specifically for this purpose. Nevertheless, there have been studies that have utilized data from the CALIOP and ATLAS sensors aboard CALIPSO and ICESat-2 satellite respectively, which were not initially intended for ocean applications (Behrenfeld et al., 2013; Behrenfeld et al., 2017; Lu et al., 2014; Lu et al., 2016; Hostetler et al., 2018; Lu et al., 2022). The Cloud-Aerosol lidar and Infrared Pathfinder Satellite Observations mission, abbreviated as CALIPSO, was jointly developed by NASA and CNES aiming to fill gaps in observation of aerosols and clouds globally. The measurements were collected with the intention of accurately getting information on the atmospheric extinction coefficient profiles, cloud height data, identifying non-spherical aerosol particles and their sizes, and discriminating water clouds from ice clouds (Winker et al., 2006). The main sensor is the Cloud-Aerosol-lidar Orthogonal Polarization (CALIOP) which is near-nadir viewing lidar sensor with two wavelength polarizations at 532 nm and 1,064 nm.
CALIOP data were used, for the first time, for ocean applications in 2007 and this work has provided the first global image of subsurface ocean with a lidar satellite (Hu et al., 2007; Behrenfeld et al., 2013) pioneered assessments of global ocean phytoplankton biomass (Cphyto) and particulate organic carbon (POC) using CALIOP particulate back-scattering coefficient, bbp(532) estimates. Several following studies improved the data processing (Lu et al., 2014; Lu et al., 2016; Lu et al., 2021a; Lu et al., 2021b; Behrenfeld et al., 2022) and used the CALIOP bbp product for studying the polar regions (Behrenfeld et al., 2017), the diel vertical migration (Behrenfeld et al., 2019) or the seasonal distributions of bbp in the Mediterranean Sea (Dionisi et al., 2020). CALIOP bbp was validated using ocean color satellite products and in-situ measurements and the results showed accurate estimates of the particulate back-scattering coefficient, bbp(532) (Behrenfeld et al., 2013; 2017; Lacour et al., 2020; Lu et al., 2021a; Bisson et al., 2021). However, these validation exercises were based on different schemes in all of those studies (different time and distance differences between the in-situ measurements and the CALIOP footprint). There exists no standard validation protocol for space-borne lidar oceanic products, such as those used for validation of passive ocean color satellite products (Bailey and Werdell, 2006). The major difficulties for validating space-borne lidar oceanic products are the lidar footprint size (70 m for CALIOP) and the revisit time (16 days) which poses a significant challenge for having a significant number of match-ups.
Alternative validation protocols need to be developed specifically for space-borne lidar sensors to ensure the accuracy and reliability of their data products. The much smaller footprint of lidar sensors in comparison to ocean color sensors swaths of 1,000+ km makes it challenging to collocate in situ data. Moreover, the repeat cycle of lidar sensors, as CALIOP, is much less frequent than ocean color sensors, making it difficult to select an appropriate time and space window for validation of lidar data due to scarcity of coincident in situ data. Recent studies have adopted various spatio-temporal scales for validating CALIOP data with in-situ BGC-Argo bbp (Lacour et al., 2020; Bisson et al., 2021). However, there is a lack of research aimed at providing clear guidelines to the scientific community regarding the optimal spatio-temporal scales for validating satellite lidar sensors.
In this study, the objective is to establish a standardized methodology with a clear and objective criterion for validating CALIOP oceanic products, bbp(532), but also other current and future space-borne lidar oceanic products, given the limited availability of datasets that makes direct validation challenging. The proposed methodology uses an objective statistical score to determine the optimal time-distance window. The CALIOP bbp(532) archive from 2010 to 2017 and globally was compared to the BGC-Argo bbp measurements through a match-up exercise, where the CALIOP footprint was co-located with the BGC-Argo measurement with criteria on the time (Δt) and distance (Δd) differences between both observations. We investigated the impact of twenty combinations of (Δt, Δd) on the accuracy of the CALIOP bbp. Sensitivities analysis was performed to showcase the advantages and limitations of our proposed validation protocol.
CALIOP measures the back-scatter lidar signal at 532 nm and 1,064 nm wavelengths and is a nadir-pointing lidar (Winker et al., 2010). The measurements in CALIOP comprises of the co-polarized and cross-polarized components of the vertically integrated back-scatter with a vertical resolution of 22.5 m in ocean waters (Behrenfeld et al., 2013; Bisson et al., 2021). The day- and nighttime lidar derived bbp products published by Behrenfeld et al., 2019 as available online (http://orca.science.oregonstate.edu/lidar_public_v2.php) were used in this study. Similar to Bisson et al., 2021, modification to this dataset was performed by using a conversion factor (β(π)/bbp) of 0.32 instead of 0.16. That is, the dataset was multiplied by a factor of 0.5. The CALIOP data used in this study are for the period 2010 to 2017 and includes both day- and night-time data.
The BGC-Argo bbp profiles at 700 nm were downloaded from the biogeochemical-argo.com website on 25-12-2021 (Claustre et al., 2020). Synthetic delay mode data were used which are quality controlled and depth adjusted data. There were about 41,420 data points with bbp data for the period between 2010 and 2017 at separate locations. Outliers were removed using 1.5 times the interquartile range method. For a comparable dataset between the CALIOP and Argo, the data had to be averaged within the mixed layer depth. This was achieved by finding the depth where the density is more than 0.03 kg·m−3 with respect to the density at the depth 10 m. The global median mixed layer depth from the BGC-Argo dataset is 18 m and has an inter-quartile range of 3.9 m (Bisson et al., 2021). However, only depths less than 50 m were considered, and the global median value was used at stations where this method of finding mixed layer depth (MLD) did not work due to a smaller number of samples in the profile. The values of the calculated BGC-Argo values were reported to be not significantly changed if the first light attenuation layer was chosen instead of mixed layer depth as in Bisson et al., 2021. Figure 1 shows the locations of the BGC-Argo data points having a match-up with the CALIOP dataset, within 16-day and 50-km spatio-temporal range.
FIGURE 1. Locations where a common matchup between the CALIOP and BGC-Argo were observed globally for the period 2008–2017.
As the wavelength at which bbp is provided (at 700 nm for BGC-Argo and at 532 nm for CALIOP), it is necessary to transform BGC-Argo bbp(700) into bbp(532) for direct comparison. This was done with the following equation:
Where Y represents the spectral slope of the backscattering spectra.
MODIS-Aqua level-3 remote sensing reflectance (Rrs) data at 443 nm and 555 nm with the 8-day average and 9-km resolution were downloaded from the NASA ocean color website. The spectral slope of the backscattering coefficient (Eq. 1) was determined through the computation from the Rrs at 443 nm and 555 nm, using the following formula (Lee et al., 2002):
This was required for converting the bbp(700) from the BGC-Argo data set to bbp(532) for comparison with the CALIOP-estimated bbp(532). Similarly, the annual MODIS Aqua derived SST values were also obtained from the NASA website. The Kd values from MODIS Aqua with 8-day average and 9 km resolution were also downloaded to be used in the depth averaging of BGC-Argo bbp as in Lacour et al., 2020.
We first present two published validation protocols for which a high number of matchups were used and then we present the scheme we developed to propose a more general and universal validation protocol of space-borne oceanic lidar products.
Bisson et al., 2021 used a decorrelation approach to choose the time-distance windows for the matchups between global BGC-Argo and CALIOP datasets for the period 2015–2017. Only day-time dataset was considered for the analysis. bbp(700) from the BGC-Argo dataset was converted to bbp(532) to match with the wavelength of CALIOP using a spectral slope calculated from inversion of MODIS-Aqua Rrs using the generalized IOP model (Werdell et al., 2013). A 3-point moving average median was performed on the BGC-Argo profiles of bbp and removal of outliers were performed (more than 1.5 inter-quartile range). The CALIOP dataset was used as processed by Behrenfeld et al., 2019 except for using a conversion factor of 0.32 instead of 0.16 for the ratio of β(π) to bbp. The depth-averaged bbp was calculated within the mixed layer depth, being the point where the density was greater than 0.03 kg·m−3 with respect to the density at 10 m depth. This is performed to match the oceanic vertical resolution of CALIOP.
To define the validation protocol, a 50-km window and 24-hour window between the BGC-Argo measurements and the CALIOP observations were used when the annual average Sea Surface Temperature (SST) of the sampling point was greater than 15°C. For stations with annual average SST less than 15°C, a 15-km and a 24-hour window were used.
Lacour et al., 2020, validated CALIOP observed bbp data with BGC-Argo data in the North Atlantic for the year 2014. They defined three configurations of spatio-temporal windows: 9-km/16 days, 1°/16 days, and 2°/1 month. The analysis included both day-and night-time data. BGC-Argo data were denoised through the removal of data points along the profile designated as “bad” or “probably bad.”
A fixed backscattering spectral slope of 0.78 was used to convert BGC-Argo bbp(700) to bbp(532). The vertical integration of the bbp profiles from the BGC-Argo to match the CALIOP data was performed through the application of the following equation.
where Kd(532) (m−1) is the diffuse attenuation coefficient of downwelling irradiance at the wavelength 532 nm. The authors calculated the diffuse attenuation coefficient by fitting a fourth-degree polynomial function of the logarithm of the downwelling irradiance (Ed(490)) measured by the floats, and then determined the mean slope over the initial 50 m of each profile. Only profiles of type 1 and type 2 (good and probably good) were considered, and any data points in the profiles flagged as “bad” or “probably bad” were excluded from analysis. For 30% of the dataset, the quality of the data was insufficient to calculate Kd(490). The mean Kd(490) was determined by averaging profiles within a 100-km radius and 20-day time period, which corresponds to the decorrelation scale of bio-optical properties, provided that the change in bbp float was less than 50%. The Kd(490) was then converted to Kd(532) using the specified equation.
The aim of the present study was to assess the best validation protocol by identifying potential impact of different combinations of time, distance, period-of-day, SST thresholds, and depth integration methods. This was achieved by comparing match-up scales defined in the studies conducted by Bisson et al., 2021; Lacour et al., 2020, and evaluating various other combinations of these factors. Table 1 summarizes the difference between this study and that of Bisson et al., 2021; Lacour et al., 2020. We have used a statistical score (to be discussed in the following section) to evaluate the different combinations of time and distance to arrive at an optimal protocol of time-distance window for the validation of space-borne lidar oceanic products with sea-truth data.
In order to determine the optimal match-up time/distance window, various combinations of time (ranging from 3 h to 16 days) and distance windows (ranging from 9 km to 50 km) were evaluated. Each of these combinations was further subdivided into day-, nighttime, and all values, and plotted separately. In addition, the combinations were also subdivided based on SST, including all SST values, SST less than 15°C, and SST greater than 15°C. The effects of different depth-averaging methods (such as in Bisson et al., 2021), in Lacour et al., 2020, with BGC-Argo Ed as input, or in Lacour et al., 2020, with MODIS Kd as input were also assessed. Statistical analyses were performed on each combination. The various distance and time windows used in this study are illustrated in Figure 2.
To evaluate each match-up combination, six statistical parameters were calculated along with scatterplots. These parameters include the slope (α) and intercept (β) of the regression line, the bias (determined by Eq. 5), the relative error (RE, determined by Eq. 6), the root mean squared error (RMSE, determined by Eq. 7), and the determination coefficient (R2), where N represents the number of match-ups.
To rank each of the match-up combinations according to its performance in relation to other match-up combinations, a scoring scheme was adopted from Müller et al., 2015; Mograne et al., 2019. This scheme evaluated the α, β, bias, RE, RMSE, and R2 of each algorithm and their range of variation between the minimum and maximum values of each statistical parameter score, considering all the combinations.
where α, β, Bias, RE, RMSE, and R2 represent the arrays with the slope, intercept, Bias, RE, RMSE and R2 for each combination of the matchups. For instance, the algorithm with the closest slope to 1 obtained the highest score of 1 for a given combination. The total score was the sum of the scores for slope (Sα, intercept (Sβ), bias (SBias, Eq. 5), RE (SRE, Eq. 6), RMSE (SRMSE, Eq. 7), and R2 (SR2). Since 6 statistical parameters were considered for the calculation of the score, the maximum value of the scores is 6. N was independently assessed, without evaluating as a part of the score because the value of N increases with the increase of the time and distance differences which led to biased estimation of the score.
As mentioned in Section 3.1, a sensitivity analysis was conducted to determine the most suitable time-distance window for the validation of oceanic products from space-borne lidars. Figure 3 presents the value of the total score depending of a given combination of time and distance windows (20 combinations in total). The analysis included time windows ranging from 3 h to 16 days, and distance windows ranging from 9 to 50 km. The SST threshold conditions described in Bisson et al. (2021) were also considered. The data were further analyzed separately for day-, nighttime, and both for each time-distance window. The comparison of each of the combinations considered in the current study with the previous studies is outlined in Table 1. In order to further evaluate the effectiveness of the scoring scheme and the availability of data for validation, the number of matchups (the number of BGC-Argo floats for each combination is provided in the Supplementary Material) for each combination of time and distance windows is provided in Figure 4. In our study, we consider all match-ups in a given time-distance window.
FIGURE 3. Statistical score for the different combinations of time and distance and SST and day-night conditions (with fixed slope).
Our objective was to identify a time-distance window that is robust and not dependent on multiple conditions. Therefore, we narrowed our analysis to only consider the case with all-SST, all-time data and BGC-Argo bbp(532) estimated using a fixed slope (Eq. 1). From Figure 3, we can observe that the value of the score decreases with the increase of the time and distance windows. The distance seems to have more impact on the value of the score than the time. For instance, if the distance window is fixed to 9-km, the value of the score decreases from 4.655 to 4.064 if the time window increases from ± 3 h to ± 24 h. If the time window is fixed to 6 h, the score decreases from 4.641 to 1.167 if the distance window increases from 9 km to 50 km. For the validation of the standard ocean color products, the time and distance windows are usually ± 3 h and ± 9 km, respectively. Based on this criterion, the score is 4.655 which is the highest score among all combinations. However, it reaches only 1947 matchups (for 53 floats). So, it is not realistic to use the standard validation protocol for space-borne oceanic products. Lacour et al. (2020) used a 9-km distance window and 16-day time window and reached only 16 matchups over more 1,000 profiles. Moreover, using these criteria, the value of the score decreases to 2.305, similar to using a 50-km window (for the same time window). Using a 16-day time window seems unrealistic to use as the time scale of ocean color processes is over few days in the open ocean and it really decreases by almost two-fold the values of the score.
From all combinations, only the ones with a score higher than 3.5 were considered. For this criterion, seven combinations (out of twenty) are available: 9-km/3-h; 9-km/6-h; 9-km/12-h; 9-km/24-h; 15-km/3-h; 15-km/24-h; 25-km/3-h. For those combinations, the number of matchups varies between 1947 (9-km/3-h) and 15,272 (9-km/24-h), corresponding to 53 and 306 floats, respectively. As the number of match-ups is the lowest for the 9-km/3-h combination, it does not seem realistic to use it. As a reminder, we used 8 years of CALIOP data and 41,420 profiles of BGC-Argo. As the sensitivity of the value of the score is not high for the time window, we propose to use 24-h. To enhance the number of match-ups, the distance window needs to be increased, compared to the usual 9-km taken for the validation of the standard ocean color products. So, we propose to use either 9-km or 15-km. Using 15-km increases the number of match-ups, from 5,554 to 15,272 (corresponding to 173 and 306 floats, respectively). For these combinations, the value of the score is 4.064 and 3.589, respectively. It is worth highlighting that our proposed time-distance window resulted in higher scores compared to the 50-km/24-h case in the SST>15°C scenario, which was employed in Bisson et al. (2021). For the case “50-km/24-h and SST>15°C,” the score is 2.772, compared to 4.064 (and 3.589) for the current proposed combination of our study. As mentioned, the score is very sensitive to the distance between the BGC-Argo floats and the CALIOP footprint. It means that it is better to reduce as much as possible this distance. Additionally, the value of the score for the 15-km/24-h combination in the SST<15°C case (Bisson et al., 2021) yielded comparable scores, with a value of 4.208 to compare with values of 4.064 and 3.589 for the 9-km/24-h and 15-km/24-h cases, respectively. This highlights that it is not necessary to add a threshold on the SST to get valuable matchups.
Concerning the protocols proposed by Lacour et al. (2020), we only included their first case: 9-km/16-days. Distances greater than 50 km were not included as the size of the ocean color patterns are meso-scales. For this case, the score reached 2.305, below the scores reached by the validation protocol proposed here. The value of the score decreased by, almost, a factor two, from 4.064 to 2.305 between a time window of 24-h and a time window of 16 days (384-h) showing that taking a time window higher than 24-h leads to unprecise comparisons between BGC-Argo floats and CALIOP product. For a window of 16 days (384-h), the distance does not impact the value of the score, varying from 2.261 (15-km) to 2.349 (25-km).
As we propose two combinations, we investigated the impact of the quality and accuracy of the CALIOP product. Figures 5, 6 present scatterplots between the BGC-Argo and CALIOP bbp(532) for the proposed time-distance windows of 9-km/24-h and 15-km/24-h, respectively. Table 2 shows the statistical parameters for both combinations.
TABLE 2. Statistical parameters on the estimates of CALIOP bbp(532) for the two best optimal combinations of time and distance windows: 9-km/24-h and 15-km/24-h.
For both combinations, most of the points are very close to the 1:1 line which means that the CALIOP bbp(532) estimates are very close to BGC-Argo bbp(532). 99 CALIOP bbp are highly underestimated by a factor of higher than 4. It means that for those cases, either the CALIOP footprint did not occur the same day as the BGC-Argo observations or during the same day early morning and late afternoon. For this latter case, the CALIOP observations happened during the night while the BGC-Argo during the day. We know that the bio-optical properties vary during the night and day (Kheireddine and Antoine, 2014). So, because of the diurnal variations of the bio-optical properties, the values of bbp can change overnight. This translates in term of statistical parameters. The increase of the distance (from 9-km to 15-km) increases the number of match-up by three-fold (from 5,554 to 15,272). This number is important as it is very difficult to get a significant number of match-ups when comparing in-situ measurements and lidar observations. Aside this difference, the statistical parameters are very similar between both combinations. For instance, the MRE and bias are very similar (RE = 36.12% for 9-km/24-h and 35.81% for 15-km/24-h; Bias = 12.84% for 9-km/24-h and 11.84% for 15-km/24-h). This confirms that the two combinations we proposed are very similar and the slight increase of the distance window does not impact the quality of the match-ups. Even if it is always preferable to choose the shortest distance between the in-situ measurements and the satellite observations, it is not always possible to do that, especially with space-borne lidars. Our proposed validation protocol provides some flexibilities without hampering the quality of the comparisons.
These statistical values are slightly higher than the ones found in Bisson et al. (2021), who reached median percentage error below 25%. This might be explained by the difference of match-ups between both studies: 15,272 for our study and 261 for the study of Bisson et al. (2021). However, the purpose of our study was not to validate per se the CALIOP bbp product but to propose an objective validation protocol.
We proposed a universal protocol validation for comparison between in-situ measurements and space-borne lidar oceanic products. To do that, we used the BGC-Argo observations as it provides a global distribution of bbp(532) since 2010. It is a valuable dataset for validation of ocean color satellite products (Bisson et al., 2019). However, the comparison between the BGC-Argo and CALIOP bbp is not straightforward for several reasons: measurement of bbp(700) vs. CALIOP bbp(532); profiles of bbp(700) vs. depth-integrated CALIOP bbp(532). We investigated the sensitivity of our analysis to these factors.
In order to compare bbp values from BGC-Argo products with those from CALIOP, BGC-Argo bbp(700) value had to be converted to bbp(532) since CALIOP estimates bbp at this wavelength. The spectral slope of the bbp value was calculated to achieve this, which could either be a variable value derived from MODIS-Aqua data, as in Bisson et al. (2021), or a fixed value of 0.78, as in Lacour et al. (2020). The choice of spectral slope can impact the validation of the CALIOP-derived bbp. Therefore, the influence of different spectral slopes on the validation results was investigated. However, our primary goal was to determine the optimal time-distance window for validating CALIOP-derived bbp data, so proposing a specific spectral slope choice was not within the scope of our work.
For our statistical analysis, we fixed the slope value at 0.78 to convert bbp(700) to bbp(532) (Eq. 1) as in Lacour et al. (2020). Figure 7 shows the scatterplot of bbp(532) estimated using a fixed slope vs. using a variable slope, considering the depth-averaged method as in Bisson et al. (2021). The variable slope was calculated as explained in Section 2.3. The selection of a particular spectral slope directly affects the conversion of bbp values at 700 nm to those at 532 nm, which is necessary for a valid comparison with the CALIOP measurements. In Figure 7, we can observe that most of the values are close to the 1:1 line. However, a bias can be observed leading to a relative error of 40%.
FIGURE 7. Comparison of Bisson et al. (2021) bbp(532) with a fixed vs. variable slope.
To enable a comparable depth resolution with CALIOP-derived bbp data, the bbp float value had to be depth-integrated. Bisson et al. (2021), and Lacour et al. (2020), used different methods to depth-integrated BGC-Argo bbp. In the results presented in Section 4, we used the method from Bisson et al. (2021). So, our results could be directly comparable with their results. However, we also used another method (Lacour et al., 2020) as shown in Eq. 2. In Lacour et al. (2020), the diffuse attenuation coefficient, Kd(532) is used to depth-integrate bbp(532). Kd can either be directly calculated by the downwelling irradiance, Ed, of BGC-Argo or by using satellite products when the downwelling irradiance is not available in BGC-Argo. The way to estimate the depth-integrated bbp impact the value of bbp and so the comparison with CALIOP. So, it is necessary to estimate the differences between the methods used to obtain the depth-integrated value.
Figures 8, 9 show the comparison between the depth-integration methods using BGC-Argo Ed or MODIS-Aqua Kd as in Lacour et al. (2020), compared to the method in Bisson et al. (2021), respectively. We can observe that the depth-integration methods have little impact on the estimation of bbp values as the values from the three methods are very similar. The relative error is of 11.81% and 3.63% between the methods using Ed or Kd in Lacour et al. (2020) and in Bisson et al. (2021), respectively. But these differences are negligeable compared to the impact of the use of a fixed slope to transform bbp(700) to bbp(532).
FIGURE 8. Impact of the depth-averaged integration: Bisson et al. (2021) bbp vs. Lacour et al. (2020) bbp estimated using BGC-Argo Ed.
FIGURE 9. Impact of the depth-averaged integration: Bisson et al. (2021) bbp vs. Lacour et al. (2020) bbp estimated using MODIS Kd.
Although the depth-integration method used can impact the calculated BGC-Argo float bbp values, our study focused solely on the time-distance window for the validation of CALIOP satellite-based lidar measurements. As such, we did not investigate the impact of different depth-integration methods in the validation to find the best method for depth integration.
Bisson et al. (2021) has suggested to use the annual global average SST as a threshold for choosing the distance window between the BGC-Argo floats and the CALIOP footprint. Our analysis proposes that this SST threshold be not be used for several reasons. First, it imposes an additional condition on the match-ups protocol, requiring an extra parameter to validate CALIOP datasets, which can impede ease of operation. Second, our results indicate that our proposed time-distance window generated superior scores in comparison to the 50-km/24-h case employed in Bisson et al. (2021), for the SST>15°C scenario. Third, even though the 15-km/24-h combination in the SST<15°C case produced comparable scores, our analysis showed that our proposed time-distance window outperformed the proposed protocol by Bisson et al. (2021), for SST<15°C. We generalized this combination for any SST value. This makes our proposed validation protocol more straightforward and easier to apply.
We focused our analysis on the combined day- and nighttime data. However, we showed in Figure 3 the values of the score for day- and nighttime only data, respectively. For the daytime configuration, the results are very similar to the day- and nighttime configuration. Both proposed combinations (9-km/24-h and 15-km/24-h) provided the best compromised in term of values of the score and the number of matchups. For instance, for the combination 9-km/24-h, the score is 4.275 for the daytime only and 4.064 for all data with the number of match-ups being 1.003*104 and 1.527*104, respectively. The distribution of the score is similar between all data and only daytime configurations. The score decreases more with the increase of the distance and the lowest values are found when the time window is 16 days (386-h). The 50-km window and time window >3-h show also very low values of the score for both cases. However, the values are lower for these configurations during daytime. For instance, for 50-km/24-h, the score is 2.003 for daytime compared to 2.405 for all data. The results are very different for the nighttime. Even if our proposed validation protocol is still valid (with values of score of 3.961 for 9-km/24-h and 4.228 for 15-km/24-h), the distribution of the score is very different with the scenario with all data. The increase of the time and distance leads to an increase of the score. However, our proposed validation protocol is valid for day- and nighttime conditions.
The study aimed at defining the optimal time-distance window for the validation protocol of space-borne lidar oceanic products. We focused our study on the particulate back-scattering coefficient, bbp, estimated from the CALIOP space-borne lidar and we compared these estimates to in-situ measurements obtained from the global BGC-Argo floats for the period 2010–2017. Our work enhances the works published by Bisson et al. (2021), and Lacour et al. (2020), by including longer period (2010–2017) and nighttime observations.
The study analyzed twenty combinations of time and distance windows between the in-situ measurements and CALIOP footprint and their impacts of the validation through the use of a statistical score, which is the combination of six statistical parameters. The values of the score were analyzed following the increase of the distance and time windows. The results showed that the optimal time-distance window for the validation protocol of CALIOP is 24 h and 9 km. However, the study also found that the distance window could be relaxed to 15 km without significantly affecting the validation results. We analyzed the assumptions made in previous published validation exercises: use of SST as a threshold for the choice of the time and distance windows; the estimates of the depth-integrated BGC-Argo bbp; the estimates of BGC-Argo bbp(532) from BGC-Argo bp(700). We showed that the SST is not necessary to use for the validation, contrary to what proposed Bisson et al. (2021); the slope of bbp has a greater impact on the calculation of the in-situ bbp than the depth-integration methods. However, this does not hamper our conclusions and our proposed validation protocol.
The findings of this study are significant as they provide guidance for the validation protocol of space-borne lidar oceanic products, which is crucial for ensuring the accuracy of satellite lidar measurements. The proposed protocol should help to develop more validation exercise of CALIOP or ATLAS oceanic products.
Publicly available datasets were analyzed in this study. This data can be found here: http://orca.science.oregonstate.edu/lidar_public_v2.php, https://dataselection.euro-argo.eu/, and https://oceancolor.gsfc.nasa.gov.
CJ was lead (or Principal Investigator) for this work, designed the study and analyzed the results. SV-C led the data processing. CJ and SV-C contributed to the writing of the manuscript. All authors contributed to the article and approved the submitted version.
This work and SV-C position are funded by CNES through the TOSCA program. This research was supported by the International Space Science Institute (ISSI) in Bern and Beijing, through ISSI/ISSI-BJ International Team project #530 (Toward A 3-D Observation of the Ocean Color: Benefit of Lidar Technique).
The authors would like to thank Kelsey Bisson for fruitful discussion and for sharing her match-ups dataset and Leo Lacour for sharing his codes and fruitful discussions. BGC-Argo data were collected and made freely available by the International Argo Program and the national programs that contribute to it. We would like to thank the Ocean Productivity Group at the Oregon State University for providing the CALIOP dataset.
The authors declare that the research was conducted in the absence of any commercial or financial relationships that could be construed as a potential conflict of interest.
All claims expressed in this article are solely those of the authors and do not necessarily represent those of their affiliated organizations, or those of the publisher, the editors and the reviewers. Any product that may be evaluated in this article, or claim that may be made by its manufacturer, is not guaranteed or endorsed by the publisher.
The Supplementary Material for this article can be found online at: https://www.frontiersin.org/articles/10.3389/frsen.2023.1194580/full#supplementary-material
Bailey, S. W., and Werdell, P. J. (2006). A multi-sensor approach for the on-orbit validation of ocean color satellite data products. Remote Sens. Environ. 102, 12–23. doi:10.1016/J.RSE.2006.01.015
Behrenfeld, M. J., Hu, Y., Hostetler, C. A., Dall’Olmo, G., Rodier, S. D., Hair, J. W., et al. (2013). Space-based lidar measurements of global ocean carbon stocks. Geophys Res. Lett. 40, 4355–4360. doi:10.1002/GRL.50816
Behrenfeld, M. J., Hu, Y., O’Malley, R. T., Boss, E. S., Hostetler, C. A., Siegel, D. A., et al. (2017). Annual boom–bust cycles of polar phytoplankton biomass revealed by space-based lidar. Nat. Geosci. 10, 118–122. doi:10.1038/ngeo2861
Behrenfeld, M. J., Gaube, P., Della Penna, A., O’Malley, R. T., Burt, W. J., Hu, Y., et al. (2019). Global satellite-observed daily vertical migrations of ocean animals. Nature 576, 257–261. doi:10.1038/s41586-019-1796-9
Behrenfeld, M. J., Hu, Y., Bisson, K. M., Lu, X., and Westberry, T. K. (2022). Retrieval of ocean optical and plankton properties with the satellite Cloud-Aerosol Lidar with Orthogonal Polarization (CALIOP) sensor: Background, data processing, and validation status. Remote Sens. Environ. 281, 113235. doi:10.1016/J.RSE.2022.113235
Bisson, K. M., Boss, E., Westberry, T. K., and Behrenfeld, M. J. (2019). Evaluating satellite estimates of particulate backscatter in the global open ocean using autonomous profiling floats. Opt. Express 27, 30191. doi:10.1364/OE.27.030191
Bisson, K. M., Boss, E., Werdell, P. J., Ibrahim, A., and Behrenfeld, M. J. (2021). Particulate backscattering in the global ocean: A comparison of independent assessments. Geophys Res. Lett. 48, e2020GL090909. doi:10.1029/2020GL090909
Bracher, A., Bouman, H. A., Brewin, R. J. W., Bricaud, A., Brotas, V., Ciotti, A. M., et al. (2017). Obtaining phytoplankton diversity from ocean color: A scientific roadmap for future development. Front. Mar. Sci. 4, 55. doi:10.3389/fmars.2017.00055
Canonico, G., Buttigieg, P. L., Montes, E., Muller-Karger, F. E., Stepien, C., Wright, D., et al. (2019). Global observational needs and resources for marine biodiversity. Front. Mar. Sci. 6. doi:10.3389/fmars.2019.00367
Chen, P., Jamet, C., Zhang, Z., He, Y., Mao, Z., Pan, D., et al. (2021). Vertical distribution of subsurface phytoplankton layer in South China Sea using airborne lidar. Remote Sens. Environ. 263, 112567. doi:10.1016/J.RSE.2021.112567
Churnside, J. H., Marchbanks, R. D., and Marshall, N. (2021). Airborne lidar observations of a spring phytoplankton bloom in the western arctic ocean. Remote Sens. (Basel) 13, 2512. doi:10.3390/rs13132512
Churnside, J. H. (2014). Review of profiling oceanographic lidar. Opt. Eng. 53, 051405. doi:10.1117/1.OE.53.5.051405
Claustre, H., Johnson, K. S., and Takeshita, Y. (2020). Observing the global ocean with biogeochemical-argo. Ann. Rev. Mar. Sci. 12, 23–48. doi:10.1146/annurev-marine-010419-010956
Collister, B. L., Zimmerman, R. C., Sukenik, C. I., Hill, V. J., and Balch, W. M. (2018). Remote sensing of optical characteristics and particle distributions of the upper ocean using shipboard lidar. Remote Sens. Environ. 215, 85–96. doi:10.1016/J.RSE.2018.05.032
Dionisi, D., Brando, V. E., Volpe, G., Colella, S., and Santoleri, R. (2020). Seasonal distributions of ocean particulate optical properties from spaceborne lidar measurements in Mediterranean and Black sea. Remote Sens. Environ. 247, 111889. doi:10.1016/J.RSE.2020.111889
Dutkiewicz, S., Hickman, A. E., Jahn, O., Henson, S., Beaulieu, C., and Monier, E. (2019). Ocean colour signature of climate change. Nat. Commun. 10, 578. doi:10.1038/s41467-019-08457-x
Ghatkar, J. G., Singh, R. K., and Shanmugam, P. (2019). Classification of algal bloom species from remote sensing data using an extreme gradient boosted decision tree model. Int. J. Remote Sens. 40, 9412–9438. doi:10.1080/01431161.2019.1633696
Goyens, C., Jamet, C., and Schroeder, T. (2013). Evaluation of four atmospheric correction algorithms for MODIS-Aqua images over contrasted coastal waters. Remote Sens. Environ. 131, 63–75. doi:10.1016/J.RSE.2012.12.006
Groom, S. B., Sathyendranath, S., Ban, Y., Bernard, S., Brewin, B., Brotas, V., et al. (2019). Satellite ocean colour: Current status and future perspective. Front. Mar. Sci. 6, 1–30. doi:10.3389/fmars.2019.00485
Hostetler, C. A., Behrenfeld, M. J., Hu, Y., Hair, J. W., and Schulien, J. A. (2018). Spaceborne lidar in the study of marine systems. Ann. Rev. Mar. Sci. 10, 121–147. doi:10.1146/annurev-marine-121916-063335
Hu, Y., Behrenfeld, M., McClain, C., O’Malley, R., Weimer, C., Signorini, S., et al. (2007). “Ocean color related studies using CALIPSO data,” in Proceedings of the NASA Ocean Color research Team meeting.
Huang, Y., Nicholson, D., Huang, B., and Cassar, N. (2021). Global estimates of marine gross primary production based on machine learning upscaling of field observations. Glob. Biogeochem. Cycles 35. doi:10.1029/2020GB006718
Ilori, C., Pahlevan, N., and Knudby, A. (2019). Analyzing performances of different atmospheric correction techniques for landsat 8: Application for coastal remote sensing. Remote Sens. (Basel) 11, 469. doi:10.3390/rs11040469
IOCCG (2010). “Atmospheric correction for remotely-sensed ocean-colour products,” in Reports of the international ocean-colour coordinating Group. Editor M. Wang (Dartmouth, Canada: IOCCG).
Jamet, C., Ibrahim, A., Ahmad, Z., Angelini, F., Babin, M., Behrenfeld, M. J., et al. (2019). Going beyond standard Ocean Color observations: Lidar and polarimetry. Front. Mar. Sci. 0, 251. doi:10.3389/FMARS.2019.00251
Kheireddine, M., and Antoine, D. (2014). Diel variability of the beam attenuation and backscattering coefficients in the northwestern Mediterranean Sea (BOUSSOLE site). J. Geophys Res. Oceans 119, 5465–5482. doi:10.1002/2014JC010007
Lacour, L., Babin, M., and Larouche, R. (2020). In situ evaluation of spaceborne CALIOP lidar measurements of the upper-ocean particle backscattering coefficient. Opt. Express 28 (18), 26989–26999. doi:10.1364/OE.397126
Lee, Z. P., Carder, K. L., and Arnone, R. A. (2002). Deriving inherent optical properties from water color: A multiband quasi-analytical algorithm for optically deep waters. Appl. Opt. 41, 5755–5772. doi:10.1364/ao.41.005755
Lu, X., Hu, Y., Trepte, C., Zeng, S., and Churnside, J. H. (2014). Ocean subsurface studies with the CALIPSO spaceborne lidar. J. Geophys Res. Oceans 119, 4305–4317. doi:10.1002/2014JC009970
Lu, X., Hu, Y., Pelon, J., Trepte, C., Liu, K., Rodier, S., et al. (2016). Retrieval of ocean subsurface particulate backscattering coefficient from space-borne CALIOP lidar measurements. Opt. Express 24, 29001. doi:10.1364/OE.24.029001
Lu, X., Hu, Y., Omar, A., Baize, R., Vaughan, M., Rodier, S., et al. (2021a). Global Ocean studies from CALIOP/CALIPSO by removing polarization crosstalk effects. Remote Sens. 13, 2769. doi:10.3390/RS13142769
Lu, X., Hu, Y., Yang, Y., Neumann, T., Omar, A., Baize, R., et al. (2021b). New Ocean subsurface optical properties from space lidars: CALIOP/CALIPSO and ATLAS/ICESat-2. Earth Space Sci. 8, e2021EA001839. doi:10.1029/2021EA001839
Lu, X., Hu, Y., Zeng, X., Stamnes, S. A., Neuman, T. A., Kurtz, N. T., et al. (2022). Deriving snow depth from ICESat-2 lidar multiple scattering measurements: Uncertainty analyses. Front. Remote Sens. 3, 36–42. doi:10.3760/cma.j.cn112148-20211203-01045
McClain, C. R. (2009). A decade of satellite Ocean Color observations. Ann. Rev. Mar. Sci. 1, 19–42. doi:10.1146/annurev.marine.010908.163650
Melet, A., Teatini, P., Le Cozannet, G., Jamet, C., Conversi, A., Benveniste, J., et al. (2020). Earth observations for monitoring marine coastal hazards and their drivers. Surv. Geophys. 41, 1489–1534. doi:10.1007/S10712-020-09594-5
Mograne, M., Jamet, C., Loisel, H., Vantrepotte, V., Mériaux, X., and Cauvin, A. (2019). Evaluation of five atmospheric correction algorithms over French optically-complex waters for the sentinel-3A OLCI Ocean Color sensor. Remote Sens. (Basel) 11, 668. doi:10.3390/rs11060668
Müller, D., Krasemann, H., Brewin, R. J. W., Brockmann, C., Deschamps, P.-Y., Doerffer, R., et al. (2015). The Ocean colour climate change initiative: I. A methodology for assessing atmospheric correction processors based on in-situ measurements. Remote Sens. Environ. 162, 242–256. doi:10.1016/j.rse.2013.11.026
Racault, M.-F., Raitsos, D. E., Berumen, M. L., Brewin, R. J. W., Platt, T., Sathyendranath, S., et al. (2015). Phytoplankton phenology indices in coral reef ecosystems: Application to ocean-color observations in the Red Sea. Remote Sens. Environ. 160, 222–234. doi:10.1016/j.rse.2015.01.019
Roddewig, M. R., Koel, T. M., Shaw, J. A., Churnside, J. H., Bigelow, P. E., Hauer, F. R., et al. (2018). Airborne lidar detection and mapping of invasive lake trout in Yellowstone Lake. Appl. Opt. 57 (15), 4111–4116. doi:10.1364/AO.57.004111
Seo, S., Park, Y.-G., and Kim, K. (2020). Tracking flood debris using satellite-derived ocean color and particle-tracking modeling. Mar. Pollut. Bull. 161, 111828. doi:10.1016/j.marpolbul.2020.111828
Shen, X., Kong, W., Chen, P., Chen, T., Huang, G., and Shu, R. (2022). A shipborne photon-counting lidar for depth-resolved ocean observation. Remote Sens. 14, 3351. doi:10.3390/RS14143351
Steinvall, O., and Björck, M. (2020). Water optical properties in Scandinavian waters and airborne optical sensing. Electro-Optical Remote Sens. XIV 11538, 1153806. doi:10.1117/12.2571124
Tavora, J., Boss, E., Doxaran, D., and Hill, P. (2020). An algorithm to estimate suspended particulate matter concentrations and associated uncertainties from remote sensing reflectance in coastal environments. Remote Sens. (Basel) 12, 2172. doi:10.3390/rs12132172
Werdell, P. J., Franz, B. A., Bailey, S. W., Feldman, G. C., Boss, E., Brando, V. E., et al. (2013). Generalized ocean color inversion model for retrieving marine inherent optical properties. Appl. Opt. 52, 2019–2037. doi:10.1364/ao.52.002019
Werdell, P. J., McKinna, L. I. W., Boss, E., Ackleson, S. G., Craig, S. E., Gregg, W. W., et al. (2018). An overview of approaches and challenges for retrieving marine inherent optical properties from ocean color remote sensing. Prog. Oceanogr. 160, 186–212. doi:10.1016/j.pocean.2018.01.001
Westberry, T. K., Silsbe, G. M., and Behrenfeld, M. J. (2023). Gross and net primary production in the global ocean: An ocean color remote sensing perspective. Earth Sci. Rev. 237, 104322. doi:10.1016/j.earscirev.2023.104322
Winker, D. M., Hostetler, C. A., Vaughan, M. A., and Omar, A. H. (2006). CALIOP algorithm theoretical basis document, part 1: CALIOP instrument, and algorithms overview. Release 2, 29.
Winker, D. M., Pelon, J., Coakley, J. A., Ackerman, S. A., Charlson, R. J., Colarco, P. R., et al. (2010). The CALIPSO mission. Bull. Am. Meteorol. Soc. 91, 1211–1230. doi:10.1175/2010BAMS3009.1
Yuan, D., Yuan, D., Yuan, D., Mao, Z., Mao, Z., Mao, Z., et al. (2022). Remote sensing of seawater optical properties and the subsurface phytoplankton layer in coastal waters using an airborne multiwavelength polarimetric ocean lidar. Opt. Express 30, 29564–29583. doi:10.1364/OE.463146
Zhang, S., Zhang, S., Zhang, S., and Chen, P. (2022). Subsurface phytoplankton vertical structure from lidar observation during SCS summer monsoon onset. Opt. Express 30, 17665–17679. doi:10.1364/OE.453094
Zheng, G., and DiGiacomo, P. M. (2017). Uncertainties and applications of satellite-derived coastal water quality products. Prog. Oceanogr. 159, 45–72. doi:10.1016/j.pocean.2017.08.007
Zhou, Y., Chen, Y., Zhao, H., Jamet, C., Dionisi, D., Chami, M., et al. (2022). Shipborne oceanic high-spectral-resolution lidar for accurate estimation of seawater depth-resolved optical properties. Light Sci. Appl. 11, 261–313. doi:10.1038/s41377-022-00951-0
Keywords: lidar, validation, remote sensing, backscattering, ocean color, ocean optics
Citation: Vadakke-Chanat S and Jamet C (2023) Validation protocol for the evaluation of space-borne lidar particulate back-scattering coefficient bbp. Front. Remote Sens. 4:1194580. doi: 10.3389/frsen.2023.1194580
Received: 27 March 2023; Accepted: 17 July 2023;
Published: 27 July 2023.
Edited by:
Panagiotis Kokkalis, Kuwait University, KuwaitReviewed by:
Vadim Pelevin, Other, FranceCopyright © 2023 Vadakke-Chanat and Jamet. This is an open-access article distributed under the terms of the Creative Commons Attribution License (CC BY). The use, distribution or reproduction in other forums is permitted, provided the original author(s) and the copyright owner(s) are credited and that the original publication in this journal is cited, in accordance with accepted academic practice. No use, distribution or reproduction is permitted which does not comply with these terms.
*Correspondence: Cédric Jamet, Y2VkcmljLmphbWV0QHVuaXYtbGl0dG9yYWwuZnI=
Disclaimer: All claims expressed in this article are solely those of the authors and do not necessarily represent those of their affiliated organizations, or those of the publisher, the editors and the reviewers. Any product that may be evaluated in this article or claim that may be made by its manufacturer is not guaranteed or endorsed by the publisher.
Research integrity at Frontiers
Learn more about the work of our research integrity team to safeguard the quality of each article we publish.