- 1Optical Remote Sensing Laboratory, The City College of New York, New York, NY, United States
- 2Earth and Environmental Sciences, The Graduate Center, New York, NY, United States
- 3Remote Sensing Division, U.S. Naval Research Laboratory, Washington, DC, United States
- 4European Organisation for the Exploitation of Meteorological Satellites (EUMETSAT), Darmstadt, Germany
Uncertainties in remote sensing reflectance
1 Introduction
Ocean Color (OC) satellite sensors provide radiometric information in multiple bands, allowing to determine spectra of absorption and backscattering of water components and through them, concentrations of chlorophyll-a, colored dissolved organic matter (CDOM) and non-algal particles (Mobley, 2022). These quantities are monitored over global and regional scales and are used in multiple applications including fisheries, ecological models (IOCCG, 2021) and for the detection of algal blooms. Thus, OC is indicative of ocean health and biochemistry and for that reason is listed as an essential climate variable (ECV) (IOCCG, 2008). Atmospheric correction is the critical part in the process of derivation of water parameters, since the water leaving radiance is about 10% of the total radiance measured by the sensor at the top of the atmosphere (TOA) in the blue/green spectral range over typical clear waters, with the other 90% coming from scattering and absorption processes in the atmosphere, including from molecules and aerosol particles, and from reflection from the water surface (Gordon and Morel, 1983; IOCCG, 2010; IOCCG, 2019).
Uncertainties in the water leaving radiance and corresponding remote sensing reflectance
The estimation of uncertainties can be carried out by comparison of the parameters determined from satellite imagery after atmospheric correction with in-situ values, which include data from the AERONET-OC Network (Zibordi et al., 2009; Zibordi et al., 2021), from buoys like Marine Optical BuoY (MOBY) (Clark et al., 1997) and from ships (Moore et al., 2015). Specifically, in Moore et al., 2015 uncertainties in
In Zibordi et al., 2022 a comprehensive assessment of OLCI products and related uncertainties was carried out over European water areas through the comparison of satellite and AERONET-OC data and the additional comparison with similar VIIRS products, showing that OLCI typically displays higher uncertainties than VIIRS in coastal waters. A special case of OLCI-B and OLCI-A called “Tandem Phase” was analyzed, with Sentinel-3B and Sentinel-3A flying 30 s apart on the same orbit, demonstrating much smaller uncertainties than with satellites on the same orbit but with a different phase, which is the default operational configuration of the satellites.
In Herrera-Estrella et al., 2021, a first version of the model used in this study was developed to evaluate the spectral composition of
In Gilerson et al., 2022, the model was expanded and elaborated to determine spectral components of
In this work, the same model is now applied to estimate spectral components of the uncertainties in
In Section 2 the model (Gilerson et al., 2022) is briefly discussed, in Section 3 satellite and in situ data are described and results are presented in Section 4. Conclusions are provided in Section 5.
2 Main relationships in the estimation of uncertainties
A brief description of the model from Gilerson et al., 2022, is given here for the convenience of the reader. The main radiometric quantity in the processing of satellite data is the remote sensing reflectance,
where
In the model,
where
Uncertainties from all components included in Eq. 1a, 1b, 1c in the recording of the signal and in the retrieval process need to be taken into account. After normalizing radiances by the downwelling irradiance,
Variances for the quantities at TOA
As one of the main assumptions of the model, all standard deviation components in Eq. 2 except
where
In Eq. 4a
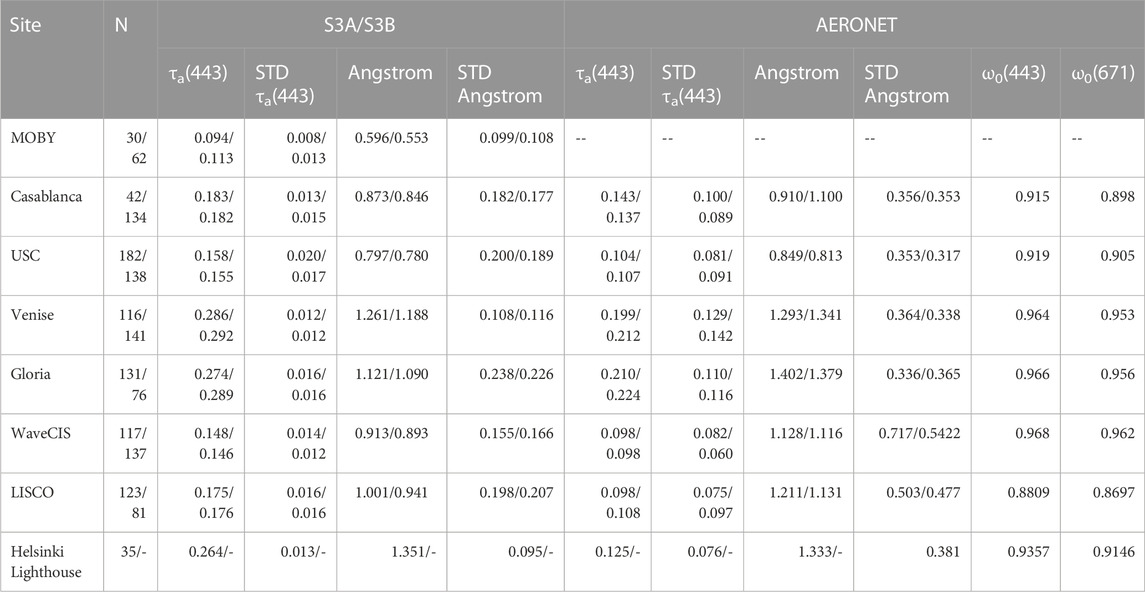
TABLE 2. Average atmospheric parameters at the sites of study determined from satellite and AERONET retrievals.
Equation 3 includes OLCI S3A and 3B system vicarious calibration uncertainties
For each available matchup between satellite and AERONET-OC measurements, all radiance spectra in Eqs 4a, 4b, 4c, 4d were calculated, then spectra were averaged over the total number of available measurements. Mean spectra were then used in the fitting procedure based on Eq. 3, and on mean
with
Biases were also calculated as
Each of radiances in Eq. 4, has individual spectral variable(s) not repeated in other equations, so all spectra of uncertainties, which were considered proportional to these radiances, should be independent of each other. An optimization procedure was carried out in the same manner as in Gilerson et al., 2022 in MATLAB using the default trust-region-reflective algorithm (Coleman and Li, 1994; Coleman and Li, 1996) to determine the respective values of the k coefficients for MOBY and each AERONET-OC site based on the spectra of the
Equation 3 can be alternatively presented as
where normalized radiance spectra
3 Satellite and AERONET-OC data
3.1 OLCI data
OLCI S3A and S3B Collection 3 level 2 Full Resolution imagery (300 m spatial resolution by pixel, EUMETSAT, 2021) was downloaded from the EUMETSAT website for the period of January 2017 to July 2022 for S3A and from May 2018 to July 2022 for S3B for the areas of MOBY in Lanai, Hawaii and seven Aerosol Robotic Network for Ocean Color (AERONET-OC) sites: Casablanca, University of South California (USC), Venise, Gloria, WaveCIS, the Long Island Sound Coastal Observatory (LISCO) and the Helsinki Lighthouse (HLT) with locations shown in Figure 1.
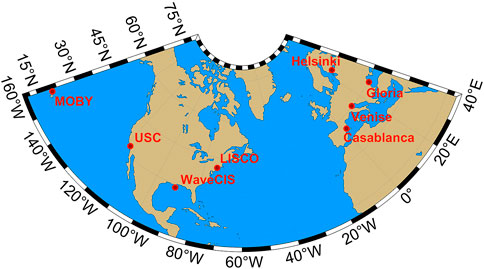
FIGURE 1. Areas of study: Global map showing MOBY area and all AERONET-OC stations used in this study.
Collection 3 was obtained using the extraction data base (EDB) workflow scripts (https://github.com/juanchossn/ThoMaS), and Level 2 SeaBASS - Ocean Colour Database (OCDB) format images were retrieved with a window size of 25 × 25 pixels centered on the previously named sites. Each level 2 file includes geophysical products of the atmosphere and ocean, such as aerosol optical thickness and Angstrom exponent at 865 nm, water-leaving reflectance at 412.5, 443.5, 490, 560, and 665 nm among others, sensor zenith angle, solar zenith angle, and quality flags.
OLCI Collection 3 level 2 operational water reflectance products are not corrected for the bidirectional reflectance distribution function (BRDF). The BRDF correction is not applied for historical reasons as the main users have typically been interested in coastal and inland waters where the standard open-ocean BRDF approach does not apply. Nevertheless, the BRDF correction was applied externally in this study to OLCI L2 products as it is necessary for the current analyses (Morel et al., 2002).
Pixels flagged by at least one of the following conditions were excluded: invalid flag, land, cloud (including ambiguous and marginal), coastline, high solar zenith angle larger than 70°, saturated flag, moderate or high glint, whitecaps, and atmospheric correction fail. It should be noted that this set of flags is slightly different from the set recommended for OLCI by EUMETSAT (EUMETSAT, 2022). A file is selected if at least half of the pixels in the set plus one was flag-free. Pixels used for matchup comparison were averaged over 5 spatial resolutions: 900, 1,500, 2,100, 3,900, and 5,100 m (3 × 3, 5 × 5, 7 × 7, 13 × 13, and 17 × 17 pixel boxes), centered at the AERONET-OC site (Hlaing et al., 2013). Average
3.2 VIIRS data
VIIRS’s Satellite Level 2—Version 2018.0 imagery was downloaded, for the same areas, from the NASA Ocean Color website https://oceancolor.gsfc.nasa.gov (Gordon and Wang, 1994; Siegel et al., 2000; Bailey et al., 2010). Standard NASA Level 2 data files for VIIRS (with a pixel resolution of 750 m at nadir) include geophysical products of the atmosphere and ocean, such as aerosol optical thickness at 862 nm, remote sensing reflectance,
Pixels flagged by at least one of the following conditions were excluded: land, cloud, failure in atmospheric correction, stray light (except for LISCO), bad navigation quality, high or moderate glint, negative Rayleigh-corrected radiance, negative water-leaving radiance, viewing angle larger than 60°, and solar zenith angle larger than 70°. A file is selected if at least half of the pixels in the set plus one was flag-free. Pixels used for matchup comparison were averaged over 3 spatial resolutions: 2,250, 3,750, and 5,250 m (3 × 3, 5 × 5, and 7 × 7 pixel boxes), centered at the AERONET-OC site (Hlaing et al., 2013; Gilerson et al., 2022). Average
3.3 AERONET-OC data
The ocean color component of the Aerosol Robotic Network (AERONET-OC) was implemented to support long-term ocean color investigations by collecting normalized water-leaving radiance and aerosol optical depth data using the SeaPRISM autonomous radiometer systems CE-318/CE-318T (CIMEL Electronique, France) deployed on offshore fixed platforms (Zibordi et al., 2009; Zibordi et al., 2021). SeaPRISM systems are used to retrieve atmospheric optical thickness and other atmospheric parameters, and modified to perform radiance measurements with a full-angle field of view of 1.2° to determine the total radiance from the sea surface,
The normalized water leaving radiances,
Several AERONET-OC stations had 551 nm band for the whole period of OLCI operation, some of them had new sensor heads with 560 nm band for some part of this period. Considering water type variability between sites and at sites themselves, introduction of some algorithm of band shift most likely would have introduced more uncertainties, so available data at AERONET-OC stations at 551 (or 560) nm were used without changes. SNPP VIIRS also has 551 nm band. Tests were conducted by changing the value of
The aerosol optical depth, aerosol inversions, and ocean color data used in this analysis are version 3 level 1.5 data, which has been cloud-screened and quality controlled to ensure the accuracy of the data. All matchups were observed within a ±2 h window between satellite overpass and in situ observation (Zibordi et al., 2009; Zibordi et al., 2021). More detailed information on AERONET-OC sites is listed in Table 1.
3.4 The Marine Optical BuoY (MOBY) data
MOBY (Marine Optical BuoY) is an autonomous buoy anchored offshore of Lanai, Hawaii. Its radiometry data is used by the NASA—OBPG and EUMETSAT as part of their ocean color validation and vicarious calibration activities (Clark et al., 1997). On each day of deployment, it collects several measurements of upwelling radiance from sensors on its underwater arms (at approximately 1, 5, and 9 m depth) and downwelling irradiance from sensors on its underwater arms as well as at the surface (Voss et al., 2017).
From the MOBY “gold” directory, data from deployments 266 to 272 (2019–2021) was collected for OLCI S3A and S3B, and 249 to 270 (2012–2021) for VIIRS-NPP. Normalized water-leaving radiance calculated from the top sensor and bottom sensor corrected for BRDF is used here because it has the larger available dataset. “Good” and “questionable” data were used to match up with satellite data. Matchups with more than 5 percent differences were excluded from the analysis. MOBY data that matched the bands from satellite sensors was collected with ±2 h of the satellite overpass.
Main atmospheric parameters at the studied sites determined from AC processing and AERONET retrievals are provided in Table 2. Absorbing aerosols were noticeable at several sites with the average
4 Results: Spectral components of uncertainties
Mean
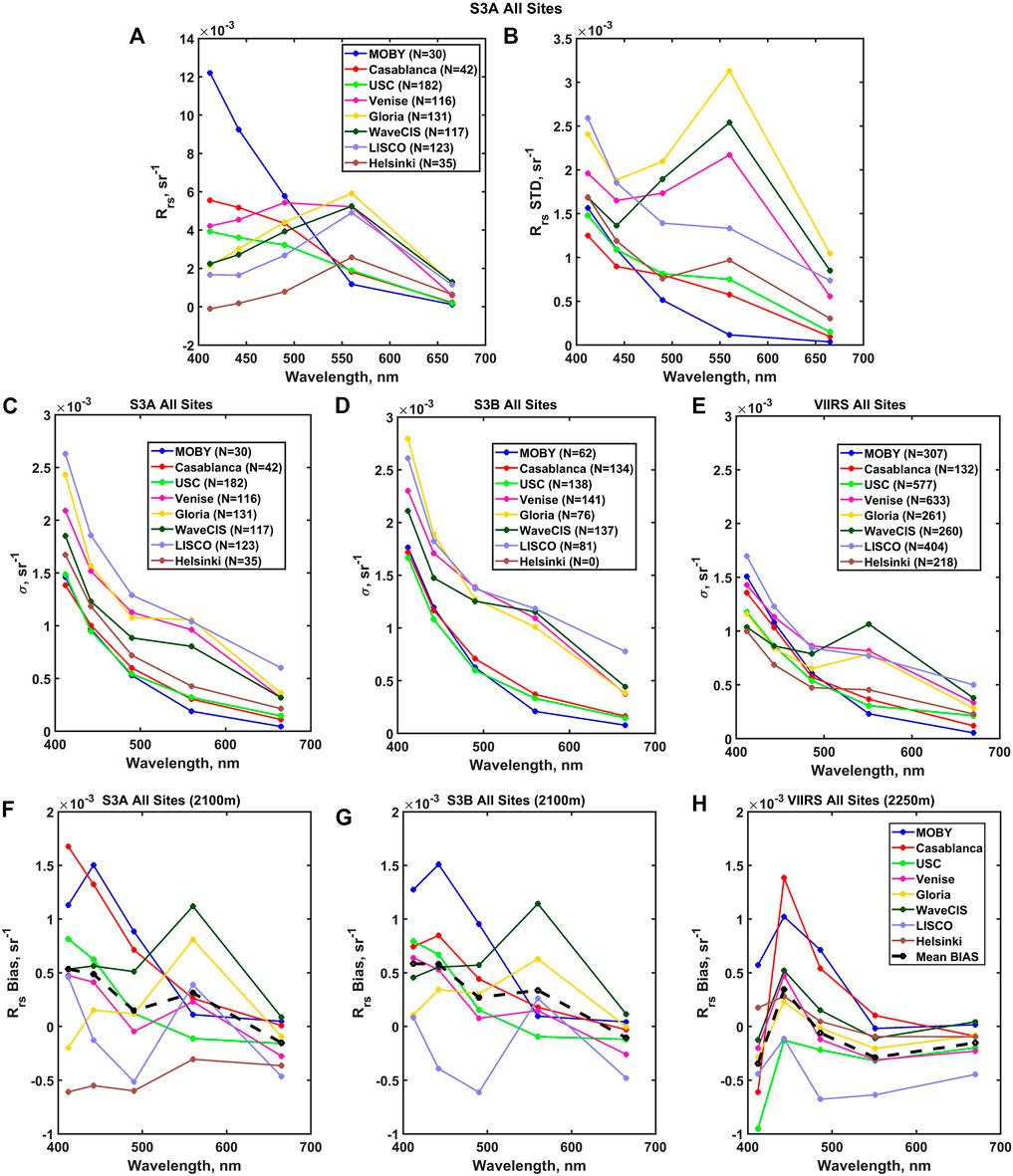
FIGURE 2. (A) Mean
Spectra for all components from Eq. 3 normalized to their maxima are shown for all stations in Figure 3 and these spectra were used in the fitting procedure. In accordance with Eq. 3
Spectra for surface effects are slightly different for different stations because of the small difference in the aerosol parameters used for their calculations. Rayleigh scattering and surface effects spectra are both related to the sky spectra, but the former is divided by the spectrum of the diffuse transmittance t and the latter was simulated based on the composition of Rayleigh and aerosol scattering, which makes these spectra distinct from each other.
Results of fitting are presented in Figure 4 for S3A and S3B. Because of the similarity of the total uncertainties’ spectra for S3A and S3B, it is not surprising that spectral components are also similar. For most of the stations fitted spectra (dash black lines) match well solid lines of total uncertainties spectra confirming that most likely all relevant components were taken into account. For all stations the main component of
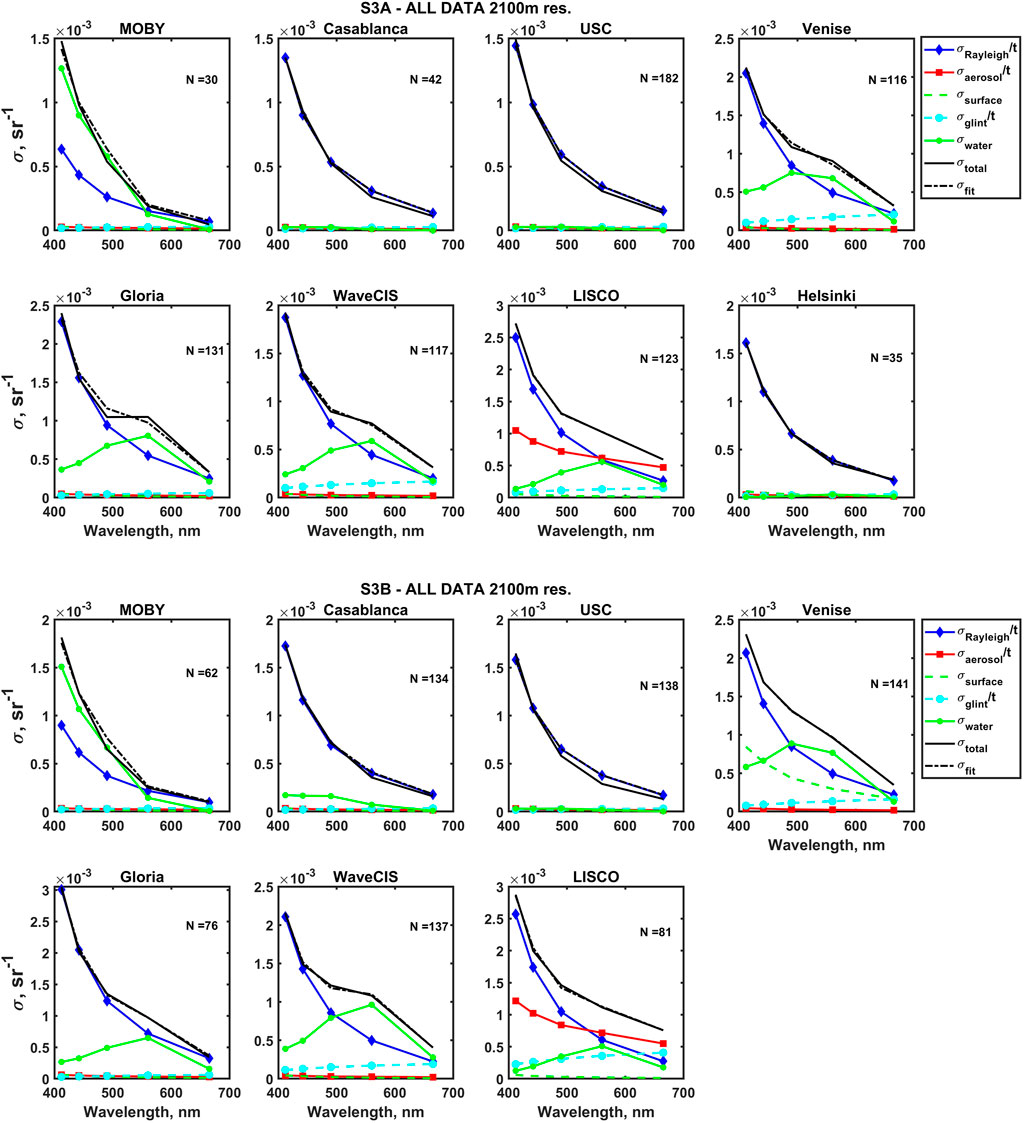
FIGURE 4. Results of fitting for all areas of study for OLCI S3A and S3B. Total uncertainty is the solid black line, and the fitted curve is dashed black line.
Results are very similar for SNPP VIIRS with relatively stable
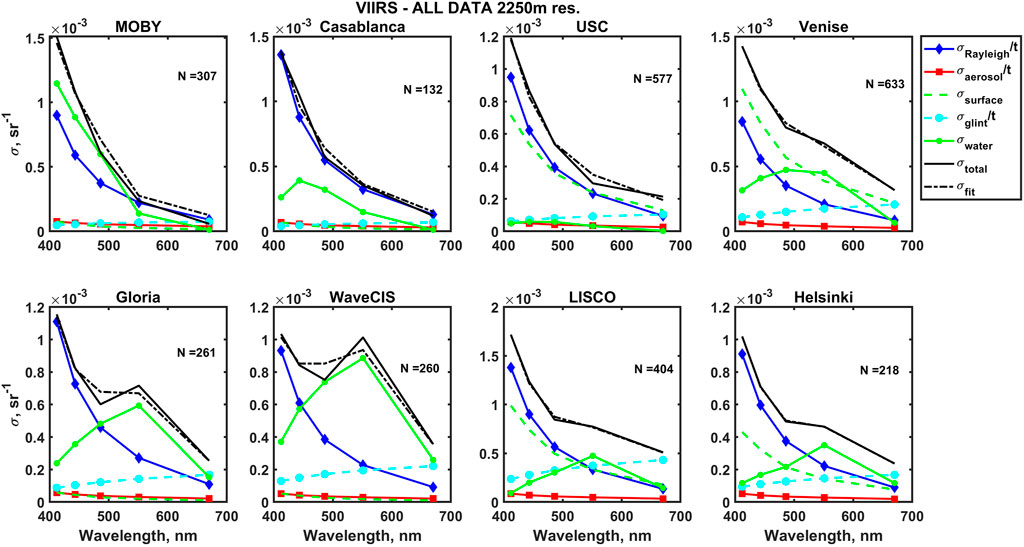
FIGURE 5. Results of fitting for VIIRS same stations. Total uncertainty is the solid black line and the fitted curve is dashed black line.
Since the validity of the assumption that the uncertainties spectra are proportional to the spectra of the components themselves can be less accurate for aerosols than for other components, it is possible that the optimization procedure underestimates the contribution of the aerosol component to the total uncertainties. Generally, however, the aerosol uncertainty levels are consistent with their estimations or slightly below them (Wang, 2007; Gao et al., 2022), where they are at the level of 10–4–4*10–4 sr−1. LISCO station has a pronounced aerosol component for both OLCI S3A and S3B, which is not visible in VIIRS. It can be partially due to the different atmospheric correction processes in OLCI and VIIRS, as well as different number of matchups for these sensors. Some inaccuracies in spectral composition can also be present after optimization because of the similarity of some of the spectra (cf. Rayleigh and surface effects spectra).
For both OLCI and VIIRS sensors the water variability term (solid green line) is very pronounced at the MOBY site. This can be partially due to the similarity of
While results have many similarities between VIIRS and OLCI sensors, some differences are present due to higher uncertainties at OLCI, differences in atmospheric correction algorithms, imperfectness of the fitting approach for the separation of components, especially with similar spectra, and the different number of matchups. Thus, for example, at the LISCO site the shape of the surface and aerosol spectra seemed to switch. For S3A/B, aerosol spectra are close to exponential with
Standard deviations
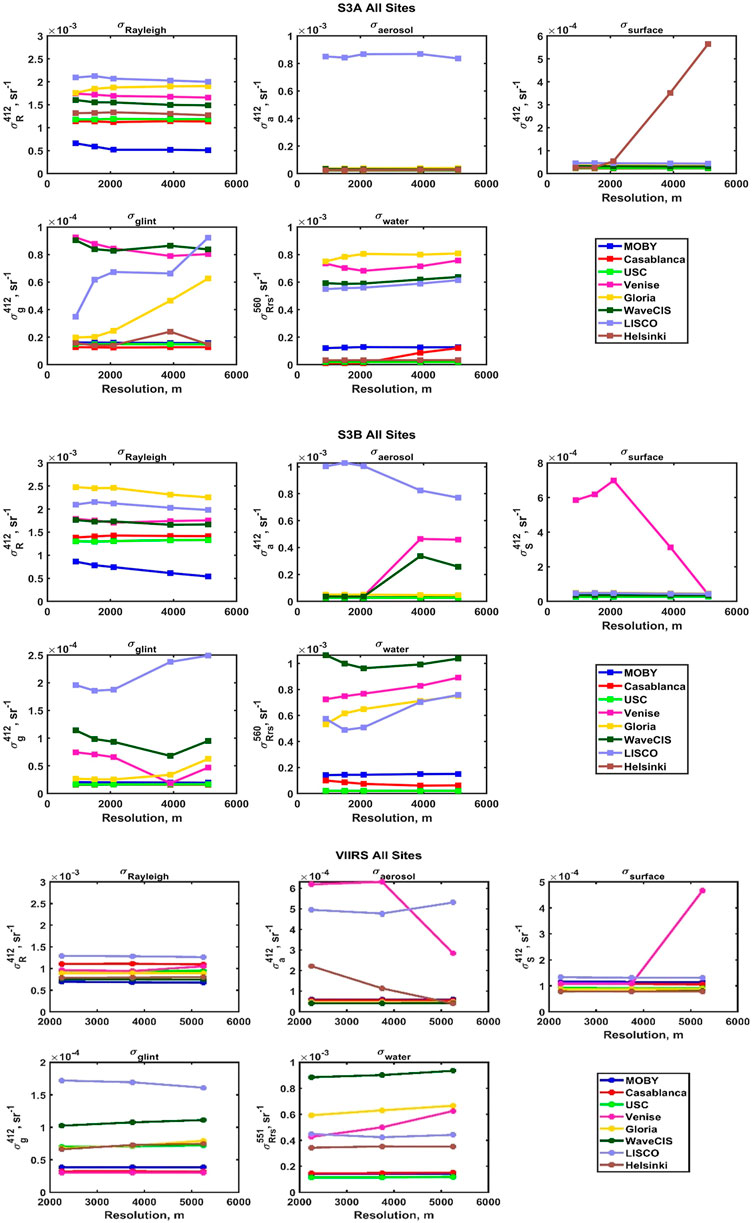
FIGURE 6. Proportionality coefficients in Eq. 7 in the form of standard deviations at referenced wavelengths.
In Gilerson et al., 2022 it was shown that
Following the hypothesis in Gilerson et al., 2022 that Rayleigh components of uncertainties are related to the standard deviation of gains
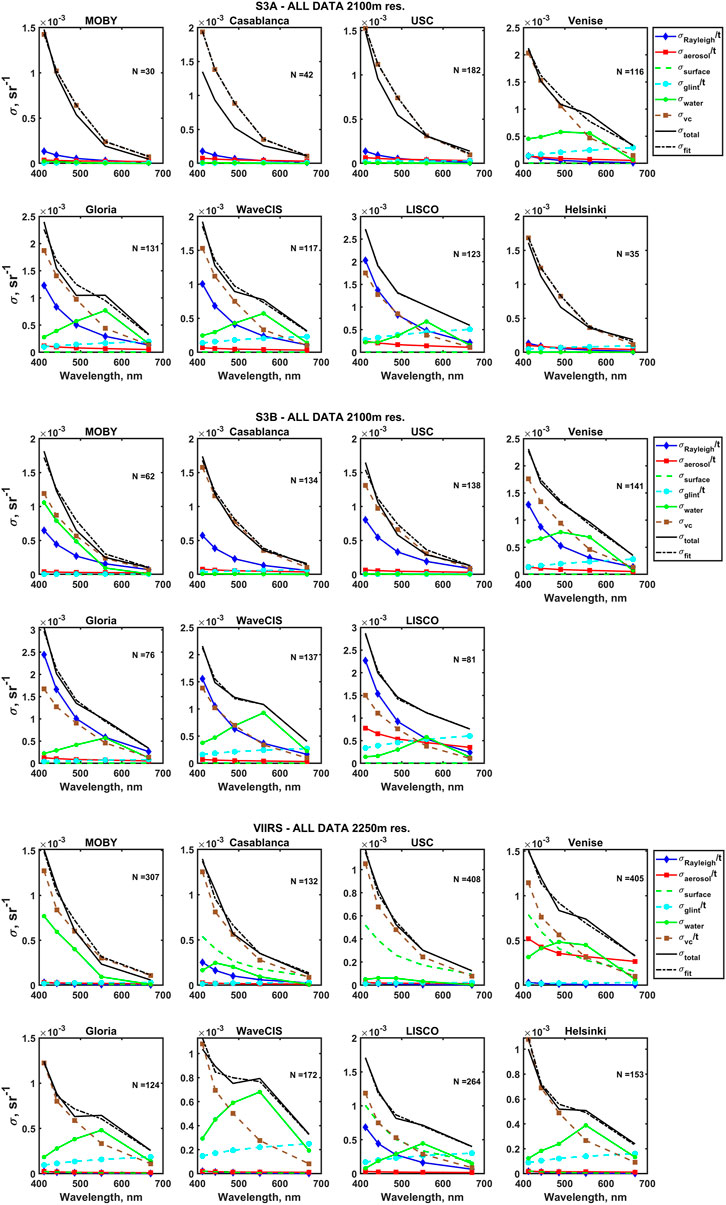
FIGURE 8. Results of fitting for all areas of study for OLCI S3A, S3B and VIIRS with VC term included.
While for VIIRS
Melin, 2022 compared satellite and AERONET-OC data for the European sites and for several sensors launched before OLCI and found that for specific stations the spectra of uncertainties from different sensors are close to each other, thus supporting the hypothesis in Gilerson et., 2022 that such uncertainties are mostly due to the variability of atmospheric parameters (or their calculation).
Zibordi et al., 2022 evaluated uncertainties for the European sites over the period of the so-called “Tandem Phase,” with Sentinel-3B and Sentinel-3A flying just 30 s apart on the same orbit, and showed spectral median percent differences lower than ±1% in the 412–560 nm interval, much lower than when the sensors were on the same orbit but with the nominal phase difference. This is also very consistent with the hypothesis of a dependence of the uncertainties on the atmospheric parameters (or their calculation), since these should be almost the same for both satellites in the Tandem Phase configuration.
As discussed in Gilerson et al., 2022, uncertainties related to AERONET-OC measurements (Gergely and Zibordi, 2014), are about 4 times smaller than the total uncertainties at all stations and thus play a small role in the total uncertainties budget. With OLCI uncertainties typically higher than for VIIRS, this is also true for the evaluation of OLCI sensors data. However, if it will be possible to make the Rayleigh component contribution to the total uncertainties smaller, remaining uncertainties can be similar to the ones from AERONET-OC and the issue will require more attention.
In Figure 9 the spectra of
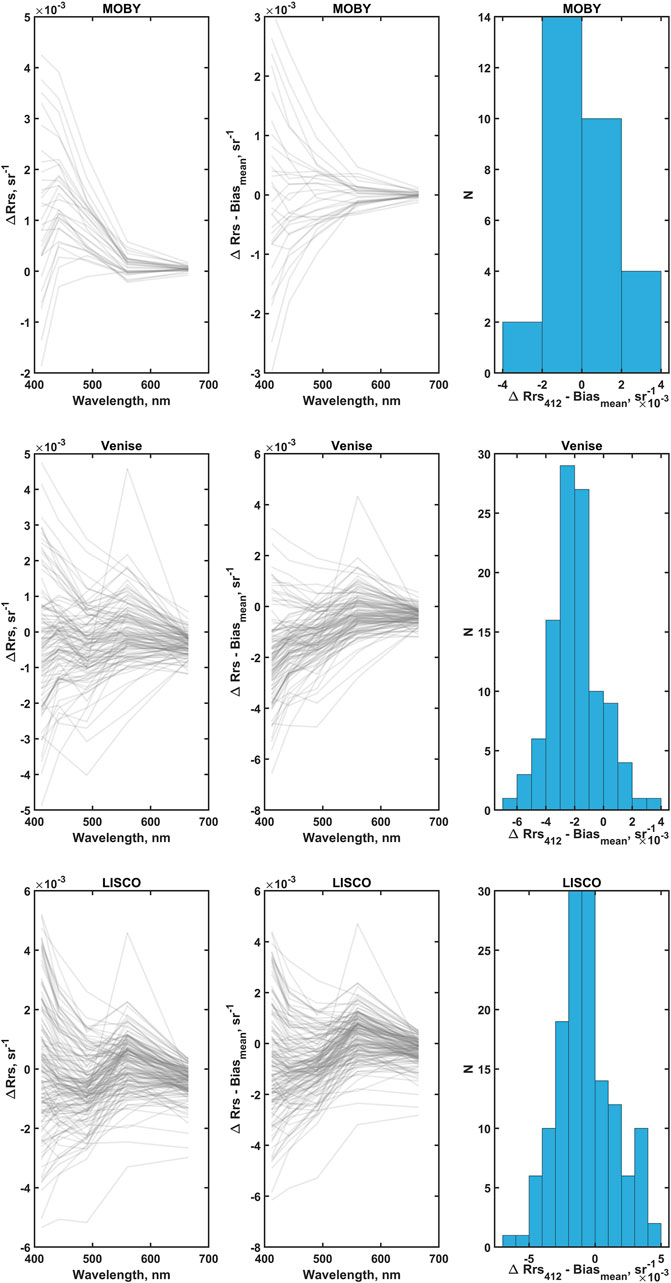
FIGURE 9. Analysis of the S3A
In Figure 10
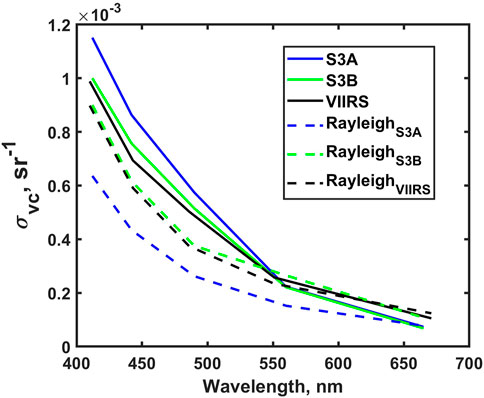
FIGURE 10. Standard deviations during system vicarious calibration for OLCI and VIIRS
5 Conclusion
Uncertainties in remote sensing reflectance for S3A and S3B OLCI sensors were evaluated by comparisons with MOBY and AERONET-OC data for several US and European sites. We applied a previously developed model for the decomposition of uncertainties spectra for OC satellite sensors to OLCI sensors and compared the results with the uncertainties from SNPP VIIRS. The uncertainties for OLCI and VIIRS are found to be spectrally similar, but at the coastal sites OLCI uncertainties are about 50% higher.
It is shown that as previously for VIIRS, the main component in
As in Gilerson et al., 2022 it is assumed that uncertainties in the Rayleigh component are mostly associated with the variability of the atmospheric parameters (ROT) or with their estimation in the current atmospheric correction algorithms (inaccuracies in modeling of the surface pressure), and/or with variability in the vertical distribution of atmospheric gaseous components. This uncertainty corresponds to about 1.5% of ROT at the MOBY site and higher at other stations. The Rayleigh spectral component of the uncertainties was equal to the standard deviation of gains in the vicarious calibration process after conversion to
The reason for the higher uncertainties of OLCI, primarily with Rayleigh spectral shape, in comparison with VIIRS should be further studied. They may be partially related to some specifics in the atmospheric correction processing. In a preliminary manner, we associate them here with the latitude of the site and thus with the solar zenith angle, where in previous studies (Zibordi et al., 2022) dependence on the viewing angle was also noticed. Such dependencies do not exist for the SNPP VIIRS sensor.
To mitigate Rayleigh-type uncertainties atmospheric correction schemes should probably include additional constraints (Steinmetz et al., 2011), which can be even different for open ocean and costal water areas.
Data availability statement
The raw data supporting the conclusion of this article will be made available by the authors, without undue reservation.
Author contributions
AG, EH-E, JA, and RF formulated the original concept of the model. JG, DD, and EK provided OLCI data, described their features and uncertainties. EH-E processed satellite and AERONET-OC data. All authors participated in the uncertainty analysis from different components and provided critical feedback to the final manuscript. All authors contributed to the article and approved the submitted version.
Funding
The funding of this study was provided by the NOAA JPSS Cal/Val and JPSS PGRR programs, CESSRST Grant, NA16SEC4810008, and the NASA OBB grant, 80NSSC21K0562.
Acknowledgments
We thank NASA OBPG for the VIIRS satellite imagery, NASA AERONET Group for processing of the satellite data and support of the operation at the LISCO site. We are grateful to Kenneth Voss and the MOBY team for the MOBY data, PIs of AERONET-OC sites: Giuseppe Zibordi (Venise, Gloria, HLT), Marco Talone and Frederic Melin from the Joint Research Centre (JRC) of the European Commission (Casablanca), Robert Arnone, Alan Wiedemann, Bill Gibson and Sherwin Ladner (WaveCIS), Burton Jones and Matthew Ragan (USC). We also thank the Graduate Center of the City University of New York, The City College of New York, NOAA Center for Earth System Sciences and Remote Sensing Technologies, and NOAA Office of Education, Educational Partnership Program for fellowship support for Eder Herrera Estrella. The statements contained within the research article are not the opinions of the funding agency or the U.S. government but reflect the authors’ opinions. We are grateful to two reviewers whose recommendations led to the improvement of the manuscript.
Conflict of interest
The authors declare that the research was conducted in the absence of any commercial or financial relationships that could be construed as a potential conflict of interest.
Publisher’s note
All claims expressed in this article are solely those of the authors and do not necessarily represent those of their affiliated organizations, or those of the publisher, the editors and the reviewers. Any product that may be evaluated in this article, or claim that may be made by its manufacturer, is not guaranteed or endorsed by the publisher.
References
Ahmad, Z., Franz, B. A., McClain, C. R., Kwiatkowska, E., Werdell, J., Shettle, E. P., et al. (2010). New aerosol models for the retrieval of aerosol optical thickness and normalized water-leaving radiances from the SeaWiFS and MODIS sensors over coastal regions and open oceans. Appl. Opt. 49, 5545. doi:10.1364/AO.49.005545
Bailey, S. W., Franz, B. A., and Werdell, P. J. (2010). Estimation of near-infrared water-leaving reflectance for satellite ocean color data processing. Opt. Express 18, 7521–7527. doi:10.1364/OE.18.007521
Brown, S. W., Flora, S. J., Feinholz, M. E., Yarbrough, M. A., Houlihan, T., Peters, D., et al. (2007). “The marine optical buoy (MOBY) radiometric calibration and uncertainty budget for ocean color satellite sensor vicarious calibration,” in Proceedings of SPIE - The International Society for Optical Engineering, 6744. doi:10.1117/12.737400
Clark, D. K., Gordon, H. R., Voss, K. J., Ge, Y., Broenkow, W., and Trees, C. C. (1997). Validation of atmospheric correction over the oceans. J. Geophys. Res. 102, 17209–17217. doi:10.1029/96JD03345
Coleman, T. F., and Li, Y. (1994). On the convergence of interior-reflective Newton methods for nonlinear minimization subject to bounds. Math. Program. 67 (2), 189–224. doi:10.1007/BF01582221
Coleman, T. F., and Li, Y. (1996). An interior, trust region approach for nonlinear minimization subject to bounds. SIAM J. Optim. 6 (2), 418–445. doi:10.1137/0806023
Cox, C., and Munk, W. (1954). Measurement of the roughness of the sea surface from photographs of the sun’s glitter. J. Opt. Soc. Am. 44 (11), 838–850. doi:10.1364/JOSA.44.000838
El-Habashi, A., Ahmed, S., Ondrusek, M., and Lovko, V. (2019). Analyses of satellite ocean color retrievals show advantage of neural network approaches and algorithms that avoid deep blue bands. J. Appl. Rem. Sens. 13 (2), 1. doi:10.1117/1.JRS.13.024509
EUMETSAT (2020). Ocean Colour system vicarious calibration tool. Available at: https://www.eumetsat.int/ocean-colour-system-vicarious-calibration-tool (Accessed December 22, 2022).
EUMETSAT. (2021). Sentinel-3 OLCI L2 Report for baseline collection Ol_l2m_003. Available at: https://www.eumetsat.int/media/47794 (Accessed December 20, 2022).
EUMETSAT (2022). Recommendations for sentinel-3 OLCI Ocean Colour product validations in comparison with in situ measurements – matchup protocols. Available at: https://www.eumetsat.int/media/44087 (Accessed December 20, 2022).
Fan, Y., Li, W., Chen, N., Ahn, J-H., Park, Y-J., Kratzer, S., et al. (2021). OC-SMART: A machine learning based data analysis platform for satellite ocean color sensors. Remote Sens. Environ. 253, 112236. doi:10.1016/j.rse.2020.112236
Fougnie, B., Marbach, T., Lacan, A., Lang, R., Shlussel, P., Poli, G., et al. (2018). The multi-viewing multi-channel multi-polarisation imager – overview of the 3MI polarimetric mission for aerosol and cloud characterization. J. Quant. Spectrosc. Radiat. Transf. 219, 23–32. doi:10.1016/j.jqsrt.2018.07.008
Franz, B. A., Bailey, S. W., Werdell, P. J., and McClain, C. R. (2007). Sensor-independent approach to the vicarious calibration of satellite ocean color radiometry. Appl. Opt. 46, 5068. doi:10.1364/AO.46.005068
Frouin, R., Schwindling, M., and Deschamps, P. Y. (1996). Spectral reflectance of sea foam in the visible and near-infrared: In situ measurements and remote sensing implications. J. Geophys. Res. Ocean. 101 (C6), 14361–14371. doi:10.1029/96JC00629
Frouin, R. J., Franz, B. A., Ibrahim, A., Knobelspiesse, K., Ahmad, Z., Cairns, B., et al. (2019). Atmospheric correction of satellite ocean-color imagery during the PACE era. Front. Earth Sci. 7–145. doi:10.3389/feart.2019.00145
Gao, M., Knobelspiesse, K., Franz, B. A., Zhai, P.-W., Sayer, A. M., Ibrahim, A., et al. (2022). Effective uncertainty quantification for multi-angle polarimetric aerosol remote sensing over ocean, Atmos. Meas. Tech., 15, 4859–4879. doi:10.5194/amt-15-4859-2022
Gergely, M., and Zibordi, G. (2014). Assessment of AERONET-OC LWN uncertainties. Metrologia 51 (1), 40–47. doi:10.1088/0026-1394/51/1/40
Gilerson, A., Malinowski, M., Herrera, E., Tomlinson, M., Stumpf, R., and Ondrusek, M. (2021). “Estimation of chlorophyll-a concentration in complex coastal waters from satellite imagery,” in Proceedings of SPIE 11752, Ocean Sensing and Monitoring XIII. doi:10.1117/12.2588004
Gilerson, A., Herrera-Estrella, E., Foster, R., Agagliate, J., Hu, C., Ibrahim, A., et al. (2022). Determining the primary sources of uncertainty in retrieval of marine remote sensing reflectance from satellite Ocean Color sensors. Front. Remote Sens. 3. doi:10.3389/frsen.2022.857530
Gordon, H. R., and Morel, A. Y. (1983). “In-water algorithms,” in Remote assessment of ocean color for interpretation of satellite visible imagery: A review. Editors H. R. Gordon, and A. Y. Morel (Berlin, Germany: Springer-Verlag). doi:10.1007/978-1-4684-6280-7,
Gordon, H. R., and Wang, M. (1992). Surface-roughness considerations for atmospheric correction of ocean color sensors 1: The Rayleigh-scattering component. Appl. Opt. 31 (21), 4247–4260. doi:10.1364/AO.31.004247
Gordon, H. R., and Wang, M. (1994). Retrieval of water-leaving radiance and aerosol optical thickness over the oceans with SeaWiFS: A preliminary algorithm. Appl. Opt. 3, 443–452. doi:10.1364/ao.33.000443
Gordon, H. R., Du, T., and Zhang, T. (1997). Remote sensing of ocean color and aerosol properties: Resolving the issue of aerosol absorption. Appl. Opt. 36, 8670. doi:10.1364/AO.36.008670
Herrera-Estrella, E., Gilerson, A., Foster, R., and Groetsch, P. (2021). Spectral decomposition of remote sensing reflectance variance due to the spatial variability from ocean color and high-resolution satellite sensors. J. Appl. Rem. Sens. 15 (2), 024522. doi:10.1117/1.JRS.15.024522
Hieronymi, M., Müller, D., and Doerffer, R. (2017). The OLCI neural network swarm (ONNS): A bio-geo-optical algorithm for open Ocean and coastal waters. Front. Mar. Sci. 4, 140. doi:10.3389/fmars.2017.00140
Hlaing, S., Harmel, T., Gilerson, A., Foster, R., Weidemann, A., Arnone, R., et al. (2013). Evaluation of the VIIRS ocean color monitoring performance in coastal regions. Remote Sens. Environ. 139, 398–414. doi:10.1016/j.rse.2013.08.013
IOCCG (2008). “Why ocean colour? The societal benefits of ocean-colour technology,” in Report No. 7 of the international ocean-colour coordinating group. Editors T. Platt, N. Hoepffner, V. Stuart, and C. Brown (Dartmouth, NS: IOCCG). doi:10.25607/OBP-97)
IOCCG (2010). “Atmospheric correction for remotely-sensed ocean-colour products,” in Reports No. 10 of the international ocean-colour coordinating group. Editor M. Wang (Dartmouth, NS: IOCCG).
IOCCG (2019). “Uncertainties in Ocean Colour remote sensing,” in Reports No. 18 of the international ocean-colour coordinating group. Editor F. Mélin (Dartmouth, NS: IOCCG). doi:10.25607/OBP-696:
IOCCG (2021). “Observation of harmful algal blooms with Ocean Colour radiometry,” in Reports No. 20 of the international ocean-colour coordinating group. Editors S. Bernard, R. Kudela, L. Robertson Lain, and G. C. Pitcher (Dartmouth, NS: IOCCG). doi:10.25607/OBP-1042
Melin, F. (2022). Validation of ocean color remote sensing reflectance data: Analysis of results at European coastal sites. Remote Sens. Environ. 280, 113153. doi:10.1016/j.rse.2022.113153
Mikelsons, K., Wang, M., Kwiatkowska, E., Jiang, L., Dessailly, D., and Gossn, J. I. (2022). Statistical evaluation of sentinel-3 OLCI Ocean Color data retrievals. IEEE Trans. Geoscience Remote Sens. 60, 1–19. doi:10.1109/TGRS.2022.3226158
C. D. Mobley (Editor) (2022). The oceanic optics book (Dartmouth, NS, Canada: International Ocean Colour Coordinating Group). doi:10.25607/OBP-1710
Moore, T. S., Campbell, J. W., and Feng, H. (2015). Characterizing the uncertainties in spectral remote sensing reflectance for SeaWiFS and MODIS-Aqua based on global in situ matchup data sets. Remote Sens. Environ. 159, 14–27. doi:10.1016/j.rse.2014.11.025
Morel, A., Antoine, D., and Gentili, B. (2002). Bidirectional reflectance of oceanic waters: Accounting for Raman emission and varying particle scattering phase function. Appl. Opt. 41 (30), 6289–6306. doi:10.1364/AO.41.006289
Oo, M., Vargas, M., Gilerson, A., Gross, B., Moshary, F., and Ahmed, S. (2008). Improving atmospheric correction for highly productive coastal waters using the short wave infrared retrieval algorithm with water-leaving reflectance constraints at 412 nm. Appl. Opt. 47 (21), 3846–3859. doi:10.1364/AO.47.003846
Qi, L., Lee, Z., Hu, C., and Wang, M. (2017). Requirement of minimal signal-to-noise ratios of ocean color sensors and uncertainties of ocean color products. J. Geophys. Res. Oceans 122 (3), 2595–2611. doi:10.1002/2016JC012558
Ransibrahmanakul, V., and Stumpf, R. P. (2006). Correcting ocean colour reflectance for absorbing aerosols. Int. J. Remote Sens. 27 (9), 1759–1774. doi:10.1080/01431160500380604
S3 OLCI Cyclic Performance Report (2021). Sentinel 3 mission performance center. Available at: https://sentinels.copernicus.eu/documents/247904/4602602/Sentinel-3-MPC-ACR-OLCI-Cyclic-Report-076-057.pdf (Accessed March 3, 2023).
Shi, W., and Wang, M. (2007). Detection of turbid waters and absorbing aerosols for the MODIS ocean color data processing. Rem. Sens. Environm. 110 (2): 149–161. doi:10.1016/j.rse.2007.02.013
Siegel, D. A., Wang, M., Maritorena, S., and Robinson, W. (2000). Atmospheric correction of satellite ocean color imagery: The black pixel assumption. Appl. Opt. 39 (21), 3582–3591. doi:10.1364/AO.39.003582
Steinmetz, F., Deschamps, P. Y., and Ramon, D. (2011). Atmospheric correction in presence of sun glint: Application to MERIS. Opt. Express 19 (10), 9783–9800. doi:10.1364/OE.19.009783
Tynes, H. H., Kattawar, G. W., Zege, E. P., KatsevPrikhach, I. L. A. S., and Chaikovskaya, L. I. (2001). Monte Carlo and multicomponent approximation methods for vector radiative transfer by use of effective Mueller matrix calculations. Appl. Opt. 40 (3), 400–412. doi:10.1364/AO.40.000400
Voss, K. J., Gordon, H. R., Flora, S., Johnson, B. C., Yarbrough, M., Feinholz, M., et al. (2017). A method to extrapolate the diffuse upwelling radiance attenuation coefficient to the surface as applied to the Marine Optical Buoy (MOBY). J. Atmos. Ocean. Technol. 34 (7), 1423–1432. doi:10.1175/JTECH-D-16-0235.1
Wang, M., and Bailey, S. W. (2001). Correction of sun glint contamination on the SeaWiFS ocean and atmosphere products. Appl. Opt. 40 (27), 4790–4798. doi:10.1364/AO.40.004790
Wang, M. (2007). Remote sensing of the ocean contributions from ultraviolet to near-infrared using the shortwave infrared bands: Simulations. Appl. Opt. 46, 1535–1547. doi:10.1364/AO.46.001535
Werdell, P. J., Behrenfeld, M. J., Bontempi, P. S., Boss, E., Cairns, B., Davis, G. T., et al. (2019). The Plankton, aerosol, cloud, ocean Ecosystem mission: Status, science, advances. Bull. Am. Meteorol. Soc. 100 (9), 1775–1794. doi:10.1175/BAMS-D-18-0056.1
Xiong, X., Angal, A., Chang, T., Chiang, K., Lei, N., Li, Y., et al. (2020). MODIS and VIIRS calibration and characterization in support of producing long-term high-quality data products. Rem. Sens. 12 (19), 3167. doi:10.3390/rs12193167
Zhang, M., Hu, C., and Barnes, B. B. (2019). Performance of POLYMER atmospheric correction of Ocean Color imagery in the presence of absorbing aerosols. IEEE Trans. Geosci. Remote. Sens. 57 (9), 6666–6674. doi:10.1109/TGRS.2019.2907884
Zibordi, G., Mélin, F., Berthon, J. F., Holben, B., Slutsker, I., Giles, D., et al. (2009). AERONET-OC: A network for the validation of Ocean Color primary products. J. Atmos. Ocean. Technol. 26 (8), 1634–1651. doi:10.1175/2009JTECHO654.1
Zibordi, G., Holben, B. N., Talone, M., D’Alimonte, D., Slutsker, I., Giles, D. M., et al. (2021). Advances in the Ocean Color component of the aerosol robotic network (AERONET-OC). Ocean. Technol. 38 (4), 725–746. doi:10.1175/JTECH-D-20-0085.1
Keywords: remote sensing reflectance, uncertainties, AERONET-OC, Rayleigh scattering, atmospheric correction, OLCI, VIIRS
Citation: Gilerson A, Herrera-Estrella E, Agagliate J, Foster R, Gossn JI, Dessailly D and Kwiatkowska E (2023) Determining the primary sources of uncertainty in the retrieval of marine remote sensing reflectance from satellite ocean color sensors II. Sentinel 3 OLCI sensors. Front. Remote Sens. 4:1146110. doi: 10.3389/frsen.2023.1146110
Received: 17 January 2023; Accepted: 06 March 2023;
Published: 21 March 2023.
Edited by:
Gennadi Milinevsky, Taras Shevchenko National University of Kyiv, UkraineReviewed by:
Martin Hieronymi, Helmholtz-Zentrum Hereon, GermanyMeng Gao, Goddard Space Flight Center, National Aeronautics and Space Administration, United States
Copyright © 2023 Gilerson, Herrera-Estrella, Agagliate, Foster, Gossn, Dessailly and Kwiatkowska. This is an open-access article distributed under the terms of the Creative Commons Attribution License (CC BY). The use, distribution or reproduction in other forums is permitted, provided the original author(s) and the copyright owner(s) are credited and that the original publication in this journal is cited, in accordance with accepted academic practice. No use, distribution or reproduction is permitted which does not comply with these terms.
*Correspondence: Alexander Gilerson, Z2lsZXJzb25AY2NueS5jdW55LmVkdQ==