- 1Takuvik Joint International Laboratory, Université Laval (Canada) - CNRS (France), ArcticNet, Québec-Océan, Département de biologie, Université Laval, Québec, QC, Canada
- 2BeamSea Associates, Loxahatchee, FL, Unites States
- 3Department of Health and Society, University of Toronto Scarborough, Toronto, ON, Canada
- 4Département de Physique, de Génie Physique et d’optique, Université Laval, Québec, QC, Canada
A better understanding of how spatial distribution patterns in important primary producers and ecosystem service providers such as macroalgae and coral are affected by climate-change and human activity-related events can guide us in anticipating future community and ecosystem response. In-person underwater field surveys are essential in capturing fine and/or subtle details but are rarely simple to orchestrate over large spatial scale (e.g., hundreds of km). In this work, we develop an automated spectral classifier for detection and classification of various macroalgae and coral species through a spectral response dataset acquired in a controlled setting and via an underwater multispectral laser serial imager. Transferable to underwater lidar detection and imaging methods, laser line scanning is known to perform in various types of water in which normal photography and/or video methods may be affected by water optical properties. Using off the shelf components, we show how reflectance and fluorescence responses can be useful in differentiating algal color groups and certain coral genera. Results indicate that while macroalgae show many different genera and species for which differentiation by their spectral response alone would be difficult, it can be reduced to a three color-type/class spectral response problem. Our results suggest that the three algal color groups may be differentiated by their fluorescence response at 580 nm and 685 nm using common 450 nm, 490 nm and 520 nm laser sources, and potentially a subset of these spectral bands would show similar accuracy. There are however classification errors between green and brown types, as they both depend on Chl-a fluorescence response. Comparatively, corals are also very diverse in genera and species, and reveal possible differentiable spectral responses between genera, form (i.e., soft vs. hard), partly related to their emission in the 685 nm range and other shorter wavelengths. Moreover, overlapping substrates and irregular edges are shown to contribute to classification error. As macroalgae are represented worldwide and share similar photopigment assemblages within respective color classes, inter color-class differentiability would apply irrespective of their provenance. The same principle applies to corals, where excitation-emission characteristics should be unchanged from experimental response when investigated in-situ.
1 Introduction
With the acceleration of climate change related effects on marine ecosystems and coastal regions around the globe (He and Silliman, 2019), new developments in ocean coastal monitoring techniques are essential in following these events and mitigating some of the impacts. One of the main factors driving environmental changes is the increase in average temperature worldwide, and regions such as the Arctic are at the forefront (Box et al., 2019; Jansen et al., 2020). Elsewhere, warm water coral bleaching events and associated coral losses are also a well-known and documented example of the impact of increasing temperatures in the marine environment (Hoegh-Guldberg, 1999; Carricart-Ganivet et al., 2012). Another consequence of increasing temperatures is the current trend of decreasing sea ice cover in Arctic and Antarctic regions, which has the potential for impact on many levels within the coastal ecosystem. Where ice normally acts as a barrier for light transmission to depths below, its loss can lead to an increase in the underwater light field and positively impact primary production in coastal ecosystems (Smale et al., 2013; Scherrer et al., 2018; Al-Habahbeh et al., 2020). Conversely, the loss of nearshore ice by the same ice-melt phenomenon can lead to coastal soil erosion by increased wave/storm action (Mentaschi et al., 2018), as well as glacier/iceshelf meltwater and sediment runoff (Hudson et al., 2014; Jack Pan et al., 2019), both of which would normally reduce light availability.
The combination of environmental factor effects makes it a challenge to forecast impacts without adequate tools for maintaining an up-to-date knowledge base of ecosystem dynamics. In this sense, important ecosystem engineers and service providers that are macroalgae/kelp (Bertocci et al., 2015; Krause-Jensen and Duarte, 2016; Teagle et al., 2017) and corals (Wild et al., 2011; Hoegh-Guldberg et al., 2017) can necessarily benefit from increased monitoring. Many kelp and other macroalgae have also long been important in human food consumption, the making of natural products and pharmaceutical applications and fertilizers (see Lähteenmäki-Uutela et al., 2021 for a review) and corals for similar applications besides consumption (Sang et al., 2019). In this context, an understanding of stand and/or reef growth dynamics and changing distributions (see Krause-Jensen et al., 2020) are key in finding appropriate resource management methods to avoid or limit overexploitation.
Coastal monitoring surveys using underwater photography and video transects can normally provide valuable information on benthic habitats. However, underwater substrates such as macroalgae and coral have distinct responses to light (Haxo and Blinks, 1950; Lüning and Dring, 1985; Kieleck et al., 2001; Eyal et al., 2015), which also makes them suitable for other types of imaging and detection methods that may provide additional but otherwise hidden information. Photosynthetic and/or photo responsive organisms such as these have evolved to exploiting visible light energy within the PAR range (i.e., 350/400–700/800 nm) for their metabolism (van den Hoek et al., 1995; Enríquez and Borowitzka, 2010; Suggett, 2010; Kirk, 2011; Lee, 2018) and typical available underwater light field (Eyal et al., 2015). In turn, this allows their study via fluorescence, but also reflectance and absorption as they are light dependent. When found underwater (vs. exposed in the intertidal), spectral response detection and imaging can be challenging due to wavelength dependent absorption (Mobley, 1994b) and suspended particle/water scattering effects (Jonasz and Fournier, 2007). In this context, both the illumination source and optical sensors are subject to these effects and may lead to loss of resolution and reduced signal intensity (i.e., Signal-to-Noise-Ratio), but the latter can be considered for and still allow imaging, detection, characterization, and classification in biological substrates in appropriate conditions (Huot et al., 2022). Much work is also being done towards creating or adapting bio-optical type models and algorithms for optimizing remote sensing-type applications in regions characterized by often complex coastal dynamics (Churnside, 2015; Hieronymi et al., 2017; Mabit et al., 2022). The information acquired can later be used to infer presence, abundance and distribution in substrates of interest. Additionally, advanced imaging and detection methods using lidar (e.g., fluorescence, differential reflectance) may also provide 3D point clouds of underwater substrates and eventually allow to estimate biomass.
Macroalgae color type differentiation has been studied previously using reflectance measurements, either by multi- or hyperspectral satellite (Oppelt, 2012), aerial (Dierssen et al., 2015; Douay et al., 2022), or above-ground detection methods (Kutser et al., 2006; Kotta et al., 2014; Chao Rodríguez et al., 2017; Olmedo-Masat et al., 2020). While red macroalgae can easily be identified close range (i.e., up to a few meters) in a mixed macroalgae color setting via their distinct fluorescence in the 580 nm range, brown and green macroalgae share the same chlorophyll-a (Chl-a) fluorescence response at 685 nm and are more difficult to differentiate solely at emission at 685 nm from the same light source (Kieleck et al., 2001). They can however be differentiated via reflectance in the 525–600 nm range (Chao Rodríguez et al., 2017; Olmedo-Masat et al., 2020) but there is still some imprecision in the process and is best done using additional observation “bands”. Comparatively, corals also show various spectral responses to excitation light (Mazel, 1995; 1997; Hochberg et al., 2004; Roth, 2014; Eyal et al., 2015; Ben-Zvi et al., 2021) which could also vary by developmental stage (Yamashita et al., 2021). Additionally, four main fluorescence bands are typically recognized and are characteristic of most coral species, (Zawada and Mazel, 2014), where variations in fluorescence output between these spectral bands may allow a certain level of classification. Detection methods relying on reflectance require an illumination source capable of emitting within the proper wavelength range, such as a white light or broad-spectrum lighting (e.g., sunlight), which can be used in air without noticeable problems related to light absorption, unlike in water. However, the use of indirect lighting in water is subject to scattering and absorption, via the large volume of water being illuminated, a situation which can be exacerbated in often less than optically transparent coastal waters. While it is possible to focus such broad-spectrum lighting to achieve a narrower target substrate illumination beam, narrow-beam focused laser imaging (or lidar) light sources are prone to give much better resolution and overall imaging capabilities (Caimi and Dalgleish, 2013), by the smaller illuminated water volume at any one time and the potential for much greater energy density on a given substrate of interest. Several in-situ methods have been used to monitor/image coral, notably by special diver-operated underwater camera (Treibitz et al., 2015), hyperspectral sensor (Chennu et al., 2017) and single excitation wavelength multispectral fluorescence Lase-Line-Scan (LLS) imager (Mazel et al., 2003). These methods eventually can provide data which can be analyzed in several ways, either whole (i.e., images) or partially (e.g., image subsets, pixels).
Spectral response remote sensing survey data are regularly analyzed by Machine Learning (ML) methods, where machine learners allow classification of data and provide various accuracy metrics. Methods such as Decision Tree (DT) (Breiman et al., 2017), Support Vector Machine (SVM) (Pal and Mather, 2005), Random Forest (RF) (Breiman, 2001) are applicable to multiple research fields, and are often compared to one another within the same study (Oppelt, 2012; Rossiter et al., 2020a; Rossiter et al., 2020b) (see Maxwell et al., 2018 for a review of these). Additionally, substrate recognition in coral images has also been done by Convolutional Neural Networks (CNNs) (Beijbom et al., 2016) and used in combination to ML (Chennu et al., 2017; Deglint et al., 2019). Otherwise, many satellite, aerial or underwater based multispectral or hyperspectral imaging methods are well suited to pixel-wise segmentation and classification by the above machine learners, or in combination with image validation datasets for data fusion. Selection of the best ML classifier is often difficult in the sense that one may perform better in one situation but less adequately in another (Maxwell et al., 2018), hence the multi-learner or combined approach.
To complement and improve upon current automated underwater classification methods for coastal benthic flora and sessile fauna, our work demonstrates the potential for differentiating between different macroalgal color types and coral under different imaging scenarios. Spectral responses generated by way of multiple laser excitation and emission wavelength parameters between macroalgae color classes, coral genera, coral species, coral structure type and coral shape. These results are visualized prior to classification as part of a process to identify different avenues to accurate classification. Machine learning classifiers are thereafter compared for accuracy in classifying spectral response by these groups.
2 Materials and methods
2.1 Imaging process and data acquisition
The process of acquiring multispectral response image datasets in macroalgae and coral was made possible using a prototype multispectral laser serial imager in a wet lab environment (Huot et al., 2022). The instrument uses 3 continuous-wave (CW) laser diode emitters operating at 450 nm, 490 nm and 520 nm, and is operated by successively scanning each laser and synchronously imaging through separate optical grade viewports into/from a large diameter experimental tank filled with seawater (approximatively 1.5 m W × 7.0 m L × 1.5 m H). Spectral response in macroalgae and corals was generated by laser line-scanning a pre-determined size area measuring approximately 90 cm × 90 cm, at 2.3 m underwater distance, onto a vertically mounted mixed living benthic substrate recreation (see Supplementary Figure S1; Supplementary Table S1 Species list). Water quality optical parameters during imaging corresponded to values in the range of Jerlov 1A waters (Solonenko and Mobley, 2015), following particulate filtration and UV sterilization for a minimum of 24 h. During scanning, reflected and emitted (i.e., fluorescence) light is recorded sequentially by a highly sensitive photodetector (photomultiplier – PMT) through a series of narrow wavelength bandpass emission filters (i.e., 450 nm, 490 nm, 520 nm, 580 nm, 685 nm). These wavelengths correspond to laser source reflectance wavelengths (i.e., 450 nm, 490 nm, 520 nm) and/or known fluorescence emission bands in macroalgae and corals. Living benthic substrates are selected for their healthy appearance (i.e., wild collected macroalgae, cultivated coral fragments) and kept attached to a fixed inert substratum inside the large saltwater biophotonics experimental tank. Specimens were kept in the stable temperature (i.e., local seawater temperature, current and lighting) environment to ensure natural spectral response from laser excitation. Image datasets were obtained for 5 consecutive days at approximate 24-hour intervals.
Imaging was done manually, with one laser source activated at a single time and emission filters used in increasing wavelength sequence for each source. The different excitation and emission wavelength combinations considered for multispectral analysis were as follows: 1) 450 nm excitation + 450 nm reflectance, 488 nm, 520 nm, 580 nm and 685 nm emission; 2) 488 nm excitation + 488 nm reflectance, 520 nm, 580 and 685 nm emission; and 3) 520 nm excitation + 520 nm reflectance, 580 nm and 685 nm emission. Each image consists of the same number of pixels (i.e., 1,000 × 1,000, 1,000,000 total) (Figures 1, 2). The process of image acquisition generated a 12 layer multispectral response layer stack, per imaging scenario. It is from this stack that spectral response can be analyzed and compared for each imaged pixel represented in this case by macroalgae, coral or artificial substrate/background values. Acquired images initially showed a typical detector optical effect, mainly a roll-off/vignetting of luminance, where the PMT sensor is not receiving as much incident light (i.e., photons) from the outer portions of its field-of-view. Images were deemed adequately corrected when little or no illumination gradient was present in the background values between algal and coral substrate pixels (Figures 1, 2). The correction was also verified on the technical target for which the intensity gradient was all but eliminated. For spectral response analysis, algal and coral pixels are first identified via thresholding of the fluorescence intensity, and background non-fluorescing pixels are excluded from analyses.
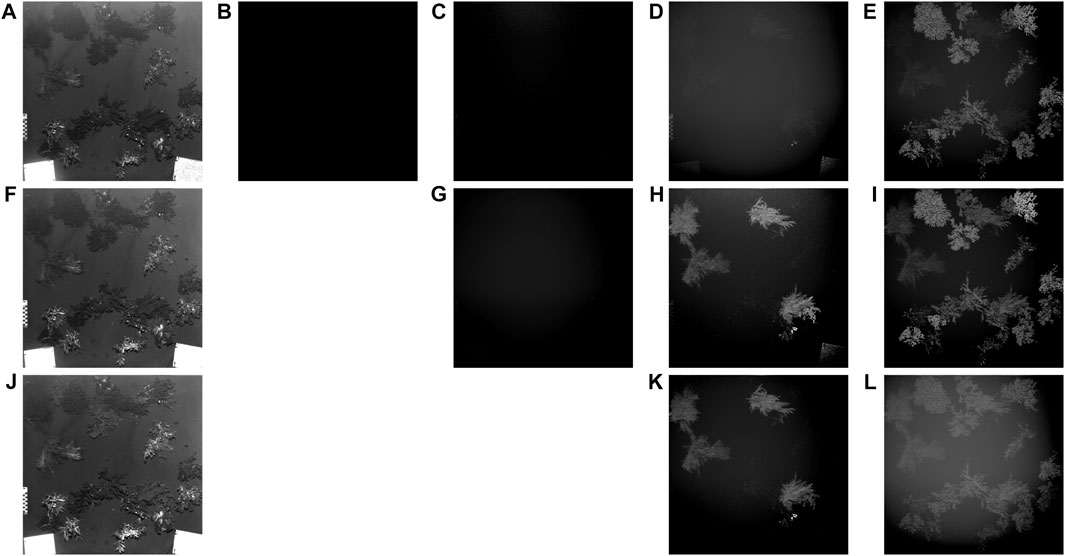
FIGURE 1. Images recorded through spectral response of macroalgae from excitation at 450 nm and reflectance at (A) 450 nm, emission at (B–E) 490 nm, 520 nm, 580 nm 685 nm; excitation at 490 nm and reflectance at (F) 490 nm, emission at (G–I) 520 nm, 580 nm 685 nm; excitation at 520 nm and reflectance at (J) 520 nm, emission at (K) through (L) 580 nm 685 nm. Images were modified for visual purposes only. Adapted from (Huot et al., 2022).
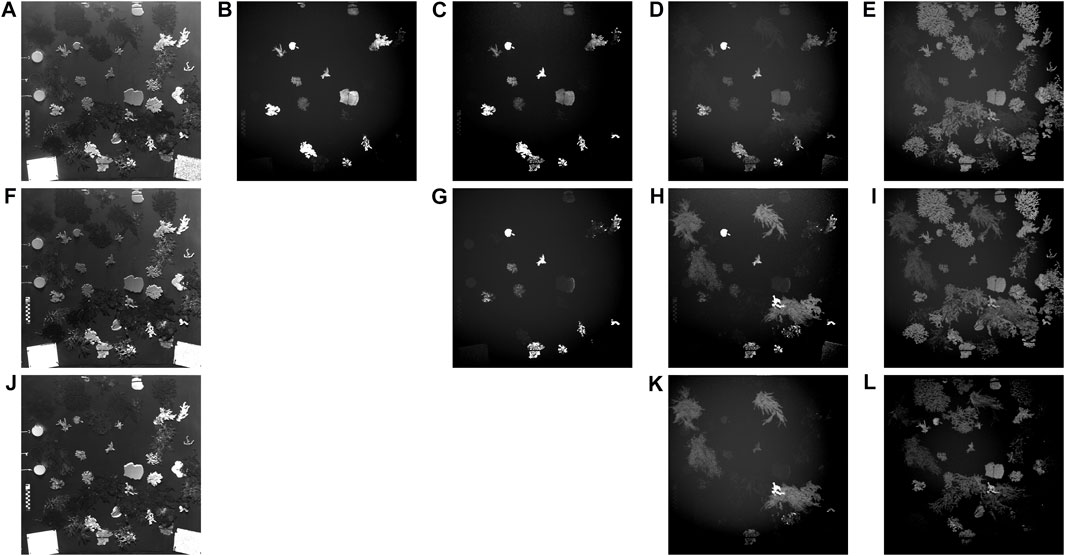
FIGURE 2. Images recorded through spectral response of macroalgae and coral species from excitation at 450 nm and reflectance at (A) 450 nm, emission at (B–E) 490 nm, 520 nm, 580 nm 685 nm; excitation at 490 nm and reflectance at (F) 490 nm, emission at (G–I) 520 nm, 580 nm 685 nm; excitation at 520 nm and reflectance at (J) 520 nm, emission at (K) through (L) 580 nm 685 nm. Images were modified for visual purposes only. Adapted from (Huot et al., 2022).
2.2 Image pixel segmentation
Macroalgae and coral pixel segmentation is done manually, following the identification of fluorescent biological pixels versus non-fluorescent background pixels. For macroalgae, the two fluorescence response layers generated from 490 nm excitation and 580 nm and 685 nm emission were used as a fluorescence thresholding binary filter for their detection (in the macroalgae only imaging test). Comparatively, spectral layers generated from 450 nm to 490 nm excitation and fluorescence emission at 490 nm, 520 nm and 685 nm were selected for identifying coral from the second imaging scenario with macroalgae and coral (see Figures 1, 2). These response layers were chosen for their strong apparent fluorescence intensity and to capture the variability in fluorescence emitted between the various macroalgae and coral specimens. This optical filtration could in theory be done using other excitation wavelengths (less so for fluorescence emission wavelengths) since light absorption in these substrates covers a large portion of the PAR spectrum.
Using the fluorescence signals as a binary pixel filter, subsequent pixel segmentation of all spectral layers was facilitated by programmed fluorescence thresholding and done using QGIS v3.4 software (QGIS Development Team, 2020). This method allowed precise pixel selection and subsequent attribution of corresponding genus and species names, as well as specimen identification (Figures 3A, B). Macroalgal and coral substrates were more difficult to segment in areas of overlap (i.e., more often macroalgae over coral), therefore these pixels were eliminated from analyses when possible but a few mis-segmented pixels remained. In the latter situation, a coral covered by a macroalgae may emit fluorescence at a wavelength characteristic for coral but may be reduced in intensity by the covering algae. Additionally, a given pixel may show multiple spectral response signals, which could increase spectral response variability in mixed and intricate substrate scenarios and hinder classification. A data processing and analysis flowchart explaining the steps from raw data to classification analyses is represented in Figure 4.
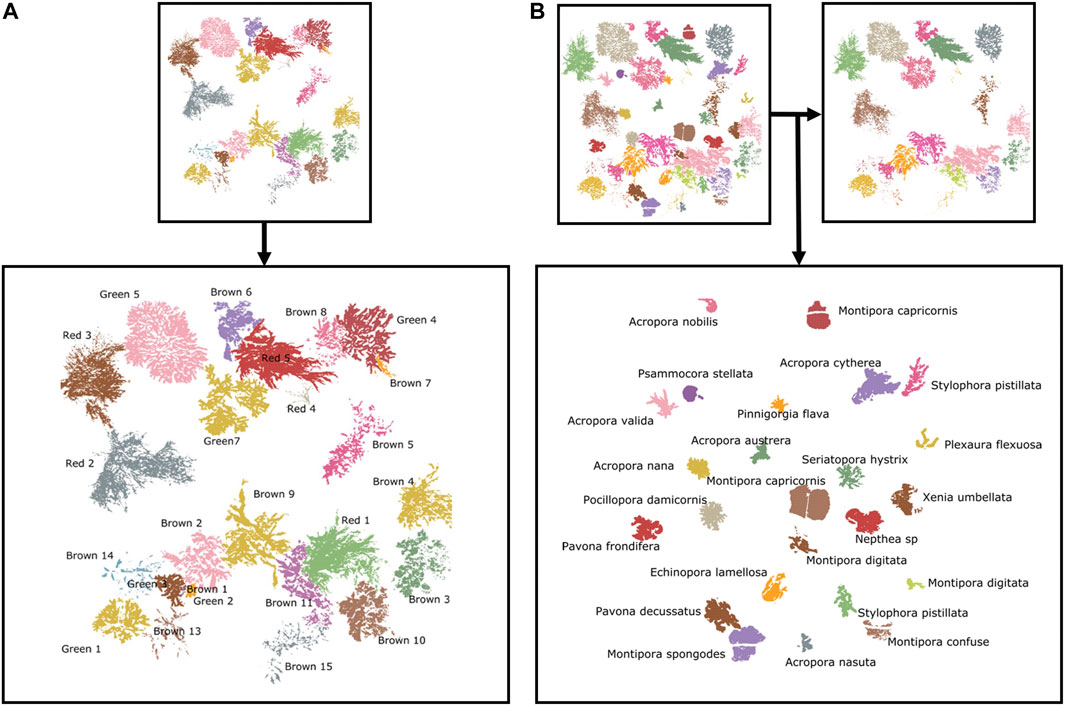
FIGURE 3. Result of segmentation of fluorescent pixels in scenarios with (A) macroalgae and (B) macroalgae + coral, each different algal and coral specimen represented in different colors for visual purposes. Using fluorescence response as thresholding tool, pixels of fluorescent nature in substrates of interest are differentiated from the non-fluorescing background.
2.3 Classification
First, density plots (see Supplementary Figures S2, S3) were done to describe pixel spectral response characteristics and their intensity by substrate type. Following this visualization procedure, explanatory variables were further examined for their contribution to spectral discrimination for each imaging scenario in Principal Components Analyses (PCAs) (R software “prcomp” library).
Second, spectral classification was evaluated at the pixel level via supervised ML methods to compare efficiency versus analysis level complexity. Using R software (v. 4.0.3), “Caret” package, the first used method was K-nearest neighbor classification (KNN). In this method, each pixel’s spectral response characteristics (i.e., light intensity values) are evaluated for its Euclidian distance to every other pixel and corresponding set of spectral responses in the dataset, using the following formula:
where x2 will, for example, be the spectral response variable on a pixel resulting from excitation at 490 nm and emission at 685 nm, and x1 will represent the previous (or in this case, the initial) pixel for the same variable. Y2 will be a second variable for the same pixel as the x2 variable, such as another set of excitation and emission parameters, and so on. KNN distance measurements are considered in this manner and pixel groups generated based on these distance measurement similarities. Pixel classification groupings are generated by assembling or distancing pixels based on these measurements, and classification verified using a subset of the data.
Secondly, the Decision Tree (DT) algorithm (Breiman et al., 2017) (R software, “rpart”: Recursive Partitioning And Regression Trees), allows selection of a single variable (i.e., decision node) from a dataset (i.e., root node) at a time which separates the dataset into two, creating either a terminal node (i.e., categorized data) or additional decision node requiring another variable (i.e., decision node) to split the dataset. This process is repeated several times while the variable chosen at one time is always the one that explains the best split with the remaining data. This process is done for a predetermined number of variables, until the classification tree reaches a classification result (i.e., leaf node). Each decision node is the result of selection of a variable which presents the best Information Gain (i.e., resulting in less entropy in the classified data), or lower Gini Index (i.e., impurity index). One same variable can be used more than once in this process.
Third, in its simplest application, Support Vector Machine (SVM) (Pal and Mather, 2005), (R software, “caret” package) consists of a method in which a line is placed within a 2D plot (or plane) in which the data, organized spatially by similarity in their spectral response characteristics in this case, is organized to best categorize the data. This line is placed between the categorical data points in a way that maximizes the distance/margin between the line and points/samples near this separation boundary that presents certain difficulty in classification (Vapnik, 1995). These points-to-line distances are the support vectors for the line. In a more complex dataset consisting of multiple variables, the analysis is made to use a Kernel method, in this case Non-linear Radial Kernel, transforming the data to pass a hyperplane (versus a line, in 2D) in attempt to classify/separate the categorical data in a way that minimizes distances to the chosen support vectors.
Fourth, Random Forest (RF) (R software, “randomForest” package) (Breiman, 2001) is a method where a predetermined number of DTs are run from the same training dataset. While all variables can be selected at one point in DT analysis explained previously, variables here are pre-selected randomly and data input into each tree is taken at random, with replacement (i.e., Bagging, or Bootstrap aggregation). Contrary to DT, RF allows a predetermined number of randomly selected predictor variables to be chosen from for each set of the DT nodes, thereby truly randomizing trees from one another and evaluating the variability between DTs. These procedures ensure a set of independently grown DTs and which lead to classification predictions based on these repeated classifications.
For additional details on machine leaning classifiers, the reader is directed to specific works explaining these classification methods. In all tested classifiers, 80:20 Train:Test datasets were used for model creation and classification validation. To remove potential spatial effects and correlation to the developed classification algorithms/models, the validation datasets taken from the 2D image matrices underwent an additional row order randomization step prior to classification validation. Training parameters for all classifiers were: control_parameters <- trainControl (method = “repeatedcv”, number = 5, repeats = 5). Training parameters were the same for all models. Effectively, the training dataset being tested is initially divided into 5 equal parts and one part is used as training set and the remaining into test dataset. The model cross-validation of these two sets is repeated 5 times and the average of the error terms calculated.
Classification analysis models were performed on two main datasets, the first with macroalgae only and the second macroalgae and coral, but with macroalgae excluded using segmentation information. These analyses were run while subtracting non-fluorescing substrate pixels (206,442 macroalgal pixels/1,000,000 total pixels) for the macroalgae only scenario, and 64,194 coral pixels for the coral scenario, to reduce processing time. As stated previously, algal and coral types and species identification were associated to the appropriate pixels, via manual/visual (i.e., pixel selection) segmentation, for classification prediction validations. Classification analyses’ model predictions, including some analyses where an attempt is made to identify macroalgae/coral from background pixels, were validated on the manually segmented datasets.
3 Results
3.1 Density plots
Density plots were first done to validate our hypotheses on favorable excitation-emission wavelength combinations for discrimination via spectral response. Initial visualization of the macroalgae only imaging scenario shows brown macroalgae with a higher proportion of high reflectance pixel values than the green or red macroalgae, especially at higher excitation wavelengths of 490 and 520 nm (Supplementary Figure S2). Second, red macroalgae can be identified in their distinct strong fluorescence response near 580 nm range, at all three excitation wavelengths 450 nm, 490 nm and 520 nm. Third, macroalgae did not show significant fluorescence emission in the 520 nm range overall or among different color classes, as expected by the literature, thereby excluding this emission wavelength for macroalgae color class differentiation. Fourth, green macroalgae have a stronger fluorescence response at 685 nm than brown or red macroalgae, but seemingly not at 520 nm excitation. The latter observations are suggestive of a potential for stronger differentiation between greens and browns by comparing emitted fluorescence at 685 nm from excitation at 490 nm and 520 nm. From these results, the following explanatory variables, each corresponding to an excitation-emission reflectance or fluorescence pair, were chosen as a subset in classification analyses: 450 nm–450 nm, 450 nm–580 nm, 450 nm–685 nm, 490 nm–490 nm, 490 nm–580 nm, 490 nm–685 nm and 520 nm–520 nm, 520 nm–580 nm, 520 nm–685 nm (9 variables).
Comparatively, spectral response in different coral genera appears similar in terms of variability to the one observed between different macroalgal color classes (Supplementary Figure S3). Fluorescence response is observable at the same wavelengths as macroalgae, and at lower wavelengths from lower excitation wavelengths of 450 nm and 490 nm. Hence, the three additional following excitation-emission fluorescence emission pairs are included in coral genera classification models: 450 nm–490 nm, 450 nm–520 nm, 490 nm–520 nm, for a total of 12 variables when added to those selected for the macroalgae classification models. Additionally, the presence of coral fluorescence emission at 520 nm from various excitation wavelengths could effectively be used to differentiate between this substrate and macroalgae, as well as fluorescence emission at 490 nm.
3.2 Principal component analyses
PCAs done on fluorescence-segmented macroalgal pixels reveal in more detail how spectral response differs between algal color groups using the previously selected excitation-emission pairs as descriptor variables (Figure 5). As expected, green macroalgae show stronger fluorescence at 685 nm from excitation at 450 and 490 nm, whereas red macroalgae are unique in their strong fluorescence response in the 580 nm region, from excitation at 490 and 520 nm mostly, but also 450 nm. Brown macroalgae are more difficult to discern from green macroalgae as both respond to excitation at selected emission wavelengths. The former however appear to have lower fluorescence response than greens at 685 nm. It is the reflectance in brown macroalgae that is a potential differentiating factor between these two algal color types, but there is noticeable variability in this response for the algae subject to the imaging process.
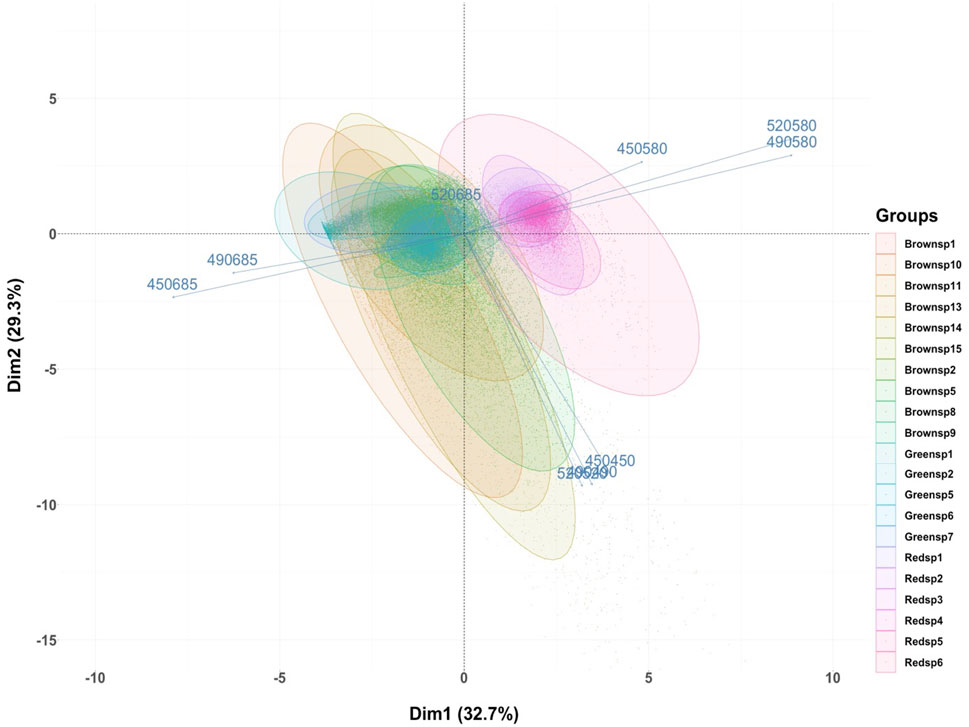
FIGURE 5. Principal Component Analysis plot for the macroalgae only scenario, (left) showing red, brown, and green macroalgal color groups, and (right), by specimens (or individual fragments) per color class, are identified, by their different response (i.e., pixel intensity) in relation to various excitation (450, 490, 520 nm) and reflectance (450, 490, 520 nm) or emission (580, 685 nm) wavelengths. Confidence level of 95% for ellipses with full scan area of 1,000 × 1,000 pixels.
PCAs performed on coral genus, specifically excluding macroalgae after pixel intensity thresholding at 520 nm fluorescence emission from excitation at 490 nm, show some differentiation between genera along with some variability (Figure 6). Species/genera capable of emitting fluorescence at 685 nm, in this case soft corals of the genus Nephthea sp. and Xenia umbellata, and less at other “non Chl-a associated” emission wavelengths investigated, drive an important part of group differentiation of corals in this scenario. Fluorescence emission at 490, 520 and 580 nm comparatively separate other genera/species from these stronger Chl-a emitting ypes. Overall, PCA plots on coral species/genera show a certain gradient in the fluorescence response in 2-D with group overlap.
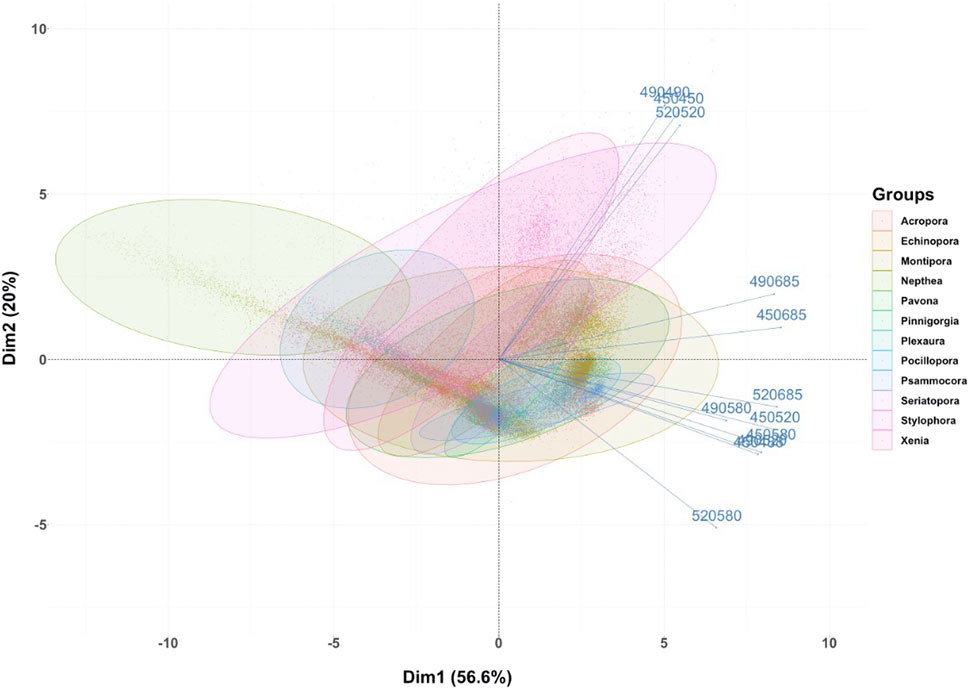
FIGURE 6. Principal Component Analysis plot for the macroalgae + coral imaging scenario, for which macroalgae image pixels were removed by fluorescence thresholding and segmentation. Identified coral species/genera (left) and genus (right) are shown by their variability in spectral response, (i.e., pixel intensity) in relation to variables consisting of the combination of one excitation (450, 490, 520 nm) and one reflectance (450, 490, 520 nm) or emission (490, 520, 580, 685 nm) wavelength parameter. Confidence level of 95% for ellipses with full scan area of 1,000 × 1,000 pixels.
An additional variable of interest is reflectance intensity from 450, 490 and 520 nm excitation, where high reflectance is apparent in most hard coral specimens, and somewhat less for soft corals. Soft corals are however attached to a hard and possibly more reflective support substrate, thereby possibly showing low reflectivity and high Chl-a induced fluorescence in the pixels with the soft coral tissue but higher reflectivity and low Chl-a induced fluorescence in the pixels where the coral support structure was located. As expected, no clear relationship is observable between coral vertical structure and fluorescence (i.e., erect or flat) (not shown), likely since vertical structure is not a strict determinant of the fluorescence mechanism, where both forms can show species of soft and hard corals. However, soft corals chosen for this study appear to be somewhat differentiable from hard corals (Figure 7), but this is most likely since most soft corals in the chosen species emit more in the 685 nm region from Chl-a fluorescence.
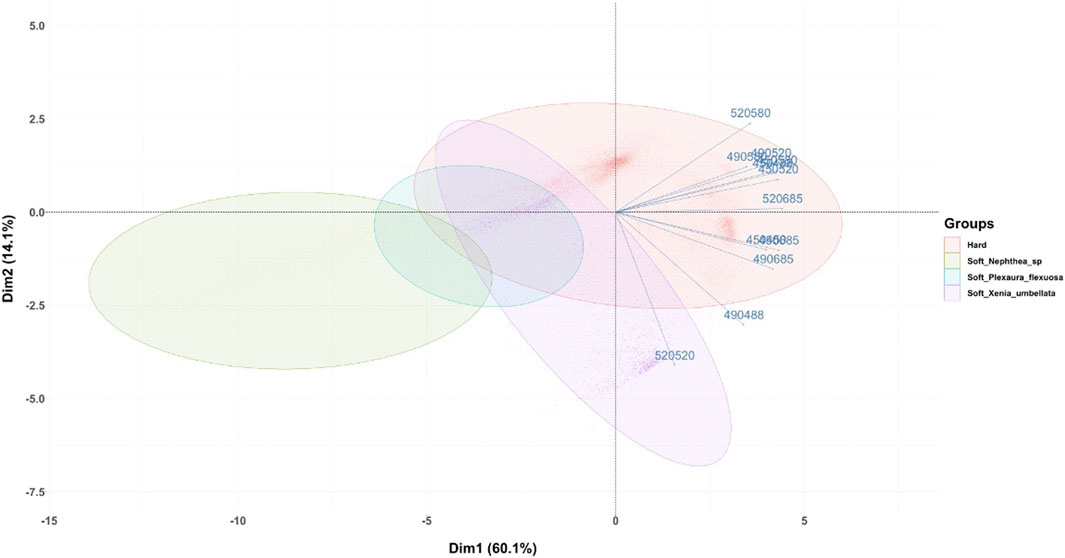
FIGURE 7. Principal Component Analysis plot for the macroalgae + coral imaging scenario, for which macroalgae image pixels were removed by fluorescence thresholding and segmentation. Corals are depicted as either hard or soft with genera/species identified in the latter, according to the variability in spectral response (i.e., pixel intensity) in relation to variables consisting of the combination of one excitation (450, 490, 520 nm) and one reflectance (450, 490, 520 nm) or emission (490, 520, 580, 685 nm) wavelength parameter. Confidence level of 95% for ellipses with full scan area of 1,000 × 1,000 pixels.
3.3 Classification
The first classification analysis considered was the DT analysis for its relative simplicity and usefulness when evaluating optical component selection, and possibly help reduce computational requirements to classification. Each evaluation node considers one spectral response descriptor, or “excitation-emission” pairing, at a time. This allows identification of classification tool based on hardware (i.e., binary, yes/no) versus more complex computational means.
3.3.1 Fluorescence intensity thresholding and Decision Tree for identifying fluorescent substrate pixels
For macroalgae, results suggest a very high classification efficiency towards identifying Chl-a fluorescent, macroalgal components, from zero/low emitting background pixels via the fluorescence response at 685 nm (i.e., Chl-a) and excitation at 490 nm (Supplementary Figure S4). This response can be used to identify fluorescent substrates, in this case green and brown macroalgae, from the surrounding background, if non-fluorescent. Otherwise, red macroalgae can be identified by emission at 580 nm, while green and brown macroalgae by their stronger fluorescence at 685 nm, from excitation at 490 nm. However, there is a misclassification rate of approximately 30%, using these two/three classifying variables to differentiate brown from green macroalgae, which appear to have similar spectral response.
While many corals can emit fluorescence strongly in the 400–520 nm wavelength range, macroalgae show stronger fluorescence emission in the 560 nm–700 nm range. This difference by itself is not sufficient for separation of the two substrate types since some corals can also emit fluorescence in this range. However, differentiation of corals from macroalgae seems efficient from reflectance comparison at 490 nm (Supplementary Figure S5), and another DT analysis (not shown) was done and shows differentiation of coral from macroalgae to be fairly accurate using the single discrimination factor of fluorescence at 520 nm, following excitation at 490 nm. This is also evident from the image dataset (i.e., Figures 1, 2) presented in the Materials and Methods section.
In an analysis where corals are pre-identified and grouped by genera (e.g., from segmentation, result of fluorescence thresholding) (i.e., not shown), there is a higher mis-classification rate than for macroalgal color type classification. This would be partially the result of there being many more coral types to differentiate by spectral response to begin with, and possibly caused by the DT’s classification algorithm which uses one spectral response variable/descriptor at a time in a situation where spectral responses are overlapping between genera and species more so than in between macroalgae color class types. Logically, spectral response for macroalgae within a same color class are likely to be very similar as well since the photopigment compositions are known to be uniform within a given color class. DT algorithm therefore appears less suited for the analysis of this more complex coral dataset where genera are considered separately.
For both macroalgae and coral classification, a few aspects of the imaging process and data preparation methods may play a part in this classification error. Notably, macroalgal/coral substrate of different color class and/or genera structural/physical overlap in adjacent pixels, leading to segmentation error and mixed spectral response signals. Also, mismatch in pixel location between overlaid spectral response layer images, due to slight wavelength-dependent differences in refraction index on the outgoing laser beam and reflected light or emitted fluorescence. Moreover, inter-pixel intensity variability within the same macroalgal or coral specimen could be influenced by pixel dimensions.
3.3.2 KNN, SVM and Random Forest learners
3.3.2.1 Classification—macroalgae
Because of the relative straightforwardness of discriminating coral from macroalgae on one or two spectral response band regions by DT algorithm, classification analyses in these two substrates are done separately for subsequent machine learners. To begin, classification results in macroalgae based on KNN, SVM and RF classifiers show different efficiency between macroalgal color groups (Table 1). Efficiency is high at 90%–96% for the more complex classifiers SVM and RF, as well as KNN, whereas DT is showing higher rate of misclassification, efficiency being at below 80% for all iterations (macroalgae only, coral only, macroalgae + coral). While DT classifier is useful in illustrating how the two main substrate groups can be identified and by which important variables in the early classification process, the remaining classifiers indicate strong potential when considering a deeper level of classification. Only RF classification results are shown in detail for brevity, as it appears to be best of 5 chosen classifiers to properly identify pre-segmented groups, by a small margin, but with higher Kappa value (see Supplementary Table S1). A confusion matrix (Figure 8) shows pixel classification numbers for the testing dataset for each macroalgal color type.
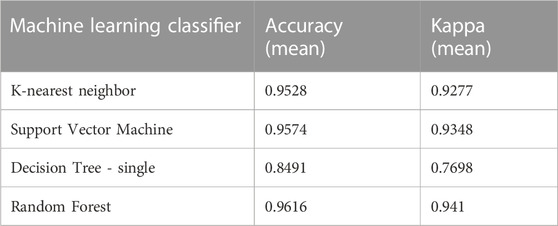
TABLE 1. NKK, SVM, DT and RF machine learner classification accuracy comparison on macroalgal spectral response at 450 nm, 490 nm and 520 nm excitation to 450 nm, 490 nm and 520 nm reflectance and 580 nm and 685 nm fluorescence emission.
Since red macroalgae formed a distinct group of clusters in the macroalgae PCA plot, a separate Random Forest classification analysis was subsequently run on the subset data to determine if the two main species (Grateloupia sp. and Halymenia sp.) could be differentiated in this context. Additionally, although there was some overlap in the green and brown macroalgal PCA plot, a separate Random Forest classification analysis was also done with the brown macroalgae to attempt differentiation between the three species present (Sargassum sp., Dictyota sp. and Padina sp.)). Separate PCA plots are not shown but classification analyses show “acceptable” results for the separate red (88% accuracy) and brown (88% accuracy) groups, albeit some misclassification (in red - class error: Grateloupia sp: 11.1%, Halymenia sp.: 11.9%)) (in brown - class error: Sargassum sp: 12.2%, Dictyota sp.: 4.87%, Padina sp.: 24.9%) (see Supplementary Figures S6, S7 confusion matrices for pixel sample numbers for each color group and corresponding species).
3.4 Classification–coral
From a visual analysis of processed spectral response images (i.e., Figure 2) and PCA grouping by spectral response (i.e., Figures 6, 7), certain coral genera/specimens indicated the possibility of spectral response differentiation. Following the same analysis structure as for macroalgae but the additional excitation and emission wavelength channels, RF algorithm shows once again to be accurate among classifiers (Table 2), along with SVM and K-nearest neighbor with near 90% accuracy, in the current test conditions. In an analysis excluding pixels where macroalgae or background pixels were present, classification parameters suggest good separability in coral genera studied (Supplementary Table S2). These results suggest that the proposed Machine Learning algorithms are appropriate in pixel-wise image spectral classification in coral, as for macroalgae.
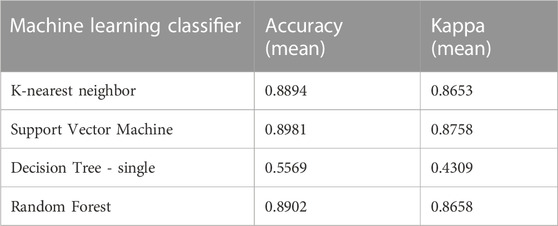
TABLE 2. NKK, SVM, DT and RF machine learner classification accuracy comparison on coral spectral response at 450 nm, 490 nm and 520 nm excitation to 450 nm, 490 nm and 520 nm reflectance and 490 nm, 520 nm, 580 nm and 685 nm fluorescence emission.
Compared prediction “accuracy” in the different classification models, which is the percentage of correctly classified data values among all data values subject to classification, “Kappa” (Cohen’s Kappa) is the accuracy but normalized to the baseline of random chance in the data. More specifically, it takes into consideration the imbalance in the distribution of data within the categorical data:
This is partially the case with the coral dataset, more specifically, where pixel numbers range from just above 600 for some corals to over 15,000 in others. Nonetheless, both accuracy and Kappa report similar average model prediction values for both macroalgae and coral. This result suggests that the unbalanced pixel ratio between groups does not appear to affect classification, and a confusion matrix shows these numbers (Figure 9). Overall, RF classification of coral as grouped by genus showed noticeable separability. This is especially the case for genus and or species that showed distinct fluorescence emission patterns. For example, soft corals Xenia sp. and Nephthea sp. emitting more so near 685 nm, as well as for corals emitting fluorescence from excitation at 450 nm, but much less at 490 nm (refer back to Figure 2).
4 Discussion
This study expands on previous work towards the detection and classification of macroalgal and coral spectral response (Bates and Craigie, 1988; Topinka et al., 1990; Kieleck et al., 2001; Baird et al., 2006; den Haan et al., 2013; Gameiro et al., 2015; Sasano et al., 2016; Huot et al., 2018). While near-field remote sensing reflectance (aerial or underwater), and RGB imagery commonly can provide detection and some level of differentiation between algal color classes and coral types, we provide an insight into underwater laser imaging biological substrate classification (e.g., Huot et al., 2022). In this sense, effective underwater imaging, detection and, ultimately, classification range are extended compared to these other methods, making them suitable in a variety of environments and situations and possibly adapted to lidar techniques. Specifically, macroalgae and coral differentiability is evaluated in a controlled but realistic simulated mixed-species benthic environment. Results show significant differences in spectral responses for different algal color groups, while certain coral genus and species show various degrees of differentiation possible.
4.1 Identifying spectral response patterns in macroalgae and corals
A strong Chl-a associated fluorescence response in green and brown macroalgae near 685 nm emission is apparent as expected and is a strong contributor to their respective clustering from red macroalgae. Additionally, this response in greens appears relatively stronger when using 450 and 490 nm excitation wavelengths than for brown macroalgae. The fluorescence intensity disparity however lessens at 520 nm excitation, indicative of an additional spectral region that could be essential in more clear-cut differentiation between green and brown types. This shared 685 nm emission response in green and brown types is apparent in the partial overlap of the 2D clusters. Our data also reveals that reds are readily and as expected, identified from greens and browns by the former’s strong response in the 580 nm wavelength range. Meanwhile, reflectance is also important in differentiating between selected algal types, appearing to be significant when differentiating brown from green macroalgae. Density plots and PCA clustering data highlights the importance of reflectance in differentiating between algal color types. However, while PCA cluster plots show strong degree of separability at 520 nm excitation, it is not sufficient by itself to segregate the algal color classes into well-defined clusters. There is however data suggesting it may reverse past 520 nm and brown macroalgae may show a higher 532/473 nm, 532/490 nm, 532/450 nm ratio of fluorescence than greens at 685 nm emission (Huot et al., 2018), and other studies have observed this pattern as well for reflectance in air (Chao Rodríguez et al., 2017; Olmedo-Masat et al., 2020).
Reflectance may be a useful feature for classification when algal types of the same color class are present in the same environment, but surface reflectivity and/or surface structures differ, characteristics which are often co-related. This can be described as surface albedo, when reflection angle is not considered but only the ratio of reflected light vs. incident light, but also BRDF (Bidirectional Reflectance Distribution Function) which considers the angle at which the incoming light is from as well as at which angles it is reflected. An example of differences in reflectance values between substrates could be between northern cold-temperate to arctic waters non-kelp brown macroalgae (e.g., Desmarestia sp.) versus kelp (e.g., Saccharina latissima, Laminaria solidungula, Alaria esculenta). Flatter surfaces of kelp are more likely to show increased reflectivity by their characteristic specular surface (i.e., non-Lambertian) than irregular or filamentous structure, such as Desmarestia sp. (more Lambertian-like). Known fluorescence reabsorption processes related to an overlap between absorption and fluorescence emission in Chl-a photopigment, occurring within more complex structured macroalgae such as Desmarestia sp., likely give the species a particular spectral reflectance and florescence signature to species with less reabsorption occurring (e.g., uni-surfaced, compact cell-wall structure) as in many kelp species (Huot et al., unpublished). Other species showing additional structural complexity, such as brown macroalgae Agarum clathratum, with its highly perforated and characteristic “kelp-like” wavy smooth surface, could be another case of a species somewhere within the spectrum of highly reflecting and fluorescing (i.e., low reabsorption) and low reflectance and high reabsorption/lowered fluorescence. Also considered highly reflective, due to their mostly calcareous nature, coralline algae were also observed on a few spot locations over Sargassum sp. during imaging tests, but these calcareous algae were not alive. Since they did not represent a sizeable surface, they were automatically excluded from datasets as part of higher valued outliers. However, when alive, their fluorescence emission at possibly confounding wavelengths to macroalgae (e.g., reds) could possibly have been differentiated by the large difference in reflectance. Similar analysis could be applied to red coralline algae (e.g., Clathromorphum sp., Corallina sp., Lithothamnium sp.), largely found in cold-temperate and arctic hard substrate sub-tidal environments, where brown macroalgae and kelp, as well as red macroalgae can be abundant. This is an example where prior knowledge of species potentially encountered during a survey is essential in understanding spectral response patterns and possible explanations in similarities and differences. In the present study, although fluorescence at 685 nm and reflectance can provide part of the information leading to the differentiation of brown versus green macroalgae, it is fluorescence at 580 nm and 685 nm wavelengths that is useful at identifying red from green/brown macroalgae, respectively, based on the random forest classification model performance. Overall, classification variables/parameters visualized in the initial PCA clustering analyses show the evident differentiation potential in spectral responses of macroalgae of differing color types.
On the other hand, the fluorescence spectral response in coral of this study does not seem to allow classification using single fluorescence response parameters as in algae (e.g., green vs. red at 580 nm) to differentiate between types. This can be due to a higher resemblance in the photopigment and fluoroprotein assemblages responsible for fluorescence and consequently, similar fluorescence response signatures, when classification parameters are considered one at a time. The exception is with soft octocorals selected in this study which are showing mostly Chl-a fluorescence response at 685 nm and less/none at other wavelengths tested, the former being a response associated to symbiotic dinoflagellate of the genus Symbiodinium (Schubert et al., 2016). While this Chl-a characteristic fluorescence response can also be present in some of symbiote-present Scleractinian corals, the latter also respond at other lower emission wavelengths by reaction from fluoroproteins (Alieva et al., 2008). Discrimination for structure, soft versus hard, is also observable to a certain extent, and can be explained by mostly Chl-a fluorescence in soft octocorals corals versus multiple fluorescence emission bands in Scleratinian corals. Species observed in this study may of course not be present at the same geographical locations, hence differentiation accuracy is most likely dependent on whether there are species with overlapping spectral response characteristics. Although it was possible to perform classification of macroalgae and coral simultaneously, we opted to identify/isolate coral image pixels from macroalgae by coral’s fluorescence response at 520 nm, which was present in species present in this study (except two species at 685 nm). Other fluorescence filter wavelengths may provide similar differentiation efficiency between these two fluorescence capable organisms. Substrate, hence, pixel overlap in images with both substrate types, would possibly have an additional effect towards reducing classification prediction accuracy since spectral response signature would be additionally mixed.
4.2 Classifying identified spectral response clusters by machine learning
Overall, chosen machine learners in this study can differentiate with high accuracy both macroalgae color classes, as well as the coral genera chosen for imaging. The 2D PCA visualization analyses already had shown color-class clustering to a reasonable degree in macroalgae, and to a lesser but still present extent for some coral genera, especially along the horizontal axis (or horizontal-diagonal axis). This visualization technique also suggests some variability in spectral response between specimens of the same species, or color class, and should be taken into account. Naturally, the consideration for higher dimensionality in the classification algorithms (i.e., SVMs), and randomized, multi-step/node DTs within Random Forest algorithm can identify the finer characteristic differences in pixel spectral response “assemblages” or signatures between macroalgal and coral specimens. This is characteristic of a few other studies where SVM and Random Forest scored high in accuracy among machine learner comparisons, albeit being relatively simple in application and comprehension.
On a comparative and perspective note, Convolutional Neural Networks (CNNs) and Deep Learning (DL) methods, are also valid choices for automated image and object classification and identification. Having access from thousands to hundreds of thousands of images is however usually required for good classification performance in complex images. In the context of multispectral laser serial imaging, reflectance image stills of different objects of interest could be isolated for an analysis appropriate format. Co-acquired high resolution images would also provide the same image classification potential and benefits. While our study did not provide sufficient images due to the nature of the experimental design (i.e., one scene without movement), image resolution shows possibility in having a CNN or DL classification process running in parallel to the machine learning fluorescence and reflectance threshold classification process. Previous authors (Beijbom et al., 2016) demonstrated promising use of a CNN to images acquired via a prototype underwater imager (Treibitz et al., 2015), to annotate and classify coral species to the genera/species level, using just a small set of images. Their trained CNN showed excellent (i.e., 90% +) and slightly greater accuracy than traditional machine learning methods (e.g., SVM) on their own data. In comparison, our current multispectral approach provides many optical channels from which a CNN or DL network could be fed based on acquired image spectral information as well as color, shape, edges, and other exploitable features. It could effectively be combined to CNN and DL image classification methodology similar to recently described work on macroalgae (Mahmood et al., 2020; D’Archino et al., 2021) and coral (Raphael et al., 2020). While the overall scale and tools used are different, similar spectral response generation and CNN or DL classification methods have been used to successfully image and classify microalgae in flow cytometry conditions (Deglint et al., 2019).
4.3 Evaluating possible imaging-related classification artefacts
In looking closer at the PCA plots of both macroalgae and corals, the fact that some of the data points for different clusters are spread out can represent variability in spectral response in these biological substrates. This variability can also be due to a number of factors related to imaging itself, such as 1) uneven detector response across the image (i.e., needs proper correction and validation, as shown earlier); 2) pixel size/resolution—fluorescence signal variability between pixels of a same specimen); 3) segmentation errors where pixels are attributed a class but are of another (e.g., coral underneath algae); 4) coral attachment substrate. The 3rd factor, pixel resolution, is a factor that can be adjusted during post-processing and is important in ML classification accuracy (Caras et al., 2017). Higher resolution has the potential to decrease classification efficiency when inter-pixel signal variability is high and individual substrate patch (e.g., one species) is much larger that the image pixel.
Classification error causes can be summed as the following: 1) overlapping spectral response characteristics, 2) more highly irregular macroalgae border/contour, 3) slight misalignment between laser beams of different color wavelength can cause error in predicting substrate type at locations where substrate is irregular/complex in contour, and 4) imaging platform movement during imaging (not the case here). These problems could be alleviated through the following methods in an improved system by 1) increasing the number of excitation sources to attempt generation of more spectral responses (i.e., fluorescence peaks). It becomes however increasingly difficult to orchestrate the data acquisition process since it is still necessary to differentiate between an emission from one excitation wavelength versus that of another; 2) increasing the total number of independent detectors and associated emission filters to generate more spectral emission bands. Spectral response signatures composed of many data points on the same “Fluorescence Intensity x Wavelength (nm) curve” could provide improved classification; 3) improving image contour pixel data pre-processing to facilitate segmentation (e.g., averaging by proximity analysis); 4) performing a more precise alignment of the optical system to obtain optimally aligned light path for all excitation sources; and 5) using an adequate Inertial Motion Unit (IMU) sensor capable of recording movement of the imaging platform and allowing 2D and/or 3D positional corrections in the final data.
4.4 Perspective
In our study and in general, red macroalgae are more easily identified from green and brown by fluorescence near 580 nm, while the two latter groups show a somewhat similar fluorescence response near 685 nm but greens responding more strongly. Methods to isolate these two latter groups from one another may however not be as straightforward as hoped, since laser wavelengths optimal for differentiation may currently be difficult or impractical to come by for underwater imaging. While 355 nm and 532 nm pulsed lasers can provide excellent beam quality, power and pulse repetition rate (in lidar), other lasers in the 400–500 nm range require a compromise between pulse repetition rate (e.g., from CW to 10 kHz), power and beam quality. Differential reflectance measurements may be promising for better results in differentiating algal color types by working with reflectance signatures through the simultaneous spectral “interrogation” of two excitation wavelengths (Rehm et al. 2018). The latter must be selected for optimizing the differentiability in macroalgal responses, but can be limiting at the time of this writing since pulsable lasers in the ranges of 450 nm–532 nm are limited in power (e.g., 473 nm), except 532 nm (i.e., doubled Nd:YAG). However, a pulsed 355 nm (i.e., tripled Nd:YAG) laser may provide means to differentiate spectral response by these means and be integrated to a 532 nm system. Continuous wave lasers could also be used for this purpose, in tandem with pulsed lasers (lidar), the former providing a better coverage of spectral excitation range in the PAR spectral range for macroalgae and coral. Otherwise, our results show that certain coral genera or species can also potentially be differentiated by their fluorescence response. The spectral range from which they may be differentiated by their fluorescence response is larger than macroalgae (e.g., see Mazel, 1995) and could provide multiple ways to differentiate groups based on their photoresponse. In related context, ongoing research on underwater laser imaging methods has in part been directed towards reducing the effects of scattering within coastal environments, for example, via laser pulse temporal modulation (Mullen et al., 2013) and experimenting with synchronous scan and bistatic configurations (Caimi and Dalgleish, 2013) (and see review by Dalgleish et al., 2013). By such, the multispectral reflectance and fluorescence detection, imaging and classification methods presented in our work could eventually be incorporated into one of these alternative laser imaging designs.
Spectral classification applied to underwater multispectral laser serial imaging of macroalgae and coral is possible to a degree through methods shown in our study. While other hyperspectral imaging methods could increase the potential number of exploitable spectral bands for spectral response signature characterization specific to color types and some genera in corals, laser serial imaging methods may offer better compromise relating to the effective distance to target as well as for working in more highly scattering and absorptive waters. This is a critical factor in developing imaging instruments for deployment on autonomous platforms such as underwater AUVs, where obstacle and hazard avoidance success should increase at longer working distances. While laser emitter limitations relating to wavelength, power and eventually the possibility to pulsate at high frequency remain important factors in generation of spectral response, due to biological constraints related to light absorption profile in photosynthetic substrates, detector architecture may be built to resemble hyperspectral sensors more closely. For example, one could assemble a multisensory array using many narrow wavelength emission filters for as many separate detectors. We may thus improve our acquisition of color group or genera specific variations in spectral response in differing biological substrates.
Since light travel in water depends on optical conditions, where Inherent Optical Properties (IPOs) such as absorption and scattering are function of wavelength, imaging range and fluorescence detection can be greatly influenced by these factors. While imaging conditions were done with success at 2.3 m and in “clear” Jerlov IA type water, we estimate that some fluorescence detection and imaging could have been extended (i.e., 3–5 m) in equally clear waters based on water IOPs, and potentially greater range with the use of lidar (pulse-gated laser line scan) (Dalgleish et al., 2009). The range could be augmented by integrating higher power CW lasers, bringing additional light to the target substrates (i.e., imaging via reflectance) and potentially generating a stronger fluorescence response. This should be optimized, especially since fluorescence emitted at higher wavelengths (e.g., from chlorophyll at 685 nm), used in detecting and classifying macroalgae and coral, will be more likely absorbed when imaging at greater range (Jerlov, 1976; Mobley, 1994b). These constraints place certain limitations on fluorescence-based underwater imaging, to the benefit of potentially acquiring higher resolution data while working at a closer range. Further, while other detection and classification methods relying on photo or video acquisition, or hyperspectral acquisition, will also be limited in more turbid waters, CW or pulsed laser imaging could provide an advantage.
To efficiently use the methods described in this work, prior knowledge of study site specifics on target substrates should aid in improving detection and classification success and allow a more thorough understanding of the results. For example, since brown macroalgae such as Arctic and cold-temperate kelp species Saccharina latissima, Agarum clathratum, Alaria esculenta, and other stand forming species across the globe (e.g., Australia, New Zealand, California) very often occur in dense and often tall (e.g., 1–5 m or more) monospecific stands, differentiation from green macroalgae may not lead to lower classification accuracy since the latter are generally more of understory or sub-canopy occurrence. In this sense, green macroalgae would be under a canopy of taller brown species. This type of information is often available in the form of local knowledge, research centers and local dive shops. Moreover, the detector design can include interchangeable filter components for maximal adaptability in specific situations. Additional sensor types such as still cameras, high-resolution photos and video can be added as secondary imaging systems to confirm detections and classification and used as additional information layers for classification purposes using images in CNNs and Deep Learning.
Data availability statement
The raw data supporting the conclusion of this article will be made available by the authors, without undue reservation.
Author contributions
MH and FD: Conception and design of the study. MH and DB: Programming aspects of database management and data processing. MH: Manuscript writing. MP and PA: Provided funding for MH. All authors contributed to the article and approved the submitted version.
Funding
This research was in part funded by Sentinel North to PA and MP and the Link Foundation, the latter providing a major portion of funding for the Harbor Branch Oceanographic Institute Summer Intern Program for which aspects related to lab experiments were made possible. PhD Scholarship to MH was paid by Sentinel North and partly by ArcticNet.
Acknowledgments
Special thanks to the Sentinel North program of Université Laval, made possible, in part, thanks to funding from the Canada First Research Excellence Fund. Also, an appreciation to Harbor Branch Oeanographic Institute (HBOI) at Florida Atlantic University's Ocean Optics team for support (B. Ramos, C. Strait, A. Tonizzo), the Link foundation at HBOI, and helpful administrative personnel for making the project possible through the summer internship program, and V. Sommers at ORA, Fort Pier, FL.
Conflict of interest
The authors declare that the research was conducted in the absence of any commercial or financial relationships that could be construed as a potential conflict of interest.
Publisher’s note
All claims expressed in this article are solely those of the authors and do not necessarily represent those of their affiliated organizations, or those of the publisher, the editors and the reviewers. Any product that may be evaluated in this article, or claim that may be made by its manufacturer, is not guaranteed or endorsed by the publisher.
Supplementary material
The Supplementary Material for this article can be found online at: https://www.frontiersin.org/articles/10.3389/frsen.2023.1135501/full#supplementary-material
References
Al-Habahbeh, A. K., Kortsch, S., Bluhm, B. A., Beuchel, F., Gulliksen, B., Ballantine, C., et al. (2020). Arctic coastal benthos long-term responses to perturbations under climate warming: Climate change impact on Arctic benthos. Philosophical Trans. R. Soc. A Math. Phys. Eng. Sci. 378, 20190355. doi:10.1098/rsta.2019.0355
Alieva, N. O., Konzen, K. A., Field, S. F., Meleshkevitch, E. A., Hunt, M. E., Beltran-Ramirez, V., et al. (2008). Diversity and evolution of coral fluorescent proteins. PLoS One 3, e2680. doi:10.1371/journal.pone.0002680
Baird, A. H., Salih, A., and Trevor-Jones, A. (2006). Fluorescence census techniques for the early detection of coral recruits. Coral Reefs 25, 73–76. doi:10.1007/s00338-005-0072-7
Bates, S. S., and Craigie, J. S. (1988). Fluorescence induction in the macroalgae chondrus crispus (rhodophyceae) and ulva sp. (chlorophyceae). Mar. Biol. 98, 457–466. doi:10.1007/BF00391536
Beijbom, O., Treibitz, T., Kline, D. I., Eyal, G., Khen, A., Neal, B., et al. (2016). Improving automated annotation of benthic survey images using wide-band fluorescence. Sci. Rep. 6, 23166–23211. doi:10.1038/srep23166
Ben-Zvi, O., Wangpraseurt, D., Bronstein, O., Eyal, G., and Loya, Y. (2021). Photosynthesis and bio-optical properties of fluorescent mesophotic corals. Front. Mar. Sci. 8, 1–12. doi:10.3389/fmars.2021.651601
Bertocci, I., Araújo, R., Oliveira, P., and Sousa-Pinto, I. (2015). Review: Potential effects of kelp species on local fisheries. J. Appl. Ecol. 52, 1216–1226. doi:10.1111/1365-2664.12483
Box, J. E., Colgan, W. T., Christensen, T. R., Schmidt, N. M., Lund, M., Parmentier, F. J. W., et al. (2019). Key indicators of arctic climate change: 1971-2017. Environ. Res. Lett. 14, 045010. doi:10.1088/1748-9326/aafc1b
Breiman, L., Friedman, J. H., Olshen, R. A., and Stone, C. J. (2017). Classification and regression trees. Boca Raton: Routledge. doi:10.1201/9781315139470
Caimi, F. M., and Dalgleish, F. R. (2013). “Subsea laser scanning and imaging systems,” in Subsea Optics and imaging. Editors J. Watson, and O. Zielinski (Cambridge: Woodhead Publishing Limited), 327–352. doi:10.1533/9780857093523.3.327
Caras, T., Hedley, J., and Karnieli, A. (2017). Implications of sensor design for coral reef detection: Upscaling ground hyperspectral imagery in spatial and spectral scales. Int. J. Appl. Earth Observation Geoinformation 63, 68–77. doi:10.1016/j.jag.2017.07.009
Carricart-Ganivet, J. P., Cabanillas-Terán, N., Cruz-Ortega, I., and Blanchon, P. (2012). Sensitivity of calcification to thermal stress varies among genera of massive reef-building corals. PLoS One 7, e32859. doi:10.1371/journal.pone.0032859
Chao Rodríguez, Y., Domínguez Gómez, J. A., Sánchez-Carnero, N., and Rodríguez-Pérez, D. (2017). A comparison of spectral macroalgae taxa separability methods using an extensive spectral library. Algal Res. 26, 463–473. doi:10.1016/j.algal.2017.04.021
Chennu, A., Färber, P., De’ath, G., de Beer, D., and Fabricius, K. E. (2017). A diver-operated hyperspectral imaging and topographic surveying system for automated mapping of benthic habitats. Sci. Rep. 7, 7122–7212. doi:10.1038/s41598-017-07337-y
Churnside, J. H. (2015). Bio-optical model to describe remote sensing signals from a stratified ocean. J. Appl. Remote Sens. 9, 095989. doi:10.1117/1.jrs.9.095989
Dalgleish, F. R., Caimi, F. M., Britton, W. B., and Andren, C. F. (2009). Improved LLS imaging performance in scattering-dominant waters. , 7317, 73170E. doi:10.1117/12.820836
Dalgleish, F. R., Vuorenkoski, A. K., and Ouyang, B. (2013). Extended-range undersea laser imaging: Current research status and a glimpse at future technologies. Mar. Technol. Soc. J. 47, 128–147. doi:10.4031/MTSJ.47.5.16
D’Archino, R., Schimel, A., Peat, C., and Anderson, T. (2021). Automated detection of large brown macroalgae using machine learning algorithms-a case study from Island Bay. Wellington, New Zealand: Publications Logistics Officer Ministry for Primary Industries.
Deglint, J. L., Jin, C., and Wong, A. (2019). Investigating the automatic classification of algae using the spectral and morphological characteristics via deep residual learning. Lect. Notes Comput. Sci. Incl. Subser. Lect. Notes Artif. Intell. Lect. Notes Bioinforma. 11663 LNCS, 269, 280. doi:10.1007/978-3-030-27272-2_23
den Haan, J., Huisman, J., Dekker, F., ten Brinke, J. L., Ford, A. K., van Ooijen, J., et al. (2013). Fast detection of nutrient limitation in macroalgae and seagrass with nutrient-induced fluorescence. PLoS One 8. 1, doi:10.1371/journal.pone.0068834
Dierssen, H. M., Chlus, A., and Russell, B. (2015). Hyperspectral discrimination of floating mats of seagrass wrack and the macroalgae Sargassum in coastal waters of Greater Florida Bay using airborne remote sensing. Remote Sens. Environ. 167, 247–258. doi:10.1016/j.rse.2015.01.027
Douay, F., Verpoorter, C., Duong, G., Spilmont, N., and Gevaert, F. (2022). New hyperspectral procedure to discriminate intertidal macroalgae. Remote Sens. (Basel) 14, 346. doi:10.3390/rs14020346
Enríquez, S., and Borowitzka, M. A. (2010). “The use of the fluorescence signal in studies of seagrasses and macroalgae,” in Chlorophyll a fluorescence in aquatic Sciences: Methods and applications (Netherlands: Springer Netherlands), 187–208. doi:10.1007/978-90-481-9268-7_9
Eyal, G., Wiedenmann, J., Grinblat, M., D’Angelo, C., Kramarsky-Winter, E., Treibitz, T., et al. (2015). Spectral diversity and regulation of coral fluorescence in a mesophotic reef habitat in the Red Sea. PLoS One 10, 01286977–e128719. doi:10.1371/journal.pone.0128697
Gameiro, C., Utkin, A. B., and Cartaxana, P. (2015). Characterisation of estuarine intertidal macroalgae by laser-induced fluorescence. Estuar. Coast Shelf Sci. 167, 119–124. doi:10.1016/j.ecss.2015.11.010
Haxo, F. T., and Blinks, L. R. (1950). Photosynthetic action spectra of macroalgae. J. General Physiology 33, 389–422. doi:10.1085/jgp.33.4.389
He, Q., and Silliman, B. R. (2019). Climate change, human impacts, and coastal ecosystems in the anthropocene. Curr. Biol. 29, R1021–R1035. doi:10.1016/j.cub.2019.08.042
Hieronymi, M., Müller, D., and Doerffer, R. (2017). The olci neural network swarm (ONNS): A bio-geo-optical algorithm for open ocean and coastal waters. Front. Mar. Sci. 4, 1. doi:10.3389/fmars.2017.00140
Hochberg, E. J., Atkinson, M. J., Apprill, A., and Andréfouët, S. (2004). Spectral reflectance of coral. Coral Reefs 23, 84–95. doi:10.1007/s00338-003-0350-1
Hoegh-Guldberg, O. (1999). Climate change, coral bleaching and the future of the world’s coral reefs. Mar. Freshw. Res. 50, 839–866. doi:10.1071/MF99078
Hoegh-Guldberg, O., Poloczanska, E. S., Skirving, W., and Dove, S. (2017). Coral reef ecosystems under climate change and ocean acidification. Front. Mar. Sci. 4. doi:10.3389/fmars.2017.00158
Hudson, B., Overeem, I., McGrath, D., Syvitski, J. P. M., Mikkelsen, A., and Hasholt, B. (2014). MODIS observed increase in duration and spatial extent of sediment plumes in Greenland fjords. Cryosphere 8, 1161–1176. doi:10.5194/tc-8-1161-2014
Huot, M., Dalgleish, F., Rehm, E., Piché, M., and Archambault, P. (2022). Underwater multispectral laser serial imager for spectral differentiation of macroalgal and coral substrates. Remote Sens. (Basel) 14, 3105. doi:10.3390/rs14133105
Huot, M., Rehm, E., Dalgleish, F. R., Piché, M., Lambert-Girard, S., and Archambault, P. (2018). “Characterizing fluorescence and reflectance properties of Arctic macroalgae as future LiDAR targets,” in Ocean sensing and monitoring X. Editors W. Will” Hou, and R. A. Arnone (USA: SPIE), 10. doi:10.1117/12.2310047
Jack Pan, B., Vernet, M., Reynolds, R. A., and Greg Mitchell, B. (2019). The optical and biological properties of glacial meltwater in an Antarctic fjord. PLoS One 14, 1–30. doi:10.1371/journal.pone.0211107
Jansen, E., Christensen, J. H., Dokken, T., Nisancioglu, K. H., Vinther, B. M., Capron, E., et al. (2020). Past perspectives on the present era of abrupt Arctic climate change. Nat. Clim. Chang. 10, 714–721. doi:10.1038/s41558-020-0860-7
Jerlov, N. G. (1976). Marine Optics. 2nd edition. Copenhagen: Elsevier Scientific Publishing Company.
Jonasz, M., and Fournier, G. R. (2007). Light scattering by particles in water: Theoretical and experimental foundations. Amsterdam, Netherlands: Academic Press. doi:10.1016/B978-0-12-388751-1.X5000-5
Kieleck, C., Bousquet, B., le Brun, G., Cariou, J., and Lotrian, J. (2001). Laser induced fluorescence imaging: Application to groups of macroalgae identification. J. Phys. D. Appl. Phys. 34, 2561–2571. doi:10.1088/0022-3727/34/16/324
Kirk, J. T. O. (2011). Light and photosynthesis in aquatic ecosystems. third edition. Cambridge, United Kindom: Cambridge University Press. doi:10.1017/CBO9781139168212
Kotta, J., Remm, K., Vahtmäe, E., Kutser, T., and Orav-Kotta, H. (2014). In-air spectral signatures of the Baltic Sea macrophytes and their statistical separability. J. Appl. Remote Sens. 8, 083634. doi:10.1117/1.jrs.8.083634
Krause-Jensen, D., Archambault, P., Assis, J., Bartsch, I., Bischof, K., Filbee-Dexter, K., et al. (2020). Imprint of climate change on pan-arctic marine vegetation. Front. Mar. Sci. 7, 1. doi:10.3389/fmars.2020.617324
Krause-Jensen, D., and Duarte, C. M. (2016). Substantial role of macroalgae in marine carbon sequestration. Nat. Geosci. 9, 737–742. doi:10.1038/ngeo2790
Kutser, T., Vahtmäe, E., and Metsamaa, L. (2006). Spectral library of macroalgae and benthic substrates in Estonian coastal waters. Proc. Est. Acad. Sci. Biol. Ecol. 55, 329–340. doi:10.3176/biol.ecol.2006.4.05
Lähteenmäki-Uutela, A., Rahikainen, M., Camarena-Gómez, M. T., Piiparinen, J., Spilling, K., and Yang, B. (2021). European Union legislation on macroalgae products. Aquac. Int. 29, 487–509. doi:10.1007/s10499-020-00633-x
Lüning, K., and Dring, M. J. (1985). Action spectra and spectral quantum yield of photosynthesis in marine macroalgae with thin and thick thalli. Mar. Biol. 87, 119–129. doi:10.1007/BF00539419
Mabit, R., Araújo, C. A. S., Singh, R. K., and Bélanger, S. (2022). Empirical remote sensing algorithms to retrieve SPM and CDOM in québec coastal waters. Front. Remote Sens. 3, 1. doi:10.3389/frsen.2022.834908
Mahmood, A., Ospina, A. G., Bennamoun, M., An, S., Sohel, F., Boussaid, F., et al. (2020). Automatic hierarchical classification of kelps using deep residual features. Sensors Switz. 20, 447. doi:10.3390/s20020447
Maxwell, A. E., Warner, T. A., and Fang, F. (2018). Implementation of machine-learning classification in remote sensing: An applied review. Int. J. Remote Sens. 39, 2784–2817. doi:10.1080/01431161.2018.1433343
Mazel, C. H. (1997). <title>Coral fluorescence characteristics: Excitation/emmission spectra, fluorescence efficiences, and contribution to apparent reflectance</title>. Ocean. Opt. XIII 2963, 240–245. doi:10.1117/12.266450
Mazel, C. H. (1995). Spectral measurements of fluorescence emission in Caribbean cnidarians. Mar. Ecol. Prog. Ser. 120, 185–191. doi:10.3354/meps120185
Mazel, C. H., Strand, M. P., Lesser, M. P., Crosby, M. P., Coles, B., and Nevis, A. J. (2003). High resolution determination of coral reef bottom cover from multispectral fluorescence laser line scan imagery. Limnol. Oceanogr. 48, 522–534. doi:10.4319/lo.2003.48.1_part_2.0522
Mentaschi, L., Vousdoukas, M. I., Pekel, J. F., Voukouvalas, E., and Feyen, L. (2018). Global long-term observations of coastal erosion and accretion. Sci. Rep. 8, 12876–12911. doi:10.1038/s41598-018-30904-w
Mobley, C. D. (1994b). Ligth and water: Radiative transfer in natural waters San Diego: Academic Press.
Mullen, L., O’Connor, S., Cochenour, B., and Dalgleish, F. (2013). State-of-the-art tools for next-generation underwater optical imaging systems. Ocean Sens. Monit. V 8724, 872402. doi:10.1117/12.2018489
Olmedo-Masat, O. M., Paula Raffo, M., Rodríguez-Pérez, D., Arijón, M., and Sánchez-Carnero, N. (2020). How far can we classify macroalgae remotely? An example using a new spectral library of species from the south west atlantic (argentine patagonia). Remote Sens. (Basel) 12, 1–33. doi:10.3390/rs12233870
Oppelt, N. (2012). Hyperspectral classification approaches for intertidal macroalgae habitat mapping: A case study in heligoland. Opt. Eng. 51, 111703. doi:10.1117/1.OE.51.11.111703
Pal, M., and Mather, P. M. (2005). Support vector machines for classification in remote sensing. Int. J. Remote Sens. 26, 1007–1011. doi:10.1080/01431160512331314083
QGIS Development Team (2020). QGIS geographic information system. Open source geospatial foundation project. Available at: http://qgis.osgeo.org. QGIS.org.
Raphael, A., Dubinsky, Z., Iluz, D., Benichou, J. I. C., and Netanyahu, N. S. (2020). Deep neural network recognition of shallow water corals in the Gulf of Eilat (Aqaba). Sci. Rep. 10, 12959. doi:10.1038/s41598-020-69201-w
Rehm, E., Dalgleish, F., Huot, M., Lagunas-Morales, J., Lambert-Girard, S., Matteoli, S., et al. (2018),“Comparing fluorescent and differential absorption LiDAR techniques for detecting algal biomass with applications to Arctic substrates“, Proc. SPIE 10631, Ocean Sensing and Monitoring X. doi:10.1117/12.2302381
Rossiter, T., Furey, T., McCarthy, T., and Stengel, D. B. (2020a). Application of multiplatform, multispectral remote sensors for mapping intertidal macroalgae: A comparative approach. Aquat. Conserv. 30, 1595–1612. doi:10.1002/aqc.3357
Rossiter, T., Furey, T., McCarthy, T., and Stengel, D. B. (2020b). UAV-mounted hyperspectral mapping of intertidal macroalgae. Estuar. Coast Shelf Sci. 242, 106789. doi:10.1016/j.ecss.2020.106789
Roth, M. S. (2014). The engine of the reef: Photobiology of the coral-algal symbiosis. Front. Microbiol. 5, 422–22. doi:10.3389/fmicb.2014.00422
Sang, V. T., Dat, T. T. H., Vinh, L. B., Cuong, L. C. V., Oanh, P. T. T., Ha, H., et al. (2019). Coral and coral-associated microorganisms: A prolific source of potential bioactive natural products. Mar. Drugs 17, 468. doi:10.3390/md17080468
Sasano, M., Imasato, M., Yamano, H., and Oguma, H. (2016). Development of a regional coral observation method by a fluorescence imaging LIDAR installed in a towable buoy. Remote Sens. (Basel) 8, 48. doi:10.3390/rs8010048
Scherrer, K. J. N., Kortsch, S., Varpe, Ø., Weyhenmeyer, G. A., Gulliksen, B., and Primicerio, R. (2018). Mechanistic model identifies increasing light availability due to sea ice reductions as cause for increasing macroalgae cover in the Arctic. Limnol. Oceanogr. 64, 330–341. doi:10.1002/lno.11043
Schubert, N., Brown, D., and Rossi, S. (2016). “Symbiotic versus non-symbiotic octocorals: Physiological and ecological implications,” in Marine animal forests (Germany: Springer International Publishing), 1–32. doi:10.1007/978-3-319-17001-5_54-1
Smale, D. A., Burrows, M. T., Moore, P., Connor, N. O., and Hawkins, S. J. (2013). Threats and knowledge gaps for ecosystem services provided by kelp forests: A northeast atlantic perspective. Ecol. Eval. 2090, 1. doi:10.1002/ece3.774
Solonenko, M. G., and Mobley, C. D. (2015). Inherent optical properties of Jerlov water types. Appl. Opt. 54, 5392. doi:10.1364/ao.54.005392
Suggett, D. J. (2010). Chlorophyll a fluorescence in aquatic Sciences: Methods and applications. Dordrecht, Netherlands: Springer. doi:10.1007/978-90-481-9268-7
Teagle, H., Hawkins, S. J., Moore, P. J., and Smale, D. A. (2017). The role of kelp species as biogenic habitat formers in coastal marine ecosystems. J. Exp. Mar. Biol. Ecol. 492, 81–98. doi:10.1016/j.jembe.2017.01.017
Topinka, J. A., Korjeff Bellows, W., and Yentsch, C. S. (1990). Characterization of marine macroalgae by fluorescence signatures. Int. J. Remote Sens. 11, 2329–2335. doi:10.1080/01431169008955178
Treibitz, T., Neal, B. P., Kline, D. I., Beijbom, O., Roberts, P. L. D., Mitchell, B. G., et al. (2015). Wide field-of-view fluorescence imaging of coral reefs. Sci. Rep. 5, 7694–7699. doi:10.1038/srep07694
van den Hoek, C., Mann, D. G., and Jahns, H. M. (1995), Algae: An introduction to phycology. Cambridge: Cambridge University Press.
Vapnik, V. N. (1995). The nature of statistical learning theory New York, NY: Sprigner. doi:10.1007/978-1-4757-2440-0
Wild, C., Hoegh-Guldberg, O., Naumann, M. S., Colombo-Pallotta, M. F., Ateweberhan, M., Fitt, W. K., et al. (2011). Climate change impedes scleractinian corals as primary reef ecosystem engineers. Mar. Freshw. Res. 62, 205–215. doi:10.1071/MF10254
Yamashita, H., Koike, K., Shinzato, C., Jimbo, M., and Suzuki, G. (2021). Can Acropora tenuis larvae attract native Symbiodiniaceae cells by green fluorescence at the initial establishment of symbiosis? PLoS One 16, e0252514. doi:10.1371/journal.pone.0252514
Keywords: laser serial imaging, multispectral, macroalgae, coral, machine learning, underwater, fluorescence, classification
Citation: Huot M, Dalgleish F, Beauchesne D, Piché M and Archambault P (2023) Machine learning for underwater laser detection and differentiation of macroalgae and coral. Front. Remote Sens. 4:1135501. doi: 10.3389/frsen.2023.1135501
Received: 01 January 2023; Accepted: 16 May 2023;
Published: 01 June 2023.
Edited by:
Peng Chen, Ministry of Natural Resources, ChinaReviewed by:
Dingtian Yang, South China Sea Institute of Oceanology (CAS), ChinaJiulin Shi, Nanchang Hangkong University, China
Copyright © 2023 Huot, Dalgleish, Beauchesne, Piché and Archambault. This is an open-access article distributed under the terms of the Creative Commons Attribution License (CC BY). The use, distribution or reproduction in other forums is permitted, provided the original author(s) and the copyright owner(s) are credited and that the original publication in this journal is cited, in accordance with accepted academic practice. No use, distribution or reproduction is permitted which does not comply with these terms.
*Correspondence: Matthieu Huot, matthieu.huot.1@ulaval.ca; Philippe Archambault, philippe.archambault@bio.ulaval.ca