- 1Remote Sensing Graduate Program, Federal University of Rio Grande do Sul, Porto Alegre, Brazil
- 2Engineering, Modelling, and Applied Social Sciences Center, Federal University of ABC, Santo André, Brazil
- 3Earth System Science Center, National Institute for Space Research, São Jose dos Campos, Brazil
Fire has been an intrinsic ecological component of the ecosystems, affecting the public, economic, and socio-cultural policies of human-nature interactions. Using fire over grassland vegetation is a traditional practice for livestock in the highland grasslands and has economic and environmental consequences that have not yet been understood. A better description of the spatio-temporal biomass burning patterns is needed to analyze the effects of creation and application in these areas. This study used remote sensing techniques based on Sentinel-2 data and machine learning algorithms to identify burning scars and compare them with a national fire collection database for the highland grasslands in the Atlantic Forest Biome in Brazil. The aim is to evaluate public management tools and legislation evolution during the 35 years of the time series analyzed. The results indicated that 12,285 ha of grasslands were converted to other uses, losing about 24% of their original formation, with 10% occurring after banned this practice in 2008. The burned areas classification using the Random Forest algorithm obtained an AUC = 0.9983. Divergences in the burned area’s extent and frequency were found between the municipality’s authorized license and those classified as burned. On average, only 43% of the burned area in the Parque Estadual do Tainhas and its buffer zone had an environmental permit in the last 5 years. This research’s results provide subsidies for revising and creating public policies and consequently help territorial management.
1 Introduction
Fire activity in Brazil is influenced by several factors, the result of complex and dynamic processes generated by interactions between climatic conditions, vegetation attributes, land use and land cover, dynamics between regeneration, productivity and ignition patterns (Fidelis et al., 2021; Pivello et al., 2021). Brazilian biomes have a long history of fire conflicts, as in the Atlantic Forest Biome (AFB), one of the most critical biodiversity hotspots in the world (Myers et al., 2000).
Currently, the AFB remnants are highly fragmented and restricted to highland grassland areas in southern Brazil, where there are mosaics of Araucaria (Brazilian pine) forests and grasslands linked to a fire events history (Pillar et al., 2009; Meireles and Shepherd, 2015).
In these areas, fire has been an intrinsic ecological component of the ecosystems, affecting the public, economic, social, and even cultural policies of human interactions with nature (Andrade et al., 2015).
In recent years, conflicts related to fire use have presented economic and environmental consequences that have not yet been clearly understood. The fire use on grassland vegetation in the northeastern Rio Grande do Sul (RS) state is a traditional practice for livestock (Carvalho and Andrades-Filho, 2019). However, it was prohibited by Law 9,519/1992 (Rio Grande do Sul, 1992). This has led to natural grassland area changes to simplified land uses, such as agriculture and forestry, leading to biodiversity loss and difficulties in recuperating degraded areas (Buisson et al., 2019). Subsequently, Law 13,931/2012, which amended Law 9,519/1992 (Rio Grande do Sul, 2012), attributes to the municipal government the power to authorize and supervise the use of fire as a grasslands management practice in areas that cannot be mechanized or as a form of phytosanitary treatment.
Therefore, a better description of biomass burning spatio-temporal pattern is needed to analyze the effects of fire legislation. So, the use of remote sensing derived products such as 1) the Modis product MCD64A1—Collection 6, with 500 m resolution at a global scale (Giglio et al., 2018); 2) the MapBiomas Fire—Collection 1, based on Landsat time series, with 30 m resolution for the Brazil territory (Alencar et al., 2022), and 3) the global forest loss by fire product, also at a spatial resolution of 30 m (Tyukavina et al., 2022) are consolidated as an essential data source by covering vast areas with a multitemporal and multispectral information.
In this study, to minimize these products limitations, such as the spatial resolution, we used Sentinel-2 data developed by the European Space Agency (ESA), which has four bands with 10 m, six with 20 m, and three bands with 60 m (ESA, 2022). Therefore, this study’s objective is to improve the spatial resolution of burned areas and compare them with the national MapBiomas Fire product to evaluate the fire use legislation over time in the highland grasslands in Atlantic Forest Biome in Brazil.
2 Materials and methods
2.1 Study area
This study was conducted in the conservation area denominate Parque Estadual do Tainhas (PET) and its buffer zone (BZ) located at latitude 29°5′15″S and longitude 50°22′4″W (Figure 1). The PET was established in 1975 to protect the grasslands and forests in the Tainhas river valley, inserted in the AFB.
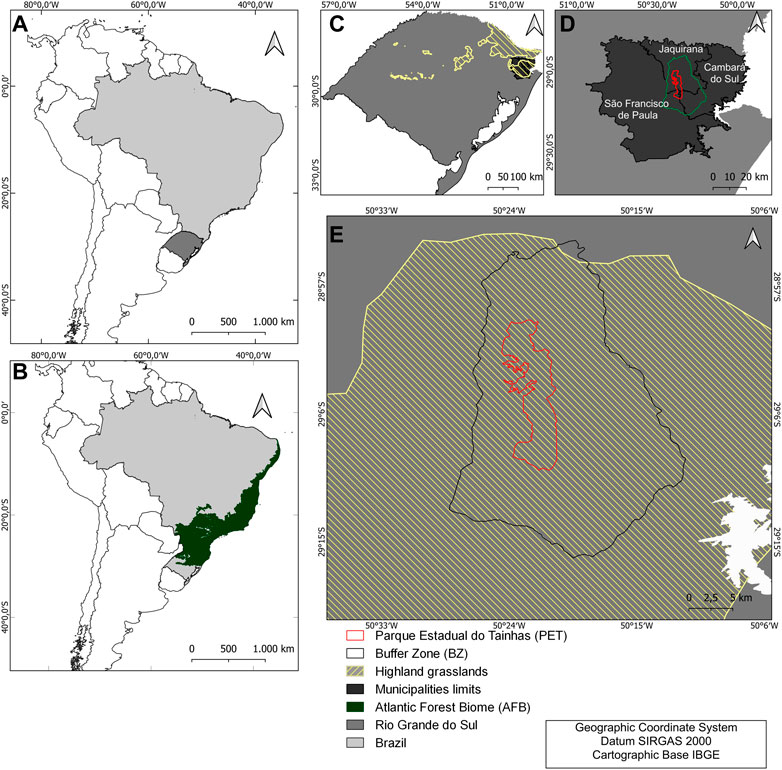
FIGURE 1. (A) Rio Grande do Sul location, (B) AFB location, (C) Highland grasslands distribution, (D) Location of the municipalities that make up PET, (E) Location of the PET and its BZ in relation to the highland grasslands.
The Park is formed by mosaics of forests with Araucaria pine, grasslands, and flooded areas (wetlands) with flatter terrain in the southern portion to valleys in the northern part (SEMA, 2008). It has an area of 6,654 ha with a BZ of approximately 60,000 ha, covering the municipalities of Jaquirana (69.8%), São Francisco de Paula (20.6%), and Cambará do Sul (9.6%) of the Park’s area. Around the PET, communities in its BZ depend on agriculture, forestry, and livestock and regularly use fire as a management tool (Bond-Buckup, 2008).
The PET is close to other protected areas, which favors the emergence of the biogeographic conditions that can contribute to the interconnection of these units through ecological corridors, helping preserve fauna and flora populations and maintain the ecosystem services balance in the region.
2.2 Burned areas classification in highland grasslands (BACHG)
The methodological approach for burned area detection used in this study is shown in the flowchart (Figure 2). We use Sentinel-2 MSI Multispectral Instrument, Level-2A data. The Level-2A product provides Bottom Of Atmosphere (BOA) imagery derived from the associated Level-1C products. The imagery used is available from the Google Earth Engine (GEE). This platform combines three tools (code editor, explorer, and client libraries) for users to perform geospatial analysis in the cloud (Adagbasa, Adelabu, and Okello, 2020). We select images from 2018 to 2022, before the start of the fire season, from January 1 to April 1, denominating as “pre-fire” images, while the post-fire images were selected from July 15 to September 15 of each year, when the “burning window” allowed by municipal legislation was established. A cloud mask was applied. First, the function defines two bitmask values for clouds and cirrus (bit 10 and bit 11, respectively) and selects the image’s pixel quality band (QA). Then, the function creates a mask that filters out all the pixels where the cloud and cirrus bits are equal to zero, indicating clear conditions.
We used the GEE to collect spectral signatures of the burned and unburned areas, which served as samples for the model classification. In addition, we used data collected from the field in the year 2020 as a reference for sampling in this year’s post-fire images. This effort resulted in 7,133 sampled pixels, manually collected as small polygons from burned areas (2,295 sampled pixels) and the unburned regions (4,838 sampled pixels).
For training, we used the shortwave infrared (SWIR) and near-infrared (NIR) bands and the result of the Normalized Burn Ratio spectral index (ΔNBR) calculation.
The NBR is a ratio of the NIR to the SWIR region, developed to identify post-fire burned areas and provide a quantitative measure of burn severity (Key and Benson, 2006). The NBR is calculated by the pre- and post-fire difference (denoted as ΔNBR) using Eqs. 1, 2.
The algorithm used for our classification was the Random Forest (RF) (Breiman, 2001; Goehry et al., 2021), which is an ensemble algorithm operated by building multiple decision trees in a training session and assigning the target class by majority vote (PAL, 2005). In this study, we used the RandomForest function in the GEE library. The parameters used were: number0fTrees (20), variablesPerSplit (null), minLeafPopulation (1), bagFraction (0.5), maxNodes (null), and seed (0). After classification, we applied a spatial filter to remove noise and fill in gaps, where burned areas smaller than or equal to 1 ha (5 × 5 pixels) were removed, and, in the same way, internal spaces were filled as burned. The spatial filter selected was the Manhattan Kernel, which generates a distance kernel based on rectilinear distance (city-block). Reduction is performed by calculating the mode (most common value) of the pixel values in a neighborhood defined by the specified kernel (or window). The filter size was determined considering the difference in the spatial resolution of the Landsat 8 satellite, which was used for the MAPBIOMAS product methodology proposed for exclusion. Finally, the Receiver Operating Characteristic (ROC curve) was calculated to measure and compare the binary classification model’s performance.
2.3 Temporal analysis of burned areas
To evaluate the management tools available in conjunction with the classification data generated, we used data on the annual area burned by MapBiomas Fire—Collection 1.0 (Alencar et al., 2022) and the land use and land cover areas classified as grassland from 1985 to 2020 available in MapBiomas Collection 7.0 (Souza et al., 2020). In addition, we also used the extent area authorized for burning by Jaquirana, São Francisco de Paula, and Cambará do Sul municipalities located within PET and its BZ.
The annual burned areas stationarity trend covered by grassland was checked using the Augmented Dickey-Fuller (ADF) test. The ADF test is an “augmented” version of the Dicker-Fuller test. The ADF test expands the test equation to include high-order regression processes in the model.
Afterward, the trend was verified through time series decomposition to verify the seasonality and the residuals. Results are obtained by first estimating the trend by applying a convolution filter to the data. Therefore, the trend is removed from the series, and the average of this unbiased series for each period is the seasonal component returned (McKinney, Perktold and Seabold, 2011).
3 Results
3.1 Reconstructing the history of fire use
The burnt areas and the grasslands from 1985 to 2020 in the PET and its BZ are shown in (Figure 3). In this figure is highlighted in vertical dotted lines, the primary legislation, which is also presented in (Table 1) together with the grasslands and the total burned area values. This analysis reveals an increasing trend for the burned area, while there is a decreasing trend for grassland in the PET.
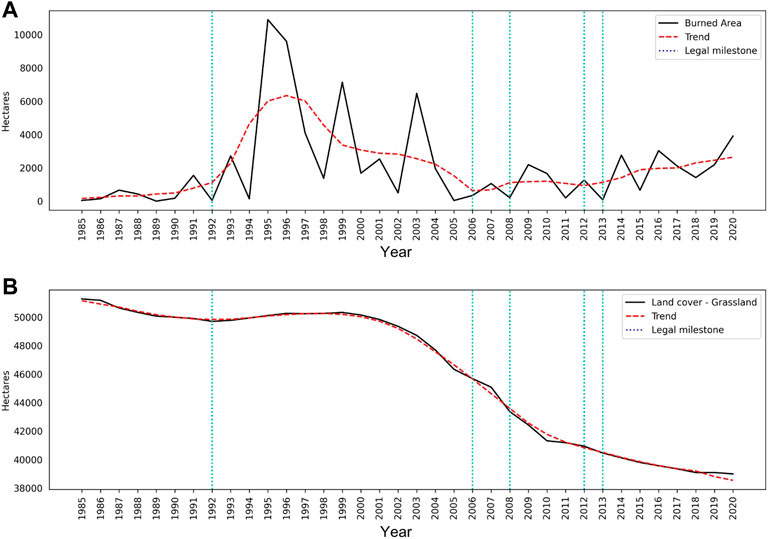
FIGURE 3. (A) Burned area accordingly MapBiomas Fire—Collection 1.0, and (B) Grassland accordingly MapBiomas—Collection 7.0, both in the PET and its BZ from 1985–2020.
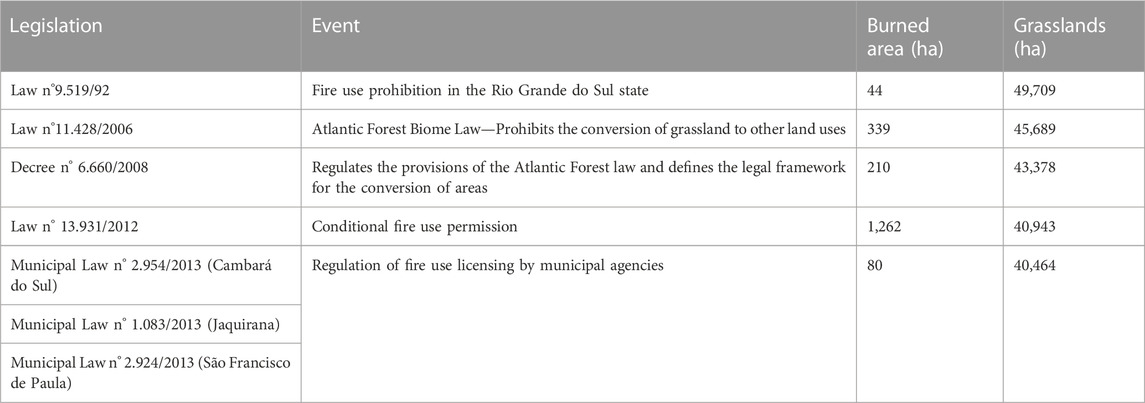
TABLE 1. Relationship of burnt areas (hectares per year) mapped and permissions of environmental permits.
We can observe that the burnt areas remained low after the fire use prohibition in 1992. However, this pattern was not established for a long time, and the highest values for the time series analyzed occurred in 1995 and 1996, with about ten thousand hectares burnt each year.
The most significant burned areas reduction occurred in 2002, reaching the lowest value in 2005, with only 35 ha. By observing the trend curves of burnt area and area occupied by grassland formation, the curve’s decline in the same years is noticed.
In 2002, the area corresponding to grassland was 49,359 ha, while in 2005, it was 46,345 ha. Therefore, the PET and its BZ lost more than 3,000 ha in only 3 years. These years precede the AFB Law creation (Brasil, 2006) and its regulation decree (Brasil, 2008), prohibiting new conversions of areas with native vegetation in the biome.
During the 35 years of the analyzed time series, 12.285 ha of grassland areas were converted to other uses, losing about 24% of their original formation, with 10% occurring after the AFB decree banned this practice in 2008 (Brasil, 2008).
Finally, concerning the burned areas only when municipalities begin to legislate about the use of fire, in 2013, a trend definition and a biannual frequency can be observed, as provided by several municipal legislation (Municipio de Cambará do Sul, 2013; Municipio de Jaquirana, 2013; Municipio de São Francisco de Paula, 2013).
3.2 Burned area and frequency of occurrence
The burned areas classification with the RF algorithm obtained an AUC = 0.9983 (Figure 4). With a visual inspection, the scar’s demarcation can be identified when comparing the pre-fire and post-fire images (Figure 5).
The results show that larger areas were burned for all years than those allowed by the municipalities’ environmental permits. In addition, larger burned areas were found in our classification than those presented by the MapBiomas Fire collection, as can be seen in (Table 2).

TABLE 2. Comparison of burned areas (ha) authorized by the municipality between mapped by mapbiomas and BACHG.
The licenses issued correspond to only 48.09% (2018), 33.62% (2019), 23.55% (2020), 69.43% (2021), and 43.54% (2022) of the area identified as burned in our classification. For the three available years of the analyzed period from the MapBiomas collection, they represent only 26.34% (2018), 29.69% (2019), and 49.74% (2020) of the burned areas.
The Mapbiomas burned areas that coincide with our classification represent only 21% of the total classified area on average (Figure 6). The MapBiomas burned omission areas are formed by scars smaller than 10 ha or with sites that present a non-continuous formation with gaps of 1 ha.
In addition to the burning extent, the frequency of areas affected by the fire was verified, and about 28% of the PET and its BZ were burned at least once, as shown in (Figure 7). Notably, according to the legislation, the areas could present a burning frequency of 2–3 times for 5 years. However, the results showed that 353 ha were burned 4 to 5 times, exceeding the legal requirements for this period. These areas are within the Jaquirana municipality territory inside the PET or near its boundaries.
4 Discussion
4.1 Legislation analysis and remote sensing
No stationary trends were found in the time series, even though there is a long history of legislation to regulate fire use and vegetation protection practices.
During the 20 years (1992–2012) of fire use practice prohibition, producers changed their economic profile, and extensive native grassland areas conversion to monocultures in the region occurred (Boziki, Beroldt, Printes, 2011; Buffon, Printes, Andrades-Filho, 2018). Grasslands are important for livestock, especially where other agricultural practices are not viable. In this study, we noticed that legislation changes over the time series are related to changes in land use.
Conservation areas (CAs) in Brazil are managed under the National System of Conservation Units (SNUC), which is designed to protect the country’s diverse ecosystems (BRASIL, 2000). The SNUC comprises 12 categories of CAs, each with specific objectives regarding protection and use. The PET falls under the Park category, which provides full protection and allows only non-consumptive uses of natural resources. This means that handling fire is not permitted inside the PET. Conservation areas like the PET are essential for preserving Brazil’s rich biodiversity and ensuring the long-term sustainability of the country’s natural resources.
The fire management practice in the winter period is expected in the region, and it is used to remove dry biomass to provide the vegetation regrowth that will be used to feed the cattle herd in spring and summer (Pillar et al., 2009).
Once forbidden, it can be replaced by other activities much more environmentally damaging than the old fire management grasslands practice, such as forestry and monocultures, which have been advancing into the grasslands (Buffon, Printes and Andrades-Filho, 2018).
It was observed during the study that municipalities lack data and tools to manage and enforce current environmental legislation. So it is necessary to evaluate the effectiveness of existing environmental management tools regulated by municipal laws related to the use of fire (Santos and Andrades-Filho, 2021).
In addition to the environmental laws presented, it was verified the irregularity in the data availability by the municipalities, which do not meet the legal provisions of free access to information in Brazil, especially regarding publicity, accessibility, and transparency, making technological advancement impossible (Brasil, 2011).
4.2 New burned area classification approach
The brief characteristics of the fire marks left on satellite imagery complicate the burned area’s detection. There are few fire products available globally, and only one at the national level (Alencar et al., 2022). So, evaluating its applicability to different regions and vegetation formations in Brazil is extremely important.
Our study found significant differences in areas mapped as burned, increasing by more than 50% in our study using a better spatial resolution. The improvement of available products is substantial and progresses with the recent technologies emergence. In previous studies of Russian grassland, the difference in the area mapped between satellites with lower spatial resolutions also increased dramatically from no burning to as much as 19% of the total study area (Dubinin et al., 2010). The strategy of using ΔNBR in conjunction with the NIR and SWIR bands for burned areas classification reduced noise and class confusion, especially with wetlands. The areas with the highest commission error were exposed soil from recently cleared forestry areas.
Due to the rugged relief and mosaics of forest and mountainous grassland vegetation that form the area’s landscape, remote sensing data with higher spatial resolution can be explored as they become available. Therefore, Earth observation can significantly support public fire prescription policies and add to other factors that consider CO2 emissions (Herrmann, Nascimento and Freitas, 2022).
5 Conclusion
This study uses remote sensing techniques to evaluate legislation related to fire use in highland grasslands in Brazil. Long-term time series and exploring new enhancement methodologies are essential to identify the main impacts of human-induced changes.
Divergences in extent and frequency were found between the burned areas authorized by the municipalities and those classified as burned. On average, only 43% of the burned area in the PET and its BZ was licensed in the last 5 years. The municipal databases had recorded only from the year 2018, and it is possible to improve the time series from the continuity of data collection.
Our newly burned area methodological classification developed in this article presented results that provide subsidies for reviewing and creating public policies and territorial management.
Data availability statement
The original contributions presented in the study are included in the article/supplementary material, further inquiries can be directed to the corresponding author.
Author contributions
PH designed the study, collected the data, performed the analysis, wrote the paper, discussed the results, and contributed to the final manuscript. VN provided critical feedback, supervised the project, verified the analytical methods, discussed the results, and contributed to the final manuscript. MF and JO verified the analytical methods, discussed the results, and contributed to the final manuscript. All authors contributed to the article and approved the submitted version.
Funding
This research was funded by Fundação de Amparo á Pesquisa do Estado de São Paulo (FAPESP) project number (2017/22269-2).
Acknowledgments
The authors thank the Graduate Program in Remote Sensing at the Federal University of Rio Grande do Sul and the Federal University of ABC in Brazil.
Conflict of interest
The authors declare that the research was conducted in the absence of any commercial or financial relationships that could be construed as a potential conflict of interest.
Publisher’s note
All claims expressed in this article are solely those of the authors and do not necessarily represent those of their affiliated organizations, or those of the publisher, the editors and the reviewers. Any product that may be evaluated in this article, or claim that may be made by its manufacturer, is not guaranteed or endorsed by the publisher.
References
Adagbasa, E. G., Adelabu, S. A., and Okello, T. W. (2020). Development of post-fire vegetation response-ability model in grassland mountainous ecosystem using GIS and remote sensing. ISPRS J. Photogrammetry Remote Sens. 164, 173–183. doi:10.1016/j.isprsjprs.2020.04.006
Alencar, A. A. C., Arruda, V. L. S., Silva, W. V. D., Conciani, D. E., Costa, D. P., Crusco, N., et al. (2022). Long-term landsat-based monthly burned area dataset for the Brazilian biomes using deep learning. Remote Sens. 14 (11), 2510. doi:10.3390/rs14112510
Andrade, B. O., Koch, C., Boldrini, I. I., Vélez-Martin, E., Hasenack, H., Hermann, J. M., et al. (2015). Grassland degradation and restoration: A conceptual framework of stages and thresholds illustrated by southern Brazilian grasslands. Nat. Conserv. 13 (2), 95–104. doi:10.1016/j.ncon.2015.08.002
Boldrini, I. (1997). Campos do Rio Grande do Sul: Caracterização fisionômica e problemática ocupacional. Porto Alegre, Brazil: Boletim do Instituto de Biociências, 1–39.
Bond-Buckup, G. (2008). Biodiversidade dos Campos de Cima da Serra. New York, NY, USA: Livro de Atividades, 96.
Boziki, D., Beroldt S, L., and Printes, C., R. (2011). Situação atual da utilização de agrotóxicos e destinação de embalagens na área de Proteção Ambiental Estadual Rota Sol, Rio Grande De Sul Brasil. Rev. Vitas – Visões 1 (31), 1–35.
Brasil, (2008). Decreto no 6.660, de 21 de novembro de 2008. Regulamenta dispositivos da Lei no 11.428, de 22 de dezembro de 2006, que dispõe sobre a utilização e proteção da vegetação nativa do Bioma Mata Atlântica. Planalto, Brazil: Planalto Gov.
Brasil, (2006). Lei no 11.428, de 22 de dezembro de 2006. Dispõe sobre a utilização e proteção da vegetação nativa do Bioma Mata Atlântica, e dá outras providências. Planalto, Brazil: Planalto Gov.
BRASIL, (2000). Sistema Nacional de Unidades de Conservação LEI No 9.985 DE 18 DE JULHO DE 2000. Planalto, Brazil: Planalto Gov.
Brasil, (2011). Lei no 12.527 de 18 de novembro de 2011. Regula o acesso a informações previsto no inciso XXXIII do art 5° no inciso II do § 3° do art 37 e no § 2° do art 216 da Constituição Federal. Planalto, Brazil: Planalto Gov.
Buffon, I., Printes, R. C., and Andrades-Filho, C. D O. (2018). Licenciamento ambiental do uso do fogo nos Campos de Cima da Serra, Rio Grande do Sul, Brasil: Determinação do período de pousio e sugestões para uma rotina de monitoramento. Rev. Eletrônica Científica UERGS 4, 447–469. doi:10.21674/2448-0479.43.447-469
Buisson, E., Le Stradic, S., Silveira, F. A. O., Durigan, G., Overbeck, G. E., Fidelis, A., et al. (2019). Resilience and restoration of tropical and subtropical grasslands, savannas, and grassy woodlands. Biol. Rev. 94 (2), 590–609. doi:10.1111/brv.12470
Carvalho, E. D., and Andrades-Filho, C. D O. (2019). Análise Espaço-Temporal do uso e cobertura do solo em São Francisco De Paula, RS/Brasil, Mudanças nos sistemas agrícolas e territórios no Brasil. Porto Alegre, Brasil: Planalto Gov. doi:10.21674/9788560231.118-145
Dubinin, M., Potapov, P., Lushchekina, A., and Radeloff, V. C. (2010). Reconstructing long time series of burned areas in arid grasslands of southern Russia by satellite remote sensing. Remote Sens. Environ. 114 (8), 1638–1648. doi:10.1016/j.rse.2010.02.010
ESA, (2022). Sentinel 2. https://sentinel.esa.int/web/sentinel/missions/sentinel-2 (Accessed August 15, 2022).
Fidelis, A., Roy, D. P., Humber, M. L., and Justice, C. O. (2021). What matters for vegetation regeneration in Brazilian subtropical grasslands: Seeders or resprouters? Flora Morphol. Distribution, Funct. Ecol. Plants 279, 151817. doi:10.1016/j.flora.2021.151817
Giglio, L. (2018). The Collection 6 MODIS burned area mapping algorithm and product. Remote Sens. Environ. 217, 72–85. doi:10.1016/j.rse.2018.08.005
Goehry, B. (2021). Random forests for time series.HAL archives-ouvertes. Available at: https://hal.archives-ouvertes.fr/hal-03129751.
Herrmann, P. B., Nascimento, V. F., and Freitas, M. W. D. d. (2022). Fire analysis in grasslands using remote sensing: A systematic review. Rev. Bras. Cartogr. 74 (2), 437–458. doi:10.14393/rbcv74n2-63739
Key, C. H., and Benson, N. C. (2006). Landscape Assessment (LA) sampling and analysis methods. 164 RMRS-GTR. Washington, D.C., United States: USDA Forest Service - General Technical Report RMRS-GTR.
McKinney, W., Perktold, J., and Seabold, S. “Time series analysis in Python with statsmodels,” in Proceedings of the 10th Python in Science Conference, (Scipy), Austin, Texas, United States, July 2011, 107–113. doi:10.25080/majora-ebaa42b7-012
Meireles, L. D., and Shepherd, G. J. (2015). Structure and floristic similarities of upper montane forests in Serra Fina mountain range, southeastern Brazil. Acta Bot. Bras. 29 (1), 58–72. doi:10.1590/0102-33062014abb3509
Municipio de Cambará do Sul, (2013). Lei municipal no 2.954, de 26 de julho de 2013. Brazil: Lei de Queima Controlada.
Municipio de Jaquirana, (2013). Lei ordinária no 1083, de 16 de julho de 2013. Brazil: Lei de Queima Controlada.
Municipio de São Francisco de Paula, (2013). Lei no 2924, de 12 de junho 2013. Brazil: Lei de Queima Controlada.
Myers, N., Mittermeier, R. A., Mittermeier, C. G., da Fonseca, G. A. B., and Kent, J. (2000). Biodiversity hotspots for conservation priorities. Nature 403 (6772), 853–858. doi:10.1038/35002501
Overbeck, G. E., Muller, S., Fidelis, A., Pfadenhauer, J., Pillar, V., Blanco, C., et al. (2007). Brazil's neglected biome: The South Brazilian Campos. Perspect. Plant Ecol. Evol. Syst. 9 (2), 101–116. doi:10.1016/j.ppees.2007.07.005
Pal, M. (2005). Random forest classifier for remote sensing classification. Int. J. Remote Sens. 26, 217–222. doi:10.1080/01431160412331269698
Pillar, V. D. P. (2009). Campos Sulinos - conservação e uso sustentável da biodiversidade. Brasília: Ministério do Meio Ambiente, 403.
Pivello, V. R., Vieira, I., Christianini, A. V., Ribeiro, D. B., da Silva Menezes, L., Berlinck, C. N., et al. (2021). Understanding Brazil's catastrophic fires: Causes, consequences and policy needed to prevent future tragedies. Perspect. Ecol. Conservation 19 (3), 233–255. doi:10.1016/j.pecon.2021.06.005
Rio Grandedo Sul, (2012). Lei no 13.931, de 30 de janeiro de 2012. Brasil: Código Florestal Estadual.
Rio Grandedo Sul, (1992). Lei no 9.519, de 21 de janeiro de 1992. Brasil: Código Florestal Estadual.
Rio Grandedo Sul, (2008). Plano de manejo do Parque Estadual do Tainhas. Porto Alegre, Brasil: Planalto Gov.
Santos, D., and Andrades-Filho, C. D. O. (2021). Uso do fogo nos campos de altitude do sul do brasil: Análise do licenciamento ambiental a partir de geotecnologias. Rev. Bras. Meio Ambiente 164, 146–164.
Souza, C. M., Z. Shimbo, J., Rosa, M. R., Parente, L. L., A. Alencar, A., Rudorff, B. F. T., et al. (2020). Reconstructing three decades of land use and land cover changes in Brazilian biomes with Landsat archive and Earth engine. Remote Sens. 12 (17), 2735. doi:10.3390/rs12172735
Keywords: fire, grasslands, ΔNBR, GEE, MapBiomas, time series
Citation: Herrmann PB, Nascimento VF, Freitas MWDd and Ometto JP (2023) Fire in highland grasslands in the Atlantic Forest Biome, a burned areas time series analysis and its correlation with the legislation. Front. Remote Sens. 4:1099430. doi: 10.3389/frsen.2023.1099430
Received: 15 November 2022; Accepted: 02 May 2023;
Published: 16 May 2023.
Edited by:
Jane Southworth, University of Florida, United StatesReviewed by:
André Lima, University of Maryland, College Park, United StatesRogério Negri, São Paulo State University, Brazil
Copyright © 2023 Herrmann, Nascimento, Freitas and Ometto. This is an open-access article distributed under the terms of the Creative Commons Attribution License (CC BY). The use, distribution or reproduction in other forums is permitted, provided the original author(s) and the copyright owner(s) are credited and that the original publication in this journal is cited, in accordance with accepted academic practice. No use, distribution or reproduction is permitted which does not comply with these terms.
*Correspondence: Pamela Boelter Herrmann, pamela.herrmann@ufrgs.br