- 1Spiri Robotics Inc., Halifax, Nova Scotia, Canada
- 2Department of Oceanography, Dalhousie University , Halifax, Nova Scotia, Canada
- 3Department of Fisheries and Oceans, Ottawa, ON, Canada
Losses of river complexity and viable habitat has led to negative effects on Atlantic salmon. With the rapid population decline of Atlantic salmon, there has been an increase in river restoration and salmon reintroduction projects, and an understanding of substrate is a vital component in the restoration of these habitats. However, the isolation and/or inaccessibility of many of these rivers make the collection of this information challenging and expensive based on conventional survey approaches. This study looks at the feasibility and accuracy of conducting substrate analysis using low-cost uncrewed aerial vehicles (UAV) at seven transects through macroscale river habitat (riffles, runs and pools) on the Upper Salmon River located in Fundy National Park near Alma, New Brunswick, Canada. Using ArcGIS, a supervised classification was conducted separating the dry and submerged substrate for higher accuracy. An object-based image analysis was conducted in PCI for delineation of substrate size. Small ideal spawning substrate was found to be concentrated in slower flowing pools while large substrate was concentrated in faster flowing riffles. The substrate analysis was conducted with an accuracy of 79% for dry substrate and 86% for submerged substrate, demonstrating the potential of UAV use in salmon habitat analysis.
1 Introduction
Rivers are complex habitats with often steep gradients in elevation, substrate type, flow, and depth (Schumm, 2007). In addition, they interface with the coastal ocean as a link between terrestrial and marine habitats. Fish and invertebrates exploit the variety of river habitats as do mammals and birds (Dubuc et al., 1990; Rushton et al., 1994; Schneider and Winemiller, 2008). Diadromous fishes such as salmonids require the full diversity of river and marine environments to complete their life cycle. Since rivers are so integral to terrestrial landscapes, they are often subject to human disturbance including dams, siltation, course alteration, eutrophication and other changes in water quality (Hamilton, 2002; Wohl, 2005; Hilton et al., 2006). These alterations interact with climate change in negative ways, e.g., deforestation reduces shade availability, by removing riverside vegetation (Garman and Moring, 1991) and bank stability by removing the roots that anchor soil (Horton et al., 2017). Changes to river habitat quality affect multiple species that depend on river structure and function for their life histories that include feeding, growth, reproduction, and migration (Thorstad et al., 2008).
An understanding of the variety of river habitats, their arrangement in the riparian landscape, and relationship to useable habitat are critical in conserving river species. Habitat structure refers to the physical composition (arrangement of physical matter such as substrate) and three-dimensional complexity of an area (McCoy and Bell, 1991). In river environments, this complexity can refer to current velocity, structural density, rugosity, porosity of substrate and substrate size diversity/distribution (Willis et al., 2005). Increased complexity creates microhabitats that diversify niche space and species density in aquatic environments (Schlosser, 1987; Holbrook and Schmitt, 2002). Moreover, river restoration efforts require this information to ensure that physical structures are reconstructed at the appropriate spatial scale and diversity to provide suitable habitats (Finstad et al., 2007).
Species distribution modelling (SDM) attempts to match presence/absence of a given species with habitat features to understand how habitat affects distribution (Elith and Leathwick, 2009). The essential component of SDM is the ability to spatially characterize habitat. Statistical models may be used to delineate the importance of upstream-downstream gradients in fish distribution (e.g., Buisson et al., 2008), but there are relatively few examples that address detailed mapping of river habitat.
Habitat mapping is used to quantify the geographic patterns and types of biophysical characteristics of the environment which influence the distribution of an organism (Brown et al., 2011). Because many river areas are isolated and/or inaccessible, habitat mapping is particularly difficult. As with any aquatic habitat, water cover precludes visual access. Structural complexity has a large influence on the success of river fish such as Atlantic salmon. The current methods used in river habitat surveys include LiDAR (light detection and ranging), which is costly (Woodget et al., 2015) or in-situ surveys, which are time consuming and difficult for broad scale analyses. In recent years, there have been rapid advancements in remote sensing technology, particularly uncrewed aerial vehicles (UAVs or drones). UAVs have become easy to use, rapidly deployable, capable of collecting high resolution data and inexpensive research tools for remote sensing (Saska et al., 2012.; Tang and Shao, 2015). Coupled with high resolution cameras, their application to river mapping is growing (Zinke and Flener, 2013; Rusnák et al., 2018)). High resolution aerial images coupled with GPS and altitude data can be used to model river morphology in a 3D approach referred to as “structure from motion” (SFM) (Westoby et al., 2012). This approach matches features in overlapping images to create a three-dimensional model using photogrammetry, in addition to producing an accurate orthomosaic of the area.
Applying SFM to drone imagery can also generate DEMs (digital elevation models) and when combined with orthomosaics of the imagery, detailed information can be extracted to determine substrate and structural complexity, based on substrate size and distribution, of the terrain (Arif et al., 2017; Marteau et al., 2017; Leduc et al., 2019; Danhoff and Huckins, 2020). Although information extraction from DEMs is widely used in terrestrial ecology (Cunliffe et al., 2016; D'Urban, 2020; Iglhaut et al., 2019; Forsmoo et al., 2018; Alonzo et al., 2018) they are an evolving tool for analysis of riverbed morphology Photogrammetry in river habitat encounter issues regarding the depth of light penetration, turbidity, reflection and refraction in submerged areas but this technique is still applicable to model river topography and substrate analysis for fluvial morphology studies (Lane, 2000; Woodget et al., 2015; Arif et al., 2017; Marteau et al., 2017; Woodget et al., 2017).
In the North Atlantic, Atlantic salmon (Salmo salar) are an iconic anadromous species, threatened or endangered throughout much of their range by river destruction, overfishing in freshwater and at sea, climate change, as well as predation and changes in pelagic food webs (Amiro et al., 2009). Atlantic salmon spend between 2 and 4 years of their life in rivers as parr (Mills, 1989), with subsequent smoltification and either single or multiple migrations between river and sea (Jonsson et al., 1991). Habitat use is specific to egg deposition, juvenile feeding and predator avoidance and habitat distribution may be different for each life stage. Many eastern Canadian populations undergo long distance ocean journeys to Greenland, with reduced survival at sea (Dadswell., 1968). However, other populations have a more localized migration, particularly those of the Inner Bay of Fundy who remain within the Bay of Fundy and Gulf of Maine (IBoF salmon; Department of Fisheries and Oceans Canada, 2010). IBoF Atlantic Salmon have recently seen population declines of up to 97% (Irvine et al., 2005) and most populations are critically endangered or extinct in individual rivers, only remaining via captive breeding and re-stocking programs.
There is an increase in projects seeking to restore breeding habitat (Einum et al., 2008), including a recovery strategy from the Government of Canada (Trzcinski, et al., 2004) and reintroduction through captive rearing programs (Clarke et al., 2016). Habitat degradation to many of the ∼40 rivers used in their life cycle plays an important yet unknown role in their early life history. Protected areas such as those in national parks therefore serve as templates for healthy river ecosystems and the distribution of natural habitats within these environments. Understanding which rivers have areas of suitable substrate diversity is vital for the recovery of the species. The most common method of data gathering for habitat analysis are in-situ surveys, these involve visual observations of detailed cross-river transects, river segments or specific known spawning habitat (Heggenes et al., 1996; Borsányi et al., 2004; Linnansaari et al., 2009; Hill et al., 2019; Marsh et al., 2020). The data is subsequently used to model and predict habitat use and potential spawning habitat. Programs such as Physical HABitat SIMulation (PHABSIM), are used to simulates the relationship between streamflow and physical characteristics, such as substrate (Borsányi et al., 2004; Moir et al., 2009). Often the focus of these studies is to collect, describe or predict the depths, velocities and substrate used by the spawning fish. Substrate in particular is important as salmon dig redds to protect against predation, freezing, shifts in substrate, and low water levels (Heggenes, 1990; Armstrong et al., 2003; Lapointe et al., 2004; Davey and Lapointe, 2007; Louhi et al., 2008). Their eggs require high concentrations of oxygen, not found in silt/sandy (<0.02 mm) substrate, and they are unable to move larger gravel (>100 mm) giving them a narrow suitable substrate spawning range (∼5.4–100 mm) (Armstrong et al., 2003; Lapointe et al., 2004; Louhi et al., 2008). Collecting in-situ surveys for this work is time consuming and generally only covers a small segment of the river. Easily deployable UAVs mounted with high resolution cameras can offer an alternative to these traditional methods.
Fundy National Park (New Brunswick) is the site of a major salmon recovery project formed as a partnership between Parks Canada, Cooke Aquaculture, Fort Folly First Nation, University of New Brunswick, and Dalhousie University (Clarke et al., 2016). Running through Fundy National Park is the Upper Salmon River (USR), which was considered devoid of native salmon populations due to logging dams placed in the early 1900s (Dadswell, 1968). The dams have since been removed and salmon restoration efforts are underway. Using the live gene bank established by the Department of Fisheries and Oceans (DFO), genetically diverse wild salmon were reared from wild smolts (Clarke et al., 2016). These juveniles were then released into the rivers, before subsequently being recaptured and reared to maturity in marine net pens to increase adult survival rates. The adult salmon were then re-released into the river for breeding. The rearing strategies employed in the Upper Salmon River led to the discovery that releasing juveniles before the onset of feeding increased their mass and fecundity over those that were kept in captivity for longer (Clarke et al., 2016), highlighting the importance of habitat in early life history. If this program is to expand to other rivers in the Inner Bay of Fundy, an assessment of available habitat is required to determine which rivers are best suited for restocking and/or restoration.
The goal of this research was to delineate salmon habitat based on substrate type in a pristine river via use of an UAV. Specifically, we explore the capability of a drone to determine substrate composition as a component of structural complexity for salmon spawning habitat with the following objectives:
• Test the utility of UAV to obtain high resolution aerial imagery of a protected river used for salmon restocking and analyze the imagery for substrate size.
• Evaluate the accuracy and validate image-based substrate classification through varying macro-habitats (riffles, pools, etc.) to determine the effects of physical characteristics (flow and depth) on imagery.
• Compare substrate composition in macrohabitats with known salmon preference to evaluate if macro-habitats can be used as an indicator of salmon spawning grounds.
2 Materials and methods
2.1 Study site
The Upper Salmon River (45.599°N, 64.948°W) is located in Fundy National Park near Alma, New Brunswick, draining into Chignecto Bay in the Bay of Fundy (Figure 1). Comprising a sand and gravel estuary with salt marsh and a macrotidal delta near the mouth of the river, the predominantly fast-flowing, boulder strewn habitat is considered critical salmon habitat by the Department of Fisheries and Oceans Canada (2010). The river, which descends from a plateau, was studied from The Forks, the name of a junction within the river leading to steep waterfalls that are considered the upper limit of salmon distribution to near sea level. The study site includes seven cross-river transects within ∼10 kilometers that encompass the extent of the salmon habitat within the river (Figure 1).
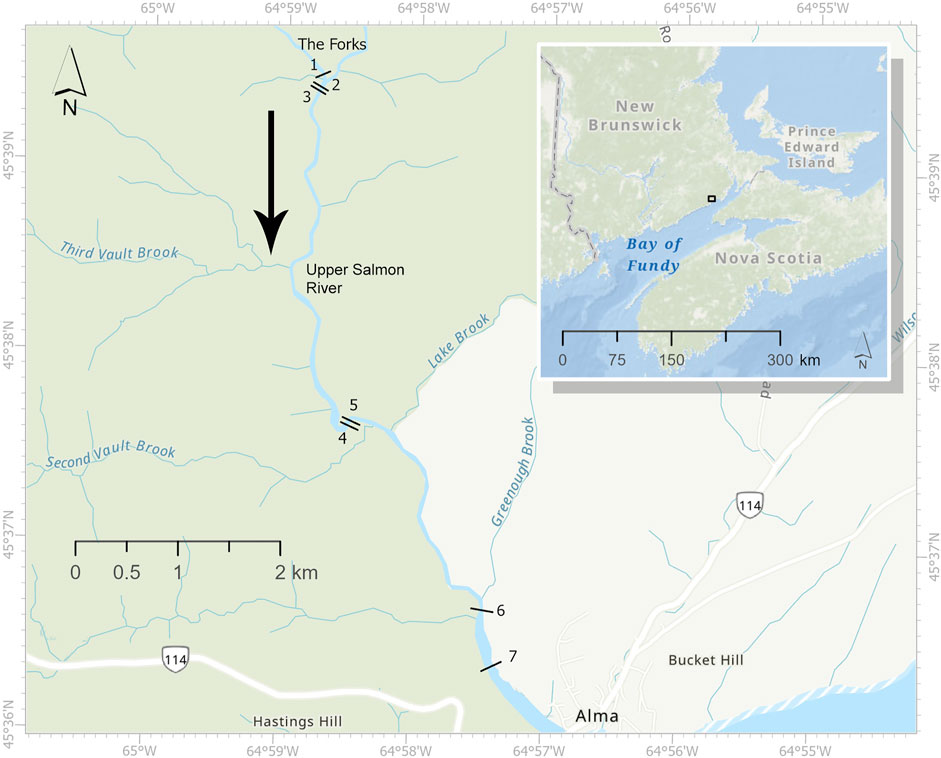
FIGURE 1. Map of the Upper Salmon River within New Brunswick created using the ArcGIS basemap. The black lines represent the approximate locations of seven transects. The inset shows the location of the Upper Salmon River (black box) within New Brunswick. The black arrow indicates the downstream flow direction. The Forks, the junction in the Upper Salmon River that leads to the upper limit of salmon distribution, is indicated on the map.
2.2 Data collection
2.2.1 Drone data
Aerial imagery was collected using a DJI Phantom 4 drone at each of the transects described above in late August of 2017 when seasonally water levels are lower than during spawning season. The depths of the transect did not exceed 120 cm, with an average depth of 7–15 cm. The seven transects were four quadrats wide (each quadrat 1 m2) and the length was the full width of the river which varied from ∼ 80 m at its widest transect (Transect 7) to ∼ 17 m at its narrowest transect (Transect 3). Drone positioning was calculated using the on-board GPS/GLONASS (global navigation satellite system), as well as the barometric pressure sensor for elevation. Imagery was obtained using the high dynamic range, high resolution camera of 4000 × 3000 pixels, standard on the drone. The drone was flown over the transects, with the downward facing camera, at 30 m altitude and encompassed >80% frontal and side overlap in imagery covering the transect locations. A buffer area was flown around the transect to allow for the overlap throughout the entire transect, with the excess area cropped in processing. Still images were taken at 7 s intervals, with the drone paused in hover. This process was repeated for each transect location and included 730 images. The imagery was processed in the software package Pix4D to create orthomosaics of each transect with a resolution of 1.7 cm per pixel.
2.2.2 Ground truth data
The pool, riffle, run classification is often used by biologists to identify spawning habitat (Gibson, 1993), which generally occurs in areas categorized by riffles, runs or the tail ends of shallow pools. These geomorphological categories, referred to herein as macro-habitats, include their transition zones (e.g., pool/riffle), where one habitat (e.g., pool) meets and flows into the other (e.g., riffle). Transects were placed in each of the river geomorphological categories, referred to herein as macro-habitat: 1) pool/riffle, 2) pool, 3) run/riffle, 4) pool/run, 5) riffle, 7) run. An additional run transect was added at a long-term monitoring site 6) as it is a well-studied area of the river. Pools are characterized by a slow current with deeper water. Riffles are shallow areas with fast turbulent water, often with rocks protruding through the surface. Runs have a moderate current and depth, with a continuous water surface, and connect areas between riffles and pools. Although previous studies have characterized depths and water velocities of these macro-habitats, the classification is often subjective and analyzed visually (Jowett, 1993; Vadas & Orth, 1998; Ward et al., 2003). Zones were identified visually within the river, by water flow and depth. Visual inspection indicated that the pool/riffle segment (Transect 1; Figure 2A) had large boulders with breaks in the surface of the water (riffles), followed by deeper, calm water with smaller substrate boulders (pool). The pool segment (Transect 2; Figure 2B) is dominated by deeper water and fine substrate (fine gravel), interspersed with large boulders. The run/riffle segment (Transect 3; Figure 2C) had breaks in the surface of the water over large/medium substrate, followed by shallower calm water (run). The pool/run segment (Transect 4; Figure 2D) had shallow water dominated by medium substrate (run), followed by the deep, darker calm water (pools). The riffle segment (Transect 5; Figure 2E) had shallower water with breaks over the rocks. The run (Transect 6; Figure 2F) was dominated by medium substrate and consisted of shallower calm water. The long-term monitoring segment (Transect 7; Figure 2G) had shallow water dominated by fine substrate and submerged vegetation.
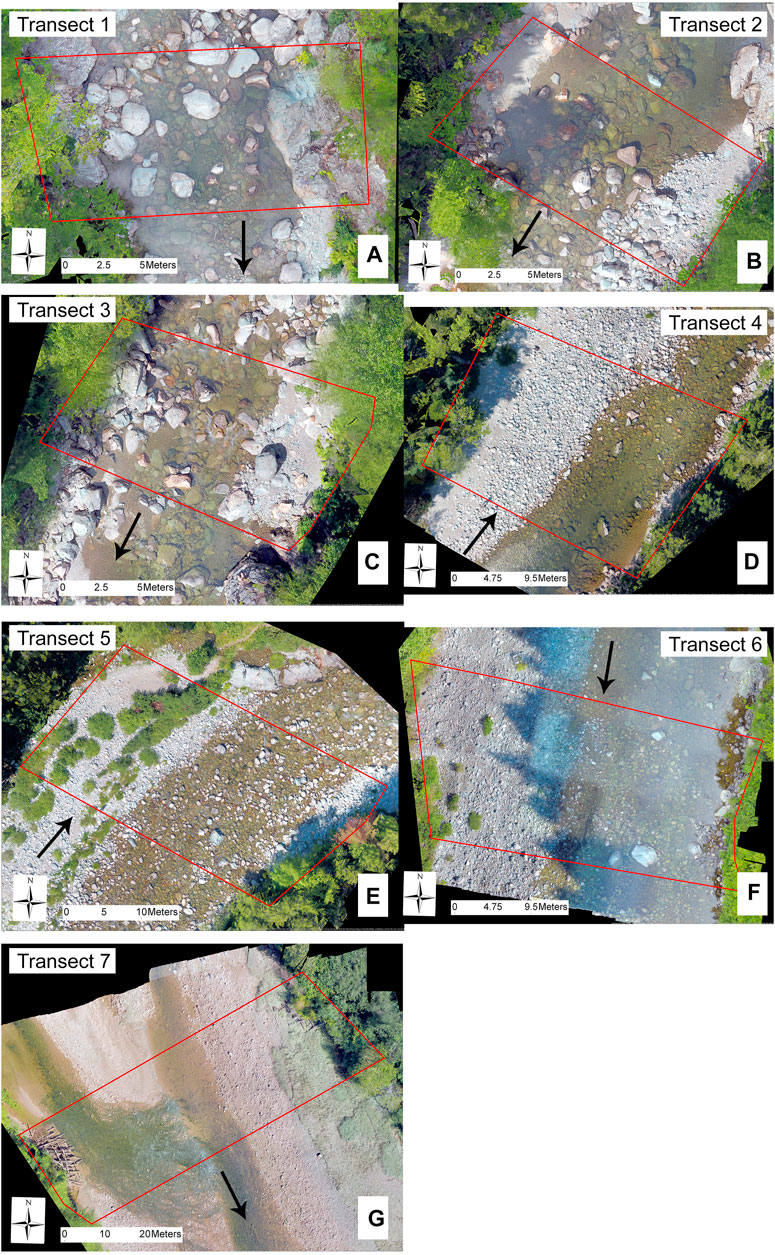
FIGURE 2. Orthomosaics for transects 1–7, transect areas denoted by the red boxes, with black arrows to indicate downstream flow direction. (A) Transect 1: Pool/Riffle, (B) Transect 2: Pool, (C) Transect 3: Run/Riffle, (D) Transect 4: Pool/Run, (E) Transect 5: Riffle, (F) Transect 6: Run/Long term monitoring site, (G) Transect 7: Run (Lowermost Site)
The substrate was classified visually in the field within the quadrats by the dominant sediment along each transect based on substrate texture categories (Table 1), ranging from silt to bedrock, based on those established by Borsányi et al. (2004). The field data was subsequently used to validate the results from Section 2.3.2.
2.3 Classification
The substrate classification was based on a similar study conducted by Arif et al. (2017). Transects underwent a simple supervised classification to separate RV, dry/exposed substrate and wet/submerged substrate (Figure 3). They subsequently underwent substrate size classification through an object-based image analysis (OBIA) in PCI Geomaticas.
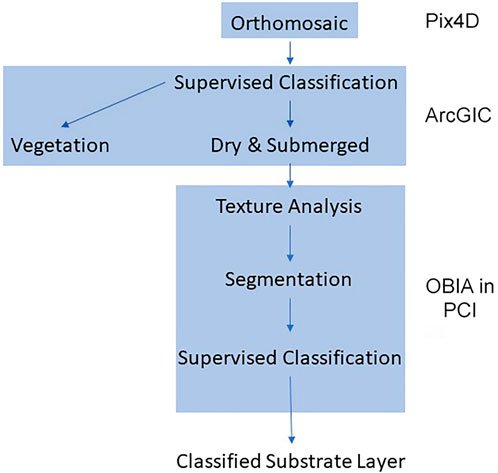
FIGURE 3. Data processing steps: The orthomosaic undergoes a supervised classification (ArcGIS) to separate the RV from the substrate. The substrate is then analyzed for texture. The pixel values of the substrate are used to segment the image into substrate sizes, which are assigned through another supervised classification in PCI.
2.3.1 Supervised classification of dry substrate, submerged substrate and riverside vegetation
Using ArcGIS, each orthomosaic was clipped around the transect locations to remove the edges and areas with insufficient overlap to be considered reliable (Figure 4). Both wet and dry substrate categories were analyzed as habitat, since drone imagery was obtained during low water level where exposed substrate would equate to potential spawning habitat during the spawning season when the water is higher. A supervised classification involves user derived training sites for all the classes provided, using pixel information within the training site to segment and classify a given area. On each transect, sections were assigned using polygons, representing each of the classes, wet, dry and RV, for training. These training classes covered ∼28% of the total area of all seven transects. An object-based classification was used in ArcGIS Pro 2.3 for sectioning into wet/dry/RV, which involved consideration of neighbouring pixels for their spectral (color) and spatial (shape) characteristics. These characteristics are part of the Image Classification Tool in ArcGIS Pro and both spectral and spatial characteristics work on separate scales ranging from 1–20 to determine how to section the image. A higher spectral value allows for a more detailed classification among objects, while a higher spatial value allocates a greater importance to spatial proximity of features, allowing for more clustered features. The ideal values for spectral and spatial detail to provide the best overall accuracy for all seven transects were determined to be 17.90 and 15 respectively, based on visual assessment and the accuracy assessment. The image underwent the supervised classification after training sites were selected from the manually sectioned polygons (Figure 4). The resulting images were 3 separate RGB (Red Green Blue) images of dry, submerged and vegetated for each transect. Vegetated areas were clipped and the data discarded, and subsequent analysis conducted on only the submerged and dry areas.
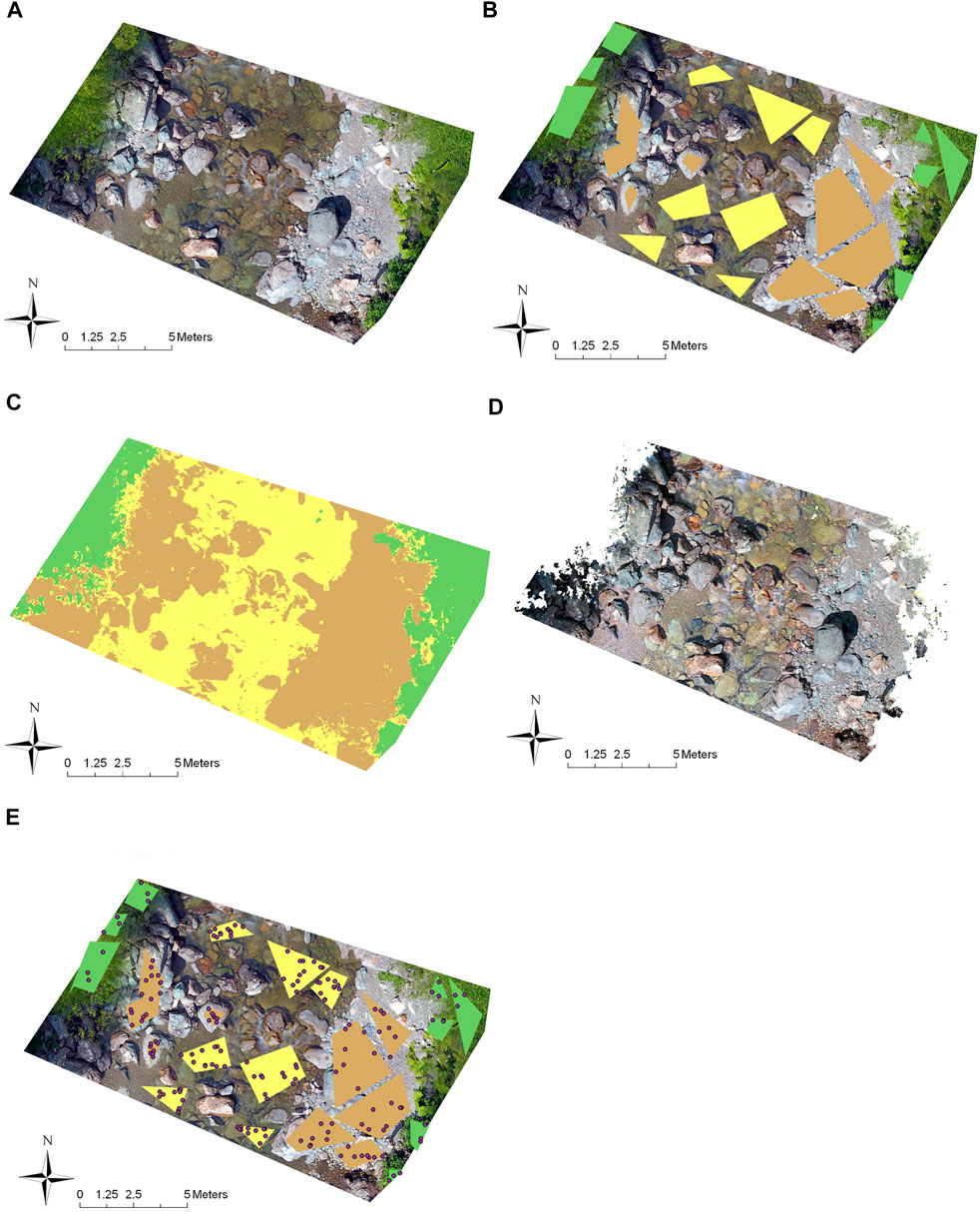
FIGURE 4. Example of the process of classifying substrate (transect 3). (A) Drone imagery of the transect. (B) Selected training sites showing the RV (green), dry (brown) and submerged (yellow) polygons. (C) Resulting classified shape files used to extract dry (brown) and submerged substrate (yellow) from the RV (green). (D) Raster layer with the RV areas removed. (E) Example from transect 3 of random point selection chosen by the ArcGIS toolset within the training areas used to produce the error matrix. The accuracy point is omitted from training and their predicted value compared to the polygon designation. Green polygons are vegetation, brown polygons are dry substrate and yellow polygons are the submerged substrate.
In order to assess the accuracy of the trained classification, the toolset selects 500 random points within the training polygons and omits them from training. The selection of points within the training polygons is a requirement of the toolset. The predicted value (wet/dry/vegetated) was compared to the polygon designation. An error analysis was conducted by determining the % agreement between training categories and predictions from image analysis.
2.3.2 Substrate classification using object-based image analysis
In object-based image analysis (OBIA) an image is first segmented based on spectral similarity while considering neighbouring pixel values (Hossain and Chen, 2019). A portion of the segments are then assigned to training sites before classification of the remaining segments based on the attributes found in the training sites. For the OBIA of substrate type (Figure 5), PCI Geomaticas 2019 was used to create small segments within wet or dry sections based on the texture of the red band (homogeneity, contrast and mean intensity), evaluated on a 10 × 10 pixel grid (1.74 cm per pixel). Texture refers to the variations in intensity values of pixels (bumpy, rough, smooth, silky) and considers spatial relationships among neighbouring pixels by examining how often specific values of pixel pairs co-occur (Arif et al., 2017). Based on Arif et al. (2017), the submerged and dry images were run through the OBIA separately to increase accuracy. Substrate type based on visual designation was assigned to a set of training segments (Figure 5). As the PCI software relies on the manual designation of training and accuracy sites, fine gravel (0.2–2 cm) was combined with the coarser gravel (2–5 cm) classification referring to 0.2–5 cm collectively as gravel, as they were not visually distinguishable from one another. Accuracy of this trained classification was quantified by comparing a separate set of predicted substrate types to visual assessment of the same segment. An error analysis was then constructed for each substrate class but reported as overall % agreement between training and predictions. The number of segments allocated to accuracy assessment was dependent on the number of segments available, the size of the segments and the size of the transect. In total there were 94, 95, 86, 76, 107, 80, and 89 accuracy assessment segments assignments to transects 1–7 respectively, with twice the amount of training segments as accuracy segments.
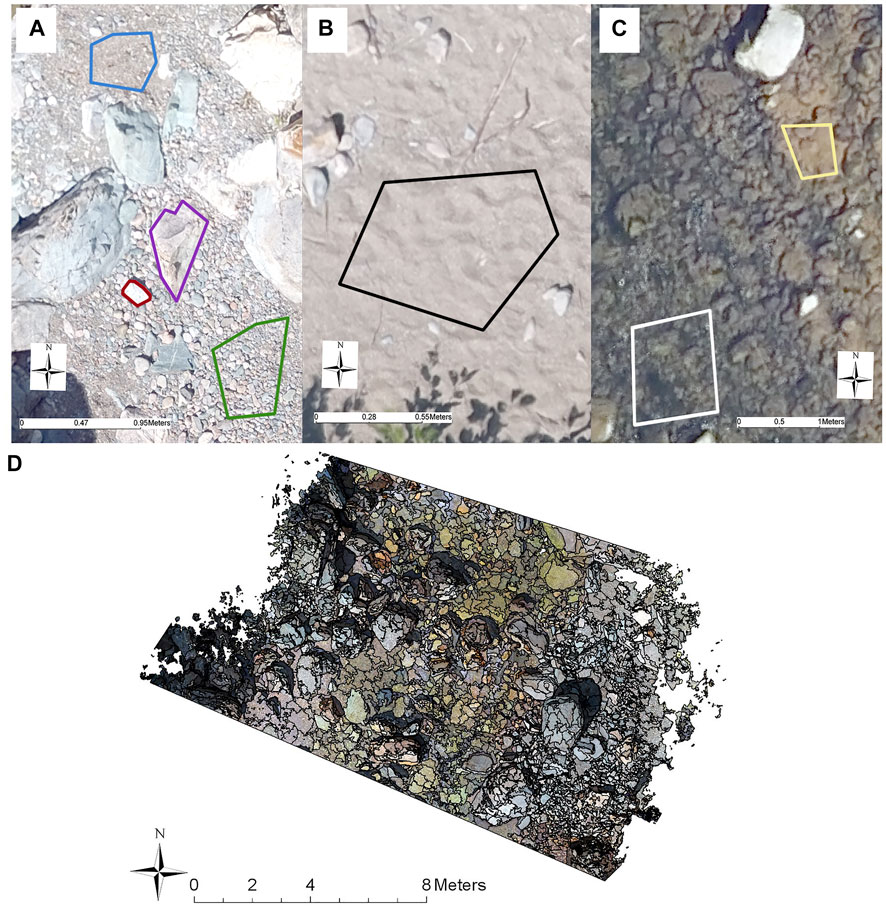
FIGURE 5. An example of ground cover samples used as training sites in the supervised classification of substrate in both dry and submerged environments. (A) purple (large boulders), red (boulders), green (gravel), blue (fine gravel). (B) black (sand). (C) white (other vegetation), yellow (silt). (D) An example of the segmentation results, overlayed on top of the dry/submerged image for transect 3.
Finally, substrate type categorized in the above method was compared to field data on substrate collected in ground truthing studies of transect 1 as a further assessment of training accuracy. Due to discrepancies in the GPS co-ordinates of the field data, only transect 1 was available for validation. A visual assessment was conducted on the orthomosaic for transect 1 (pool/riffle transition zone), where the field quadrat substrate results were mapped over the PCI analysis. The dominate substrate type for each quadrat was compared and an error analysis was conducted between both sets of data, similar to the supervised classification.
2.4 Spawning habitat
Although measurements vary, Atlantic salmon have been reported to breed in substrate as small as 0.54 cm to as large as 10 cm (Armstrong et al., 2003), with 1.6–6.4 cm being considered an ideal range (Louhi et al., 2008). In the present study, the gravel category (0.2–5 cm) was considered to be ideal spawning substrate, recognizing that other factors (e.g. flow) are also important in spawning habitat.
3 Results
Imagery captured from the drone produced seven orthomosaic images (Figure 2), which were clipped and used to extract transect data.
3.1 Supervised classification
The supervised classification was conducted over all seven transects and extracted submerged substrate, dry substrate and RV (Figure 6). Certain large boulders were classified as both submerged and dry substrate when they were observed to project out of the water. Using the training sites, substrate was extracted with minimal misidentification in the RV class, while submerged and dry substrate had some areas of misidentification. Areas of dry substrate shaded by RV were occasionally misclassified, but areas of dry substrate shaded by large boulders were not.
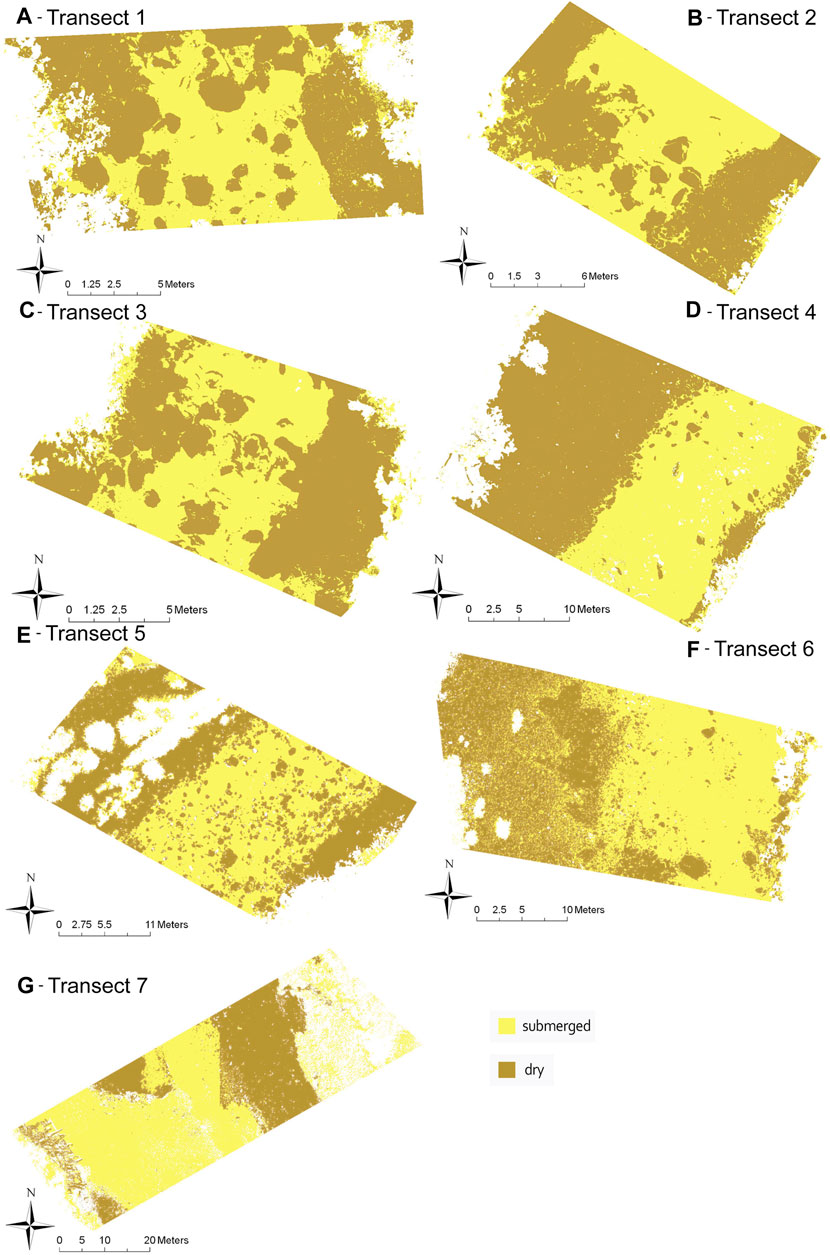
FIGURE 6. Classified orthomosaics for transects 1–7 (A–G) with RV removed using a simple supervised classification. Yellow is submerged substrate and brown is dry substrate.
Using the randomly assigned points within the training sites (Figure 4) allowed predictions to be made for dry, wet, and RV categories for each transect. When compared to training categories the average accuracy of all seven transects (% agreement between categories) was 90%, with no values lower than 74.7% (Table 2). The highest accuracy was in transects 5, 3, 4 and 1, representing transects in riffle, run/riffle, pool/run and pool/riffle respectively, with each transect scoring above 96% accuracy. The lowest accuracy was in transects 7, 2 and 6, representing transects in the southern most site, pool and run respectively, with transects scoring between 74.7% and 89.4% accuracy. These results indicate that regardless of river habitat, the imagery was able to adequately separate emerged and submerged substrate and RV with an accuracy of at least 75%, but in most cases exceeding 90%.
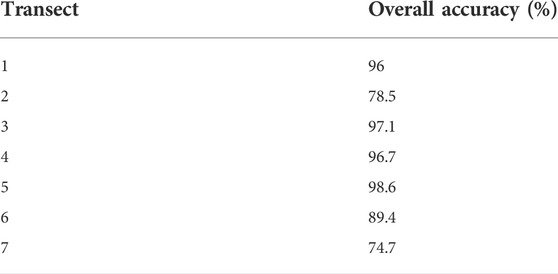
TABLE 2. Overall accuracy of the supervised classification for segmentation transects 1–7, which is the percent agreement between categories in the accuracy points and classified image.
3.2 Spawning habitat
After supervised classification was used to separate larger habitat division (wet and dry), OBIA allowed delineation of substrate. Most of the substrate consisted of a range from fine gravel to boulders (Figure 7). The end member categories of bedrock and silt were represented only in transects 1 and 7 respectively. Underwater vegetation, appearing as green moss was also only represented in transect 7, categorized as “other vegetation.” Two of the transects (2 and 6) had shadows across part of the transect which caused misclassification of the submerged and dry substrate but did not noticeably affect the substrate classification. Transect 1, near the Forks, had very little fine substrate in the dry portion of the transect, and so boulders, large gravel and gravel were combined (Figure 8). Consequently, as fine gravel could not be separated from the other fine substrate, none of the dry transect 1 was considered to be ideal substrate for salmon spawning. Of the submerged portion of this transect, 16% was ideal spawning grounds, leading to a total of 8% of transect 1 as suitable spawning substrate (Figure 8A). On transect 2, very close to transect 1, large boulders were also present, but the substrate was dominated by sand and gravel, as might be expected in the quieter waters of a pool. Thus, a total of 34% was ideal substrate in both the dry and submerged areas (Figure 8B). Transect 3 (run/riffle) was similar to transect 1 with a dominance of coarse substrate especially in the dry portion. It included a total of 17.5% area categorized as ideal spawning substrate with 6% in the dry area and 29% in the submerged area (Figure 8C). Transect 4 (pool/run) consisted mostly of boulders and large gravel, similar in wet and dry areas, and contained 11.5% of ideal spawning grounds, 14% in the dry area and 9% in the submerged (Figure 8D). Transect 5 (riffle), although close to transect 4, was dominated by gravel in the submerged area, but by boulders in the dry habitat. It contained 4% total ideal spawning substrate with none in the submerged area and 8% in the dry area (Figure 8E). On both transects 4 and 5, despite the presence of gravel, the substrate was too coarse for abundant spawning habitat. Transect 6 (run) was dominated by large gravel and boulders in both wet and dry areas, and thus only 2.5% of the area was determined to be ideal substrate, with none in the submerged and 5% in the dry area (Figure 8F). Transect 7 is furthest downstream where there are depositional areas and the river broadens. It was the only transect with silt. It was interesting that suitable spawning substrate was only present in dry areas. Based on this difference it contained 21% overall ideal substrate with 42% in the dry area (Figure 8G).
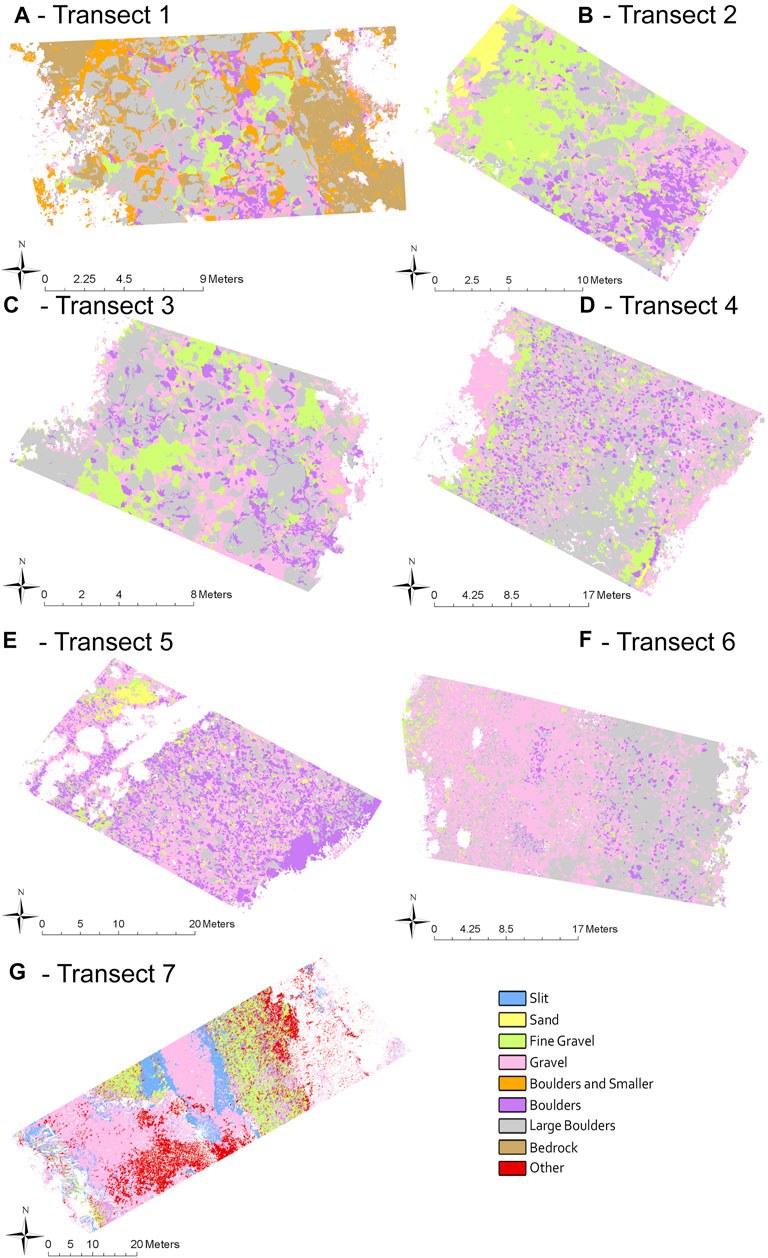
FIGURE 7. Substrate size of transects 1–7(A–G) classified through OBIA. Substrate size includes “other” on transect 7 for submerged vegetation and “boulder and smaller” only used on transect 1 where fine substrate was limited. Submerged and dry substrate are analyzed separately but presented together for ease of analysis.
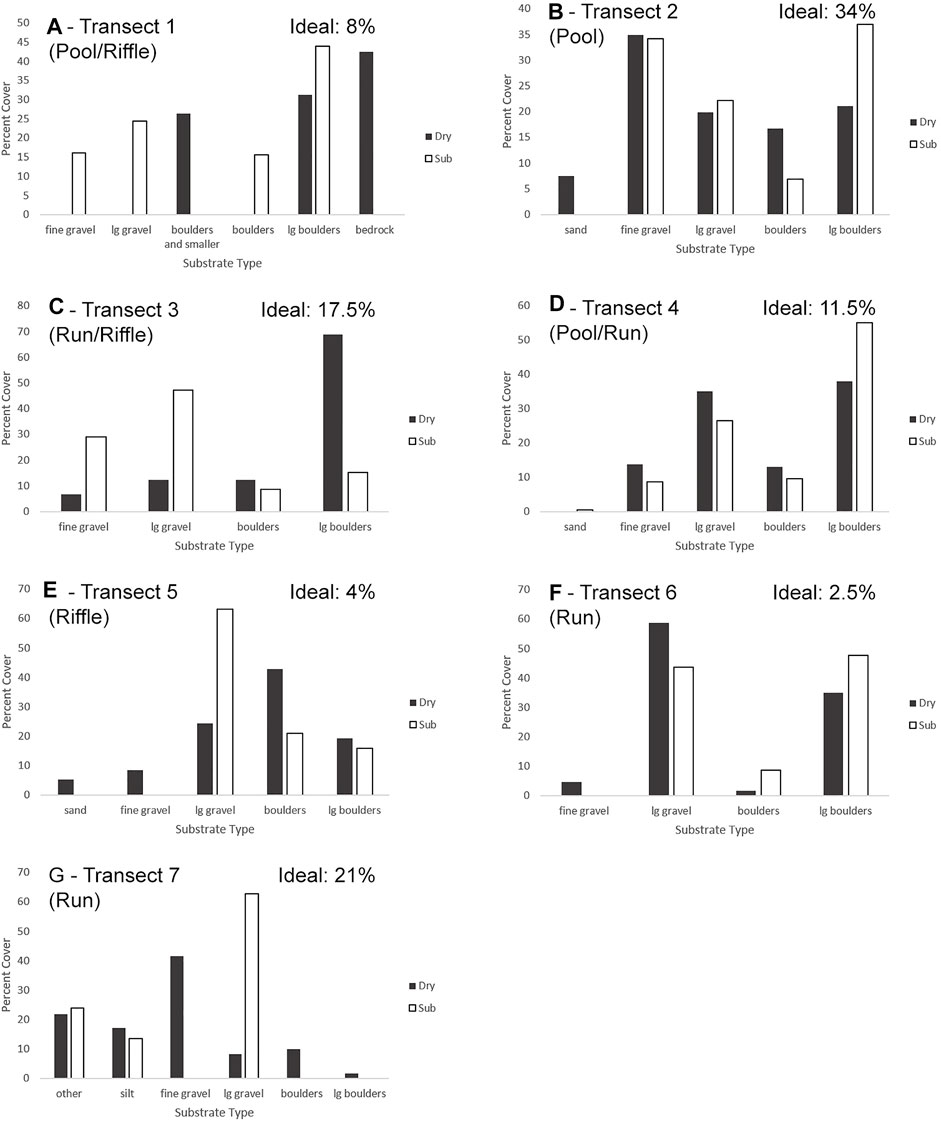
FIGURE 8. Object-based image analysis results for transects 1–7 (A–G) on both submerged and dry portions of the river, presented in percent coverage, along with the percent of ideal substrate on each transect. Dry and submerged are processed separately and % are calculated based on individual (dry or submerged) segments but presented together for comparison. Ideal substrate is the total amount of fine gravel found in the combined dry and submerged portions of the transect.
3.2.1 Accuracy assessment: Substrate texture
Dry and Submerged substrate were identified as either silt, sand, fine gravel, large gravel, boulders, large boulders, bedrock or other vegetation with an overall accuracy of 79% for the dry substrate and 86% for the submerged substrate (Table 3). Over most of the transects, submerged substrate categorization had a higher accuracy than dry substrate categorization, with submerged substrate on transect 7 categorized with complete accuracy. Only on transect 1 (pool/riffle), did the dry substrate have a higher accuracy than the submerged substrate. Within the dry substrate the highest accuracy was on transects 6 (run), and 3 (run/riffle), and the lowest accuracy was on transects 5 (riffle) and 4 (pool/run). The transect with the highest submerged substrate accuracy was 7 (run) and 6 (run), with transects 3 (run/riffle), 1 (pool/riffle) and 5 (riffle) having the lowest accuracy.
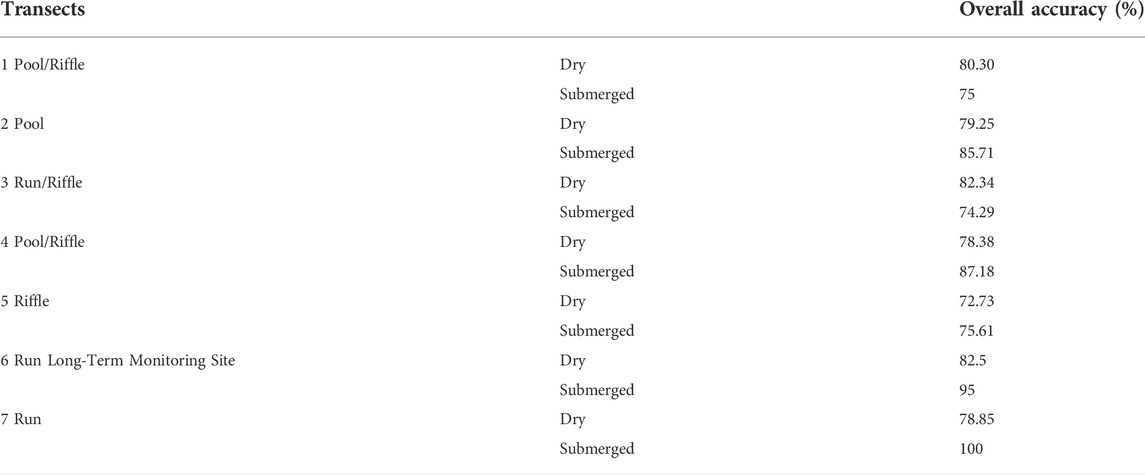
TABLE 3. Accuracy assessment conducted on the object-based imagine analysis of transects 1–7 for both dry and submerged substrate, which is the percent agreement between the training and predicted segments.
3.3 Ground truth data
An assessment was conducted to compare the ground truth data to the PCI analysis for transect 1. In this comparison, 75% of the substrate sizes matched between the two analyses. The most noticeable misclassification was that 12% of the ground truth points were classified as large boulders (>40 cm) while classified as boulders (20–40 cm) in the PCI analysis, with ∼96% of this misclassification occurring in the submerged substrate. The 4% of the misclassification within the dry substrate occurred in areas where tree branches obscured the imagery.
4 Discussion
Pools, riffles, and runs have long been used as descriptive classifications within rivers in fluvial morphology (Allan and Castillo, 2007) and biological studies (Cunjak, 1988). Descriptions vary in the literature, with use of visual assessments or unstated criteria (Jowett, 1993) and removal of runs as a category in some studies (Montgomery et al., 1999). These macro-habitat classifications have been used to assess juvenile salmon habitat (Rimmer et al., 1984), as well as partition rivers for sampling, with the assumption that physical conditions within classes are similar (Jowett, 1993). The results of this study indicate that the substrate distribution within the macro-habitat designation is highly variable. In addition, the transects do not represent consistent substrate mixes for regions of the river since adjacent transects were also highly variable in composition. Hillslope erosion and transport of boulders and thus bank slope and composition play a major role in macro-substrate generation in the river bed (Glade et al., 2019). Although many studies agree that substrate is a vital component of salmon habitat (Armstrong et al., 2003), there are surprisingly few studies focused on river substrate classification methods using UAV, with most methods relying on small scale in-situ surveys. The available studies have concentrated on general feasibility of methods and not on variation in physical characteristics which subsequently affect the applicability of survey results (Arif et al., 2017; Danhoff and Huckins, 2020).
The drone was not used to classify the distribution of riffles, runs, and pools on the larger sections of the river either by visual or image analysis methods. While this might be feasible, examination of riffle areas as spawning habitat (Malcolm et al., 2004) may be inaccurate since substrate variation is so prevalent within the macro-habitats. River restoration efforts work with a template of idealized spawning macro-habitat, but both substrate and riverbank morphology are manipulated (Van Zyll De Jong et al., 1997). In wild rivers, the present results suggest that the relationship of riffle/run designation to the potential location of redds is not certain.
4.1 Object analysis
The submerged substrate classification had an overall higher accuracy than the dry substrate classification, with the exception of the pool/riffle and run/riffle transects. Both riffle and pool transects were a concern for accuracy due to the possible distortion caused by depth in the pool transections (>70 cm) and ripples on the surface of riffles transects. To limit these effects, the drone was flown during times of low flow, as suggested by Lane (2000). The Upper Salmon River has very clear water as due to the coarse substrate, with the riverbed visible even in deeper water. These coupled factors mitigated the effects of pools, with only areas of riffles affecting accuracy. Other factors such as forest vegetation caused a lower accuracy for the dry substrate where visibility was affected by overhanging branches.
4.1.1 Effects of macro-habitat on classification accuracy
Although transect 1 ran through a transition zone of a riffle and a pool, both of which could limit visibility of the substrate, the analysis was still conducted with a 75% accuracy. When comparing the raw RGB image to the classified substrate image, it is evident that neither the riffled water nor the depth of the water obscured the image significantly. Areas of shade seemed to have the most effect on the classification, with some areas of substrate being misclassified as large gravel. In the transition zone at the tail end of a riffle, there were more large boulders rather than gravel, which matches conditions expected in a riffle, as smaller substrate would be less likely retained in high flow areas (Jowett, 1993).
Most issues in accuracy for both the dry and submerged transects stemmed from lack of training sites. As the transects covered relatively small areas of the river, substrate that was less common within the transect limited selection of appropriate training sites. The dry portion of the pool/riffle transect 1 had very little finer substrate and substrate smaller than the large boulder class was grouped. The reduction in classes correlated with an increase in accuracy of the transect which is less desirable when aggregation of classes obscures subtle changes in habitat distribution. When analyzing substrate, classes that serve different functions such as spawning suitability should not be grouped.
Riffle areas have the potential to be ideal spawning habitat, owing to swift flowing water, aeration and availability of fine substrate (Coulombe-Pontbriand and Lapointe, 2004; Fitzsimons et al., 2013). In the submerged classification of transect 3 (run/riffle), there were patches of fine gravel in both the northeast and southwest areas that would have higher water flow and smaller grain size, both characteristics of potential salmon spawning grounds (Armstrong et al., 2003). The classification had the lowest accuracy of the submerged transects (74%). All three transects through riffles had lower accuracy, possibly due to turbulence at the water surface of the riffles. The sub-classifications with the most overlap/error at this transect were boulders and large boulders, commonly in riffles, suggesting that the distortion at the water surface may have led to confusion error in OBIA. Arif et al. (2017) used similar methods to analyze substrate from UAV imagery and found that the larger grain sizes resulted in a higher number of misclassifications. They concluded that altering texture layers could help mitigate this problem, without identifying the underlying cause. We note that increased error at coarse substrate size has minimal impact on the assessment of finer substrate classes, such as the fine gravel, important in salmon spawning habitat.
Transect 7 through the run was situated in the southernmost site, the only one containing silt/mud, and submerged vegetation. The submerged area was difficult to assign training sites, since the silt/mud and other vegetation appeared murky in the water on the RGB images. Although this sediment class was more challenging to visually identify, the classification resulted in 100% accuracy, primarily because there were only two sediment classes both expressing distinctive textures. The textural segmentation could therefore distinguish the difference in bottom characteristics which were more difficult to visually designate on the RGB images. Lacking the depth of the pools and the visual distortions of the riffles, the run transect had a higher accuracy.
4.2 Spawning grounds
The preferred substrate size for Atlantic salmon spawning reported in the literature ranges from gravel as fine as 0.54 cm to as large as 10 cm (Armstrong et al., 2003). In the current study, ideal spawning substrate was based on the classifications assessed with object-based image analysis, designated fine gravel (0.2–5 cm). The pool transect 2) had the largest area of ideal spawning ground (34%), as well as the highest amount of sand. Pools are characterized by deeper water and slower currents, and the low flow in these areas leads to sedimentation of smaller substrates (Jowett, 1993). However, low flow and finer sediment may also signify lower aeration in the substrate which can affect the survival rate of eggs (Lapointe et al., 2004). Redd digging behaviour in salmon aids in the removal of smaller sediments to increase oxygen to the eggs (Everest et al., 1987), but salmon also prefer higher water velocity of 31–55 cm s−1 (Gibson, 1993), characteristically occurring in runs (Jowett, 1993). Although pools may contain the largest relative areas of ideal substrate, low flow/aeration makes them less suitable for spawning. Transition zones at the downstream end of the pools (pool/run, pool/riffle), can lead to higher water velocity at the tail end of the pool while maintaining smaller substrate deposits. These areas have been linked to spawning (Malcolm et al., 2004) and are constructed for this purpose in river restoration (Payne & Lapointe, 1997). Runs are characterized by shallow, swift water that does not break at the surface (Jowett, 1993), but are variable in water velocity and substrate size. Among the transects in this study, ideal spawning substrate (fine gravel) comprised 21% of total available substrate at on the long-term monitoring site (transect 7), and 2.5% of total available substrate in the other run, transect 6. Although transect 6 did not have much fine gravel, it contained abundant large gravel. Salmon are able to spawn in larger substrate when other factors suit their needs (Gibson, 1993), such that among substrate and flow, runs contain many suitable variables for spawning habitat (Armstrong et al., 2003). Runs have also been linked to overwintering habitat of juvenile salmon (Cunjak, 1988), creating vital habitat for the salmon at other life stages.
Riffles tend to have faster currents and are often characterized visually by a broken water surface (Jowett, 1993). Riffle transect 5 had mostly large gravel and boulders and very little ideal spawning habitat. Transect 3 had abundant large boulders, but also small patches of finer sediment typically located behind large protruding rocks, caused by a lower flow and settlement of smaller grain sizes. This resulted in an average of 17.5% of ideal spawning habitat. In the literature, riffles are considered to have good potential spawning characteristics (Malcolm et al., 2004). Although runs have the potential to be spawning habitat based on flow and depth, not much is written linking the two, with studies generally referencing riffle and pool/riffle transition zones when discussing spawning habitat (Fitzsimons et al., 2013). As stated above, transition zones between riffles/runs and pools also allow for a decrease in flow and so the settling of smaller substrate. Although riffles may not be as suitable for spawning as transition zones, a study by Bagliniere and Champigneulle (1986) determined that they were very productive locations for juvenile salmon, even compared to runs.
4.3 Comparison and application
Habitat degradation has been detrimental to Atlantic salmon populations, with declining populations throughout Europe and North American (Parrish et al., 1998).
IBoF Atlantic salmon have been strongly affected, seeing a historical decrease in returning adult salmon from 40,000 to 500 (Irvine et al., 2005). With a large habitat range, 32 of the 50 rivers located in the Inner Bay of Fundy, and limited funds, conservation efforts must be focused on rivers with high spawning potential. The common method for river habitat assessment is in-situ surveys. This method was used in parallel to the drone surveys for comparison and validation.
The drone was able to cover all seven transects within 2 days, the foot travel between locations comprising the most time. Two people were able to easily complete the drone surveys, working in unison as one flew the drone while the other focused on wilderness safety concerns by spotting birds of prey and avoiding river landings by finding suitable landing sites. In comparison, in-situ surveys took significantly longer with each transect taking a day and requiring a much larger team. In contrast the image analysis took longer to complete and was largely dependent on computing power. Where the substrate for the in-situ surveys were analyzed based on dominant substrate within the quadrats, the drone surveys determined the substrate size for any given location along the transect. While both are subject to human error, the more training data provided to the object-based image analysis, the more accurate the results.
Although both physical survey and remote sensing methods are valid, exploration into object-based image analysis using UAVs would allow for larger sections of rivers to be surveyed with higher accuracy and in shorter periods of time. As spawning ground is closely linked to substrate size, these broad-scale substrate analyses could be used to determine breeding potential of rivers, allowing for restoration efforts to be focused on rivers that have the highest spawning potential. Broad-scale substrate analyses can also be used to aid in restoration efforts that use river substrate manipulation. The addition of spawning gravel was found to successfully enhance juvenile production in rivers, improving habitat quality and increasing egg survival (Barlaup et al., 2008; Pulg et al., 2021). The addition of boulder clusters, V-dams and half-log covers has also been found to increase salmon density and biomass (de Jong and Cowx, 2016). Through the utilization of current object-based image analysis techniques coupled with high resolution photographs captured by UAVs, substrate analyses can help determine which restoration techniques (spawning gravel or boulder clusters) are needed. Although helpful for restoration efforts, these analyses can also be used to further our understanding of salmon behavior. For example, by studying what substrate is affected by ice formation, overwinter sheltering of salmon in rivers can be better understood (Linnansaari et al., 2009).
5 Conclusion
Habitat degradation is a serious threat to the river productivity, affecting all life stages of the Salmo salar and other salmonids (Einum et al., 2008). Recent efforts, including in Norway and North America, have sought to increase salmon habitat by large scale manipulation of substrate through the addition, removal and redistribution of varying substrate sizes (Fjellheim et al., 2003; Emerson, 2014; Têtu et al., 2016). Although substrate is a vital component of spawning habitat (Armstrong et al., 2003), it can also increase the fitness of other life stages, including prey availability (Mitchell et al., 1998). Van Zyll De Jong et al. (1997) suggested that the most effective way to increase the density of juvenile salmon was by manipulating substrate clusters through the addition of boulders. Current salmon habitat studies often utilize on-ground sampling of the river, relying on observational data and photographs (Malcolm et al., 2004; Louhi et al., 2008; Moir et al., 2009). Although UAVs have become more popular in recent years, very few studies have looked at their potential for substrate analysis in rivers for biological study (Arif et al., 2017). Based on our results, they emerge as a powerful tool in river mapping, especially effective in otherwise inaccessible terrain.
This study demonstrated that diversity in substrate within macro-habitat lessens the value of this crude traditional classification in categorizing spawning habitat. It also identified visibility issues in the broken water surface of riffles as a source of inaccuracy for object-based image analysis. At the same time, substrate alone does not determine spawning habitat. Pool transect 2 had by far the highest amount of ideal spawning substrate, but other conditions in pools such as flow subtract from suitability indicated by substrate. River bottom texture should be viewed as a starting point for assessing habitat suitability, adding other data layers to better quantify spawning habitat.
Data availability statement
The datasets presented in this study can be found in online repositories. The names of the repository/repositories and accession number(s) can be found below: https://dalspace.library.dal.ca/handle/10222/80,123.
Author contributions
CG led the research for her MSc thesis, conducted the GIS and analysis work, and co-wrote the article. JG received funding for the project, planned the research, participated in the field work and analysis, and co-wrote the article. CB provided expertise in substrate analysis and mapping, and edited the article. JB provided expertise in field work with the drone and assisted in GIS.
Funding
This research was funded by an Natural Sciences and Engineering Research Council Canada grant to JG as part of the NSERC-Cooke Industrial Research Chair in Sustainable Aquaculture.
Acknowledgments
This article is based on my Master’s thesis published on the Dalhousie University website at http://hdl.handle.net/10222/80123. As such I would like to thank and acknowledge the contributions of my supervisor Jonathan Grant, as well as my advisory committee, Ramon Filgueira, Paul Hill and Craig Brown. I would also like to thank and acknowledge the contributions by Kurt Samways and his crew for providing me with data and their expertise. As well as Corey Clarke, Bruce Persaud and all the folks at Fundy National Park who assisted in the field and allowed us to use their facilities.
Conflict of interest
Author CG is employed by Spiri Robotics Inc.
The remaining authors declare that the research was conducted in the absence of any commercial or financial relationships that could be construed as a potential conflict of interest.
Publisher’s note
All claims expressed in this article are solely those of the authors and do not necessarily represent those of their affiliated organizations, or those of the publisher, the editors and the reviewers. Any product that may be evaluated in this article, or claim that may be made by its manufacturer, is not guaranteed or endorsed by the publisher.
References
Allan, J. D., and Castillo, M. M. (2007). Stream ecology: Structure and function of running waters. Switzerland: Springer Science & Business Media.
Alonzo, M., Andersen, H. E., Morton, D. C., and Cook, B. D. (2018). Quantifying boreal forest structure and composition using UAV structure from motion. Forests 9 (3), 119. doi:10.3390/f9030119
Amiro, P. G., Brazner, J. C., and Giorno, J. L. (2009). Assessment of the recovery potential for the Atlantic salmon designatable unit inner Bay of Fundy: Threats. Fisheries and Oceans Canada. Ottawa, ON (Canada): Science
Arif, M. S. M., Gülch, E., Tuhtan, J. A., Thumser, P., and Haas, C. (2017). An investigation of image processing techniques for substrate classification based on dominant grain size using RGB images from UAV. Int. J. Remote Sens. 38 (8-10), 2639–2661. doi:10.1080/01431161.2016.1249309
Armstrong, J. D., Kemp, P. S., Kennedy, C. J., Ladle, M., and Milner, N. J. (2003). Habitat requirements of Atlantic salmon and brown trout in rivers and streams. Fish. Res. 62, 143–170. doi:10.1016/S0165-7836(02)00160-1
Baglinière, J. L., and Champigneulle, A. (1986). Population estimates of juvenile Atlantic salmon, Salmo salar, as indices of smolt production in the R. Scorff, Brittany. J. Fish. Biol. 29 (4), 467–482. doi:10.1111/j.1095-8649.1986.tb04962.x
Barlaup, B. T., Gabrielsen, S. E., Skoglund, H., and Wiers, T. (2008). Addition of spawning gravel—A means to restore spawning habitat of atlantic salmon (Salmo salar L.), and anadromous and resident brown trout (Salmo trutta L.) in regulated rivers. River Res. Appl. 24, 543–550. doi:10.1002/rra.1127
Borsányi, P., Alfredsen, K., Harby, A., Ugedal, O., and Kraxner, C. (2004). A meso-scale habitat classification method for production modelling of Atlantic salmon in Norway. Hydroecol. Appl. 14, 119–138. doi:10.1051/hydro:2004008
Brown, C. J., Smith, S. J., Lawton, P., and Anderson, J. T. (2011). Benthic habitat mapping: A review of progress towards improved understanding of the spatial ecology of the seafloor using acoustic techniques. Estuar. Coast. Shelf Sci. 92 (3), 502–520. doi:10.1016/j.ecss.2011.02.007
Buisson, L., Blanc, L., and Grenouillet, G. (2008). Modelling stream fish species distribution in a river network: The relative effects of temperature versus physical factors. Ecol. Freshw. Fish. 17 (2), 244–257. doi:10.1111/j.1600-0633.2007.00276.x
Clarke, C. N., Fraser, D. J., and Purchase, C. F. (2016). Lifelong and carry‐over effects of early captive exposure in a recovery program for Atlantic salmon (Salmo salar). Anim. Conserv. 19 (4), 350–359. doi:10.1111/acv.12251
Coulombe‐Pontbriand, M., and Lapointe, M. (2004). Geomorphic controls, riffle substrate quality, and spawning site selection in two semi‐alluvial salmon rivers in the Gaspé Peninsula, Canada. River Res. Appl. 20 (5), 577–590. doi:10.1002/rra.768
Cunjak, R. A. (1988). Behavior and microhabitat of young Atlantic salmon (Salmo salar) during winter. Can. J. Fish. Aquat. Sci. 45 (12), 2156–2160. doi:10.1139/f88-250
Cunliffe, A. M., Brazier, R. E., and Anderson, K. (2016). Ultra-fine grain landscape-scale quantification of dryland vegetation structure with drone-acquired structure-from-motion photogrammetry. Remote Sens. Environ. 183, 129–143. doi:10.1016/j.rse.2016.05.019
Dadswell, M. J. (1968). Atlantic Salmon (Salmo salar) investigations in the point wolfe and upper Salmon rivers, Fundy national park. Ottawa, ON (Canada): Canadian Wildlife Service.
Danhoff, B. M., and Huckins, C. J. (2020). Modelling submerged fluvial substrates with structure‐from‐motion photogrammetry. River Res. Appl. 36 (1), 128–137. doi:10.1002/rra.3532
Davey, C., and Lapointe, M. (2007). Sedimentary links and the spatial organization of Atlantic salmon (Salmo salar) spawning habitat in a Canadian Shield river. Geomorphology 83 (1-2), 82–96. doi:10.1016/j.geomorph.2006.06.011
de Jong, M. V. Z., and Cowx, I. G. (2016). Long-term response of salmonid populations to habitat restoration in a boreal forest stream. Ecol. Eng. 91, 148–157. doi:10.1016/j.ecoleng.2016.02.029
Department of Fisheries and Oceans Canada (2010). “Recovery strategy for the atlantic salmon (Salmo salar), inner Bay of Fundy populations [final],” in Species at risk act recovery strategy series (Ottawa, Ontario: Fisheries and Oceans Canada).
Dubuc, L. J., Krohn, W. B., and Owen, R. B. (1990). Predicting occurrence of river otters by habitat on Mount Desert Island, Maine. J. Wildl. Manag. 54, 594–599. doi:10.2307/3809355
Einum, S., Nislow, K. H., Reynolds, J. D., and Sutherland, W. J. (2008). Predicting population responses to restoration of breeding habitat in Atlantic salmon. J. Appl. Ecol. 45 (3), 930–938. doi:10.1111/j.1365-2664.2008.01464.x
Elith, J., and Leathwick, J. R. (2009). Species distribution models: Ecological explanation and prediction across space and time. Annu. Rev. Ecol. Evol. Syst. 40, 677–697. doi:10.1146/annurev.ecolsys.110308.120159
Emerson, P. W. (2014). In-stream habitat enhancement for juvenile atlantic salmon in the little main restigouche river, New Brunswick. Fredericton, NB (Canada): Doctoral dissertation, University of New Brunswick.
Everest, F. H., Beschta, R. L., Scrivener, J. C., Koski, K. V., Sedell, J. R., and Cederholm, C. J. (1987). “Fine sediment and salmonid production: A paradox,” in Streamside management and forestry and fishery interactions. Editors E. O. Salo, and T. W. Cundy (College of forest resources University of Washington), 89–142.
Finstad, A. G., Einum, S., Forseth, T., and Ugedal, O. (2007). Shelter availability affects behaviour, size‐dependent and mean growth of juvenile Atlantic salmon. Freshw. Biol. 52 (9), 1710–1718. doi:10.1111/j.1365-2427.2007.01799.x
Fitzsimons, J. D., Dalton, A., MacVeigh, B., Heaton, M., Wilson, C., and Honeyfield, D. C. (2013). Use of stable isotopes to identify redds of putative hatchery and wild Atlantic Salmon and evaluate their spawning habitat and egg thiamine status in a Lake Ontario tributary. North Am. J. Fish. Manag. 33 (4), 741–753. doi:10.1080/02755947.2013.806380
Fjellheim, A., Barlaup, B. T., Gabrielsen, S. E., and Raddum, G. G. (2003). Restoring fish habitat as an alternative to stocking in a river with strongly reduced flow. Int. J. Ecohydrol. Hydrobiology 3 (1), 17. https://www.researchgate.net/publication/282674631_Restoring_fish_habitat_as_an_alternative_to_stocking_in_a_river_with_strongly_reduced_flow.
Forsmoo, J., Anderson, K., Macleod, C. J., Wilkinson, M. E., and Brazier, R. (2018). Drone‐based structure‐from‐motion photogrammetry captures grassland sward height variability. J. Appl. Ecol. 55 (6), 2587–2599. doi:10.1111/1365-2664.13148
Garman, G. C., and Moring, J. R. (1991). Initial effects of deforestation on physical characteristics of a boreal river. Hydrobiologia 209 (1), 29–37. doi:10.1007/BF00006715
Gibson, R. J. (1993). The atlantic salmon in fresh water: Spawning, rearing and production. Rev. Fish. Biol. Fish. 3 (1), 39–73. doi:10.1007/BF00043297
Hamilton, S. K. (2002). Human impacts on hydrology in the Pantanal wetland of South America. Water Sci. Technol. 45 (11), 35–44. doi:10.2166/wst.2002.0377
Heggenes, J. (1990). Habitat utilization and preferences in juvenile Atlantic salmon (Salmo salar) in streams. Regul. Rivers Res. Mgmt. 5 (4), 341–354. doi:10.1002/rrr.3450050406
Heggenes, J., Saltveit, S. J., and Lingaas, O. (1996). Predicting fish habitat use to changes in water flow: Modelling critical minimum flows for atlantic salmon, Salmo salar, and brown trout, S. trutta. Regul. Rivers Res. Mgmt. 12 (2‐3), 331–344. doi:10.1002/(sici)1099-1646(199603)12:2/3<331::aid-rrr399>3.0.co;2-e
Hill, N. L., Trueman, J. R., Prévost, A. D., Fraser, D. J., Ardren, W. R., and Grant, J. W. (2019). Effect of dam removal on habitat use by spawning Atlantic salmon. J. Gt. Lakes. Res. 45 (2), 394–399. doi:10.1016/j.jglr.2019.01.002
Hilton, J., O'Hare, M., Bowes, M. J., and Jones, J. I. (2006). How green is my river? A new paradigm of eutrophication in rivers. Sci. total Environ. 365 (1-3), 66–83. doi:10.1016/j.scitotenv.2006.02.055
Holbrook, S. J., and Schmitt, R. J. (2002). Competition for shelter space causes density-dependent predation mortality in damselfishes. Ecology 83, 2855–2868. doi:10.1890/0012-9658(2002)083
Horton, A. J., Constantine, J. A., Hales, T. C., Goossens, B., Bruford, M. W., and Lazarus, E. D. (2017). Modification of river meandering by tropical deforestation. Geology 45 (6), 511–514. doi:10.1130/G38740.1
Hossain, M. D., and Chen, D. (2019). Segmentation for object-based image analysis (OBIA): A review of algorithms and challenges from remote sensing perspective. ISPRS J. Photogrammetry Remote Sens. 150, 115–134. doi:10.1016/j.isprsjprs.2019.02.009
Iglhaut, J., Cabo, C., Puliti, S., Piermattei, L., O’Connor, J., and Rosette, J. (2019). Structure from motion photogrammetry in forestry: A review. Curr. For. Rep. 5 (3), 155–168. doi:10.1007/s40725-019-00094-3
Irvine, J. R., Gross, M. R., Wood, C. C., Holtby, L. B., Schubert, N. D., and Amiro, P. G. (2005). Canada's Species at Risk Act: An opportunity to protect endangered salmon. Fisheries 30 (12), 11–19. doi:10.1577/1548-8446(2005)30[11
Jonsson, N., Hansen, L. P., and Jonsson, B. (1991). Variation in age, size and repeat spawning of adult Atlantic salmon in relation to river discharge. J. Animal Ecol. 60, 937–947. doi:10.2307/5423
Jowett, I. G. (1993). A method for objectively identifying pool, run, and riffle habitats from physical measurements. N. Z. J. Mar. Freshw. Res. 27 (2), 241–248. doi:10.1080/00288330.1993.9516563
Lane, S. N. (2000). The measurement of river channel morphology using digital photogrammetry. Photogrammetric Rec. 16 (96), 937–961. doi:10.1111/0031-868X.00159
Lapointe, M. F., Bergeron, N. E., Bérubé, F., Pouliot, M. A., and Johnston, P. (2004). Interactive effects of substrate sand and silt contents, redd-scale hydraulic gradients, and interstitial velocities on egg-to-emergence survival of Atlantic salmon (Salmo salar). Can. J. Fish. Aquat. Sci. 61 (12), 2271–2277. doi:10.1139/f04-236
Leduc, P., Peirce, S., and Ashmore, P. (2019). Short communication: Challenges and applications of structure-from-motion photogrammetry in a physical model of a braided river. Earth Surf. Dynam. 7 (1), 97–106. doi:10.5194/esurf-7-97-2019
Linnansaari, T., Alfredsen, K., Stickler, M., Arnekleiv, J. V., Harby, A., and Cunjak, R. A. (2009). Does ice matter? Site fidelity and movements by atlantic salmon (Salmo salar L.) parr during winter in a substrate enhanced river reach. River Res. Appl. 25 (6), 773–787. doi:10.1002/rra.1190
Louhi, P., Mäki‐Petäys, A., and Erkinaro, J. (2008). Spawning habitat of atlantic salmon and brown trout: General criteria and intragravel factors. River Res. Appl. 24 (3), 330–339. doi:10.1002/rra.1072
Malcolm, I. A., Soulsby, C., Youngson, A. F., Hannah, D. M., McLaren, I. S., and Thorne, A. (2004). Hydrological influences on hyporheic water quality: Implications for salmon egg survival. Hydrol. Process. 18 (9), 1543–1560. doi:10.1002/hyp.1405
Marsh, J. E., Lauridsen, R. B., Gregory, S. D., Beaumont, W. R., Scott, L. J., Kratina, P., et al. (2020). Above parr: Lowland river habitat characteristics associated with higher juvenile Atlantic salmon (Salmo salar) and brown trout (S. trutta) densities. Ecol. Freshw. Fish. 29 (4), 542–556. doi:10.1111/eff.12529
Marteau, B., Vericat, D., Gibbins, C., Batalla, R. J., and Green, D. R. (2017). Application of Structure-from-Motion photogrammetry to river restoration. Earth Surf. Process. Landf. 42 (3), 503–515. doi:10.1002/esp.4086
McCoy, E. D., and Bell, S. S. (1991). “Habitat structure: The evolution and diversification of a complex topic,” in Habitat structure (Dordrecht: Springer), 3–27. doi:10.1007/978-94-011-3076-9_1
Mills, D. (1989). Ecology and management of Atlantic salmon. London: Springer Science & Business Media.
Mitchell, J., McKinley, R. S., Power, G., and Scruton, D. A. (1998). Evaluation of Atlantic salmon parr responses to habitat improvement structures in an experimental channel in Newfoundland, Canada. Regul. Rivers Res. Manag. An Int. J. Devoted River Res. Manag. 14 (1), 25–39. doi:10.1002/(SICI)1099-1646(199801/02)14:1%3C25::AID-RRR474%3E3
Moir, H. J., Gibbins, C. N., Buffington, J. M., Webb, J. H., Soulsby, C., Brewer, M. J., et al. (2009). A new method to identify the fluvial regimes used by spawning salmonidsPHABSIM modelling of atlantic salmon spawning habitat in an upland stream: Testing the influence of habitat suitability indices on model output. River Res. Appl.River Res. Appl. 6621 (99), 14041021–14081034. doi:10.1139/F09-136Moir10.1002/rra.869
Montgomery, D. R., Beamer, E. M., Pess, G. R., and Quinn, T. P. (1999). Channel type and salmonid spawning distribution and abundance. Can. J. Fish. Aquat. Sci. 56 (3), 377–387. doi:10.1139/f98-181
Parrish, D. L., Behnke, R. J., Gephard, S. R., McCormick, S. D., and Reeves, G. H. (1998). Why aren't there more Atlantic salmon (Salmo salar)? Can. J. Fish. Aquat. Sci. 55 (S1), 281–287. doi:10.1139/d98-012
Payne, B. A., and Lapointe, M. F. (1997). Channel morphology and lateral stability: Effects on distribution of spawning and rearing habitat for atlantic salmon in a wandering cobble-bed river. Can. J. Fish. Aquat. Sci. 54 (11), 2627–2636. doi:10.1139/f97-171
Pulg, U., Lennox, R. J., Stranzl, S., Espedal, O. E., Gabrielsen, S. E., Wiers, T., et al. (2021). Long-term effects and cost-benefit analysis of eight spawning gravel augmentations for Atlantic salmon and Brown trout in Norway. Hydrobiologia 849, 485–507. doi:10.1007/s10750-021-04646-2
Rimmer, D. M., Paim, U., and Saunders, R. L. (1984). Changes in the selection of microhabitat by juvenile Atlantic salmon (Salmo salar) at the summer–autumn transition in a small river. Can. J. Fish. Aquat. Sci. 41 (3), 469–475. doi:10.1139/f84-056
Rushton, S. P., Hill, D., and Carter, S. P. (1994). The abundance of river corridor birds in relation to their habitats: A modelling approach. J. Appl. Ecol. 31, 313–328. doi:10.2307/2404546
Rusnák, M., Sládek, J., Kidová, A., and Lehotský, M. (2018). Template for high-resolution river landscape mapping using UAV technology. Measurement 115, 139–151. doi:10.1016/j.measurement.2017.10.023
Saska, M., Krajník, T., Faigl, J., Vonásek, V., and Přeučil, L. (20122012). Low cost mav platform ar-drone in experimental verifications of methods for vision based autonomous navigation.” in IEEE/RSJ international conference on intelligent Robots and systems, 4808–4809. doi:10.1109/IROS.2012.6386277
Schlosser, I. J. (1987). “A conceptual framework for fish communities in small warmwater streams,” in Community and evolutionary ecology of North American stream fishes. Editors W. J. Matthews, and D. C. Heins (Norman: University of Oklahoma Press), 17
Schneider, K. N., and Winemiller, K. O. (2008). Structural complexity of woody debris patches influences fish and macroinvertebrate species richness in a temperate floodplain-river system. Hydrobiologia 610 (1), 235–244. doi:10.1007/s10750-008-9438-5
Tang, L., and Shao, G. (2015). Drone remote sensing for forestry research and practices. J. For. Res. (Harbin). 26 (4), 791–797. doi:10.1007/s11676-015-0088-y
Têtu, C., Mitchell, S., MacInnis, C., and Taylor, B. R. (2016). Restoration of a Nova Scotia stream to enhance Atlantic salmon provides few benefits to benthic invertebrates. Proc. N. S. Inst. Sci. 48 (2), 211. doi:10.15273/pnsis.v48i2.6656
Thorstad, E. B., Økland, F., Aarestrup, K., and Heggberget, T. G. (2008). Factors affecting the within-river spawning migration of Atlantic salmon, with emphasis on human impacts. Rev. Fish. Biol. Fish. 18 (4), 345–371. doi:10.1007/s11160-007-9076-4
Trzcinski, M. K., Gibson, A. J. F., Amiro, P. G., Randall, R. G., and Burlington, O. (2004). "Inner Bay of Fundy Atlantic salmon (Salmo salar) critical habitat case study," in Science advisory secretariat research document 2004/114. Ottawa, ON (Canada): Department of Fisheries and Oceans Canada.
Vadas, R. L., and Orth, D. J. (1998). Use of physical variables to discriminate visually determined mesohabitat types in North American streams. Rivers-Fort Collins 6, 143
Van Zyll De Jong, M. C., Cowx, I. G., and Scruton, D. A. (1997). An evaluation of instream habitat restoration techniques on salmonid populations in a Newfoundland stream. Regul. Rivers Res. Manag. an Int. J. Devoted River Res. Manag. 13 (6), 603–614. doi:10.1002/(SICI)1099-1646(199711/12)13:6%3C603::AID-RRR487%3E3.0
Ward, T. A., Tate, K. W., Atwill, E. R., Lile, D. F., Lancaster, D. L., McDougald, N., et al. (2003). A comparison of three visual assessments for riparian and stream health. J. Soil Water Conservation 58 (2), 83. https://www.researchgate.net/publication/240613740_A_Comparison_of_Three_Visual_Assessments_for_Riparian_and_Stream_Health.
Westoby, M. J., Brasington, J., Glasser, N. F., Hambrey, M. J., and Reynolds, J. M. (2012). ‘Structure-from-Motion’photogrammetry: A low-cost, effective tool for geoscience applications. Geomorphology 179, 300–314. doi:10.1016/j.geomorph.2012.08.021
Willis, S. C., Winemiller, K. O., and Lopez-Fernandez, H. (2005). Habitat structural complexity and morphological diversity of fish assemblages in a Neotropical floodplain river. Oecologia 142 (2), 284–295. doi:10.1007/s00442-004-1723-z
Wohl, E. (2005). Compromised rivers: Understanding historical human impacts on rivers in the context of restoration. Ecol. Soc. 10 (2), 202. doi:10.5751/es-01339-100202
Woodget, A. S., Austrums, R., Maddock, I. P., and Habit, E. (2017). Drones and digital photogrammetry: From classifications to continuums for monitoring River Habitat and hydromorphology. WIREs Water 4 (4), e1222. doi:10.1002/wat2.1222
Woodget, A. S., Carbonneau, P. E., Visser, F., and Maddock, I. P. (2015). Quantifying submerged fluvial topography using hyperspatial resolution UAS imagery and structure from motion photogrammetry. Earth Surf. Process. Landf. 40 (1), 47–64. doi:10.1002/esp.3613
Keywords: salmon, remote sensing, river habitat, substrate mapping, habitat mapping, UAV, object based image analysis, structure from motion
Citation: Giroux C, Grant J, Brown CJ and Barrell J (2022) Remote sensing of river habitat for salmon restoration. Front. Remote Sens. 3:993575. doi: 10.3389/frsen.2022.993575
Received: 13 July 2022; Accepted: 08 August 2022;
Published: 02 September 2022.
Edited by:
David Richard Green, University of Aberdeen, United KingdomReviewed by:
Borbala Hortobagyi, UMR5600 Environnement Ville Societe (EVS), FranceMohammed Oludare Idrees, University of Ilorin, Nigeria
Copyright © 2022 Giroux, Grant, Brown and Barrell. This is an open-access article distributed under the terms of the Creative Commons Attribution License (CC BY). The use, distribution or reproduction in other forums is permitted, provided the original author(s) and the copyright owner(s) are credited and that the original publication in this journal is cited, in accordance with accepted academic practice. No use, distribution or reproduction is permitted which does not comply with these terms.
*Correspondence: Chantal Giroux, chantal.giroux11@gmail.com