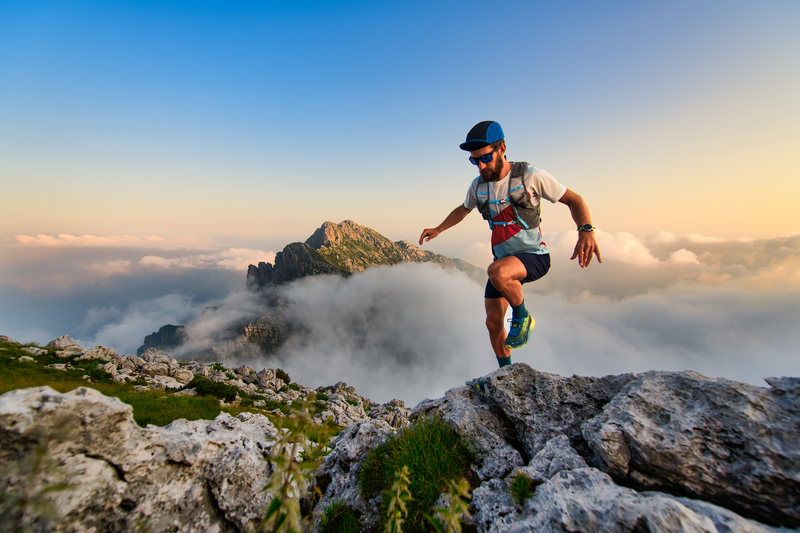
94% of researchers rate our articles as excellent or good
Learn more about the work of our research integrity team to safeguard the quality of each article we publish.
Find out more
ORIGINAL RESEARCH article
Front. Remote Sens. , 11 May 2022
Sec. Atmospheric Remote Sensing
Volume 3 - 2022 | https://doi.org/10.3389/frsen.2022.887456
This article is part of the Research Topic Anthropogenic Emission Monitoring with the Copernicus CO2 Monitoring Mission View all 8 articles
The European Copernicus programme plans to install a constellation of multiple polar orbiting satellites (Copernicus Anthropogenic CO2 Monitoring Mission, CO2M mission) for observing atmospheric CO2 content with the aim to estimate fossil fuel CO2 emissions. We explore the impact of potential CO2M observations of column-averaged CO2 (XCO2), nitrogen dioxide (NO2), and aerosols in a 200 × 200 km2 domain around Berlin. For the quantification of anticipated XCO2 random and systematic errors we developed and applied new error parameterisation formulae based on artificial neural networks. For the interpretation of these data, we further established a CCFFDAS modelling chain from parameters of emission models to XCO2 and NO2 observations to simulate the 24 h periods preceeding simulated CO2M overpasses over the study area. For one overpass in winter and one in summer, we present a number of assessments of observation impact in terms of the posterior uncertainty in fossil fuel emissions on scales ranging from 2 to 200 km. This means the assessments include temporal and spatial scales typically not covered by inventories. The assessments differentiate the fossil fuel CO2 emissions into two sectors, an energy generation sector (power plants) and the complement, which we call “other sector.” We find that combined measurements of XCO2 and aerosols provide a powerful constraint on emissions from larger power plants; the uncertainty in fossil fuel emissions from the largest three power plants in the domain was reduced by 60%–90% after assimilating the observations. Likewise, these measurements achieve an uncertainty reduction for the other sector that increases when aggregated to larger spatial scales. When aggregated over Berlin the uncertainty reduction for the other sector varies between 28% and 48%. Our assessments show a considerable contribution of aerosol observations onboard CO2M to the constraint of the XCO2 measurements on emissions from all power plants and for the other sector on all spatial scales. NO2 measurements onboard CO2M provide a powerful additional constraint on the emissions from power plants and from the other sector. We further apply a Jacobian representation of the CCFFDAS modelling chain to decompose a simulated CO2 column in terms of spatial emission impact. This analysis reveals the complex structure of the footprint of an observed CO2 column, which indicates the limits of simple mass balances approaches for interpretation of such observations.
In the resolution of the COP26 Climate Summit in Glasgow 2021, known as the Glasgow Climate Pact, the historic decision of phasing out fossil fuel emissions was taken. This is in support of the 2015 Paris Agreement (UNFCCC, 2015) where the Parties have agreed to reduce global emissions of greenhouse gases in order to reduce global warming to below 2°C. As part of this agreement the implementation of a transparency framework which requires the regular reporting of national greenhouse gas inventories is foreseen. In the 2019 refinement (Calvo Buendia et al., 2019) of their guidelines for national greenhouse gas inventories (Eggleston et al., 2006), the Intergovernmental Panel on Climate Change proposes to put into practice a system supporting the emissions inventory reporting by an independent approach based on atmospheric observations and inverse modelling.
The European Commission’s Copernicus programme is preparing an operational Monitoring and Verification Support (MVS) capacity with a constellation of multiple polar orbiting satellitesm, i.e. CO2M, as an essential component (Janssens-Maenhout et al., 2020). CO2M is planned with imaging capability sampling XCO2 at a resolution of 4 km2 (ESA, 2020). The goal of the MVS capacity is to derive anthropogenic CO2 emissions both at the national scale as well as the scale of megacities and for certain emission hot spots (Pinty et al., 2017). The systematic attribution of such atmospheric concentration data to specific emission sources is a complex task (Balsamo et al., 2021) calling for comprehensive inverse modelling systems, which ideally include component models that simulate fossil fuel emissions and biogenic fluxes (see, e.g., Ciais et al., 2015).
To date, the most widespread tool for quantitative interpretation of atmospheric concentration measurements are atmospheric transport inversions (Enting, 2002). At the city scale, one of the pioneering experiments to estimate greenhouse gas fluxes is the Indianapolis Flux Experiment (INFLUX), based mainly on a surface measurement network including continuous measurements from tall towers, flask samples, eddy-flux towers, surface-based TCCON-FTS instruments, as well as regular aircraft sampling of greenhouse gases (Davis et al., 2017). Using this observational network, Lauvaux et al. (2016) inferred fossil CO2 emissions at km-scale spatial resolution from an atmospheric inverse modelling system. Turnbull et al. (2019) compared emission estimates of CO2 from an inventory-based method with two different atmospheric inversion systems and found an agreement among these methods within 7% for whole-city fossil fuel CO2 emission, when taking into account additional proxy tracers for fossil fuel emissions such as radiocarbon in the inversions. However, these estimates have been derived only for the dormant season with minimum influence by CO2 exchange fluxes from terrestrial biosphere activities.
In addition to XCO2 measurements, CO2M will also observe the atmospheric aerosol load as well as the atmospheric NO2 column (ESA, 2020). While aerosol observations help to reduce uncertainties in the retrieved XCO2 product (Houweling et al., 2019; Rusli et al., 2021), NO2 is co-emitted with fossil fuel CO2 and its atmospheric concentration carries information about the processes behind fossil fuel emissions, their locations and strengths. Reuter et al. (2019) demonstrated the use of co-located observations of XCO2 by OCO-2 and of the NO2 column by S5P to estimate the CO2 flux through a plume from its cross section and the wind speed. In the context of CO2M, the SMARTCARB (Satellite Measurements of Auxiliary Reactive Trace gases for fossil fuel CARBon dioxide emission estimation) study combined a plume detection algorithm with a data-driven mass-balance approach to estimate CO2 emissions of cities and power plants showing the usefulness of complementary NO2 observations for constraining hot spot emissions (Brunner et al., 2019; Kuhlmann et al., 2019; Kuhlmann et al., 2020; Kuhlmann et al., 2021). Based on a data set of simulated random and systematic errors to be expected in XCO2 retrievals from CO2M measurements (Buchwitz et al., 2013) (in the following called level 2 error files and abbreviated L2e files), a study by Wang et al. (2020) assessed the capability of CO2M to quantify fossil emissions from emissions hotspots and megacities. Technically, they applied the quantitative network design approach (Kaminski and Rayner, 2017) to backpropagate these simulated observational uncertainties to uncertainties in surface fluxes. To enhance the computational efficiency of their underlying transport inversion system, the atmospheric transport was approximated by a Gaussian plume model. One of their recommendations is to construct “integrated inversion systems that exploit multiple types of measurements”.
Exactly this strategy is pursued by the Carbon Cycle Fossil Fuel Data Assimilation System (CCFFDAS) approach. By combining models of atmospheric transport and of surface emissions it is capable of integrating a range of diverse data streams as observational constraints. The step from a transport inversion to such a comprehensive CCFFDAS has considerable impact on the layout of the inverse problem in at least two respects. First, while the transport inversion directly solves for an unknown flux field, the CCFFDAS solves for a combination of process parameters as well as initial- and boundary conditions of the process modules and simulates surface fluxes in a subsequent step. Second, while the flux field derived by the transport inversion relies to a large extent on a regularisation of the inverse problem through external prior information in the flux space, the CCFFDAS achieves this regularisation through process understanding incorporated in the fossil and biogenic modules. These changes in the layout of the inverse problem are expected to significantly impact the interpretation of the CO2M data.
A first Carbon Cycle Fossil Fuel Data Assimilation System was developed and applied by Kaminski et al. (2022) to assess the added value of CO2M for quantifying sectoral fossil fuel CO2 emissions of countries at global scale. For this global domain it was, however, impossible to achieve a resolution of the CCFFDAS modelling chain (in particular of its atmospheric transport component) as fine as the 4 km2 resolution of CO2M. The current study presents a new version of CCFFDAS that operates on the CO2M resolution, but at the cost of a spatially limited modelling domain. At this resolution, CCFFDAS can be used to quantify emissions of cities and point sources such as power plants; also one of the objectives of the planned MVS capability. The objectives of this study are:
1. To present a prototype of this regional CCFFDAS at the native resolution of the XCO2 observations and demonstrate its functioning using a domain centred around a megacity, in our case Berlin, which includes a large number of fossil fuel power plants,
2. To illustrate the usefulness of a Jacobian representation of modelling chain for interpretation of the atmospheric measurements,
3. To assess the capability of CO2M to constrain fossil fuel CO2 emissions of the city and the surrounding area including the point sources (i.e., power plants),
4. To assess the added value of NO2 column observations complementing XCO2 measurements and the role of spatial differentiation in the ratio of emission factors for NO2 and CO2, and
5. To assess the added value of simultaneous measurements of the atmospheric aerosol load.
We set up a CCFFDAS modelling chain to simulate two CO2M XCO2 images over the Berlin area, one on 3 February 2008 and one on 3 July 2008, starting 24 h before the respective acquisitions. The flow of information in the forward sense is shown in Figure 1. The CO2M observation impact is assessed through the quantitative network design approach that is described in Section 2.6. This approach is based on a representation of the modelling chain through a Jacobian matrix that quantifies the sensitivity of the measurements as a function of the control vector (Section 3). Our control vector consists of the surface emissions and the lateral inflow of CO2 as well as scaling factors of the NO2/CO2 emission ratio. Our 24 h simulation period is sufficiently long to ensure that the initial concentration has left our 200 × 200 km2 domain under typical wind conditions, i.e., we can safely ignore it in the control vector. The components of the modelling chain are presented in the subsequent subsections.
To link the emission models to XCO2 and NO2 observations by CO2M we use version 5.2.1 of the Community Multiscale Air Quality model (Zenodo, 2018), which can be run as an offline tracer transport model with meteorological input fields derived from the Weather and Research Forecast model (WRF, version 3.9.1.1, Skamarock et al., 2008). Our model domain of 200 km by 200 km around Berlin is shown in Figure 2. Over this domain we operate the model at a horizontal resolution of 2 km by 2 km with 32 vertical layers. This domain was the inner-most of four WRF domains with resolutions of 54, 18, 6 and 2 km, using one-way nesting (i.e., no feedback from finer to coarser domains). The WRF simulations were configured as a series of short forecasts each spanning 24 h, initialised with 12 h of spin-up. Initial and boundary conditions were derived from the ECMWF’s ERA-Interim reanalysis product (at 6-hourly, 0.75° resolution), with higher-resolution sea-surface temperatures from the “real-time global” SST archive from NCEP (https://polar.ncep.noaa.gov/sst/ophi/; at daily, 1/12° resolution). The outer domain of the WRF grid was forced with the ERA-Interim analysis via grid-nudging above the boundary layer. Key physics parameterisations are given in Supplementary Table S8. Note that the cumulus parameterisation was switched off for the inner-most domain, which was run at a 2 km spatial resolution.
A subsequent processing step is the mapping from the CMAQ grid to the locations of successful CO2M retrievals, for which the L2e files provide the centre coordinates of the respective pixels. This mapping used the two CMAQ grid cells with their centres within a 2 km radius from the centre of the retrieval pixel, weighted in inverse proportion to the distance of their CMAQ grid centres to the centre of the retrieval pixel. For the XCO2 computation we assume a uniform averaging kernel with values of 1, which is a good approximation for cloud-free conditions that is valid over all seasons, see Figure 6 of Buchwitz et al. (2013). The averaging kernel for NO2 is based on the TROPOMI NO2 retrieval (Eskes et al., 2019), and is valid for clear sky retrievals. To cover summer and winter conditions, we used the average of all averaging kernels on two orbits in July 2018, between 30 and 65° latitude in both hemispheres. The averaging kernel is zero in the stratosphere, because this is a tropospheric NO2 product.
For the time scales addressed in the present study, CO2 is considered chemically inert. By contrast, the loss of NO2 due to chemical processes needs to be taken into account. The main reaction determining the lifetime of NO2 in the lower troposphere is its oxidation by the hydroxyl radical into nitric acid, as represented by the following chemical reaction,
in which M is the molecular density of air, and Kno2oh the pressure and temperature dependent reaction rate constant. As other studies (see, e.g., Beirle et al., 2011) we focus on this OH reaction and neglect the formation of organic nitrates and peroxyacyl nitrates (PANs) as well as the shift in the photostationary balance between NO and NO2 (as influenced by O3). Beirle et al. (2011) provides typical NO2 lifetimes of 4 h during summer and 8 h during winter for the 12:45 UTC local overpass time of OMI at mid latitudes. However, the chemical transformation of NO2 to nitric acid varies with the diurnally and seasonally varying hydroxyl abundance, air temperature and pressure. To take these spatio-temporal variations into account for the target days in 2008, we make use of hydroxyl radical distributions, temperature, and pressure from the CAMS reanalysis (Flemming et al., 2015). The reanalysis data is available at 3 hourly interval, 9 × 9 km2 horizontal resolution and 60 vertical hybrid sigma-pressure levels from the surface to the top of the atmosphere. The coefficients for the reaction rate constant, combining the high and low pressure limits, are taken from Atkinson et al. (2004). The chemical lifetime of NO2 due to Eq. 1 is derived as follows,
with [OH] being the number density of the hydroxyl radical in molec./cm3. Supplementary Figure S1 shows the expected shorter lifetimes during summer than during winter over our study area. Large variations in lifetime are found, which are explained in part by the presence of clouds, attenuating the UV irradiance below the cloud base. A strong diurnal cycle is seen also, with the longest lifetimes in the early morning during winter before sun rise. During summer, the mean values around noon correspond reasonably well with the earlier mentioned estimates from Beirle et al. (2011). During winter, however, substantially longer lifetimes are found in the range of 20 and 50 h around noon.
We simulated NO2 emissions over 24 h before the overpass around 11:00 UTC. The urban NO2 plume measured by CO2M represents emissions in a time window starting several hours before the overpass time of the satellite. Therefore, the average lifetime can be substantially longer than the reported daytime values. Our model used a custom set up for the chemistry simulation that employs a single value of the lifetime for this 24 h period over all height levels. For the winter case, we thus decided to use an infinite lifetime, which has the advantage that we can use the same Jacobian for NO2 and CO2. Supplementary Figure S2 shows the height dependence of the summer life time. Overall we think that the global-scale estimate of 4 h from Beirle et al. (2011) represents a reasonable average over time and height for our summer period, which also falls in the range of 3.6 (±0.8) hours estimated by Schaub et al. (2007) for the summer period over Switzerland.
For the electricity generation sector we take the locations of power plants, temporal emission profiles, as well as annual emissions of CO2 and NOx from a data set (Super et al., 2020) compiled as part of the European commissions’s CHE project (Balsamo et al., 2021). Supplementary Table S1 shows the 10 power plants in the domain with highest CO2 emissions. We compute vertical profiles through a detailed plume rise simulation based on an implementation (Brunner et al., 2019) of the guidelines by the association of Germany engineers (VDI). As the VDI procedure requires a number of plant characteristics as input we limited the detailed plume calculation to 11 power plants within the domain for which these characteristics were available. These power plants are a combination of the power plants with the highest CO2 emissions in the area and of several power plants operated by Vattenfall. The input variables to the plume rise simulation were provided by a combination of Pregger and Friedrich (2009), Berlin’s Senate Department for Environment, Transport and Climate (Andreas Kerschbaumer, personnel communication) and the plant’s webpages1. As an example, Figure 3 shows plume rise simulations for Jänschwalde, the largest power plant in the domain, and for Reuter West, the largest power plant in Berlin, for both study periods. For the remaining plants we used the standard profile from Bieser et al. (2011). The approach yields a vertical emission profile for each power plant and each hour of our simulation period, which is then used to distribute the emissions over the 32 vertical levels of our transport model. The vertical extent of the plumes increases in summer during daytime due to the growth of the atmospheric boundary layer encompassing the height of the plume.
FIGURE 3. Plume rise simulation for Jänschwalde (left) and Reuter-West (right) for 3 February 2008 (top) and for 3 July 2008.
The control vector for the electricity generation sector consists of the CO2 emission of each power plant and of a scaling factor for the NO2 to CO2 emission ratio, which also absorbs uncertainties in the NO2 to NOx ratio. Prior uncertainties of the CO2 emission are assumed to be a constant fraction of 20% of the emission; a constant fraction of 10% is also explored (see Section 4). With regard to the degree of differentiation of the scaling factor of the NO2/XCO2 emission ratio we explore three cases:
Uniform: All plants in our domain share the same scaling factor.
Per type: All plants in our domain of the same fuel type share the same scaling factor.
Per plant: Each plant in our domain has an individual scaling factor.
The prior value for the scaling factor is 1. The (relative) prior uncertainty of the emission factor ratio is calculated from reported emission factor uncertainties averaged for several countries, following the approach used by Super et al. (2020). Supplementary Table S2 shows the relative emission factor uncertainty per fuel type. The average uncertainty was used for all plants of which we could not identify the fuel type and also for the case “uniform”.
Our “other” sector accounts for fossil fuel emissions from all sectors except electricity generation. Other sector emissions of CO2 and NOx are also taken from the data set by Super et al. (2020). The control vector for the other sector consists of the CO2 emission into each model grid cell and of a scaling factor for the NO2 to CO2 emission ratio. In our experiments, we use a (spatially uncorrelated) prior uncertainty of 52.8% of the emissions into a grid cell for each grid cell, which translates to a 20% prior uncertainty when aggregated over Berlin. For the scaling factor of the NO2/CO2 emission ratio we use a prior of 1 and the prior uncertainty for the average over fuel types (Supplementary Table S2).
The terrestrial biosphere model we used to calculate the natural terrestrial CO2 exchange fluxes is based on the Simple Diagnostic Biosphere Model (SDBM, Knorr and Heimann, 1995), which was used by Kaminski et al. (2002) for assimilation of CO2 and by Kaminski et al. (2017) for assimilation of XCO2.
Here we use a new implementation on the 2 km by 2 km grid of the transport model with a time step of 1 hour. It calculates the uptake of CO2 by photosynthesis (expressed as Gross Primary Productivity, GPP) using the light-use efficiency approach:
where α is a plant water stress factor, β a temperature-dependent efficiency scalar, FAPAR the fraction of absorbed photosynthetically active radiation, and PAR the incident photosynthetically active radiation, assumed to be 50% of solar incoming radiation. Light use efficiency, ϵ, and-saturation-level PAR, PAR0, are model parameters.
The water stress factor is determined by
and α being the lesser of α′ and 1. AET and PET are actual and potential evapotranspiration, respectively. The difference to Knorr and Heimann (1995) is the additional FAPAR in the denominator, which accounts for the decreased water demand when plants only cover part of the land surface. The use of evapotranspiration takes into account the fact that plants tend to control most evapotranspiration in water-limited conditions and require transpiration in order to maintain photosynthesis.
The temperature-dependent scalar follows Mahadevan et al. (2008) and is given by
and the condition β = β′ if β′ is between 0 and 1, but β = 0 if β′ < 0 and β = 1 if β′ > 1 (i.e., β is restricted to values between 0 and 1). Ta is air temperature, and Tmin, Topt and Tmax are parameters set to 0, 20 and 40°C, respectively.
Ecosystem respiration, R, is calculated following a Q10 functional relationship with air temperature Ta and is modulated by a different water stress factor compared to photosynthesis, assumed to be the ratio of plant available soil water, w divided by the maximum plant available water holding capacity of the soil, wmax:
Q10 expresses the ratio of respiration at air temperature Ta + 10°C to that at Ta, with Ta measured in °C, and R0 is the respiration rate under standard conditions (w = wmax, Ta = 0). FAPARgs is FAPAR during the growing season, which is defined as the period for which Net Ecosystem Exchange (NEE = GPP-R) is positive. FAPAR is used to account for the impact of available plant material for decomposition.
Soil moisture is updated hourly using a bucket scheme following Knorr and Heimann (1995), with an evapotranspiration supply equal to cw ⋅ w/wmax, and a demand equal to evapotranspiration by Priestley and Taylor (1972), which equals PET. AET is computed as the minimum of supply and demand at an hourly time step, and uses precipitation and incoming solar and thermal radiation as input. cw is a model parameter and describes root zone water supply in the absence of soil water limitation.
The control variables for the terrestrial biosphere model component are the parameters ϵ, PAR0, R0, Q10, and cw. The model was calibrated against NEE from the complete set of 166 Tier-1 FLUXNET2015 sites (Pastorello et al., 2017) available at the time of download (6 February 2019). Site IDs are provided in Appendix A. We use NEE derived using the variable u-star method, reference selected on the basis of model efficiency 2. The parameter values of the calibrated model are ϵ = 0.0071538 gC/W/hour, PAR0 = 404.62 W/m2, R0 = 0.059024 gC/m2/hour, Q10 = 1.7622, and cw = 0.52621 mm/h. For our assessments, we use the calibrated model and assign a prior uncertainty of 20% to each of the five parameters.
In summary, the model uses as driving data gridded fields of temperature, precipitation, incoming solar and thermal radiation and FAPAR. The climate data fields are taken from the ERA5 reanalysis data set 3 on a 0.25° grid. We aggregated the FAPAR product (Pinty et al., 2011) derived with JRC-TIP (Pinty et al., 2007) from its native 1km resolution to the 2 km by 2 km model grid and spatially interpolated the climate data to the same grid using two-dimensional triangulation-based linear interpolation.
The Quantitative Network Design (QND) formalism is presented in detail by Kaminski and Rayner (2017), see also Tarantola (2005) and Rayner et al. (2019). In brief, it performs a rigorous uncertainty propagation from the observations to a target quantity of interest relying on the indirect link from the observations to the target variables established by a numerical model. The link has to be indirect, because, in general there is no direct link from the observations to the target quantity. There are, however, direct links from the control vector, which includes the uncertain inputs to the modelling chain, to target quantities and observations. For our experiments, the control vector is composed of the fossil fuel emissions from power plants, the fossil fuel emissions from the other sector, scaling factors for the NO2/CO2 emission ratio, and the parameters of the terrestrial biosphere model.
The target quantities are fossil fuel emissions for each power plant and from the other sector on the 2 × 2 km2 pixel scale and aggregated to larger scales, including the scale of Berlin districts and of the entire city. The observational impact on the target quantities is quantified by the following two-step procedure: The first step uses the observational information to reduce the uncertainty in the control vector, i.e., from a prior to a posterior state of information. The second step propagates the posterior uncertainty forward to the simulated target quantity.
Within the QND formalism, we represent all involved quantities by probability density functions (PDFs). We typically assume a Gaussian form for the prior control vector and the observations, if necessary after a suitable transformation. The Gaussian PDFs’ covariance matrices express the uncertainty in the respective quantities, i.e., C (x0) and C (d obs) for the prior control vector and the observations. In the context of these PDFs we will use the term uncertainty to refer to its full covariance matrix in the case of a vector quantity. In the case of a scalar quantity or a given vector component it refers to the square root of the entry on the diagonal of the full covariance matrix corresponding to that particular vector component. In the latter case the uncertainty refers to one standard deviation of the marginal PDF corresponding to that component.
For the first QND step we use a mapping M from control variables onto equivalents of the observations. In our notation the observation operators that map the model state onto the individual data streams [see Kaminski and Mathieu (2017)] are incorporated in M. Let us first consider the case of a linear model, for which we denote by M′ the Jacobian matrix of M, i.e., the derivative of M with respect to x. In this case, the posterior control vector is described by a Gaussian PDF with uncertainty C(x), which is given by
where the data uncertainty C(d) is the combination of two contributions:
The term C (dobs) expresses the uncertainty in the observations and C (dmod) the uncertainty in the simulated equivalents of the observations M(x). The first term in Eq. 7 expresses the impact of the observations and the second term the impact of the prior information. In the non-linear case we use Eq. 7 as an approximation of C(x).
The mapping N involved in the second QND step is the mapping from the control vector onto a target quantity, y. The Jacobian matrix N′ of the mapping N is employed to approximate the propagation of the posterior uncertainty in the control vector C(x) forward to the uncertainty in a target quantity, σ(y) via
If the model were perfect, σ(ymod) would be zero. In contrast, if the control variables were perfectly known, the first term on the right-hand side would be zero. The structural uncertainty of M is captured in the term C (dmod) in Eq. 8 and of N in the term σ(ymod) in Eq. 9, as well as the uncertainty in those process parameters, boundary and initial values that are not included in the control vector.
We note that (through Eqs 7, 9) the posterior target uncertainty solely depends on the prior and data uncertainties, the contribution of the model error to the uncertainty in the simulated target variable, σ(ymod), as well as the observational and target Jacobians (quantifying the linearised model responses of the simulated observation equivalent and of the target quantities). Hence, the QND formalism can be employed to evaluate hypothetical candidate networks. Candidate networks are characterised by observational data type, location, sampling frequency and time, and data uncertainty but not the observational value. Here, we define a network as the complete set of the characterisation of observations used to constrain the model. We quantify the performance of a candidate network by its ability to reduce the uncertainty of the target variables.
Each CO2M satellite has a repeat cycle of 11 days. We apply our QND framework around the CCFFDAS to the simulated uncertainty in single measurement retrievals of CO2M for two specific overpasses with low cloud cover over our study domain around Berlin, one in winter and one in summer. The XCO2 systematic uncertainty has a main contribution from perturbations of the optical path of the Earth reflected sun light that reaches the detector, due to scattering on atmospheric particles, notably aerosols and ice crystals in subvisible cirrus clouds. In the ESA AeroCarb project (Houweling et al., 2019) this error has been quantified by radiative transfer modelling, accounting for multiple scattering on aerosol particles that were simulated using the WRF-Chem model (Chen et al., 2016). The simulated radiance spectra were used in the Remote Sensing of Greenhouse Gases for Carbon Cycle Modeling (RemoTeC) algorithm to retrieve XCO2, using generic a priori assumptions on aerosols, as used for example in the retrieval of XCO2 from GOSAT. Notice that the GOSAT retrieval makes use of a bias correction to TCCON data, which is not used in the AeroCarb computations in order to quantify the performance of the instrument concept itself. Similar computations were performed assuming that the CO2 spectrometer on CO2M is accompanied by a multi-angular polarimeter (MAP) for measuring aerosols. The AeroCarb computations for Berlin cannot directly be used here, because different days were used focusing on the year 2013.
Instead we used a neural network that was trained on RemoTeC GOSAT retrievals as part of the EU CHE project (Strandgren, 2020). The neural network requires the following parameters as input: solar zenith angle (SZA), viewing zenith angle (VZA), AOTNIR, AOTSWIR1, AOTSWIR2, AlbedoNIR, AlbedoSWIR1, AlbedoSWIR2 (see Supplementary Table S3), where AOT denotes aerosol optical thickness and the superscripts NIR (near infrared), SWIR1 (short-wave infrared), and SWIR2, respectively refer to the bands at 870, 1640, and 2050 nm.
The systematic error generated by the neural network has been compared to the AeroCarb error simulations. The required wavelength dependent surface albedos were available from the AeroCarb dataset. Wavelength dependent AOTs have been computed using WRF-Chem derived information on the effective particle radius. Figure 4 shows a comparison between AeroCarb and neural network generated errors. Some differences are seen, with the neural network showing larger errors on average, especially for surface albedos larger than 0.3–0.5. However, given the wide range of conditions that is covered by the neural network, and the limited set of input parameters that is used, the differences remain within the expected range. To generate data sets of random and systematic errors over our study domain we ran the trained neural network for each retrieval provided in the L2e files. Most of the input parameters required by the neural network (first column of Supplementary Table S3) are provided with the L2e files (middle column), some were computed from the available parameters (last column). In winter, input values for the VZA reach up to 15.58°. As the validity domain of the neutral network is 0° ≤ VZA ≤14°, values above 14° were mapped onto 14°.
FIGURE 4. Comparison of XCO2 systematic error from the AeroCarb error computations (red) for the Berlin winter (left) and summer (right) cases and corresponding errors from the GOSAT trained neural network (blue).
To quantify XCO2 systematic errors for retrievals that make use of information on aerosols from a MAP we cannot simply extend the existing neural network, because of the lack of a suitable training dataset from GOSAT. To nevertheless obtain an indicative estimate of the accuracy that can be gained, we trained another neural network quantifying the ratio of systematic XCO2 errors with/without the use of a MAP. This neural network uses the same 8 input parameters as before (see Supplementary Table S3), and has been trained on the AeroCarb simulated systematic XCO2 errors for Berlin with and without MAP. Given the difficulty to train a generic neural network based only on the two cases (winter and summer) that are available from AeroCarb for Berlin, we trained two networks, one for winter and another one for summer. The performance of these neural networks compared with test datasets is shown in Figure 5. As can be seen, the information on aerosols obtained from a MAP allows the systematic XCO2 error to be reduced by about a factor of 10. Finally, we used this second trained neural network to generate data sets of systematic errors with MAP over our study domain (bottom panel of Figure 6).
FIGURE 5. Evaluation results for the neural network to compute the ratio of the systematic XCO2 errors for the cases with (nominator) and without (denominator) MAP for the Berlin winter (left, R2 = 0.80, bias = −8.3 ⋅ 10–4) and summer (right, R2 = 0.63, bias = −3.1 ⋅ 10–3) cases.
FIGURE 6. Random (top) and systematic errors without (middle) and with (bottom) MAP for winter (left) and summer (right) study period. Diamond: Schwarze Pumpe; triangle: Jänschwalde.
To test the sensitivity of our results to the error specification we used the L2e files Buchwitz et al. (2013) as an alternative (Figure 7). For each of the three error specifications, we computed the variance of the XCO2 observational uncertainty as the sum of the variances of random and systematic errors. This reflects the assumption that systematic and random errors are independent of each other and also in space.
FIGURE 7. Random (left) and systematic (right) errors from L2e data set for the winter (top) and summer (bottom) study period. Diamond: Schwarze Pumpe; triangle: Jänschwalde.
For the retrieval uncertainty in tropospheric column NO2 we follow the estimates of Lorente et al. (2019) for the TROPOMI NO2 retrieval. The total uncertainty has contributions from the slant column retrieval, the stratospheric correction to obtain tropospheric sub columns, and the air mass factor correction. The slant column uncertainty is estimated at 0.5–0.6 ⋅ 1015 molec.cm−2 for the TROPOMI retrieval, and reflects the instrumental signal/noise. Therefore this term can be treated as a random uncertainty. Based on comparisons with NDACC measurements, the stratospheric correction is estimated to be 10% uncertain on the size of the stratospheric subcolumn, translating into an uncertainty on the tropospheric NO2 column of 0.3 ⋅ 1015 molec. cm−2. Stratospheric NO2 maximises at 30–40 km altitude and shows a predominantly zonal distribution that varies with season and local time (Beirle et al., 2016). This error is, hence, expected to show a significant spatio-temporal coherence on the spatial scale of cities, and can therefore be assumed to be systematic. It may be possible to derive a large-scale correction for this error, which would leave us with small-scale deviations of that correction. For these small-scale deviations we regard the above uncertainty of 0.3 ⋅ 1015 molec. cm−2 as a conservative estimate and assume no spatial correlation. The TROPOMI NO2 validation to ground based measurements shows a low bias of 25%, which is assumed to be due mainly to the air mass correction. This error can be bias corrected, and therefore needs not to be accounted for as an uncertainty. We regard random and systematic error as independent and compute the variance of the our NO2 observational uncertainty as the sum of the variances of random and systematic errors.
As mentioned, the QND approach relies on a Jacobian that quantifies the sensitivity of the CO2M observations with respect to the control vector (Section 2.6). This subsection presents the respective components of this Jacobian.
For the electricity sector we performed individual simulations of the plumes at the CO2M overpass time of the 170 power plants in the domain. For each power plant, the respective column of the Jacobian is the ratio of the simulated XCO2 divided by the emission over 24 h. Figure 8 shows examples for two of the power plants, the largest plant in the domain at Jänschwalde and the large plant in Schkopau, located in the southwestern corner of the domain and hidden by clouds in the winter and summer cases. We will come back to this aspect in Section 5. To indicate that we refer to specific one-day periods rather than entire seasons, we use the terms “winter case” and “summer case” throughout.
FIGURE 8. Jacobians for two large power plants (respective locations indicated by black dots), Jänschwalde (top) and Schkopau (bottom), winter (left) and summer (right). Sensitivity of XCO2 with respect to emissions over the past 24 h (ppm/kgC).
A Jacobian expressing the sensitivity of the simulated XCO2 with respect to an emission from the other sector into any given surface grid cell was computed by a corresponding forward simulation with a unit emission into that grid cell (see Supplementary Figure S3). For area sources a suitable display format is to select a measurement at a particular point and show its sensitivity to surface emissions on the grid of the domain, often denoted as footprint of the measurement. As an example, Figure 9 shows the sensitivity (footprint) of a CO2 column over the centre of Berlin (Brandenburg Gate) with respect to surface emissions from the other sector. The change in display style with respect to the plumes of the power plants also implies that we expect regions of high sensitivity upwind of the measurement point, while the plume of a power plant emission is located downwind of the plant location. We note that the footprint of the XCO2 column is long and, in the summer case also somewhat blurred. We will come back to this point.
FIGURE 9. Jacobians for surface emissions from other sector in winter (left) and summer (right). Sensitivity of XCO2 over Brandenburg Gate with respect to surface emissions over the past 24 h (ppm/kgC).
Figure 9 is complemented by the sensitivity to the inflow from the lateral boundaries, which Figure 10 shows for the summer case. It is remarkable that the impact of the inflow is far from uniform in the vertical domain, a clear indication of the change in wind direction with height. For example near the surface we have high impact of the inflow from the southeast, which is consistent with the structure of the impact from the surface emissions shown in Figure 9. In the stratosphere there is, however, also large impact of inflow from the west. The final component of the Jacobian quantifies the sensitivity of simulated XCO2 with respect to the parameters in the terrestrial biosphere model.
FIGURE 10. Jacobians for inflow from the four lateral boundaries in summer. Sensitivity of XCO2 over Brandenburg Gate with respect to inflow over the past 24 h (ppm/kgC).
The top panels of Figure 11 shows an alternative way of displaying the footprints shown in Figure 9. For each grid cell it shows the contribution of the sensitivity to emissions into that grid cell as a percentage of the sum of the sensitivities to emissions into all surface grid cells, i.e., excluding the lateral inflow. It shows us that no single grid cell contributes more than ∼1% to the overall sensitivity. This has the important consequence that, in the absence of additional information on the field of surface emissions, the information in a single measurement is insufficient for the attribution of the measurement to a particular emission point, e.g., the surface pixel over which the measurement was performed. This underlines the role of the three-dimensional atmospheric transport in the interpretation of XCO2 observations and indicates the limits of simple mass balance approaches.
FIGURE 11. Footprint of XCO2 over Brandenburg Gate, contribution of sensitivity to emissions into grid cell as a percentage of the sum of the sensitivities to emissions into all surface grid cells (top) and decomposition of the contribution of the other sector emission into each grid cell to simulated XCO2 over Brandenburg Gate (bottom) in winter (left) and summer (right).
The top panels of Figure 11 also suggests a further use of the Jacobians in addition to their role in an inverse modelling/quantitative network design system. We can apply them as an efficient way of 1) simulating the XCO2 signal from surface emissions and 2) for decomposing that signal into the contributions of emissions from individual emission locations to the overall signal (Kaminski et al., 1996; Kaminski et al., 1999). For example for a spatially homogenous emission field, a value of 0.4% in a grid cell indicates that the emissions from that grid cell contribute 0.4% to the overall signal from the entire emission field. We know that the real emission field is not homogeneous. Using our other sector emission field (see Section 2.4) we obtain an overall response of 0.13 ppm in the winter case and 0.11 ppm in the summer case and the decomposition of that response is shown in the bottom panels of Figure 11.
Table 1 provides the list of experiments that were conducted for the two study periods, i.e., in winter and in summer. The default experiment (experiment 1) employs the CO2M error files based on the artificial neural network assuming the availability of the MAP. It does not use any NO2 measurements and assumes prior uncertainties of 20% for each power plant, each natural flux parameter, and of other sector for Berlin (translating to a relative uncertainty of 52.8% at the pixel level). For the lateral inflow it assumes an uncertainty of 1 ppm that is fully correlated within each group of 5 grid cells (i.e., over 10 km) in the horizontal direction and otherwise completely uncorrelated. Experiment 2 also addresses a setup with only XCO2 measurements but it uses the L2e files instead of those derived from the neural networks, which are used in Experiments 1 and 3. Experiment 3 uses the error files based on the artificial neural network, but without availability of the MAP. Experiments 4–6 equal experiment 1 but include NO2 as a further observational constraint. These three experiments differ in the degree of differentiation of the scaling factor for the NO2/CO2 emission ratio for power plants (see Section 2.3). Finally, experiment 7 explores the sensitivity with respect to the prior uncertainty in the CO2 emissions from power plants in that is uses a relative uncertainty of 10% compared to 20% in the default experiment.
The seven experiments listed in Table 1 provide a rich suite of results, which is provided in Figures 12, 13 (and in tabular form in Supplementary Tables S4–S7). We first provide a detailed presentation of experiments 1 and 4 followed by an overview on all experiments.
FIGURE 12. Uncertainty reduction for ten largest power plants and all experiments in winter (left) and summer (right).
FIGURE 13. Uncertainty reduction for the other sector at spatial scales from entire domain to grid cell and all experiments in winter (left) and summer (right).
In the default setup (experiment 1), a large uncertainty reduction (≈60%–90%) is found for the three largest power plants and a moderate uncertainty reduction (up to 10%–20%) for the next largest (Figure 12). The uncertainty reduction is higher in the summer case, when both random and systematic errors are lower (see Section 2.7). The plant in Schkopau is a special case as it is covered by clouds in both periods (see Section 3). Due to its location on the western boundary in combination with easterly winds in the winter case its plume is not observed over our domain in that period.
Next, we present uncertainty reductions for the other sector on the scales of the 2 km by 2 km grid cells, aggregated over Berlin districts, over the entire city, over some other towns in the domain, and over the entire domain (Figure 13). As for the power plants, the uncertainty reduction is considerably larger in the summer case. While the uncertainty reduction is low (up to 4% in the winter case and 8% in the summer case) at the grid cell scale (top panels of Figure 14) and focuses on grid cells with higher emissions, it increases for emissions accumulated to the Berlin district scale (up to about 10% in the winter case and 20% in the summer case) and is higher for districts with higher emissions (not shown). At the scale of Berlin we see a sizeable uncertainty reduction (about 28% in the winter case and 47% in the summer case).
FIGURE 14. Uncertainty reduction for other sector emissions at 2 km by 2 km scale over Berlin in winter (left) and summer (right) with (experiment 1, top) without NO2 (experiment 4, bottom).
Experiment 4 adds NO2 measurements to the default setup (experiment 1). The extra NO2 measurements increase the uncertainty reduction for the large power plants (Figure 12) in the summer and winter cases. In relative terms the increase in uncertainty reduction is particularly high for the plants which were not well observed with XCO2 alone and those with high NO2/CO2 emission ratio (compare Supplementary Table S1). The absolute reduction in posterior uncertainty through the addition of the NO2 measurements is, however, highest for the larger power plants (see Supplementary Table S4). In relative terms the increase in uncertainty reduction is higher in the winter case, when the constraint by XCO2 alone is weaker. The best overall performance of XCO2 and NO2 is, however, achieved in the summer case (see also Supplementary Table S6), when both random and systematic errors are lower (see Section 2.7).
Likewise for the other sector, the extra NO2 measurements increase the uncertainty reduction on all scales in the summer and winter cases. On the grid cell scale uncertainty reductions reach now 25% in the winter case and 40% in the summer case (bottom panels of Figure 14). High values reflect the combination of high emission ratio with high CO2 emissions. On the Berlin district scale the relative increase in uncertainty reduction is particularly high for districts that were not well constrained by XCO2 alone such as Spandau. In absolute terms the posterior uncertainty decreases most for districts with larger emissions such as Charlottenburg-Wilmersdorf. With the extra NO2 measurements the uncertainty reduction in the other sector emissions aggregated over Berlin increases to about 50% in the winter case and 60% in the summer case. The best overall performance of XCO2 and NO2 for the other sector is achieved in the summer case.
Figure 12 shows the uncertainty reduction for the 10 largest power plants and all experiments of the winter (left) and the summer (right) periods. The default experiment performs better than the experiment with the L2e files for all power plants, reflecting the lower random and systematic errors of the default case (Figures 6, 7) The MAP improves the impact of the CO2M measurements for all power plants and in the summer and winter cases. Over our study domain, the impact of the MAP is particularly high in the winter case. Even with reduced prior uncertainty there is strong uncertainty reduction for large power plants, in particular in the winter case, when XCO2 alone leaves more scope for improvement and the atmospheric NO2 lifetime is longer. The differentiation of the scaling factors in the NO2/CO2 emission ratio has an impact on the uncertainty reduction. As expected, a uniform scaling factor yields higher uncertainty reduction than a scaling factor per plant. This is because the uniform scaling factor is constrained by the atmospheric observations of all plants (transfer of information between plants), while an independent plant-specific scaling factor is only constrained by the atmospheric observations of the plant in question. In other words, the case of the uniform scaling factor imposes more prior knowledge as it removes the independence of the scaling factors. In between these two cases lies the case with a scaling factor per fuel type, with the exception of the largest of the ten power plants where it outperforms the case with uniform scaling factor. The four largest plants belong to the type burning solid fuel, for which there are two competing effects when changing from the case “uniform” to the case “fuel type”. First, the prior uncertainty in the emission ratio for solid fuel is considerably lower than the average we use in the uniform case, which increases the performance of the NO2 measurements. Second, the transfer of information from one power plant to the next through the use of the same scaling factor for the emission rate is obviously weaker in the case “fuel type” than in the case “uniform”, because the information is shared between fewer power plants. For the larger plants the first effect dominates the second. In the winter case this concerns the first four power plants (see Supplementary Table S4) and in the summer case the first two (see Supplementary Table S6).
Figure 13 shows the uncertainty reduction for the other sector at spatial scales from the entire domain to grid cell and all experiments in the winter (left) and the summer (right) periods. The default case performs better than the case with the L2e files over all scales. The MAP improves the impact of the CO2M measurements over all scales and in the summer and winter cases. Over our study domain, the impact of the MAP is particularly high in the winter case. Increasing the differentiation of the scaling factor for the NO2/CO2 emission ratio of the power plants (from experiment 4 to experiment 5 to experiment 6) yields a slight decrease of the performance of the other sector. This is a typical example demonstrating a general feature of the CCFFDAS, namely that, through the atmospheric constraint, better prior information (same emission ratio between power plants) on one sector (here electricity generation) is translated to better information on the remaining sectors (here the other sector). Similarly, the reduced prior uncertainty for the power plants (experiment 7), yields a slight performance increase for the other sector.
The setup of the CCFFDAS (through our specification of the control vector, see Section 2) and of the experiments (through the sensitivities we investigated, see Section 4) account for a specific choice of uncertain elements in the processing chain and its inputs. Further uncertain factors not covered through inclusion into the control vector nor through sensitivity experiments include the temporal shape of fossil fuel emission profiles, the spatial differentiation of the NO2/CO2 emission ratio for the other sector, the correlation between uncertainties in NO2 and XCO2 measurements and in space, uncertainties in NO2 measurements, lateral NO2 influx, and structural uncertainties in the models of fossil fuel emissions, natural fluxes, and atmospheric transport including atmospheric chemistry (NO2 lifetime). We note that all these factors can be handled by the QND approach with a suitable setup of experiments. Some of them would, however, require an extension/improvement of the modelling chain. The inclusion of uncertainty in the temporal emission profiles would, for example, lead to a refined fossil fuel emission model. Uncertainties through the use of an atmospheric NO2 lifetime could be reduced through inclusion of a dedicated module for (possibly simplified) simulation of atmospheric chemistry. The inclusion of structural errors is explicitly foreseen in Eqs 8, 9 and would require a systematic assessment of model error, which is a complex task. We note that many structural errors, e.g., those of the transport model, affect all our experiments in a similar way and are, hence, less relevant when we compare the assessments between experiments.
To appraise our posterior fossil fuel emission uncertainties we have to balance opposing aspects: Neglecting the above sources of uncertainty in our experimental setup has certainly led to an underestimation of posterior uncertainty. On the other hand, there are several factors that, in a future CCFFDAS, would reduce posterior uncertainties. First, our prior emission uncertainties are rather conservative, experiment 7 has demonstrated how reduced prior uncertainty for the energy sector reduces the posterior uncertainty for both sectors. Second, there is scope for reducing structural errors in a CCFFDAS with better, more accurate models of fossil fuel emissions, natural fluxes, and atmospheric transport. Third, the CCFFDAS approach allows to complement the atmospheric observations with further, more direct observations that constrain the models for fossil fuel emissions and natural fluxes. The inclusion of such complementary observations in a CCFFDAS will reduce the degrees of freedom in the inverse problem and thus also improve the leverage of the atmospheric measurements. Likewise the assimilation of meteorological data streams from both in situ and space-borne sensors will help to constrain atmospheric dynamics and reduce the effect of transport. With the above considerations our posterior uncertainties might give a good indication of what is feasible with CO2M observations in future inversion systems.
This study explored the impact of CO2M observations in a 200 × 200 km2 domain around Berlin. For the quantification of XCO2 random and systematic errors the study developed and applied new error parameterisation formulae based on artificial neural networks for cases with and without MAP. The study further established a CCFFDAS modelling chain from parameters of emission models to XCO2 and NO2 observations and computed a full Jacobian matrix representation of this modelling chain for the 24 h periods preceeding simulated CO2M overpasses over the study area in summer and winter. This Jacobian quantifies the sensitivity of the simulated measurements with respect to the parameters in the emission models and enables a rigorous uncertainty propagation (QND approach) to assess the impact of CO2M observations on fossil fuel emissions. As a by-product, the full Jacobian also provides the footprint of the CO2 column and allows us to decompose a simulated CO2 column in terms of spatial emission impact. This analysis reveals the complicated structure of the footprint of an observed CO2 column, which indicates the limits of simple mass balances approaches for interpretation of such observations.
The study conducted a number of assessments of observation impact in terms of the posterior uncertainty in fossil fuel emissions over 24 h on scales ranging from 2 to 200 km. This means the assessments include temporal and spatial scales typically not covered by inventories. Our typical metric is the relative reduction of the uncertainty in emissions through the information in the CO2M measurements compared to the prior uncertainty. The assessments differentiate the fossil fuel CO2 emission into two sectors, an energy generation sector (power plants) and the complement (“other sector”).
We find that XCO2 measurements alone provide a powerful constraint on emissions from larger power plants and a constraint on emissions from the other sector that increases when aggregated to larger spatial scales. The MAP improves the impact of the CO2M measurements for all power plants and for the other sector on all spatial scales. Over our study domain, the impact of the MAP is particularly high in the winter case. NO2 measurements provide a powerful additional constraint on the emissions from power plants and from the other sector. Through the atmospheric constraint, more prior information on the CO2 emissions from power plants or on the differentiation of the NO2/CO2 emission factor reduces the uncertainty in CO2 emissions from the other sector.
Our results suggest that the capability of CO2M measurements to constrain fossil fuel emissions varies between summer and winter cases. The main factor behind the larger constraint in the summer case are lower random and systematic errors in XCO2 measurements. There are, however, exceptions related to factors such as cloud cover and atmospheric transport.
The setup of the CCFFDAS and of the experiments focus on specific uncertain elements in the processing chain and its inputs. Further uncertain factors such as structural model errors are not covered, so that the quantitative assessments with the current prototype might be interpreted as a lower limit for posterior uncertainty. We can, however, expect that, with sufficient research effort, future CCFFDAS’s will be able to benefit from more accurate models of fossil fuel emissions (finer sectoral resolution, further observational constraints), of natural fluxes (more terrestrial observations including CO2M measurements of solar induced fluorescence, better meteorological driving data), and of atmospheric transport (constrained by observations of local meteorological conditions) and improved prior information. In this sense our performance assessments may provide a realistic indication of what can be achieved. In summary we find that the combination of CO2M with a suitable inversion system can provide useful estimates for urban scale emission reporting/verification. As for the global CCFFDAS (Kaminski et al., 2022, see), possible application modes are either a verification mode, in which the system is operated largely independently from inventory information or a synergy mode that derives a best emissions estimate by integrating bottom up information.
The original contributions presented in the study are included in the article/Supplementary Material, further inquiries can be directed to the corresponding author.
TK, MS, and PR conceived the study. SH and SL computed lifetimes of NO2, the algorithm for simulation of XCO2 error data set, and error estimates for NO2 column. MB and MR prepared XCO2 error data set. MV, JS, and PR conducted atmospheric transport simulations. GK and DB provided algorithm for plume rise simulation and its parameterisation and MV made the simulations. SD, HD, and IS prepared emission data base and uncertainty estimates on emission factors. MV prepared further input data sets and graphics. TK conducted experiments and prepared first draft of manuscript. All authors contributed to discussion and presentation of results.
This study was funded by the European Space Agency under Contract No. 4000122014 and through the European Union’s Horizon 2020 research and innovation programme under grant agreements No. 776186 (the CHE project) and No. 958927 (the CoCO2 project).
Authors TK, MV, and WK are employed by The Inversion Lab.
The remaining authors declare that the research was conducted in the absence of any commercial or financial relationships that could be construed as a potential conflict of interest.
All claims expressed in this article are solely those of the authors and do not necessarily represent those of their affiliated organizations, or those of the publisher, the editors and the reviewers. Any product that may be evaluated in this article, or claim that may be made by its manufacturer, is not guaranteed or endorsed by the publisher.
We thank Andreas Kerschbaumer for providing stack heights and diameters from the Berlin Kataster, and Fabian Sandau and his colleagues from the German Environment Agency (Umweltbundesamt) for providing a data base of power production by German power plants. We thank Nadine Gobron (EC-JRC) and Monica Robustelli (EC-JRC) for providing full resolution JRC-TIP products (https://fapar.jrc.ec.europa.eu/). We thank Bernard Pinty for his enthusiastic support of this study which reflected his strong commitment to promote space-borne CO2 measurements.
The Supplementary Material for this article can be found online at: https://www.frontiersin.org/articles/10.3389/frsen.2022.887456/full#supplementary-material
1https://braunkohle.de/wp-content/uploads/2018/04/LEAG_Standortflyer_KW_Jaenschwalde_WEB.pdf (accessed: 2021-12-16); https://de.wikipedia.org/wiki/Kraftwerk_Boxberg (accessed: 2021-12-16); https://de.wikipedia.org/wiki/Kraftwerk_Schwarze_Pumpe(accessed: 2021-12-16); https://de.wikipedia.org/wiki/Kraftwerk_Schkopau (accessed: 2021-12-16); https://de.wikipedia.org/wiki/Heizkraftwerk_Reuter_West (accessed: 2021-12–16) https://powerplants.vattenfall.com/de/buch (accessed: 2021-12-16); https://powerplants.vattenfall.com/de/klingenberg (accessed: 2021-12-16); https://powerplants.vattenfall.com/de/moabit(accessed: 2021-12-16); https://powerplants.vattenfall.com/de/lichtefelde (accessed: 2021-12-16); https://powerplants.vattenfall.com/de/mitte (accessed: 2021-12-16); https://powerplants.vattenfall.com/de/reuter (accessed: 2021-12-16).
2See https://fluxnet.fluxdata.org/data/fluxnet2015-dataset/fullset-data-product/.
3Available from the Copernicus data store at https://cds.climate.copernicus.eu/cdsapp#!/dataset/reanalysis-era5-single-levels?tab=form.
The calibration of model for natural fluxes uses data from FLUXNET. According to FLUXNET data use policy, the FLUXNET IDs have to be listed along with the citation Pastorello et al. (2017):
AR-SLu, AR-Vir, AT-Neu, AU-Ade, AU-ASM, AU-Cpr, AU-Cum, AU-DaP, AU-DaS, AU-Dry, AU-Emr, AU-Fog, AU-Gin, AU-GWW, AU-How, AU-Lox, AU-RDF, AU-Rig, AU-Rob, AU-Stp, AU-TTE, AU-Tum, AU-Wac, AU-Whr, AU-Wom, AU-Ync, BE-Bra, BE-Lon, BE-Vie, BR-Sa3, CA-Man, CA-NS1, CA-NS2, CA-NS3, CA-NS4, CA-NS5, CA-NS6, CA-NS7, CA-Qfo, CA-SF1, CA-SF2, CA-SF3, CH-Cha, CH-Dav, CH-Fru, CH-Lae, CH-Oe1, CH-Oe2, CN-Cha, CN-Cng, CN-Dan, CN-Din, CN-Du2, CN-Ha2, CN-HaM, CN-Qia, CN-Sw2, CZ-BK1, CZ-BK2, CZ-wet, DE-Akm, DE-Geb, DE-Gri, DE-Hai, DE-Kli, DE-Lkb, DE-Obe, DE-RuR, DE-RuS, DE-Seh, DE-SfN, DE-Spw, DE-Tha, DK-Fou, DK-NuF, DK-Sor, DK-ZaF, DK-ZaH, ES-LgS, ES-Ln2, FI-Hyy, FI-Jok, FI-Lom, FI-Sod, FR-Fon, FR-Gri, FR-LBr, FR-Pue, GF-Guy, IT-BCi, IT-CA1, IT-CA2, IT-CA3, IT-Col, IT-Cp2, IT-Cpz, IT-Isp, IT-La2, IT-Lav, IT-MBo, IT-Noe, IT-PT1, IT-Ren, IT-Ro1, IT-Ro2, IT-SR2, IT-SRo, IT-Tor, JP-MBF, JP-SMF, NL-Hor, NL-Loo, NO-Adv, NO-Blv, RU-Che, RU-Cok, RU-Fyo, RU-Ha1, SD-Dem, SN-Dhr, US-AR1, US-AR2, US-ARb, US-ARc, US-ARM, US-Blo, US-Cop, US-GBT, US-GLE, US-Ha1, US-KS2, US-Los, US-Me1, US-Me2, US-Me6, US-MMS, US-Myb, US-Ne1, US-Ne2, US-Ne3, US-NR1, US-ORv, US-PFa, US-Prr, US-SRG, US-SRM, US-Syv, US-Ton, US-Tw1, US-Tw2, US-Tw3, US-Tw4, US-Twt, US-UMB, US-UMd, US-Var, US-WCr, US-Whs, US-Wi0, US-Wi3, US-Wi4, US-Wi6, US-Wi9, US-Wkg, ZA-Kru, ZM-Mon.
Atkinson, R., Baulch, D. L., Cox, R. A., Crowley, J. N., Hampson, R. F., Hynes, R. G., et al. (2004). Evaluated Kinetic and Photochemical Data for Atmospheric Chemistry: Volume I - Gas Phase Reactions of Ox, HOx, NOx and SOx Species. Atmos. Chem. Phys. 4, 1461–1738. Available at: https://www.atmos-chem-phys.net/4/1461/2004/. doi:10.5194/acp-4-1461-2004
Balsamo, G., Engelen, R., Thiemert, D., Agusti-Panareda, A., Bousserez, N., Broquet, G., et al. (2021). The CO2 Human Emissions (CHE) Project: First Steps towards a European Operational Capacity to Monitor Anthropogenic CO2 Emissions. Front. Remote Sens. 2, 32. doi:10.3389/frsen.2021.707247
Beirle, S., Boersma, K. F., Platt, U., Lawrence, M. G., and Wagner, T. (2011). Megacity Emissions and Lifetimes of Nitrogen Oxides Probed from Space. Science 333, 1737–1739. doi:10.1126/science.1207824
Beirle, S., Hörmann, C., Jöckel, P., Liu, S., Penning de Vries, M., Pozzer, A., et al. (2016). The STRatospheric Estimation Algorithm from Mainz (STREAM): Estimating Stratospheric NO2 from Nadir-Viewing Satellites by Weighted Convolution. Atmos. Meas. Tech. 9, 2753–2779. Available at: https://amt.copernicus.org/articles/9/2753/2016/doi:10.5194/amt-9-2753-2016
Bieser, J., Aulinger, A., Matthias, V., Quante, M., and Denier van der Gon, H. A. C. (2011). Vertical Emission Profiles for Europe Based on Plume Rise Calculations, Environ. Pollut. 159, 2935–2946. Available at: http://www.sciencedirect.com/science/article/pii/S0269749111002387, doi:10.1016/j.envpol.2011.04.030
Brunner, D., Kuhlmann, G., Marshall, J., Clément, V., Fuhrer, O., Broquet, G., et al. (2019). Accounting for the Vertical Distribution of Emissions in Atmospheric CO2 Simulations. Atmos. Chem. Phys. 19, 4541–4559. Available at: https://www.atmos-chem-phys.net/19/4541/2019/. doi:10.5194/acp-19-4541-2019
Buchwitz, M., Reuter, M., Bovensmann, H., Pillai, D., Heymann, J., Schneising, O., et al. (2013). Carbon Monitoring Satellite (CarbonSat): Assessment of Atmospheric CO2 and CH4 Retrieval Errors by Error Parameterization. Atmos. Meas. Tech. 6, 3477–3500. Available at: https://www.atmos-meas-tech.net/6/3477/2013/. doi:10.5194/amt-6-3477-2013
Calvo Buendia, E., Tanabe, K., Kranjc, A., Baasansuren, J., Fukuda, M., Ngarize, S., et al. (2019). 2019 Refinement to the 2006 IPCC Guidelines for National Greenhouse Gas Inventories. Technical report. Intergovernmental Panel on Climate Change. Available at: https://www.ipcc-nggip.iges.or.jp/public/2019rf/index.html (Accessed April 28, 2022).
Chen, Y., Cheng, Y.-F., Nordmann, S., Birmili, W., Denier van der Gon, H. A. C., Ma, N., et al. (2016). Evaluation of the Size Segregation of Elemental Carbon (EC) Emission in Europe: Influence on the Simulation of EC Long-Range Transportation. Atmos. Chem. Phys. 16, 1823–1835. Available at: https://www.atmos-chem-phys.net/16/1823/2016/. doi:10.5194/acp-16-1823-2016
Ciais, P., Crisp, D., van der Gon, H., Engelen, R., Heimann, M., Janssens-Maenhout, G., et al. (2015). Towards a European Operational Observing System to Monitor Fossil CO2 Emissions - Final Report from the Expert Group, European Commission, Copernicus Climate Change Service. Available at: https://www.copernicus.eu/sites/default/files/2019-09/CO2_Blue_report_2015.pdf (Accessed April 28, 2022).
Davis, K. J., Deng, A., Lauvaux, T., Miles, N. L., Richardson, S. J., Sarmiento, D. P., et al. (2017). The Indianapolis Flux Experiment (INFLUX): A Test-Bed for Developing Urban Greenhouse Gas Emission Measurements. Elem. Sci. Anthropocene 5. doi:10.1525/elementa.188
Eggleston, H. S., Buendia, L., Miwa, K., Ngara, T., and Tanabe, K. (2006). IPCC Guidelines for National Greenhouse Gas Inventories. Technical report. Intergovernmental Panel on Climate Change. Available at: http://www.ipcc-nggip.iges.or.jp/public/2006gl/index.html (Accessed April 28, 2022).
Enting, I. G. (2002). Inverse Problems in Atmospheric Constituent Transport. Cambridge, U.K.: Cambridge University Press.
ESA (2020). Tech. rep. Version 3.0. Copernicus CO2 Monitoring Mission Requirements Document (MRD). Available at: https://esamultimedia.esa.int/docs/EarthObservation/CO2M_MRD_v3.0_20201001_Issued.pdf (Accessed: October 1, 2020)
Eskes, H., van Geffen, J., Boersma, F., Eichmann, K., Apituley, A., Pedergnana, M., et al. (2019). Sentinel-5 Precursor/TROPOMI Level 2 Product User Manual Nitrogendioxide. Technical Report S5P-KNMI-L2-0021-MA, KNMI.
Flemming, J., Huijnen, V., Arteta, J., Bechtold, P., Beljaars, A., Blechschmidt, A.-M., et al. (2015). Tropospheric Chemistry in the Integrated Forecasting System of ECMWF. Geosci. Model Dev. 8, 975–1003. Available at: https://gmd.copernicus.org/articles/8/975/2015/. doi:10.5194/gmd-8-975-2015
Houweling, S., Landgraf, J., van Heck, H., Vlemmix, T., and Tao, W. (2019). AEROCARB – Study on Use of Aerosol Information for Estimating Fossil CO2 Emissions, Final Report: Synthesis and Recommendation, ESA Study RFP/3-14860/17/NL/FF/gp. Technical Report. Available at: https://www.frontiersin.org/articles/10.3389/frsen.2021.689838/full#B13.
Janssens-Maenhout, G., Pinty, B., Dowell, M., Zunker, H., Andersson, E., Balsamo, G., et al. (2020). Toward an Operational Anthropogenic CO2 Emissions Monitoring and Verification Support Capacity. Bull. Am. Meteorological Soc. 101, E1439–E1451. doi:10.1175/BAMS-D-19-0017.1
Kaminski, T., Giering, R., and Heimann, M. (1996). Sensitivity of the Seasonal Cycle of CO2 at Remote Monitoring Stations with Respect to Seasonal Surface Exchange Fluxes Determined with the Adjoint of an Atmospheric Transport Model. Phys. Chem. Earth 21, 457–462. doi:10.1016/s0079-1946(97)81142-1
Kaminski, T., Heimann, M., and Giering, R. (1999). A Coarse Grid Three-Dimensional Global Inverse Model of the Atmospheric Transport, 1, Adjoint Model and Jacobian Matrix. J. Geophys. Res. 104, 18535–18553.
Kaminski, T., Knorr, W., Rayner, P., and Heimann, M. (2002). Assimilating Atmospheric Data into a Terrestrial Biosphere Model: A Case Study of the Seasonal Cycle. Glob. Biogeochem. Cycles 16, 14–1–14–16. doi:10.1029/2001gb001463
Kaminski, T., and Mathieu, P.-P. (2017). Reviews and Syntheses: Flying the Satellite into Your Model: on the Role of Observation Operators in Constraining Models of the Earth System and the Carbon Cycle. Biogeosciences 14, 2343–2357. doi:10.5194/bg-14-2343-2017
Kaminski, T., and Rayner, P. J. (2017). Reviews and Syntheses: Guiding the Evolution of the Observing System for the Carbon Cycle through Quantitative Network Design. Biogeosciences 14, 4755–4766. Available at: https://www.biogeosciences.net/14/4755/2017/. doi:10.5194/bg-14-4755-2017
Kaminski, T., Scholze, M., Rayner, P., Voßbeck, M., Buchwitz, M., Reuter, M., et al. (2022). Assimilation of Atmospheric CO2 Observations from Space Can Support National CO2 Emission Inventories. Environ. Res. Lett. 17, 014015. doi:10.1088/1748-9326/ac3cea
Kaminski, T., Scholze, M., Vossbeck, M., Knorr, W., Buchwitz, M., and Reuter, M. (2017). Constraining a Terrestrial Biosphere Model with Remotely Sensed Atmospheric Carbon Dioxide, Remote Sens. Environ. 203, 109–124. Available at: http://www.sciencedirect.com/science/article/pii/S0034425717303838, doi:10.1016/j.rse.2017.08.017
Knorr, W., and Heimann, M. (1995). Impact of Drought Stress and Other Factors on Seasonal Land Biosphere CO2 Exchange Studied through an Atmospheric Tracer Transport Model. Tellus B 47, 471–489. doi:10.1034/j.1600-0889.47.issue4.7.x
Kuhlmann, G., Broquet, G., Marshall, J., Clément, V., Löscher, A., Meijer, Y., et al. (2019). Detectability of CO2 Emission Plumes of Cities and Power Plants with the Copernicus Anthropogenic CO2 Monitoring (CO2M) Mission. Atmos. Meas. Tech. 12, 6695–6719. Available at: https://amt.copernicus.org/articles/12/6695/2019/. doi:10.5194/amt-12-6695-2019
Kuhlmann, G., Brunner, D., Broquet, G., and Meijer, Y. (2020). Quantifying CO2 Emissions of a City with the Copernicus Anthropogenic CO2 Monitoring Satellite Mission. Atmos. Meas. Tech. 13, 6733–6754. Available at: https://amt.copernicus.org/articles/13/6733/2020/. doi:10.5194/amt-13-6733-2020
Kuhlmann, G., Henne, S., Meijer, Y., and Brunner, D. (2021). Quantifying CO2 Emissions of Power Plants with CO2 and NO2 Imaging Satellites. Front. Remote Sens. 2. doi:10.3389/frsen.2021.689838 https://www.frontiersin.org/article/10.3389/frsen.2021.689838.
Lauvaux, T., Miles, N. L., Deng, A., Richardson, S. J., Cambaliza, M. O., Davis, K. J., et al. (2016). High-resolution Atmospheric Inversion of Urban CO2 Emissions during the Dormant Season of the Indianapolis Flux Experiment (INFLUX), J. Geophys. Res. Atmos. 121, 5213–5236. Available at: https://agupubs.onlinelibrary.wiley.com/doi/abs/10.1002/2015JD024473. doi:10.1002/2015JD024473
Lorente, A., Boersma, K. F., Eskes, H. J., Veefkind, J. P., van Geffen, J. H. G. M., de Zeeuw, M. B., et al. (2019). Quantification of Nitrogen Oxides Emissions from Build-Up of Pollution over Paris with TROPOMI. Sci. Rep. 9, 1–10. doi:10.1038/s41598-019-56428-5
Mahadevan, P., Wofsy, S. C., Matross, D. M., Xiao, X., Dunn, A. L., Lin, J. C., et al. (2008). A Satellite-Based Biosphere Parameterization for Net Ecosystem CO2exchange: Vegetation Photosynthesis and Respiration Model (VPRM). Glob. Biogeochem. Cycles 22. Available at: https://agupubs.onlinelibrary.wiley.com/doi/abs/10.1029/2006GB002735. doi:10.1029/2006GB002735
Pastorello, G., Papale, D., Chu, H., Trotta, C., Agarwal, D., Canfora, E., et al. (2017). A New Data Set to Keep a Sharper Eye on Land-Air Exchanges. Eos, Trans. Am. Geophys. Union (Online) 98. doi:10.1029/2017eo071597
Pinty, B., Clerici, M., Andredakis, I., Kaminski, T., Taberner, M., Verstraete, M. M., et al. (2011). Exploiting the MODIS Albedos with the Two-Stream Inversion Package (JRC-TIP): 2. Fractions of Transmitted and Absorbed Fluxes in the Vegetation and Soil Layers. J. Geophys. Res. 116. doi:10.1029/2010JD015373
Pinty, B., Janssens-Maenhout, G., Dowell, M., Zunker, H., Brunhes, T., Ciais, P., et al. (2017). An Operational Anthropogenic CO2 Emissions Monitoring & Verification Support Capacity - Baseline Requirements, Model Components and Functional Architecture. EUR 28736 EN. European Commission Joint Research Centre. doi:10.2760/08644
Pinty, B., Lavergne, T., Voßbeck, M., Kaminski, T., Aussedat, O., Giering, R., et al. (2007). Retrieving Surface Parameters for Climate Models from MODIS-MISR Albedo Products. J. Geophys. Res. 112, D10116. doi:10.1029/2006jd008105
Pregger, T., and Friedrich, R. (2009). Effective Pollutant Emission Heights for Atmospheric Transport Modelling Based on Real-World Information. Environ. Pollut. 157, 552–560. doi:10.1016/j.envpol.2008.09.027
Priestley, C. H. B., and Taylor, R. J. (1972). On the Assessment of Surface Heat Flux and Evaporation Using Large-Scale Parameters. Mon. Weather Rev. 100, 81–92. doi:10.1175/1520-0493(1972)100<0081:OTAOSH>2.3.CO;2
Rayner, P. J., Michalak, A. M., and Chevallier, F. (2019). Fundamentals of Data Assimilation Applied to Biogeochemistry. Atmos. Chem. Phys. 19, 13911–13932. Available at: https://www.atmos-chem-phys.net/19/13911/2019/. doi:10.5194/acp-19-13911-2019
Reuter, M., Buchwitz, M., Schneising, O., Krautwurst, S., O'Dell, C. W., Richter, A., et al. (2019). Towards Monitoring Localized CO2 Emissions from Space: Co-located Regional CO2 and NO2 Enhancements Observed by the OCO-2 and S5P Satellites. Atmos. Chem. Phys. 19, 9371–9383. Available at: https://www.atmos-chem-phys.net/19/9371/2019/. doi:10.5194/acp-19-9371-2019
Rusli, S. P., Hasekamp, O., aan de Brugh, J., Fu, G., Meijer, Y., and Landgraf, J. (2021). Anthropogenic CO2 Monitoring Satellite Mission: the Need for Multi-Angle Polarimetric Observations. Atmos. Meas. Tech. 14, 1167–1190. Available at: https://amt.copernicus.org/articles/14/1167/2021/. doi:10.5194/amt-14-1167-2021
Schaub, D., Brunner, D., Boersma, K. F., Keller, J., Folini, D., Buchmann, B., et al. (2007). SCIAMACHY Tropospheric NO2 over Switzerland: Estimates of NOx Lifetimes and Impact of the Complex Alpine Topography on the Retrieval. Atmos. Chem. Phys. 7, 5971–5987. Available at: https://acp.copernicus.org/articles/7/5971/2007/. doi:10.5194/acp-7-5971-2007
Skamarock, W., Klemp, J., Dudhia, J., Gill, D., Barker, D., Duda, M., et al. (2008). A Description of the Advanced Research WRF Version3. NCAR Tech. Note NCAR/TN-475+STR. Boulder, CO: NCAR.
Strandgren, J. (2020). D2.5 Synthetic Satellite Datasets, CHE Project Deliverable Report. Available at: https://www.che-project.eu/sites/default/files/2020-10/CHE-D2-5-V1-0.pdf. (Accessed April 28, 2022).
Super, I., Dellaert, S. N. C., Visschedijk, A. J. H., and Denier van der Gon, H. A. C. (2020). Uncertainty Analysis of a European High-Resolution Emission Inventory of CO2 and CO to Support Inverse Modelling and Network Design. Atmos. Chem. Phys. 20, 1795–1816. Available at: https://www.atmos-chem-phys.net/20/1795/2020/. doi:10.5194/acp-20-1795-2020
Tarantola, A. (2005). Inverse Problem Theory and Methods for Model Parameter Estimation. Philadelphia: SIAM. doi:10.1137/1.9780898717921
Turnbull, J. C., Karion, A., Davis, K. J., Lauvaux, T., Miles, N. L., Richardson, S. J., et al. (2019). Synthesis of Urban CO2 Emission Estimates from Multiple Methods from the Indianapolis Flux Project (INFLUX). Environ. Sci. Technol. 53, 287–295. doi:10.1021/acs.est.8b05552
UNFCCC (2015). UNFCCC: Conference of the Parties (COP), Adoption of the Paris Agreement. Proposal by the President. Technical report United Nations. Available at: http://unfccc.int/resource/docs/2015/cop21/eng/l09.pdf. (Accessed April 28, 2022).
Wang, Y., Broquet, G., Bréon, F.-M., Lespinas, F., Buchwitz, M., Reuter, M., et al. (2020). PMIF v1.0: Assessing the Potential of Satellite Observations to Constrain CO2 Emissions from Large Cities and Point Sources over the Globe Using Synthetic Data. Geosci. Model Dev. 13, 5813–5831. Available at: https://gmd.copernicus.org/articles/13/5813/2020/. doi:10.5194/gmd-13-5813-2020
Zenodo (2018). US EPA Office of Research and Development: CMAQ. doi:10.5281/zenodo.1212601 Available at: https://www.frontiersin.org/articles/10.3389/frsen.2021.689838/full#B13.
Keywords: CO2 monitoring mission, NO2, anthropogenic fossil fuel emissions, carbon cycle fossil fuel data assimilation system, inverse modelling
Citation: Kaminski T, Scholze M, Rayner P, Houweling S, Voßbeck M, Silver J, Lama S, Buchwitz M, Reuter M, Knorr W, Chen HW, Kuhlmann G, Brunner D, Dellaert S, Denier van der Gon H, Super I, Löscher A and Meijer Y (2022) Assessing the Impact of Atmospheric CO2 and NO2 Measurements From Space on Estimating City-Scale Fossil Fuel CO2 Emissions in a Data Assimilation System. Front. Remote Sens. 3:887456. doi: 10.3389/frsen.2022.887456
Received: 01 March 2022; Accepted: 13 April 2022;
Published: 11 May 2022.
Edited by:
Zhengqiang Li, Aerospace Information Research Institute (CAS), ChinaReviewed by:
Francois-Marie Breon, CEA Saclay, FranceCopyright © 2022 Kaminski, Scholze, Rayner, Houweling, Voßbeck, Silver, Lama, Buchwitz, Reuter, Knorr, Chen, Kuhlmann, Brunner, Dellaert, Denier van der Gon, Super, Löscher and Meijer. This is an open-access article distributed under the terms of the Creative Commons Attribution License (CC BY). The use, distribution or reproduction in other forums is permitted, provided the original author(s) and the copyright owner(s) are credited and that the original publication in this journal is cited, in accordance with accepted academic practice. No use, distribution or reproduction is permitted which does not comply with these terms.
*Correspondence: Thomas Kaminski, VGhvbWFzLkthbWluc2tpQEludmVyc2lvbi1MYWIuY29t
Disclaimer: All claims expressed in this article are solely those of the authors and do not necessarily represent those of their affiliated organizations, or those of the publisher, the editors and the reviewers. Any product that may be evaluated in this article or claim that may be made by its manufacturer is not guaranteed or endorsed by the publisher.
Research integrity at Frontiers
Learn more about the work of our research integrity team to safeguard the quality of each article we publish.