- 1CIRES/Earth Lab, University of Colorado Boulder, Boulder, CO, United States
- 2Department of Geosciences, Boise State University, Boise, ID, United States
- 3Jet Propulsion Laboratory, California Institute of Technology, Pasadena, CA, United States
- 4School of Natural Resources and the Environment, The University of Arizona, Tucson, AZ, United States
- 5School of Geosciences, University of Edinburgh, Edinburgh, United Kingdom
- 6Minnesota Department of Natural Resources, Grand Rapids, MN, United States
- 7Battelle, National Ecological Observatory Network Program, Boulder, CO, United States
Assessing functional diversity and its abiotic controls at continuous spatial scales are crucial to understanding changes in ecosystem processes and services. Semi-arid ecosystems cover large portions of the global terrestrial surface and provide carbon cycling, habitat, and biodiversity, among other important ecosystem processes and services. Yet, the spatial trends and patterns of functional diversity in semi-arid ecosystems and their abiotic controls are unclear. The objectives of this study are two-fold. We evaluated the spatial pattern of functional diversity as estimated from small footprint airborne lidar (ALS) with respect to abiotic controls and fire in a semi-arid ecosystem. Secondly, we used our results to understand the capabilities of large footprint spaceborne lidar (GEDI) for future applications to semi-arid ecosystems. Overall, our findings revealed that functional diversity in this ecosystem is mainly governed by elevation, soil, and water availability. In burned areas, the ALS data show a trend of functional recovery with time since fire. With 16 months of data (April 2019-August 2020), GEDI predicted functional traits showed a moderate correlation (r = 41–61%) with the ALS predicted traits except for the plant area index (PAI) (r = 11%) of low height vegetation (<5 m). We found that the number of GEDI footprints relative to the size of the fire-disturbed areas (=< 2 km2) limited the ability to estimate the full effects of fire disturbance. However, the consistency of diversity trends between ALS and GEDI across our study area demonstrates GEDI’s potential of capturing functional diversity in similar semi-arid ecosystems. The capability of spaceborne lidar to map trends and patterns of functional diversity in this semi-arid ecosystem demonstrates its exciting potential to identify critical biophysical and ecological shifts. Furthermore, opportunities to fuse GEDI with complementary spaceborne data such as ICESat-2 or the upcoming NASA-ISRO Synthetic Aperture Radar (NISAR), and fine scale airborne data will allow us to fill gaps across space and time. For the first time, we have the potential to monitor carbon cycle dynamics, habitats and biodiversity across the globe in semi-arid ecosystems at fine vertical scales.
Introduction
Understanding the drivers of ecosystem processes and services at regional and global scales provide pivotal knowledge to assess ecosystem responses under changing conditions (Díaz et al., 2007; Isbell et al., 2015). Functional traits, including morphological, physiological and phenological characteristics, depict the growth, reproduction, and survival of individual species and individual plants (Bu et al., 2019). Further, variations in functional traits are shown to be linked to environmental conditions and their fluctuations over time (Schneider et al., 2017). For example, (Ahrens et al., 2020) demonstrated that functional traits such as leaf size and specific leaf area show variation with changes in temperature and precipitation. In addition to climate factors, other influences including human intervention and pathogens can alter functional traits and their spatial distribution (Oliva et al., 2020).
The distribution of functional traits within and between species of an ecosystem represent the demography, resilience, and response strategies to disturbance (Díaz et al., 2004; Poorter and Markesteijn, 2008; Serbin et al., 2019). Therefore, a wealth of research uses functional trait-based approaches to assess ecosystem processes and services including productivity, nutrient cycling and biodiversity (Hooper et al., 2002; Bardgett and van der Putten, 2014; Violle et al., 2014; Wieczynski et al., 2019). For example, direct observations of functional traits are widely utilized at various spatial and temporal scales, mostly in forested ecosystems, to elucidate overall structure, function, and diversity (Funk et al., 2017; Wieczynski et al., 2019). In addition, functional traits have been utilized in assessing community assembly processes across a variety of traits (e.g., Pakeman and Stockan, 2014; Medeiros et al., 2019).
Selection of representative functional traits and scaling those traits are critically important as ecosystem to global scale processes are a function of combined traits of co-occurring species and their abundance (Funk et al., 2017). However, large-scale functional diversity measurements are limited due to the lack of spatially continuous data sets (Jetz et al., 2016), where functional diversity is derived from the distribution of functional trait values at a given spatial grain and extent (Mouchet et al., 2010). Availability of remotely sensed data at fine to coarse spatial and temporal scales facilitates upscaling traits from local to regional and global scales relevant for ecosystem service management (Abelleira Martinez et al., 2016; Braun et al., 2018; Schimel, et al., 2013), and functional leaf traits are now available across biomes in North America (Wang et al., 2020). Among many, the traits that represent canopy architecture (morphological functional traits) show direct relationships between carbon storage (Rödig et al., 2019), habitat distribution and quality (Bae et al., 2019), and biodiversity (Bagaram et al., 2018), and are widely used to characterize regional to global scale ecosystem processes. Importantly, lidar remote sensing provides opportunities to accurately estimate the morphological functional traits in order to map morphological diversity at local and regional scales (Schneider, et al., 2017; Zheng et al., 2021). With the launch of the Global Ecosystem Dynamics Investigation (GEDI) mission, we have new opportunities to map functional traits and biodiversity at global scales in forest ecosystems (Marselis et al., 2019; Rödig et al., 2019; Schimel and Schneider, 2019; Dubayah et al., 2020; Schneider et al., 2020). Nevertheless, the applicability of GEDI in estimating functional traits and diversity in semi-arid ecosystems with short and sparse vegetation is still unknown.
Semi-arid ecosystems cover approximately 40% of the terrestrial landscape and show large dynamicity in ecosystem structure and function (Conti and Díaz, 2013). Hence, semi-arid ecosystems greatly impact global carbon dynamics, productivity, and habitat quality (Poulter et al., 2015). Structure-functioning relationships in frequently disturbed semi-arid ecosystems are unclear, largely due to gaps in spatially continuous data and the weak response of sparse and short height vegetation in optical remote sensing (Kulawardhana et al., 2017; Stavros et al., 2017). In particular, understanding semi-arid ecosystem responses to global change is challenging due to the complex and dynamic interactions among multiple ecosystem functions. Assessing the spatial patterns of functional diversity and its abiotic controls are critically important towards unraveling this complexity (Schlesinger et al., 1990; D´Odorico et al., 2013). Especially, understanding how community assembly is controlled by the balance of abiotic drivers is important in predicting the response of ecosystems to environmental change (Pakeman and Stockan, 2014). Further, functional diversity estimates provide the input data to help constrain model accuracies of ecosystem processes at landscape scales in different regions across the globe (Stavros et al., 2017; Braghiere et al., 2019; Dashti et al., 2021).
In this study, our objectives were to 1) evaluate the spatial pattern of functional diversity estimated from small footprint airborne lidar (ALS) with respect to abiotic controls and fire in a semi-arid ecosystem; and 2) assess GEDI’s potential of capturing the semi-arid ecosystem diversity at regional scales using GEDI data from 16 months (April 2019–August 2020) over the same study area. We selected a range of abiotic controls including topography, distance to water, topographic wetness index, and soil. Finally, we explored how disturbance from fire regulates the functional diversity in this semi-arid ecosystem.
Methods
Study Area
The study was carried out in the Reynolds Creek Experimental Watershed (RCEW) in southwest Idaho (Figure 1). The study area is characterized by a range of topography (1,100–2,200 m) and vegetation communities. While many varieties of grass, forbs, and shrubs dominate the low elevations, trees of aspen (Populus tremuloides), juniper (Juniperus occidentalis), and Douglas fir (Pseudotsuga menziesii) mark the high elevations. Low stature sagebrush (Artemisia spp.), bitterbrush (Purshia tridentata), and grass of varying densities and cover are found throughout RCEW. In addition, riparian vegetation with cottonwood (Populus trichocarpa) and willow (Alnus, Ceanothus, and Salix species) are found within valleys, and mostly along streams across the watershed. The study area has experienced prescribed and natural fires and supports grazing. As a consequence, invasion of cheatgrass (Bromus tectorum) in native shrub areas and juniper encroachment have occurred in this study area during the last few decades.
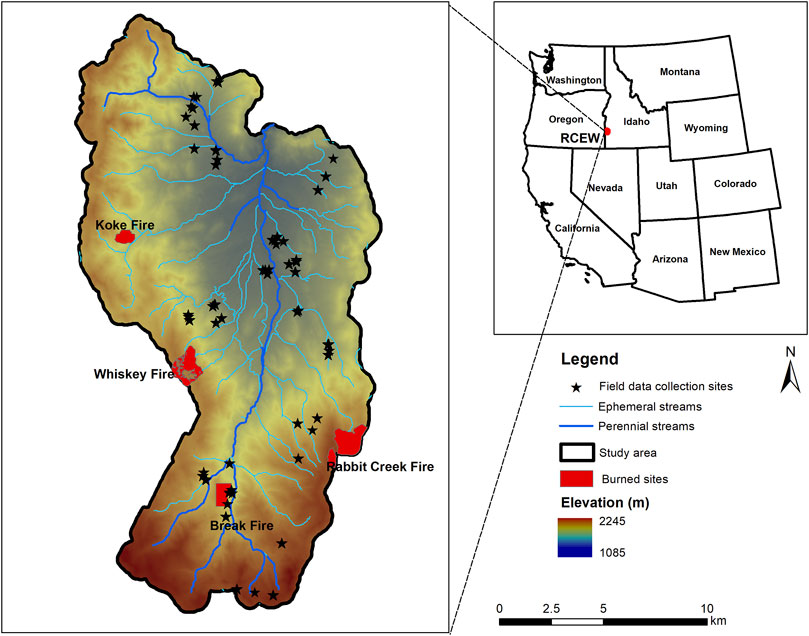
FIGURE 1. Reynolds Creek Experimental Watershed, Idaho, USA, with topographic gradient and stream network. The black stars represent 10 × 10 m field plots across the watershed.
Field Data
Reference field data were collected at 43 plots at 10 × 10 m (Figure 1). The plots were selected using a stratified random sampling approach considering the complicated terrain conditions. We established 5 transects at 1, 3, 5, 7, and 9 m in each plot. We collected canopy heights of all the shrubs, plant area index (PAI) and images at 2 m intervals along each transect totaling 20 measurements per plot using a ceptometer (AccuPAR LP-80, Decagon Devices Inc., Pullman, WA, USA) and a camera (Nikon COOLPIX AW120) respectively. The ceptometer data provides estimates of PAI following (Norman and Welles, 1983). Plot scale PAI was estimated by averaging the 20 measurements (Glenn et al., 2017). The collected images were analyzed using the “SamplePoint” freeware program to estimate the species abundance presented within each plot (v1.59, Booth et al., 2006). Each photo approximately covered 2 m2 on the ground. We placed 100 equally spaced grid points within each photo and identified the material under each grid point as vegetation species, dead wood, litter or as bare ground which were later used to estimate the percent cover of each material in those plots. Field estimated functional traits were used to assess the accuracy of ALS estimated functional traits.
Abiotic Factors
We selected six abiotic factors identified as potential factors driving the vegetation dynamics in the study area from previous research (e.g. Seyfried et al., 2018; Seyfried et al., 2001). They are elevation, slope, aspect, topographic wetness index (TWI), soil, and distance to water (DTW) (Supplementary Figure S1). The topographic variables of altitude, slope, aspect, and topographic wetness index (TWI) were estimated using the small footprint airborne lidar derived 3 m digital elevation model. The slope data were categorized into 10° groups between 0–90°. The aspect data were categorized into two major directions as north (+/− 90°–180° from south) and south (0°–+/− 90° from south). We used only north-south aspects as the study area shows vegetation growth dynamics primarily on these two aspects (Seyfried et al., 2001). We did not use the flat aspect category as there were not many pixels in this category due to the complex topography of the study area. Stream networks and soil types were retrieved from the Reynolds Creek Critical Zone Observatory database (GIS Server, Reynolds Creek Critical Zone Observatory, 2015). Euclidian distance from perennial and from all streams to each pixel were estimated and used as the distance to water (DTW). We did not use climate data such as precipitation or temperature as the local distribution of these two variables within the study area is primarily controlled by elevation and aspect (Seyfried et al., 2018).
Airborne Lidar Data
Small-footprint waveform lidar data were acquired August 2014 using the NASA Airborne Snow Observatory (ASO) (Riegl, 2017) (RIEGL Laser Measurement Systems GmbH, Horn, Austria) dual laser scanner (wavelength = 1,064 nm, outgoing pulse width = 3 ns). The study area was scanned at a pulse repetition rate of 400 kHz per laser and the backscattered signal was sampled at 1 ns interval. All the lidar waveforms in each flight line were Gaussian decomposed following the workflow of (Ilangakoon et al., 2018). We estimated spatial coordinates, incident angle, pulse width, amplitude, and scattering cross section of all echoes in each waveform. The resulting average point density across the watershed was 10–14 pts/m2 with positional accuracies of 0.14 m in vertical and 0.11 m in horizontal directions. The vertical and horizontal positional accuracies were assessed using TerraScan software.
ALS Functional Traits
The point cloud resulting from the waveform Gaussian decomposition was used to estimate the ALS based plant functional traits. Finding the bare ground is critical yet challenging as the low-stature vegetation in the study area tends to widen the waveform without a separate vegetation pulse. We used a pulse width threshold (3.2 ns) to separate bare ground from vegetation based on (Ilangakoon et al., 2018). Then, all lidar returns were height normalized using the ground plane and were aggregated into 10 × 10 m spatial pixels. A spatial resolution similar to the field plot size was used to accommodate both trees and shrubs while also allowing us to identify small variations of functional diversity in shrub-dominated areas. Canopy height is the maximum height from all above ground returns from each 10 × 10 m pixel area. PAI was estimated as,
where, P(θ) and G(θ) are the gap fraction at incident angle θ and projection function, respectively (Zheng et al., 2016). Ω is the clumping index and L is the true leaf area index (Norman and Campbell, 1989). The gap fraction was estimated as,
where σg is the scattering cross sections from ground returns and σall is the scattering cross sections from all returns at a given angle. Vegetation sparseness of the study area resulted in very similar spectral signatures for both the bare ground and the canopy (Dashti et al., 2019). We did not correct the PAI for canopy and ground reflectance. Estimates of the scattering cross section from small footprint waveform lidar are explained in Wagner (2010) and Ilangakoon et al. (2018). We predicted the foliage height diversity (FHD) at plot resolution (10 × 10 m). We used plot scale LAI profiles developed only from the above ground point cloud. The above ground lidar points were fragmented into 20 cm layers. The 20 cm layer thickness was used given that it is approximately 2 times the point cloud vertical uncertainty. For each layer, the ratio between numbers of points in each layer to the total number of points was calculated. The total foliage height diversity was then estimated using the following equation (MacArthur and MacArthur 1961).
where, pi is the fraction of foliage in ith canopy layer.
Once the three traits were estimated at 10 × 10 m pixel resolution for the whole study area, the trait values were normalized to vary between 0 and 1 following (Schneider et al., 2017).
Functional Diversity
We used three morphological traits (CH, PAI, and FHD) to estimate measures of functional diversity for the study area. We selected CH, PAI, and FHD as they can be directly estimated from both discrete return and waveform lidar and are widely used to represent a range of plant and ecosystem functions (e.g. canopy architecture, ecosystem productivity, habitat quality). We estimated three functional diversity indices: richness, divergence, and evenness, combining the above mentioned three morphological traits. Functional diversity indices were calculated around each sample unit (AKA each ALS pixel) across the watershed. To calculate functional diversity around each ALS pixel, we selected a square neighborhood with a side length of 1,000 m. This spatial neighborhood was selected in order to include at least 10 GEDI footprints within each neighborhood and to be compatible with GEDI raster products (Dubayah et al., 2020). The distribution of PAI, CH, and FHD values of all the pixels within the 1,000 m square neighborhood was then analyzed to derive the functional diversity indices following the below equations from (Villéger et al., 2008; Schneider et al., 2017).
In this study, we describe functional diversity as a 3-D trait space where the axes are represented by the three functional traits. However, functional diversity can be represented as a multidimensional trait space based on the number of traits used to estimate the diversity indices (Villéger et al., 2008, Schneider et al.,2017). The values of functional diversity indices are then calculated based on the relative distribution of functional traits values extracted from each 1000 m spatial neighborhood (Supplementary Figure S2). The functional richness is the convex hull volume covered by the PAI, CH, and FHD of the pixels within the selected neighborhood mapped in the 3-D trait space. Functional divergence (Fdiv) is estimated as:
where, S is the number of pixels used to map the functional divergence, dGi is the Euclidean distance between the ith pixel and the centre of gravity of 3D trait space defined by CH, FHD, and PAI as trait axes, and
where, EWl is the Euclidian distance of branch l in the minimum spanning tree, and S-1 is the number of branches. Here, S is the number of pixels used and the subscript i represents the 1,000 m square neighborhood space used to estimate the functional diversity (Supplementary Figure S2C).
The resulting values were assigned as the center pixel functional diversity to generate a seamless functional diversity map of the study area.
Statistical Analysis
We first investigated the correlation among functional diversity indices estimated from ALS and the abiotic factors. To analyze the effects of abiotic factors on functional diversity, we randomly selected 200 data points across the watershed covering the full range of functional diversity and the abiotic factors. We resampled the environmental factors (elevation, slope, aspect, topographic wetness index, distance to the nearest stream, and soil type) to the same 1,000 m spatial resolution of the functional diversity. We used the average of the continuous values (e.g. elevation). For categorical variables (e.g. soil type), we converted them into dummy variables and used the major category within each 1,000 m space. We set the minimum distance between data points greater than 1,020 m to avoid the mutual inclusion of niche spaces. A Gaussian generalized linear model with an identity link function was applied to the scaled continuous and dummy categorical variables with the diversity indices as the response variable to evaluate the effects of abiotic factors on functional diversity. The relative importance of each factor in the linear model was used (R package relaimpo, calc. relimp) to assess the capability of all factors together and each factor separately to explain the variance of each diversity index estimated from ALS and GEDI.
Functional Diversity Shift Under Fire Disturbance
To study how fire may shift the functional diversity, we applied a “space for time” approach to four different fires distributed across the study area. These are the Koke (natural fire, 2014), Whiskey mountain (prescribed fire, 2005), Break (prescribed fire, 2002) and Rabbit creek (natural fire, 1996). The burned areas varied from 0.5 to 2 km2. To assess the disturbance driven diversity shift, we generated circular buffer zones with 500 m distance in the unburned areas around each fire. The functional traits and diversity values of all the ALS pixels within each burned area and their surrounding unburned areas were then extracted. Trait and diversity indices of each burned pixel and the means of undisturbed values from each fire were used to predict the percent recovery. The resultant percent trait and diversity within the fires and the original traits and diversity values from the undisturbed areas were separately fitted with non-linear functions (Gompertz, polynomial, and Chapman-Richards) and the best fit models were used to interpret the post-fire recovery trajectory. Given the limited number of fires in the study area, we did not evaluate other potential factors for fire recovery rates (e.g. impact of environmental gradient on fire recovery rate). To understand the marginal spatial neighborhood size that can show the disturbance driven diversity shifts, we estimated the functional diversity with varying spatial neighborhood sizes (30–500 m).
GEDI Data and Functional Traits
To evaluate the potential of GEDI data to capture functional diversity in semi-aid ecosystems, we used GEDI 1B (v001), GEDI 2A (v001) and GEDI 2B (v001) data between April 2019 to August 2020. Data were downloaded from the Land Processes Distributed Active Archive Center (LP DAAC) using the GEDI finder tool (https://lpdaacsvc.cr.usgs.gov/services/gedifinder). In this study, we used GEDI footprints with a quality flag of 1 (Sensitivity >0.90). Horizontal geolocation accuracy of the first version GEDI data are approximately 20 m. As this may greatly impact our results, using the correlation matching algorithm (Blair and Hofton 1999) implemented in the GEDI simulator (Hancock et al., 2019) and the ALS point cloud data, we geo-corrected the GEDI data before estimating the functional diversity indices. The GEDI simulator simulates GEDI-like waveforms using the ALS point cloud and compares them with the real GEDI waveforms within a given horizontal region (∼20 m in this case). The location that has the highest correlation between the simulated and the real GEDI waveform provides the offset of the real GEDI waveform. After geo-correction, we extracted functional traits that resembles the same functional traits we derived from ALS data. The RH98 metric from the GEDI 2A product was used as the canopy height. LAI and FHD from the GEDI 2B product were used as the PAI and FHD, respectively, in this research. We used GEDI 2A and 2B products based on the default waveform processing algorithm setting. In addition, several other parameters (beam and sensitivity) were extracted to evaluate the factors affecting the accuracy of GEDI estimated functional traits. In this study, we used ALS based ground slope, ALS estimated vegetation height, ALS estimated cover, and GEDI beam (power vs coverage) and sensitivity as factors that may affect the retrieval of GEDI functional traits following (Liu et al., 2021). A relative importance matrix from a random forest regression model was used to assess the impact of the above-mentioned factors on GEDI functional traits retrievals. Given the limited number of field samples that overlap with the real GEDI footprints, we used ALS data as the reference data to assess the accuracy of GEDI predicted functional traits.
Results
ALS Derived Functional Traits
Our field observations showed a range of functional trait values for PAI (0.19–1.88) and CH (0.31 m–2.52) with mean values of 0.76 and 1.20 m, respectively. Figures 2A,B show the correlation between the functional traits estimated from 10 × 10 m field plots and traits predicted from ALS point clouds within the field plots. Field observed functional traits show a strong correlation (r = 71–88%) with ALS derived functional traits. However, the ALS underestimates the field observed vegetation heights and overestimates the PAI estimates. The ALS height underestimation is compatible with previous ALS predicted heights in similar study areas (Streutker and Glenn, 2006; Mitchell et al., 2011). This ALS height underestimation could be due to the uncertainty in bare ground detection (Glenn et al., 2011). The PAI overestimation could be due to reflectance similarities between the canopy and bare ground.
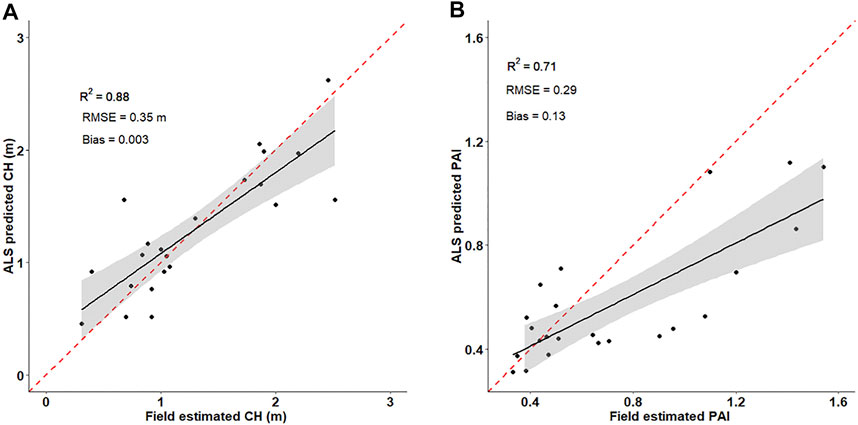
FIGURE 2. Correlation between (A) field and ALS estimated canopy height canopy heights, (B) field and ALS estimated plant area index (PAI). The solid green lines represent the best fit line while the gray areas are the 95% confidence interval. The dashed red line represents the 1:1 line.
Environmental Controls of Functional Diversity
ALS estimated functional richness values ranged between 0.0–0.11 (Figure 3A). The evenness and divergence show relatively larger values compared to the richness. The ALS evenness and divergence range between 0.31–0.99 and 0.55–0.86 (Figures 3B,C), correspondingly. The dense tree-dominant southern portion of the study area is characterized by large richness and small evenness values whereas the shrub-dominant central and northern portions of the watershed are characterized by the opposite. Evenness and richness differentiate the tree-shrub ecotones and relative densities within shrub dominant areas. A variable functional divergence is observed throughout the watershed (Figure 3C).
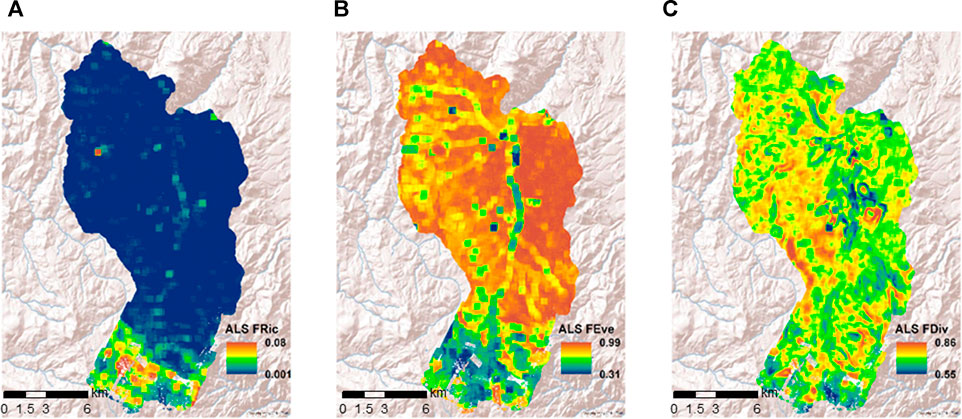
FIGURE 3. Functional diversity mapped using a 1,000 m spatial neighborhood from ALS predicted functional traits. (A) Functional richness (ALS FRic), (B) Functional evenness (ALS FEve), and (C) Functional divergence (ALS FDiv).
The trends in functional diversity indices with abiotic factors are shown in Figure 4. Functional richness slightly decreases with an increase in elevation until 1,600 m and then has a rapid increase with increased elevation (Figure 4A). The ALS predicted functional richness increases from south to north aspects (Figure 4B). ALS predicted functional evenness values decrease with a change from south to north aspects. In contrast, functional evenness values increase when the distance to water increases. There was no trend observed when we considered both perennial and ephemeral streams together to estimate the distance to water. Among all environmental factors studied, distance to water is the only factor that shows a trend with functional divergence predicted from the ALS data. This trend is generally positive, with functional divergence increasing with an increase in distance to water, especially at higher elevations (Figure 4F).
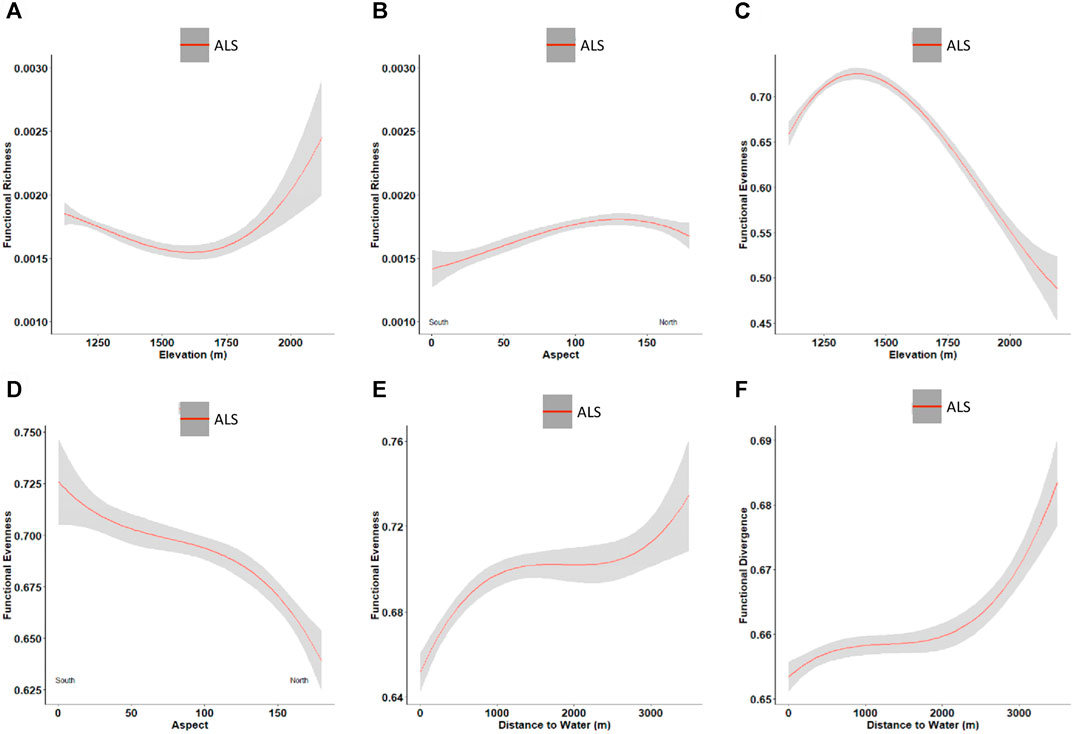
FIGURE 4. Trends between ALS predicted functional diversity and abiotic controls. The red curves represent the mean variation of diversity indices predicted from ALS and the surrounding gray area represents the standard deviation. Functional richness with (A) elevation; (B) aspect. Functional evenness with (C) elevation; (D) aspect; (E) distance to water. Functional divergence with (F) distance to water.
Functional Diversity in Fire Disturbed Areas
We analysed ALS functional traits and diversity indices over selected burned and unburned areas to infer the fire resilience of the study area. We used a fire chronosequence spanning 1996–2014 to understand if there are shifts of post-fire traits and diversity through vegetation recovery. One way ANOVA indicated that all burned and unburned groups are significantly different (95% confidence level) (Supplementary Figure S3). Based on the tested models, a 2nd order polynomial function provided the best fit and is used hereafter to describe the post-fire recovery. Based on ALS data, CH shows an initial decrease followed by an increase towards the undisturbed state (100% recovery state) (Figure 5A). PAI and FHD show a faster recovery to the undisturbed state (∼18 years post-fire); however, CH does not reach the undisturbed state during the study period. Though functional richness increased initially, a drastic decrease is demonstrated ∼9 years post-fire. In contrast, functional evenness continuously increases (Figure 5E). This post-fire increase in functional evenness indicates that fire may changes the state of the ecosystem driving it to a more functionally even ecosystem. Functional divergence shows a similar behaviour to functional richness (Figure 5F).
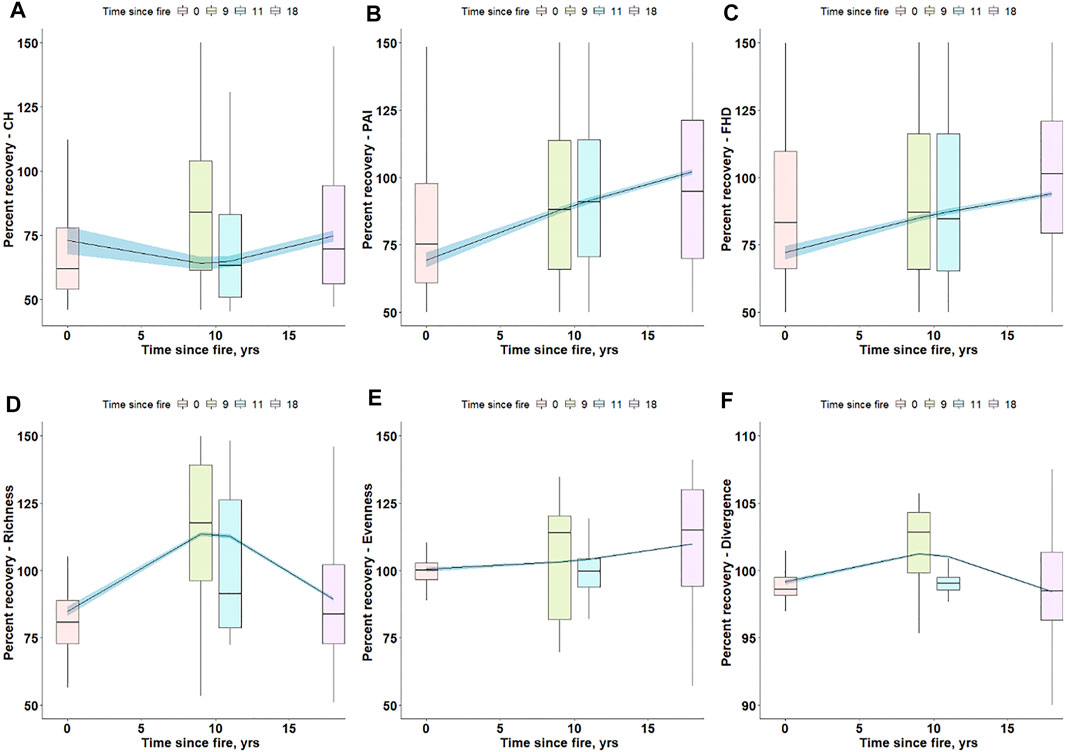
FIGURE 5. Percent recovery of functional traits (A–C) and diversity (D–F) across the fire chronosequence (1996–2014) predicted from ALS data. The box plots represent the percent recovery data distribution within a given year. The black line and blue shaded area represent the mean percent recovery and 95% confidence interval of functional trait/diversity post-fire, respectively. The box plots show the raw data (functional traits and diversity) distribution extracted from burned areas colored based on time since fire.
We further estimated the functional diversity of the four fires at a range of spatial neighbourhood sizes with 30 m increments using ALS predicted functional indices (Supplementary Figure S4). To calculate functional diversity, three or more measurements from each functional trait are considered. The minimum neighbourhood of 30 m was selected as it is 3 times bigger than the ALS pixel size. By selecting a square neighbourhood, we were constrained to 30 m being the minimum we could use. After the minimum neighbourhood was selected, the increment can be any size. We used 30 m intervals. At smaller neighbourhoods, functional richness from disturbed and undisturbed areas cannot be differentiated (Supplementary Figure S4). Functional richness values and their uncertainty increases with an increase in the spatial neighbourhood size. On the contrary, at smaller neighbourhoods, the differences in functional evenness and divergence between disturbed and undisturbed areas are clearly visible. These differences gradually diminish with an increase in the spatial neighbourhood (∼270 m) (Supplementary Figure S4). The uncertainty of functional evenness and divergence estimates also decrease when the spatial neighbourhood size increases. The results further indicated that functional diversity values resulted from each selected neighbourhood are significantly different (Supplementary Figures S6–S8).
GEDI Predicted Functional Traits in a Semi-Arid Ecosystem
Our random forest regression based relative importance values show that ground slope is the most important error factor for all three GEDI based functional trait estimates (Figure 6). In addition, ALS estimated canopy cover and canopy height influenced the GEDI trait retrievals. The ground slope varied between 0 and >60° in our study area. Due to this known influence of ground slope on large footprint lidar backscatter waveforms (Silva et al., 2018; Dong et al., 2019), we further investigated how GEDI predicted functional traits vary with ground slope. The distribution of ALS and GEDI estimated functional traits with slope are displayed in Supplementary Figure S9. The results indicate that GEDI predicted canopy height (r = 26%) and FHD (r = 51%) show a positive correlation with ground slope. This moderately strong correlation of FHD with slope could be mainly due to the shorter heights of the vegetation (<5 m) where the ground and vegetation signals likely be in mixed. We expect this slope-FHD correlation to be smaller in areas with taller vegetation. Based on our study and other related studies (Schneider et al., 2020; Liu et al., 2021), we used GEDI data only from areas with slopes less than 20° for the analysis hereafter.

FIGURE 6. Relative importance measured as percent increase in mean standard error (%IncMSE) of error factors in functional trait retrievals from GEDI. Variables with larger (%IncMSE) values are more important. (A) GEDI estimated canopy height (CH); (B) GEDI estimated plant area index (PAI), and (C) GEDI estimated foliage height diversity (FHD).
To compare GEDI functional traits with those predicted from ALS, we used the same surface coverage for both ALS and GEDI (25 m). We used the GEDI simulator based geo-correction results to find overlapping ALS and GEDI footprints. We then estimated ALS functional traits at 25 m spatial resolution from those best matched ALS point clouds. GEDI functional traits show low to moderate correlation (r = 11–61%) with ALS predicted functional traits (Figure 7). Our results indicate that GEDI data have difficulty estimating vegetation heights less than 2 m (red circle in Figure 7A). Further, GEDI data overestimate FHD (RMSE = 2.45), canopy heights (RMSE = 4.69 m) and the PAI (RMSE = 1.97) in this study area. This overestimation can be attributed to its large pulse length relative to the shorter vegetation heights in this area. In addition, the differences in the algorithms used to estimate the functional traits from the two laser systems (ALS and GEDI) may further cause these overestimations.
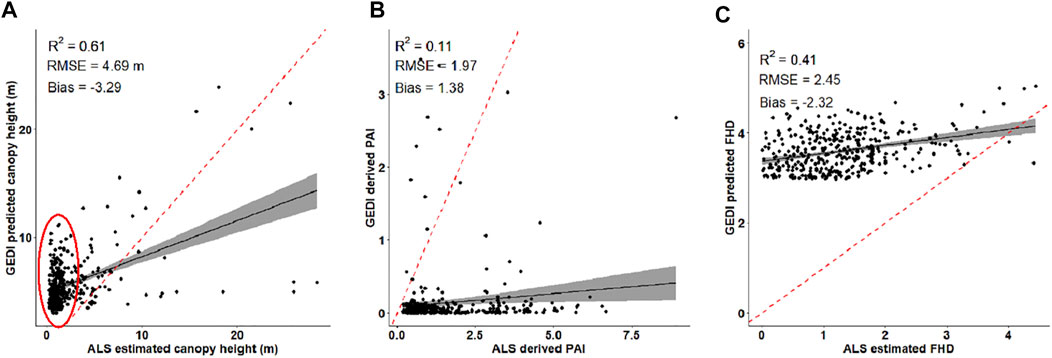
FIGURE 7. Relationship between ALS and GEDI predicted functional traits, including: (A) canopy height, (B) plant area index (PAI), and (C) foliage height diversity (FHD). The solid lines represent the best fit line while the gray areas are the 95% confidence interval. The red ellipse represents vegetation heights less than 2 m.
GEDI Predicted Functional Diversity in a Semi-Arid Ecosystem
GEDI shows small functional richness values, ranging between 0.001–0.07 (Figure 8A). The evenness and divergence show relatively larger values compared to the richness. The GEDI evenness vary between 0.55 and 0.86 (Figures 8B,C). As in ALS diversity maps, GEDI evenness and richness differentiate the tree-shrub ecotones and relative densities within shrub dominant areas. Compared to ALS functional divergence, discrete high functional divergence patches are observed throughout the watershed (Figure 8C). The correlation between ALS and GEDI predicted functional richness, functional evenness, and functional divergence are 24, 20 and 5%, respectively (Figures 8D,E). In addition, functional richness predicted from both ALS and GEDI show consistent inverse correlation (∼60%) with their respective functional evenness (Supplementary Figure S10). Though we observed moderate correlations between GEDI and ALS predicted functional traits (CH and FHD), these low correlations of functional diversity could be mainly due to the sparseness of GEDI footprints within each 1,000 m spatial neighbourhood (∼20–100 footprints compared to ∼1,600 pixels from ALS).
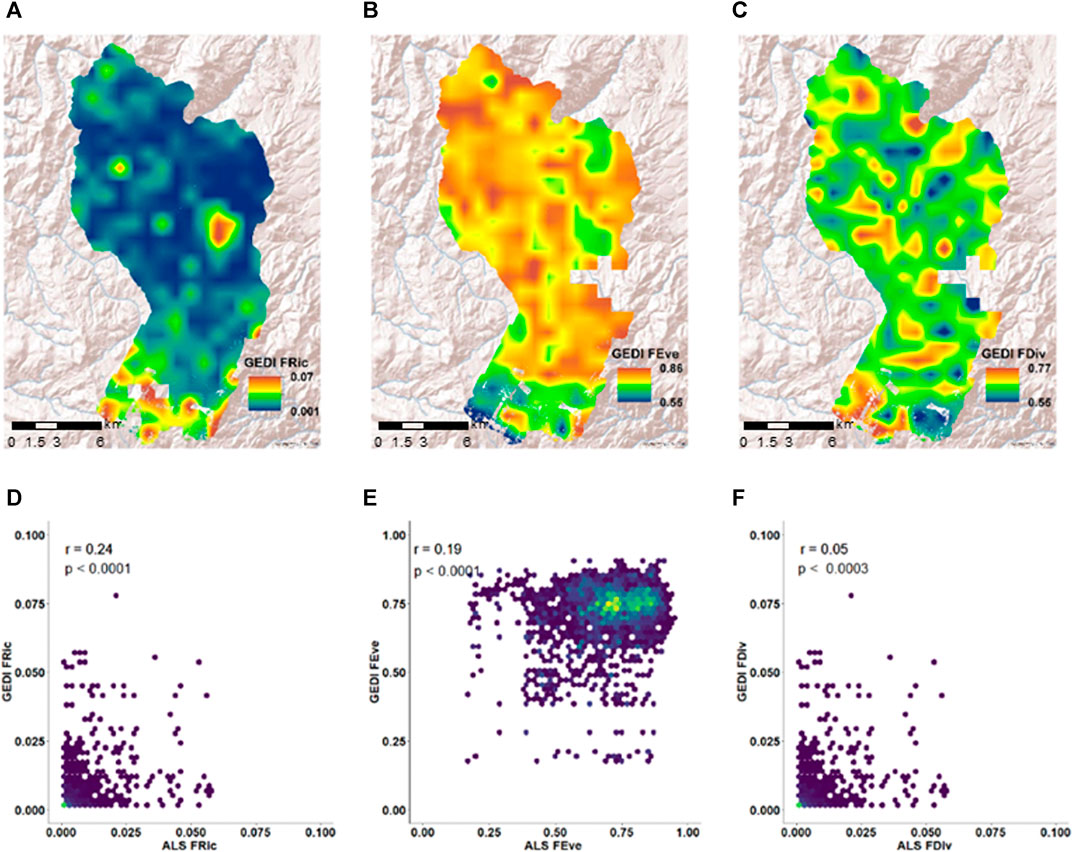
FIGURE 8. Functional diversity mapped using a 1,000 m spatial neighborhood GEDI data and their correlation with ALS derived functional diversity indices. Top row: GEDI predicted (A) functional richness (GEDI FRic), (B) Functional evenness (GEDI FEve), (C) Functional divergence (GEDI FDiv). Bottom row: Correlation between (D) ALS functional richness (ALS FRic) and GEDI predicted functional richness (GEDI FRic), (E) ALS functional evenness (ALS FEve) and GEDI predicted functional evenness (GEDI FEve), (F) ALS functional divergence (ALS FDiv) and GEDI predicted functional divergence (GEDI FDiv).
However, there are consistent trends between ALS and GEDI diversity with abiotic factors. As in ALS, functional richness slightly decreases with an increase in elevation until 1,600 m and then has a rapid increase with increased elevation (Figure 9A). The GEDI predicted functional richness shows an initial decrease on south aspects and an increase on north aspects (Figure 9B). The functional evenness shows a consistent trend with both the ALS and GEDI data (Figure 9C). The GEDI predicted functional evenness values decrease with a change from south to north aspects. The GEDI functional evenness and divergence resulted in with similar patterns as observed with ALS predicted evenness and divergence respectively (Figure 9F).
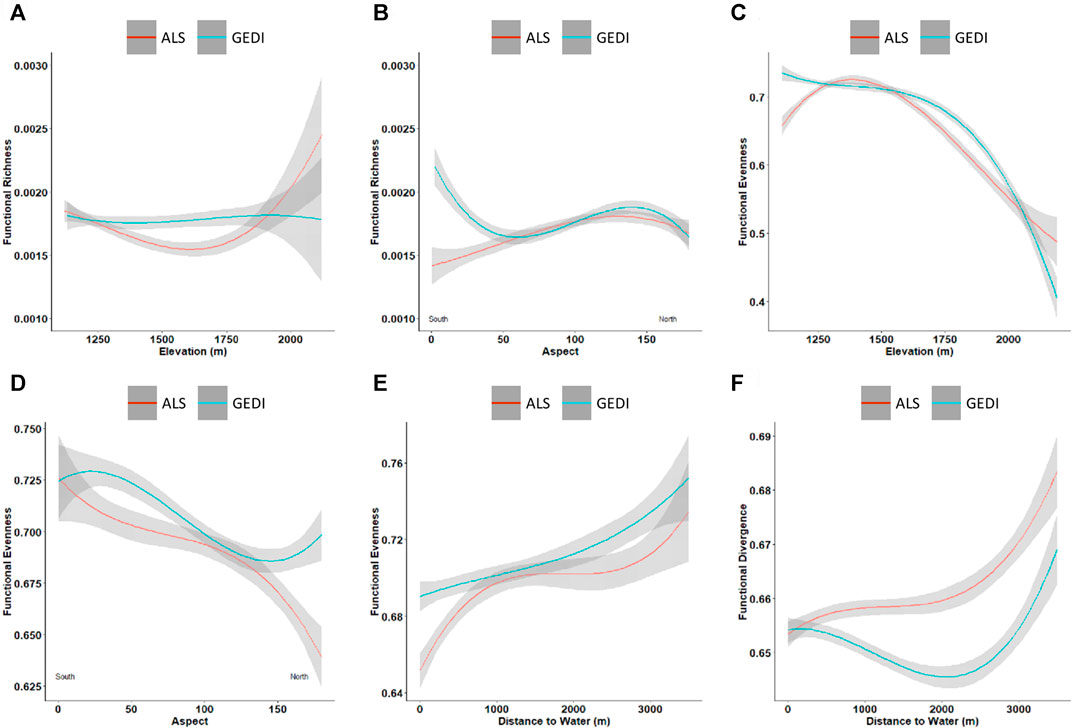
FIGURE 9. Trends between functional diversity and abiotic controls. The red and blue curves represent the mean variation of diversity indices predicted from ALS and GEDI data, respectively, and the surrounding gray area represents the standard deviation. Functional richness with (A) elevation; (B) aspect. Functional evenness with (C) elevation; (D) aspect; (E) distance to water. Functional divergence with (F) distance to water.
Due to the limited coverage of GEDI data (April 2019- August 2020), we did not have enough (>10) GEDI footprints over the burned areas to evaluate the post-fire functional shifts at GEDI scale in this semi-arid ecosystem. However, we emphasize the importance of such a study with appropriate GEDI coverage (two or potentially more years of data collection) in the future, as the GEDI mission aims to address global biodiversity and habitat change, which are directly related to the functional diversity.
Discussion
ALS Based Functional Diversity in Semi-Arid Ecosystems
Our results emphasize that the relative distribution of elevation, soil, distance to water, and aspect control the trends and patterns of functional diversity in this ecosystem. Functional richness is the niche extent in the trait space. We observed large functional richness at high elevations, especially in the southern portion of the study area. This region receives the highest precipitation throughout the year, mostly as snow, which helps maintain perennial streamflow (Harrison et al., 2020). The major soil type is the Harmehl-Gabica association characterized by deep soil profiles, thick surficial A horizons, and high organic matter on north facing slopes (Seyfried et al., 2000). Due to the availability of water, soil nutrients, and solar radiation, high elevation areas provide favorable conditions for conifers, aspen and mountain sagebrush and these species have diverse morphological characteristics. The diverse topographic gradients over short distances limits the spatial extension of this functionally rich zone. Hence, the vegetation in the southern portion of the study area may have higher competition for resources leading to a lower overall functional evenness. In addition, this region shows a mean annual temperature range from 4–16°C providing favorable conditions for vegetation growth. Due to a strong dependency with elevation, we did not include precipitation or temperature as abiotic factors. Future studies including these climatic variables and their temporal variability will help us understand the temporal and spatial dynamics of functional diversity. Contrary to this study, a decrease in functional richness at high elevations due to increases in aridity and decreases in temperatures have been observed in several other studies (Schneider et al., 2017; Durán et al., 2019; Wieczynski et al., 2019).
In contrast, the lower elevations in the central and north portions of the study area are relatively dry with lower and intermittent precipitation. The northern watershed consists of shallow, rocky soils with mesic soil temperatures favoring sagebrush species. This unique and important plant community in the northern Great Basin ecoregion is referred to as the sagebrush-steppe and is co-dominated by big sagebrush and several perennial grasses and forbs. The functional evenness is the distribution of trait abundance in the occupied niche space. Our functional trait maps show that variability in traits in these regions is minimal. Rather than the abundance, the distribution of structurally similar vegetation in the niche space is reflected by the evenness. This further explains the negative correlation between functional richness and evenness. Sparsely distributed, structurally similar shrubs can effectively utilize the entire range of resources available. Hence, we observe functionally even, but functionally less-rich landscapes in the lower elevations of the study area. This low functional richness may also be a reflection of environmental tolerance (beta niches) to disturbance such as grazing (Tilman and Downing 1994). In contrast, the water availability along perennial streams favors the growth of dense, structurally variable riparian vegetation contributing to a relatively low functional evenness. Our results of this increased plant diversity with wetter environmental conditions in water limited environments are confirmed by Harrison et al. (2020). This soil and elevation dependency in functional richness and evenness in this study area are further confirmed by our generalized linear models and are consistent with both ALS and GEDI (Figure 10 A, B, D, and E and Supplementary Figure S10).
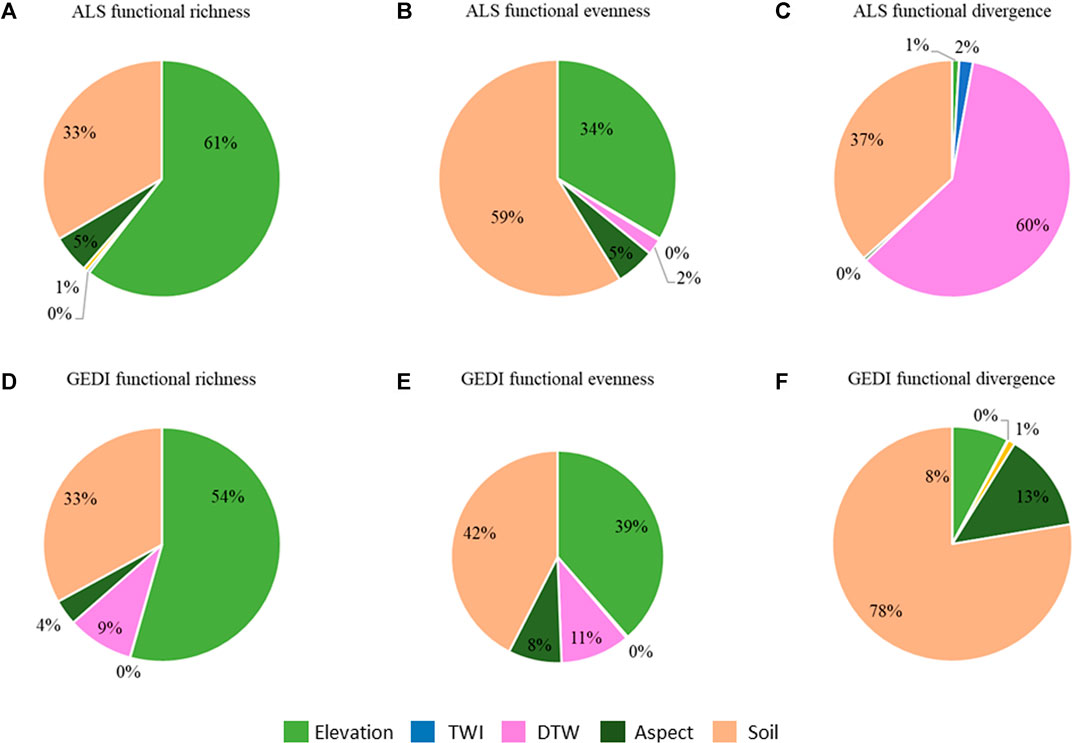
FIGURE 10. Relative importance of each abiotic factor from generalized linear models that explain the variability of diversity indices. Diversity indices estimated from ALS (A–C) and derived from GEDI (D–F).
Remotely sensed functional diversity indicates how the niche is grouped in trait space. Though we observe similar morphological traits at lower elevation and diverse morphological traits at higher elevations, the local grouping or the patchiness of the vegetation is mainly governed by the soils. Our relative importance values resulting from generalized linear models also confirmed elevation, soil, and distance to water as important for functional divergence (Figures 10C, F). The less relative importance of distance to water with the GEDI predicted data could be due to sparseness of the GEDI footprints across the watershed. In addition, water availability provides the necessary wetness for vegetation to grow and survive. Hence, different densities of vegetation can be observed in the trait space leading to dynamic divergence characteristics in the study area, in all ecotones. This explains why the functional divergence shows a trend only with distance to water. The variable functional diversity in the study area may resemble the maximum use of available resources. Overall, this study shows that both the fine resolution airborne lidar can predict functional richness, evenness and divergence of the semi-arid study area and the functional diversity in this study area is mainly driven by the elevation, soil, and water availability.
Functional Diversity Shifts Under Disturbance
Disturbance and time-since-disturbance show a significant influence on driving the functional diversity in the study area. Further, the functional diversity in the fire regions is highly dependent on the spatial neighborhood size as well as the size of fire. While we did not have enough GEDI footprints to characterize the relatively small fires, our GEDI simulations indicate that after 2 years of the mission, GEDI coverage is likely to be sufficient (Ilangakoon, 2020). Similar functional richness over disturbed and undisturbed areas from ALS data may be due to standing dead or burned wood in the disturbed area. Integrating other remote sensing techniques that can differentiate woody materials from leafy vegetation could resolve this (e.g. Li et al., 2018). However, the increase of functional richness of both disturbed and undisturbed areas with the expansion of neighborhood size is due to the potential merging of different traits associations. Larger neighborhood sizes include areas outside of the burn. However, this analysis provides a change in functional diversity compared to purely unburned areas. This helps to understand if an area has undergone a disturbance even if the neighborhood size is bigger than the size of disturbance. This can be especially helpful when using coarse spatial resolution data where the burned areas are only represented by a few pixels (e.g. wall to wall data from MODIS).
All three functional traits and diversity indices show a clear separation between disturbed and undisturbed areas. The differences disappear at large spatial extents though, where functional evenness and divergence of burned and unburned areas converge. This convergence emphasizes the importance of using fine resolution remote sensing data such as ALS to estimate fine-scale disturbance effects. At global scales, this could be potentially accomplished fusing GEDI data with ICESat-2 and Synthetic Aperture Radar (SAR), including the upcoming NISAR (Bae et al., 2019; Qi and Dubayah, 2016, 2019). Though functional richness and divergence show relatively lower values immediately post-fire compared to the undisturbed areas, functional evenness increases immediately after fire. Disturbance results in a functionally different and disconnected landscape from its surrounding. The rate of recovery largely affects native habitat, especially for sage grouse (a threatened species), and potential changes in ecosystem processes. The ideal habitat for sage grouse requires connected mosaics of sagebrush steppe, which allow them safe migration, secure shelter, and food resources (Stiver et al., 2015). In addition, disturbance-driven fragmentation strongly affects biodiversity and resource distributions (e.g. ecological functions and processes), especially along edges of the disturbance (Collinge, 1996). Recovery from disturbance in the face of global change represents a substantial challenge to agencies that manage these lands. Our study shows that assessing functional diversity can help identify areas for restoration or other management activities to consider for treatment.
Stability and post-disturbance recovery of individual species and the community are important components of resilience to disturbance. For example, ecological resilience, an important aspect used to understand ecosystem resilience, is the estimate of post-disturbance vegetation structure recovery (Cole et al., 2014). The resilience of this ecosystem to wildfire thus can be gauged by the rate at which the vegetation recovers in structure and diversity. Our results show that this ecosystem recovers to its background state within 10–15 years, both in terms of functional traits and their diversity (except FHD). The fire return interval of sagebrush-dominated ecosystems can vary from decades to centuries (Baker, 2006; Heyerdahl et al., 2006; Mensing et al., 2006) though the invasion of non-native grass like cheatgrass can shorten fire return intervals (Balch et al., 2013). Based on historic research and our findings, the study area is likely in a stable and resilient state to wildfires. However, with increasing fire size, frequency, and severity, and non-native grass invasion in the western US, this ecosystem may also take an alternative unstable state. Our results further emphasize the unprecedented opportunities to characterize ecosystem resilience in addition to vegetation structure to assess wildfire impacts on ecosystems across spatially continuous scales.
GEDI in Semi-Arid Ecosystems
Functional traits and their diversity in an ecosystem can indicate resource availability and response mechanisms to environmental perturbations such as fires. The potential of GEDI to predict functional traits and diversity of semi-arid ecosystems thus has important global implications. While the correlation between GEDI and ALS estimates of canopy height and FHD confirmed the potential of GEDI in these heterogeneous semi-arid ecosystems, the poor correlation with ALS predicted PAI emphasized the need to further investigate GEDI’s feasibility in low-height vegetation environments with full GEDI coverage. Our study area mainly consists of short shrubs and grass (<5 m) where vegetation return is likely to be mixed in with the ground return. These mixed signals may incorrectly classify vegetation as ground leading to this PAI underestimate. We expect stronger correlation between ALS and GEDI predicted PAI in biomes where there is a clear inflection point between the ground and canopy (in areas where vegetation is taller than 5 m). However, the correlations of field estimated functional traits with those estimated from both the ALS and simulated GEDI data (Ilangakoon, 2020) provide a baseline to understand GEDI’s feasibility and challenges to track trends and patterns of functional traits at regional and global scales in similar ecosystems. In this study, we used real GEDI data with the default algorithm setting for vegetation metric calculations. Our findings may improve by implementing algorithms optimized for the study area, correcting for known differences between the two instruments (e.g., pulse widths of GEDI = 15.6 ns, ALS = 3 ns), and with additional GEDI coverage over time. In addition, correction of ALS functional trait estimates for the reflectance differences between the bare ground and the canopy of different plant functional types may help to constrain the ALS predicted PAI overestimation compared to the field estimates, as well as GEDI estimates. GEDI data version 1 used a default global reflectance ratio (1.5) between the canopy and bare ground while we considered a value of 1 in the ALS estimates due to the observed similarity between the spectral signatures of the ground and the canopy of the dominant plant functional type (shrub). However, the study area also has grass and trees which can cause the ratio of canopy and ground reflectance to vary accordingly. Though we used the LAI profile estimated from the ALS and GEDI data to calculate FHD, the vertical resolution we used for ALS-FHD estimate is 0.20 m whereas it is 5 m for GEDI-FHD estimates (Tang and Armston, 2019). We used 0.20 m layers from the ALS point cloud in order to capture fine-scale variation of FHD and to evaluate GEDI’s feasibility in this ecosystem. The differences in vertical resolution and reflectance ratios between the two datasets may help explain the low to moderate correlation and uncertainties between the ALS and GEDI trait predictions. Derivation of FHD at higher vertical resolution using original georeferenced GEDI waveforms (GEDI 1B product) may provide better FHD values for these types of low height vegetation environments. Further, we observed approximately 48% of GEDI footprints in this study area are on slopes >20° and these slopes influence our GEDI based trait estimates. Future investigations may choose to utilize a slope adaptive method to correct for the influence of the ground slope in waveform lidar predicted metrics as explained in Wang et al. (2019) or applying a physical slope correction (Park et al., 2014).
While 16 months of GEDI sampling is not sufficient to provide a comprehensive description of the disturbance, GEDI’s capability of estimating functional traits (CH and FHD) in this semi-arid ecosystem demonstrates its exciting potential to identify critical biophysical and ecological shifts. Footprint-based GEDI functional diversity estimates showed consistent trends with abiotic factors as with ALS data. Though the sparseness of GEDI footprints (∼20–100 footprints within a 1 km2 area) limited our ability to map functional diversity at local and regional scales, this could be potentially accomplished fusing GEDI data with ICESat-2 and Synthetic Aperture Radar (SAR), including the upcoming NISAR (Bae et al., 2019; Qi and Dubayah, 2016; Qi et al., 2019). This information will help us understand the potential of GEDI to monitor the changes in carbon-cycle dynamics, habitats and biodiversity across the globe in semi-arid ecosystems. The errors in GEDI predicted functional traits in a semi-arid ecosystem such as our study area will result in errors of secondary products such as functional diversity, biomass, and carbon stocks. Hence, it is important to identify methods and algorithms with properly documented workflows appropriate for the ecosystem, such as our efforts here with functional traits. Ultimately, this study highlights the capabilities and opportunities for further research and refinement in order to utilize GEDI for global scale estimates of biomass, carbon, and biodiversity.
Data Availability Statement
Publicly available datasets were analyzed in this study. This data can be found here: 10.3334/ORNLDAAC/1503.
Author Contributions
NI and NG designed the study. NI, NG, HD, and LS processed and analyzed data. NI, NG, FS, HD, SH, LS, and T.G. wrote the paper.
Funding
This work was supported by the NASA Terrestrial Ecology (NNX14AD81G) and NASA Earth and Space Science Fellowship 2017 (NESSF 2017) (17-EARTH17F-0209) programs. The research carried out at the Jet Propulsion Laboratory, California Institute of Technology, was under a contract with the National Aeronautics and Space Administration (80NM0018D0004). This material is also based in part upon work supported by the National Science Foundation through the NEON Program. The National Ecological Observatory Network is a program sponsored by the National Science Foundation and operated under cooperative agreement by Battelle Memorial Institute.
Conflict of Interest
The authors declare that the research was conducted in the absence of any commercial or financial relationships that could be construed as a potential conflict of interest.
Publisher’s Note
All claims expressed in this article are solely those of the authors and do not necessarily represent those of their affiliated organizations, or those of the publisher, the editors and the reviewers. Any product that may be evaluated in this article, or claim that may be made by its manufacturer, is not guaranteed or endorsed by the publisher.
Acknowledgments
The authors would like to thank the USDA Agricultural Research Service, Boise, Idaho, for field access and support, Alex Boehm (Biological Science Technician Plants, USDA, ARS), and Reynolds Creek Critical Zone Observatory (RCCZO) for providing soil, stream network and fire data. We acknowledge Boise Center Aerospace Laboratory, Boise State University for assistance in the field data collection.
Supplementary Material
The Supplementary Material for this article can be found online at: https://www.frontiersin.org/articles/10.3389/frsen.2021.743320/full#supplementary-material
References
Abelleira Martínez, O. J., Fremier, A. K., Günter, S., Ramos Bendaña, Z., Vierling, L., Galbraith, S. M., et al. (2016). Scaling up Functional Traits for Ecosystem Services with Remote Sensing: Concepts and Methods. Ecol. Evol. 6 (13), 4359–4371. doi:10.1002/ece3.2201
Ahrens, C. W., Andrew, M. E., Mazanec, R. A., Ruthrof, K. X., Challis, A., Hardy, G., et al. (2020). Plant Functional Traits Differ in Adaptability and Are Predicted to Be Differentially Affected by Climate Change. Ecol. Evol. 10 (1), 232–248. doi:10.1002/ece3.5890
Bae, S., Levick, S. R., Heidrich, L., Magdon, P., Leutner, B. F., Wöllauer, S., et al. (2019). Radar Vision in the Mapping of forest Biodiversity from Space. Nat. Commun. 10 (1), 4757. doi:10.1038/s41467-019-12737-x
Bagaram, M. B., Giuliarelli, D., Chirici, G., Giannetti, F., and Barbati, A. (2018). UAV Remote Sensing for Biodiversity Monitoring: Are Forest Canopy Gaps Good Covariates. Remote Sensing 10 (9), 1397. doi:10.3390/rs10091397
Baker, W. L. (2006). Fire and Restoration of Sagebrush Ecosystems. Wildl. Soc. Bull. 34 (1), 177–185. doi:10.2193/0091-7648(2006)34[177:farose]2.0.co;2
Balch, J. K., Bradley, B. A., D'Antonio, C. M., and Gómez-Dans, J. (2013). Introduced Annual Grass Increases Regional Fire Activity across the Arid Western USA (1980-2009). Glob. Change Biol. 19 (1), 173–183. doi:10.1111/gcb.12046
Bardgett, R. D., and van der Putten, W. H. (2014). Belowground Biodiversity and Ecosystem Functioning. Nature 515 (7528), 505–511. doi:10.1038/nature13855
Blair, J. B., and Hofton, M. A. (1999). Modeling Laser Altimeter Return Waveforms over Complex Vegetation Using High‐resolution Elevation Data. Geophys. Res. Lett. 26 (16), 2509–2512.
Booth, D. T., Cox, S. E., and Berryman, R. D. (2006). Point Sampling Digital Imagery with 'SamplePoint'. Environ. Monit. Assess. 123 (1-3), 97–108. doi:10.1007/s10661-005-9164-7
Braghiere, R. K., Quaife, T., Black, E., He, L., and Chen, J. M. (2019). Underestimation of Global Photosynthesis in Earth System Models Due to Representation of Vegetation Structure. Glob. Biogeochem. Cycles 33, 1358–1369. doi:10.1029/2018GB006135
Braun, D., Damm, A., Hein, L., Petchey, O. L., and Schaepman, M. E. (2018). Spatio-temporal Trends and Trade-Offs in Ecosystem Services: An Earth Observation Based Assessment for Switzerland between 2004 and 2014. Ecol. Indicators 89, 828–839. doi:10.1016/j.ecolind.2017.10.016
Bu, W., Huang, J., Xu, H., Zang, R., Ding, Y., Li, Y., et al. (2019). Plant Functional Traits Are the Mediators in Regulating Effects of Abiotic Site Conditions on Aboveground Carbon Stock-Evidence from a 30 Ha Tropical forest Plot. Front. Plant Sci. 9, 1958. doi:10.3389/fpls.2018.01958
Cole, L. E., Bhagwat, S. A., and Willis, K. J. (2014). Recovery and Resilience of Tropical Forests after Disturbance. Nat. Commun. 5 (1), 3906–3907. doi:10.1038/ncomms4906
Collinge, S. K. (1996). Ecological Consequences of Habitat Fragmentation: Implications for Landscape Architecture and Planning. Landscape Urban Plann. 36 (1), 59–77. doi:10.1016/s0169-2046(96)00341-6
Conti, G., and Díaz, S. (2013). Plant Functional Diversity and Carbon Storage - an Empirical Test in Semi-arid forest Ecosystems. J. Ecol. 101 (1), 18–28. doi:10.1111/1365-2745.12012
Dashti, H., Glenn, N. F., Ustin, S., Mitchell, J. J., Qi, Y., Ilangakoon, N. T., et al. (2019). Empirical Methods for Remote Sensing of Nitrogen in Drylands May lead to Unreliable Interpretation of Ecosystem Function. IEEE Trans. Geosci. Remote Sensing 57 (6), 3993–4004. doi:10.1109/tgrs.2018.2889318
Dashti, H., Pandit, K., Glenn, N. F., Shinneman, D. J., Flerchinger, G. N., Hudak, A. T., et al. (2021). Performance of the Ecosystem Demography Model (EDv2.2) in Simulating Gross Primary Production Capacity and Activity in a Dryland Study Area. Agric. For. Meteorology 297, 108270. doi:10.1016/j.agrformet.2020.108270
Díaz, S., Hodgson, J. G., Thompson, K., Cabido, M., Cornelissen, J. H. C., Jalili, A., et al. (2004). The Plant Traits that Drive Ecosystems: Evidence from Three Continents. J. vegetation Sci. 15 (3), 295–304. doi:10.1111/j.1654-1103.2004.tb02266.x
Díaz, S., Lavorel, S., de Bello, F., Quetier, F., Grigulis, K., and Robson, T. M. (2007). Incorporating Plant Functional Diversity Effects in Ecosystem Service Assessments. Proc. Natl. Acad. Sci. 104 (52), 20684–20689. doi:10.1073/pnas.0704716104
Dong, L., Tang, S., Min, M., and Veroustraete, F. (2019). Estimation of Forest Canopy Height in Hilly Areas Using Lidar Waveform Data. IEEE J. Sel. Top. Appl. Earth Observations Remote Sensing 12 (5), 1559–1571. doi:10.1109/JSTARS.2019.2908682
Dubayah, R., Blair, J. B., Goetz, S., Fatoyinbo, L., Hansen, M., Healey, S., et al. (2020). The Global Ecosystem Dynamics Investigation: High-Resolution Laser Ranging of the Earth's Forests and Topography. Sci. remote sensing 1, 100002. doi:10.1016/j.srs.2020.100002
Durán, S. M., Martin, R. E., Díaz, S., Maitner, B. S., Malhi, Y., Salinas, N., et al. (2019). Informing Trait-Based Ecology by Assessing Remotely Sensed Functional Diversity across a Broad Tropical Temperature Gradient. Sci. Adv. 5 (12), eaaw8114. doi:10.1126/sciadv.aaw8114
Funk, J. L., Larson, J. E., Ames, G. M., Butterfield, B. J., Cavender‐Bares, J., Firn, J., et al. (2017). Revisiting the H Oly G Rail: Using Plant Functional Traits to Understand Ecological Processes. Biol. Rev. 92 (2), 1156–1173. doi:10.1111/brv.12275
Glenn, N. F., Spaete, L. P., Sankey, T. T., Derryberry, D. R., Hardegree, S. P., and Mitchell, J. J. (2011). Errors in LiDAR-Derived Shrub Height and crown Area on Sloped Terrain. J. Arid Environments 75 (4), 377–382. doi:10.1016/j.jaridenv.2010.11.005
Glenn, N. F., Spaete, L. P., Shrestha, R., Li, A., Ilangakoon, N., Mitchell, J., et al. (2017). Shrubland Species Cover, Biometric, Carbon and Nitrogen Data, Southern Idaho, 2014. Oak Ridge, Tennessee, USA: ORNL DAAC. doi:10.3334/ORNLDAAC/1503
Hancock, S., Armston, J., Hofton, M., Sun, X., Tang, H., Duncanson, L. I., et al. (2019). The GEDI Simulator: A Large‐Footprint Waveform Lidar Simulator for Calibration and Validation of Spaceborne Missions. Earth Space Sci. 6, 294–310. doi:10.1029/2018EA000506
Harrison, S., Spasojevic, M. J., and Li, D. (2020). Climate and Plant Community Diversity in Space and Time. Proc. Natl. Acad. Sci. USA 117, 4464–4470. doi:10.1073/pnas.1921724117
Heyerdahl, E. K., Miller, R. F., and Parsons, R. A. (2006). History of Fire and Douglas-fir Establishment in a savanna and Sagebrush–Grassland Mosaic, Southwestern Montana, USA. For. Ecol. Manag. 230 (1-3), 107–118. doi:10.1016/j.foreco.2006.04.024
Hooper, D., Buchmann, N., Degrange, V., Diaz, S., Gessner, M., Grime, P., et al. (2002). Species Diversity, Functional Diversity and Ecosystem Functioning. Biodiversity Ecosyst. Functioning: A Curr. Synth., 195–208.
Ilangakoon, G. Y. M. N. T. (2020). “Complexity and Dynamics of Semi-arid Vegetation Structure, Function and Diversity across Spatial Scales from Full Waveform Lidar,”. [dissertation thesis] (Boise, ID: Boise State University Theses and Dissertations), 1658. doi:10.18122/td/1658/boisestate
Ilangakoon, N. T., Glenn, N. F., Dashti, H., Painter, T. H., Mikesell, T. D., Spaete, L. P., et al. (2018). Constraining Plant Functional Types in a Semi-arid Ecosystem with Waveform Lidar. Remote Sensing Environ. 209, 497–509. doi:10.1016/j.rse.2018.02.070
Isbell, F., Craven, D., Connolly, J., Loreau, M., Schmid, B., Beierkuhnlein, C., et al. (2015). Biodiversity Increases the Resistance of Ecosystem Productivity to Climate Extremes. Nature 526 (7574), 574–577. doi:10.1038/nature15374
Jetz, W., Cavender-Bares, J., Pavlick, R., Schimel, D., Davis, F. W., Asner, G. P., et al. (2016). Monitoring Plant Functional Diversity from Space. Nat. Plants 2 (3), 16024. doi:10.1038/NPLANTS.2016.24
Kulawardhana, R. W., Popescu, S. C., and Feagin, R. A. (2017). Airborne Lidar Remote Sensing Applications in Non-forested Short Stature Environments: a Review. Ann. For. Res. 60 (1), 173–196. doi:10.15287/afr.2016.719
Li, Z., Strahler, A., Schaaf, C., Jupp, D., Schaefer, M., and Olofsson, P. (2018). Seasonal Change of Leaf and Woody Area Profiles in a Midlatitude Deciduous forest Canopy from Classified Dual-Wavelength Terrestrial Lidar point Clouds. Agric. For. Meteorology 262, 279–297. doi:10.1016/j.agrformet.2018.07.014
Liu, A., Cheng, X., and Chen, Z. (2021). Performance Evaluation of GEDI and ICESat-2 Laser Altimeter Data for Terrain and Canopy Height Retrievals. Remote Sensing Environ. 264, 112571. doi:10.1016/j.rse.2021.112571
MacArthur, R., and MacArthur, J. (1961). On Bird Species Diversity. Ecology 42, 595–599. doi:10.2307/1932254
Marselis, S. M., Tang, H., Armston, J., Abernethy, K., Alonso, A., Barbier, N., et al. (2019). Exploring the Relation between Remotely Sensed Vertical Canopy Structure and Tree Species Diversity in Gabon. Environ. Res. Lett. 14 (9), 094013. doi:10.1088/1748-9326/ab2dcd
Medeiros, C. D., Scoffoni, C., John, G. P., Bartlett, M. K., Inman‐Narahari, F., Ostertag, R., et al. (2019). An Extensive Suite of Functional Traits Distinguishes Hawaiian Wet and Dry Forests and Enables Prediction of Species Vital Rates. Funct. Ecol. 33 (4), 712–734. doi:10.1111/1365-2435.13229
Mensing, S., Livingston, S., and Barker, P. (2006). Long-term Fire History in Great Basin Sagebrush Reconstructed from Macroscopic Charcoal in spring Sediments, Newark Valley, Nevada. West. North Am. Naturalist 66 (1), 64–77. doi:10.3398/1527-0904(2006)66[64:lfhigb]2.0.co;2
Mitchell, J. J., Glenn, N. F., Sankey, T. T., Derryberry, D. R., Anderson, M. O., and Hruska, R. C. (2011). Small-footprint LiDAR Estimations of Sagebrush Canopy Characteristics. Photogramm Eng. Remote Sensing 77 (5), 521–530. doi:10.14358/pers.77.5.521
Mouchet, M. A., Villéger, S., Mason, N. W. H., and Mouillot, D. (2010). Functional Diversity Measures: an Overview of Their Redundancy and Their Ability to Discriminate Community Assembly Rules. Funct. Ecol. 24 (4), 867–876. doi:10.1111/j.1365-2435.2010.01695.x
Norman, J. M., and Campbell, G. S. (1989). “Canopy Structure,” in Plant Physiological Ecology (Dordrecht: Springer), 301–325. doi:10.1007/978-94-009-2221-1_14
Norman, J. M., and Welles, J. M. (1983). Radiative Transfer in an Array of Canopies 1. Agron.j. 75 (3), 481–488. doi:10.2134/agronj1983.00021962007500030016x
Oliva, J., Redondo, M. Á., and Stenlid, J. (2020). Functional Ecology of forest Disease. Annu. Rev. Phytopathol. 58, 343–361. doi:10.1146/annurev-phyto-080417-050028
Pakeman, R. J., and Stockan, J. A. (2014). Drivers of Carabid Functional Diversity: Abiotic Environment, Plant Functional Traits, or Plant Functional Diversity. Ecology 95 (5), 1213–1224. doi:10.1890/13-1059.1
Park, T., Kennedy, R., Choi, S., Wu, J., Lefsky, M., Bi, J., et al. (2014). Application of Physically-Based Slope Correction for Maximum forest Canopy Height Estimation Using Waveform Lidar across Different Footprint Sizes and Locations: Tests on LVIS and GLAS. Remote Sensing 6 (7), 6566–6586. doi:10.3390/rs6076566
Poorter, L., and Markesteijn, L. (2008). Seedling Traits Determine Drought Tolerance of Tropical Tree Species. Biotropica 40 (3), 321–331. doi:10.1111/j.1744-7429.2007.00380.x
Poulter, B., MacBean, N., Hartley, A., Khlystova, I., Arino, O., Betts, R., et al. (2015). Plant Functional Type Classification for Earth System Models: Results from the European Space Agency's Land Cover Climate Change Initiative. Geosci. Model. Dev. 8 (7), 2315–2328. doi:10.5194/gmd-8-2315-2015
Prim, R. C. (1957). Shortest Connection Networks and Some Generalizations. Bell Syst. Tech. J. 36, 1389–1401. doi:10.1002/j.1538-7305.1957.tb01515.x
Qi, W., and Dubayah, R. O. (2016). Combining Tandem-X InSAR and Simulated GEDI Lidar Observations for forest Structure Mapping. Remote Sensing Environ. 187, 253–266. doi:10.1016/j.rse.2016.10.018
Qi, W., Lee, S.-K., Hancock, S., Luthcke, S., Tang, H., Armston, J., et al. (2019). Improved forest Height Estimation by Fusion of Simulated GEDI Lidar Data and TanDEM-X InSAR Data. Remote Sensing Environ. 221, 621–634. doi:10.1016/j.rse.2018.11.035
Riegl (2017). Riegl LMS-Q1560. Available at: http://www.riegl.com/nc/products/airborne-scanning/produktdetail/product/scanner/38/(Accessed September 11, 2017).
Rödig, E., Knapp, N., Fischer, R., Bohn, F. J., Dubayah, R., Tang, H., et al. (2019). From Small-Scale forest Structure to Amazon-wide Carbon Estimates. Nat. Commun. 10 (1), 5088. doi:10.1038/s41467-019-13063-y
Schimel, D. S., Asner, G. P., and Moorcroft, P. (2013). Observing Changing Ecological Diversity in the Anthropocene. Front. Ecol. Environ. 11 (3), 129–137. doi:10.1890/120111
Schimel, D., and Schneider, F. D. (2019). Flux Towers in the Sky: Global Ecology from Space. New Phytol. 224 (2), 570–584. doi:10.1111/nph.15934
Schlesinger, W. H., Reynolds, J. F., Cunningham, G. L., Huenneke, L. F., Jarrell, W. M., Virginia, R. A., et al. (1990). Biological Feedbacks in Global Desertification. Science 247 (4946), 1043–1048. doi:10.1126/science.247.4946.1043
Schneider, F. D., Ferraz, A., Hancock, S., Duncanson, L. I., Dubayah, R. O., Pavlick, R. P., et al. (2020). Towards Mapping the Diversity of Canopy Structure from Space with GEDI. Environ. Res. Lett. 15, 115006. doi:10.1088/1748-9326/ab9e99
Schneider, F. D., Morsdorf, F., Schmid, B., Petchey, O. L., Hueni, A., Schimel, D. S., et al. (2017). Mapping Functional Diversity from Remotely Sensed Morphological and Physiological forest Traits. Nat. Commun. 8 (1), 1441. doi:10.1038/s41467-017-01530-3
Serbin, S. P., Wu, J., Ely, K. S., Kruger, E. L., Townsend, P. A., Meng, R., et al. (2019). From the Arctic to the Tropics: Multibiome Prediction of Leaf Mass Per Area Using Leaf Reflectance. New Phytol. 224 (4), 1557–1568. doi:10.1111/nph.16123
Seyfried, M., Harris, R., Marks, D., and Jacob, B. (2001). Geographic Database, Reynolds Creek Experimental Watershed, Idaho, United States. Water Resour. Res. 37 (11), 2825–2829. doi:10.1029/2001wr000414
Seyfried, M., Lohse, K., Marks, D., Flerchinger, G., Pierson, F., and Holbrook, W. S. (2018). Reynolds Creek Experimental Watershed and Critical Zone Observatory. Vadose zone j. 17 (1), 1–20. doi:10.2136/vzj2018.07.0129
Seyfried, M. S., Harris, R. C., Marks, D., and Jacob, B. (2000). A Geographic Database for Watershed Research, Reynolds Creek Experimental Watershed, Idaho, USA. Tech. Bull. NWRC 3, 2825.
Silva, C. A., Saatchi, S., Garcia, M., Labriere, N., Klauberg, C., Ferraz, A., et al. (2018). Comparison of Small- and Large-Footprint Lidar Characterization of Tropical Forest Aboveground Structure and Biomass: A Case Study from Central Gabon. IEEE J. Sel. Top. Appl. Earth Observations Remote Sensing 11 (10), 3512–3526. doi:10.1109/JSTARS.2018.2816962
Stavros, E. N., Schimel, D., Pavlick, R., Serbin, S., Swann, A., Duncanson, L., et al. (2017). ISS Observations Offer Insights into Plant Function. Nat. Ecol. Evol. 1 (7), 0194. doi:10.1038/s41559-017-0194
Stiver, S. J., Rinkes, E. T., Naugle, D. E., Makela, P. D., Nance, D. A., and Karl, J. W. (2015). Sage-Grouse Habitat Assessment Framework: Multiscale Habitat Assessment Tool. Denver, CO: Bureau of Land Management and Western Association of Fish and Wildlife Agencies Technical Reference, 6710–6711.
Streutker, D. R., and Glenn, N. F. (2006). LiDAR Measurement of Sagebrush Steppe Vegetation Heights. Remote Sensing Environ. 102 (1-2), 135–145. doi:10.1016/j.rse.2006.02.011
Tang, H., and Armston, J. (2019). Algorithm Theoretical Basis Document (ATBD) for GEDI L2B Footprint Canopy Cover and Vertical Profile Metrics. Greenbelt, MD, USA: Goddard Space Flight Center.
Tilman, D., and Downing, J. A. (1994). Biodiversity and Stability in Grasslands. Nature 367 (6461), 363–365. doi:10.1038/367363a0
Villéger, S., Mason, N. W. H., and Mouillot, D. (2008). New Multidimensional Functional Diversity Indices for a Multifaceted Framework in Functional Ecology. Ecology 89 (8), 2290–2301. doi:10.1890/07-1206.1
Violle, C., Reich, P. B., Pacala, S. W., Enquist, B. J., and Kattge, J. (2014). The Emergence and Promise of Functional Biogeography. Proc. Natl. Acad. Sci. 111 (38), 13690–13696. doi:10.1073/pnas.1415442111
Wagner, W. (2010). Radiometric Calibration of Small-Footprint Full-Waveform Airborne Laser Scanner Measurements: Basic Physical Concepts. ISPRS J. Photogrammetry Remote Sensing 65 (6), 505–513. doi:10.1016/j.isprsjprs.2010.06.007
Wang, Y., Ni, W., Sun, G., Chi, H., Zhang, Z., and Guo, Z. (2019). Slope-adaptive Waveform Metrics of Large Footprint Lidar for Estimation of forest Aboveground Biomass. Remote Sensing Environ. 224, 386–400. doi:10.1016/j.rse.2019.02.017
Wang, Z., Chlus, A., Geygan, R., Ye, Z., Zheng, T., Singh, A., et al. (2020). Foliar Functional Traits from Imaging Spectroscopy across Biomes in Eastern North America. New Phytol. 228, 494–511. doi:10.1111/nph.16711
Wieczynski, D. J., Boyle, B., Buzzard, V., Duran, S. M., Henderson, A. N., Hulshof, C. M., Kerkhoff, A. J., McCarthy, M. C., Michaletz, S. T., Swenson, N. G., Asner, G. P., Bentley, L. P., Enquist, B. J., and Savage, V. M. (2019). Climate Shapes and Shifts Functional Biodiversity in Forests Worldwide. Proc. Natl. Acad. Sci. USA 116 (2), 587–592. doi:10.1073/pnas.1813723116
Zheng, G., Ma, L., Eitel, J. U., He, W., Magney, T. S., Moskal, L. M., et al. (2016). Retrieving Directional gap Fraction, Extinction Coefficient, and Effective Leaf Area index by Incorporating Scan Angle Information from Discrete Aerial Lidar Data. IEEE Trans. Geosci. Remote Sensing 55 (1), 577–590.
Keywords: airborne laser scanning, GEDI, full-waveform, functional diversity, drylands, abiotic controls, fire chronosequence, recovery
Citation: Ilangakoon N, Glenn NF, Schneider FD, Dashti H, Hancock S, Spaete L and Goulden T (2021) Airborne and Spaceborne Lidar Reveal Trends and Patterns of Functional Diversity in a Semi-Arid Ecosystem. Front. Remote Sens. 2:743320. doi: 10.3389/frsen.2021.743320
Received: 18 July 2021; Accepted: 25 October 2021;
Published: 12 November 2021.
Edited by:
Sorin C. Popescu, Texas A&M University, United StatesReviewed by:
Craig Glennie, University of Houston, United StatesQin Ma, Mississippi State University, United States
Copyright © 2021 Ilangakoon, Glenn, Schneider, Dashti, Hancock, Spaete and Goulden. This is an open-access article distributed under the terms of the Creative Commons Attribution License (CC BY). The use, distribution or reproduction in other forums is permitted, provided the original author(s) and the copyright owner(s) are credited and that the original publication in this journal is cited, in accordance with accepted academic practice. No use, distribution or reproduction is permitted which does not comply with these terms.
*Correspondence: Nayani Ilangakoon, Z2luaWthbmRhLmlsYW5nYWtvb25AY29sb3JhZG8uZWR1