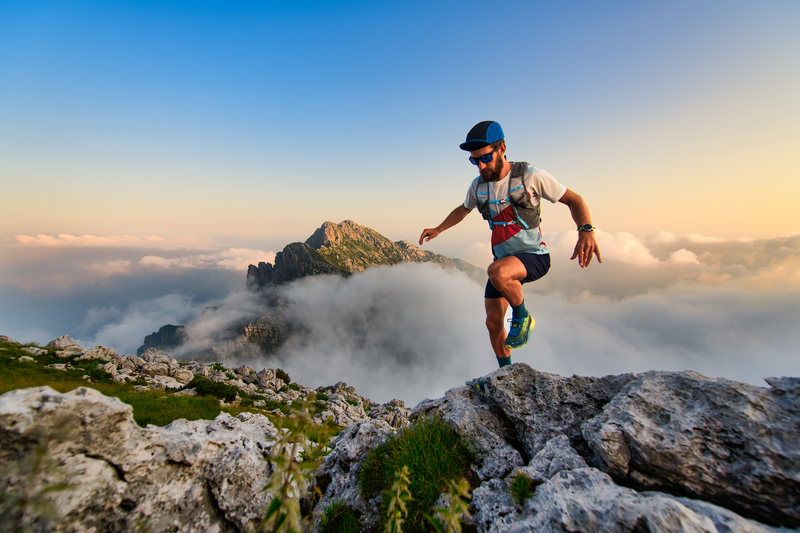
94% of researchers rate our articles as excellent or good
Learn more about the work of our research integrity team to safeguard the quality of each article we publish.
Find out more
ORIGINAL RESEARCH article
Front. Rehabil. Sci.
Sec. Work Rehabilitation
Volume 6 - 2025 | doi: 10.3389/fresc.2025.1545460
This article is part of the Research Topic Mapping the Unseen: Advancements and Innovations in Spatial Epidemiology for Disease Dynamics and Public Health Interventions View all 7 articles
The final, formatted version of the article will be published soon.
You have multiple emails registered with Frontiers:
Please enter your email address:
If you already have an account, please login
You don't have a Frontiers account ? You can register here
Under-reporting of occupational diseases (ODs) could be attributed to poor medical accessibility, which is rarely discussed previously. The aim of our cross-sectional study is to evaluate how ODs reporting is impeded by long travel distance/time (TD/TT) to nearest major occupational medicine clinics. Methods Using data from NODIS, Taiwan's ODs surveillance system, and annual Manpower Survey from 2008 to 2018, we calculate each district's incidence rate of ODs (IROD) and expected IROD based on industries and job titles. Each town's TD/TT to nearest major occupation medicine clinics is estimated by Google Maps' Distance Matrix API. Quasi-Poisson regression model is used to investigate effect of TD and TT on IROD, while industries and job titles are adjusted by offsetting expected IROD. Subgroup analysis is then carried out to check the effect of employment status, sickness absence, and reporting years. Results 3420 cases of definite ODs are included in our study. Using quasi-Poisson regression model, after adjusting industry types and job titles, TD and TT have significant effect on IROD. As TD/TT increase by 10 km/10 mins, IROD decreases by 10.90%/10.73%. It is estimated that around 200 OD cases per year or 40% ODs are therefore under-reported. In subgroup analysis, only mildly-sicked workers are still significantly affected by TD and TT.Our study shows how poor medical accessibility leads to under-reporting, especially for mildly-sicked cases, and up to 40% ODs could be under-reported. Using this method, we can evaluate cost-effectiveness of adding reporting hospital in areas with poor medical accessibility.
Keywords: occupational disease, GIS - Geographic Information System, medical accessibility, Under-reporting, IRR (Incidence Rate Ratio), occupational health centers
Received: 06 Feb 2025; Accepted: 14 Mar 2025.
Copyright: © 2025 Chen, Chu, Huang, Chen, Guo, Su and Chen. This is an open-access article distributed under the terms of the Creative Commons Attribution License (CC BY). The use, distribution or reproduction in other forums is permitted, provided the original author(s) or licensor are credited and that the original publication in this journal is cited, in accordance with accepted academic practice. No use, distribution or reproduction is permitted which does not comply with these terms.
* Correspondence:
Ping Hui Chen, Department of Environmental and Occupational Medicine, National Taiwan University Hospital Hsin-Chu Branch, Hsinchu, Taiwan
Disclaimer: All claims expressed in this article are solely those of the authors and do not necessarily represent those of their affiliated organizations, or those of the publisher, the editors and the reviewers. Any product that may be evaluated in this article or claim that may be made by its manufacturer is not guaranteed or endorsed by the publisher.
Research integrity at Frontiers
Learn more about the work of our research integrity team to safeguard the quality of each article we publish.