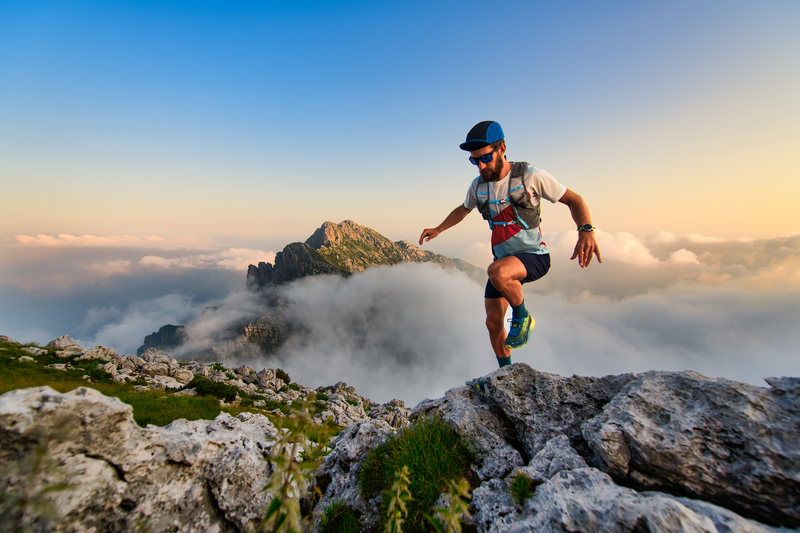
94% of researchers rate our articles as excellent or good
Learn more about the work of our research integrity team to safeguard the quality of each article we publish.
Find out more
ORIGINAL RESEARCH article
Front. Rehabil. Sci.
Sec. Rehabilitation for Musculoskeletal Conditions
Volume 6 - 2025 | doi: 10.3389/fresc.2025.1539804
This article is part of the Research Topic Advancing Muscle Health: From Technical and Clinical Research to Practice View all 3 articles
The final, formatted version of the article will be published soon.
You have multiple emails registered with Frontiers:
Please enter your email address:
If you already have an account, please login
You don't have a Frontiers account ? You can register here
Ultrasound is a cost-effective and reliable method to determine skeletal muscle architecture. However, manual analysis of fascicle length (FL) and pennation angle (PA) can be arduous and subjective among raters, particularly among novice raters. Alternatives to manual processing have been proposed that expedite the evaluation of muscle architecture and afford more consistency. While using algorithms has provided dependable results of muscle architecture, it has often focused on variables of passive range of motion and submaximal contractions. To fully understand the impact of muscle architecture using semi-automated analysis, an investigation of a broad range of contraction intensities is needed. The purpose of this study was to develop and determine the intra-rater and inter-rater reliability of a custom, semi-automated algorithm to extract measures of muscle thickness, pennation angle, and fascicle length, and second to compare the semi-automated measures to measures extracted manually from the same novice raters while accounting for differences between contraction intensities.Methods: Fifteen resistance-trained individuals (male: n = 6, female: n = 9) completed this study. Images were collected during four contraction intensities relative to maximal voluntary isometric contractions (MVIC) (at rest, 30%, 70%, and MVIC) and analyzed by three novice raters to compare the semi-automated algorithm and manual measurement in the vastus lateralis.Results: Intra-rater reliability for manual measures was poor for FL (ICCs: 0-0.30), poor to good for PA (ICCs: 0.46-0.77), and moderate to good for muscle thickness (MT) (ICCs: 0.55 -0.84).For the semi-automated algorithm, the intra-rater reliability was good to excellent for FL (range: 0.90-0.99), PA (range: 0.88-0.99), and MT (range: 0.996-0.999) across all contraction intensities.The findings of this study suggest that the reliability of manual measurement is lower when novice raters perform image analyses compared to the semi-automated method.Therefore, careful consideration and training should be provided when considering manual assessment of muscle architecture values, and standardized identification methods and features in algorithm development may be a better method for reproducibility.
Keywords: ultrasound, Muscle, Fascicle length, Pennation angle, Reliability
Received: 04 Dec 2024; Accepted: 17 Mar 2025.
Copyright: © 2025 Luera, Shields, Bozarth, MacLennan, Walker, Hernandez-Sarabia, Estrada, DeFreitas and Crawford. This is an open-access article distributed under the terms of the Creative Commons Attribution License (CC BY). The use, distribution or reproduction in other forums is permitted, provided the original author(s) or licensor are credited and that the original publication in this journal is cited, in accordance with accepted academic practice. No use, distribution or reproduction is permitted which does not comply with these terms.
* Correspondence:
Scott K Crawford, University of Wisconsin-Madison, Madison, United States
Disclaimer: All claims expressed in this article are solely those of the authors and do not necessarily represent those of their affiliated organizations, or those of the publisher, the editors and the reviewers. Any product that may be evaluated in this article or claim that may be made by its manufacturer is not guaranteed or endorsed by the publisher.
Research integrity at Frontiers
Learn more about the work of our research integrity team to safeguard the quality of each article we publish.