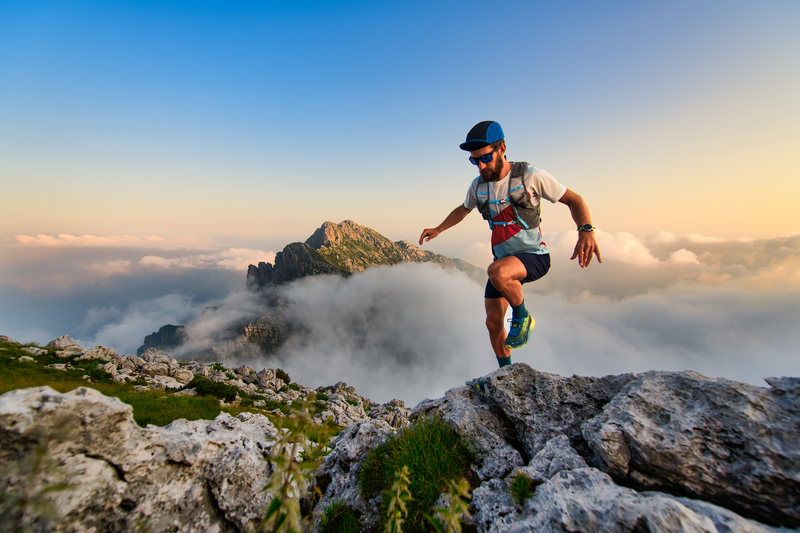
94% of researchers rate our articles as excellent or good
Learn more about the work of our research integrity team to safeguard the quality of each article we publish.
Find out more
MINI REVIEW article
Front. Rehabil. Sci. , 26 January 2024
Sec. Rehabilitation Engineering
Volume 5 - 2024 | https://doi.org/10.3389/fresc.2024.1246773
This article is part of the Research Topic Machine Learning in Rehabilitation Sciences View all 3 articles
Lower limb rehabilitation is essential for recovery post-injury, stroke, or surgery, improving functional mobility and quality of life. Traditional therapy, dependent on therapists' expertise, faces challenges that are addressed by rehabilitation robotics. In the domain of lower limb rehabilitation, machine learning is progressively manifesting its capabilities in high personalization and data-driven approaches, gradually transforming methods of optimizing treatment protocols and predicting rehabilitation outcomes. However, this evolution faces obstacles, including model interpretability, economic hurdles, and regulatory constraints. This review explores the synergy between machine learning and robotic-assisted lower limb rehabilitation, summarizing scientific literature and highlighting various models, data, and domains. Challenges are critically addressed, and future directions proposed for more effective clinical integration. Emphasis is placed on upcoming applications such as Virtual Reality and the potential of deep learning in refining rehabilitation training. This examination aims to provide insights into the evolving landscape, spotlighting the potential of machine learning in rehabilitation robotics and encouraging balanced exploration of current challenges and future opportunities.
Lower limb rehabilitation is vital for recovery after injury, stroke, or surgery, impacting mobility and quality of life (1). Traditional techniques mainly involved physical therapy, including manual exercises. These methods, although effective, had limitations such as dependence on therapist skills and being physically demanding (2).
Technological advances led to mechanized methods like treadmill training with body weight support and robot-assisted therapy. Body-weight-supported treadmill rehabilitation has demonstrated efficacy in stroke recovery (3). Robot-assisted therapy, in particular, has proven to improve walking speed and distance in patients with stroke compared to conventional therapy (4). Nevertheless, they also present challenges such as high costs (5).
Recently, machine learning has shown potential in reducing costs in lower limb rehabilitation, offering solutions that can be managed with less dependency on highly skilled therapists. This cost-efficiency aspect, highlighted in studies such as Das et al. (6), aligns with the growing need for more accessible rehabilitation options (6). Following this, machine learning also plays a pivotal role in optimizing personalized rehabilitation protocols and predict patient outcomes (7). However, challenges like data privacy, the need for extensive datasets, and model interpretability restrict its clinical application (8).
This review explores the potential of integrating machine learning into lower limb rehabilitation, focusing on its enhancement of rehabilitation robotics. It examines applications in optimizing personalized protocols, predicting recovery, and real-time feedback, and discuss challenges in model interpretability, economics, and regulation. Furthermore, it outlines future prospects, such as incorporating deep learning and virtual reality to enhance rehabilitation training. Selected articles are categorized in Table 1. This review emphasizes balancing current challenges with future opportunities in the evolving field of lower limb rehabilitation.
Table 1. Summary of selected scientific literature on machine learning in lower limb rehabilitation robotics.
Robotic-assisted rehabilitation, enhanced by machine learning algorithms, addresses patients' unique needs and conditions to increase lower limb rehabilitation efficiency. This intersection of robotics and machine learning results in highly individualized, adaptive treatment plans, real-time feedback, and sophisticated data analysis (14).
Within the realm of robotic-assisted rehabilitation, Deep Neural Networks (DNNs) play a pivotal role in deciphering intricate biomechanical data and movement patterns captured through devices like gait analysis tools. These networks excel in identifying subtle alterations in gait, allowing the rehabilitation system to adapt interventions precisely to each patient's unique requirements (21, 22). Notably, DNNs have been effectively employed in tailoring robot-assisted exercises based on the severity of paralysis, leading to more effective and personalized therapy outcomes (23). For instance, Just as presented in the study by Esquenazi et al. (9), their research used exoskeletal robotics to stimulate walking rehabilitation of paraplegic patients (9). patients with varying mobility levels receive exercises tailored to their abilities, ensuring optimal progress.
In contrast, Decision Trees emerge as a strategic asset for predicting necessary adaptations in rehabilitation strategies (24). By considering factors such as heart rate, balance, and other biomechanical data, these trees forecast improvements in motor skills during rehabilitation, guiding informed modifications to treatment plans (25). Decision Trees prove invaluable in discerning the most suitable robot-assisted interventions for specific patient groups, such as individuals with varying degrees of upper limb impairment due to chronic stroke (13, 26). This underscores the critical role of Decision Trees in shaping tailored and effective rehabilitation paths.
A successful neuro-rehabilitation journey necessitates precise progress monitoring and anticipatory capabilities, both of which can be facilitated through machine learning techniques (27). For instance, the Lokomat gait trainer deploys Reinforcement Learning (RL) to dynamically calibrate the level of assistance provided to patients, ensuring an appropriate challenge level that aids in fostering recovery (28). Acknowledging that a singular assistance mode may not suffice for diverse patient needs, Keller, Rauter, and Riener devised the As Needed (AAN) path controller. This innovation tailors the assistance mode to the patient's condition, adding a layer of adaptability to conventional robot-assisted systems (12). In addition to real-time tracking, accurate predictions about rehabilitation progress and the optimization of therapeutic approaches heavily depend on historical data. Specialized deep learning models tailored for time series data, including Recurrent Neural Networks (RNNs) and Long Short-Term Memory (LSTM) networks, offer powerful predictive capabilities (11). An illustration of this lies in the ReWalk robot exoskeleton system, which employs supervised learning to fine-tune assistance intensity based on previous therapy data, ensuring a consistent trajectory of rehabilitation progress (29, 30). In essence, the synergy between advanced machine learning models like Deep Neural Networks, Decision Trees, Reinforcement Learning, and time-sensitive models such as RNNs and LSTMs is pivotal in revolutionizing the landscape of robotic-assisted rehabilitation. These models collectively enable meticulous analysis of biometric data, tailoring of treatment plans, and precise tracking of recovery progress, culminating in highly effective and personalized therapeutic interventions for patients.
Exploring machine learning's role in lower limb rehabilitation, it's essential to contrast it with traditional methods. Traditional approaches have relied on manual or mechanical techniques, but machine learning introduces enhanced capabilities, significantly enriching rehabilitation processes (18). Machine learning, especially in lower limb rehabilitation robotics (MLLERR), excels in action intention recognition and gait analysis. An example is the innovative use of a Bayesian optimization algorithm, adeptly bridging the gap between virtual simulations and real-world application (17, 31). This approach was demonstrated in a study using offline data mining and machine learning to predict and evaluate movements in human exoskeleton systems (16).
In lower limb exoskeletons, a standout is the advanced squatting action controller. It showed remarkable accuracy in joint angle tracking, maintaining performance even under a 100N external disturbance force, highlighting its reliability (19). Similarly, a human motion recognition system achieved an impressive 99.61% accuracy in identifying various actions, including falls (17).
Machine learning notably impacts lower limb rehabilitation, enhancing motion controller and exoskeleton performance (15). It can significantly reduce knee extension muscle load during assisted squats, demonstrating its potential in easing physical strain (32, 33). Though still emerging in prosthetics and orthotics, machine learning promises more efficient management of prostheses and orthoses (34).
Table 2 delineates machine learning's benefits over traditional methods in this field. In essence, while traditional techniques form the bedrock of lower limb rehabilitation, machine learning's integration promises increased accuracy, adaptability, and tailored care, addressing the complexities of human gait. Its evolving role is poised to become increasingly pivotal in rehabilitation (35).
A clinically-based research is needed to maximise lower limb rehabilitation efficacy utilising machine learning and rehabilitative robots. This goal can only be achieved this way. To complete the task, this is the only method. In clinical rehabilitation, Erdaş, Sümer and Kibaroğlu found that a deep learning model, specifically a convolutional neural network (CNN), is better at predicting stroke patients' gait patterns. CNN predicted gait patterns more accurately which was shown by the CNN's superior accuracy than other models. Convolutional neural networks excel at this. This is because the model outperformed conventional methods because this model outperformed established statistical methodologies (36). However, Katakam et al. found that a random forest model predicts functional gains in orthopaedic patients equally well. Closer inspection revealed that these people have improved their functioning. This measure was taken to anticipate functional capability enhancements (37). Machine learning models' accuracy and efficacy are crucial to their usability. This is one of the most important factors in using these models in therapy. Deep neural networks, random forests, and support vector machines have variable degrees of success in predicting rehabilitation results across research (38). This must be considered to ensure accuracy. Remembering this information is crucial for this kind of challenges. Selecting the right model requires careful consideration of the clinical scenario's environmental factors. This is because clinical situations include many environmental factors. If this approach is being followed, it will make sure the model works well and is relevant to the rehabilitation situation being reviewed. Applying machine learning to real-world situations is a major challenge to smooth integration into clinical operations. This is a crucial hurdle to overcome in order to complete the job. Vollmer et al. highlighted the importance of researching both the accuracy of machine learning applications and the practical challenges of implementing them in clinical settings. This research should address the issues raised. This research is necessary to highlight the problem's severity. Performing a comparative study was particularly harder because the patients were different and there were several rehabilitation methods (39). Some researcher recommended adapting machine learning models to clinical situations. Models may work effectively in controlled situations but struggle to meet patient needs in clinical practice. This is because clinical practice feels more natural (40). These models had several flaws, as indicated. It is crucial to have multi-modal data sources. It showed that biomechanical data and patient-reported outcomes can predict knee rehabilitation patients' functional outcomes (41). This was shown by researchers. This method was used to predict functional application results. This comprehensive strategy, which improves machine learning application efficiency, allows for specialized insights into rehabilitation sequences. Evaluation and explanation are crucial when implementing machine learning models in healthcare. Models that provide explicit explanations of predictions and such explanations are crucial, since such models supply both explanations. These models help doctors build trust with patients and make informed judgements. Together with machine insights, this is done (42). A thorough comparison of machine learning models in clinical rehabilitation data is needed to optimize lower limb rehabilitation. This research is necessary for progress. This requirement must be met to achieve desired results. This will enable therapy efficacy improvements, which is vital. Researchers can contribute to the machine learning-rehabilitative robotics conversation by addressing efficacy, accuracy, real-world applicability, patient heterogeneity, multi-modal data integration, and interpretability. Since these fields intersect, the conversation is happening there. This is because the discourse is happening where these two fields intersect. Due to this situation, they can shape the future of customized and effective lower limb rehabilitation treatments.
Interpretability in machine learning models is crucial, especially in lower limb rehabilitation (43). This refers to the degree to which a human can comprehend the decision-making process of a machine learning model (44). In the medical domain, and particularly in lower limb rehabilitation, understanding the rationale behind a model's recommendations is paramount. This is because the stakes are high, and decisions directly impact a patient's health and quality of life.
Lack of interpretability can lead to a variety of issues. First, if a model's decisions cannot be understood or explained, it can lead to mistrust from patients, which can hinder their rehabilitation progress (45). For example, if a robotic-assisted therapy device, guided by a machine learning algorithm, recommends a specific exercise regimen, the patient might be hesitant to comply if the rationale is not clear. Second, uninterpretable models can be dangerous in healthcare settings as incorrect interpretation can lead to inappropriate treatment decisions (46). Consider a scenario where an algorithm predicts a slow recovery trajectory; if clinicians cannot understand how this prediction was derived, they might make ill-informed adjustments to the therapy. Lastly, lack of interpretability can pose a challenge in terms of regulatory compliance, as healthcare is a highly regulated field that often requires an explanation of treatment decisions (47–49).
Strategies to improve model interpretability are, therefore, critical. One approach is to use simpler, easily interpretable models like linear regression or decision trees (44). However, this may compromise prediction accuracy. Alternatively, post-hoc interpretation methods like LIME or SHAP can be used to explain complex models (50). These methods approximate the model's decisions locally using a simpler model. Another strategy is to incorporate domain knowledge in model development. This can help in creating models that align with well-understood clinical principles, thereby improving interpretability (51).
AI-assisted health and medical devices face significant challenges, particularly in economic, regulatory, and practical contexts. Lower limb rehabilitation robots require substantial investment, posing accessibility issues for patients, especially in resource-constrained scenarios (52). Although research suggests that eventual cost savings could arise from improved patient outcomes and operational efficiency, the initial investment required for rehabilitation robots is a substantial hurdle. It's crucial to acknowledge and address the substantial upfront expenses by devising approaches to enhance affordability and accessibility, thereby promoting broader adoption (53). Also, strategies to minimize costs and enhance coverage, such as improving technical designs, optimizing manufacturing processes, and implementing effective rehabilitation strategies, should be prioritized in future studies (54).
The medical sector operates within strict regulatory frameworks aimed at safeguarding patient well-being and treatment effectiveness. Navigating the intricate journey from experimental prototypes to commercially viable AI-assisted rehabilitation devices involves traversing complex regulatory pathways. Demonstrating compliance with stringent medical device regulations, including obtaining approvals from regulatory bodies, entails a time-consuming and resource-intensive endeavor. Collaborative efforts among researchers, manufacturers, and regulatory authorities are indispensable to expedite and streamline this transitional process (55).
Additionally, AI-assisted rehabilitation faces implementation challenges. Rehabilitation robots require specialized knowledge, posing a significant hurdle for healthcare professionals (56, 57). The adaptation of new rehabilitation techniques may pose challenges due to changes required in existing treatment plans and demands on the implementation environment (10, 58, 59).
High equipment costs and maintenance needs can also reduce acceptance (20, 60). However, the potential benefits of AI and rehabilitation robots in lower limb rehabilitation are compelling (61, 62). Personalized rehabilitation treatments provided by robots like Amadeo offer improved rehabilitation efficiency (63).
The triumph of AI-assisted lower limb rehabilitation hinges upon securing the trust and active participation of both patients and clinicians. Patients must feel assured and grasp the advantages of these technologies in their recovery journey. Skepticism or lack of comprehension concerning AI-driven recommendations can impede patient adherence. Moreover, clinicians' endorsement of AI assistance is pivotal, as they play a crucial role in treatment determinations. Fostering alignment between technological progress and the preferences of patients and clinicians necessitates adept communication and comprehensive education (64).
One of the most important areas to examine in the future of this discipline is new applications and machine learning techniques in therapeutic rehabilitation. Even though the research has illuminated current methods, there is still room for creative approaches. One of the significant field of the research is to find the small solutions that may enhance the treatment outcomes. This objective of this research can be achieved with the help of clinical rehabilitation as well as machine learning research. Rehabilitation programs may benefit from a more personalized approach that tailor's therapy to patients' needs. This strategy tailor's therapy to each patient's qualities and reactions. Investigating machine learning model scalability and generalizability across clinical populations may lead to further research. Therefore, it is crucial to understand how these models function across a wide range of demographic groupings to ensure their efficacy in various settings. This personalized method makes machine learning deployment more flexible. Through equity, this technique helps create accessible and equitable rehabilitation solutions for all. Real-time monitoring and feedback systems also make investigating extra study intriguing. Researchers can create machine learning algorithms that dynamically tailor rehabilitation therapy to patient needs. These models use wearable sensors and continuous data streams. This is achievable because these models learn from their own experiences. This proactive and adaptive strategy could revolutionize rehabilitation by providing timely and individualized therapies. These interventions help people recover from injuries during the dynamic rehabilitation process. To make matters worse, one of the best areas of future research is the ethical implications of machine learning in therapeutic rehabilitation. One of the most important subjects to study. This is one of the most important topics of research. The importance of addressing data privacy, authorization, and algorithmic bias is unquestionable given the growing adoption of these technologies in healthcare. Researchers can help rehabilitation settings use machine learning ethically by investigating and addressing ethical issues. Ethical assessment and minimization can achieve this. This technique will help patients trust doctors.
This extensive analysis of machine learning in clinical rehabilitation shows the many ways these technologies can improve patient outcomes. The research shows how machine learning has been used for targeted treatments, predictive modelling, and result evaluations. The ever-changing clinical rehabilitation environment, which emphasizes customized and flexible therapies, requires acknowledging changes. The appraisal of future research shows how important it is to comprehend clinical rehabilitation and machine learning. The junction is where the two fields intersect, explaining this disparity. The current trend involves a paradigm shift towards tailored medicines that consider each patient's uniqueness. This proposal is part of the planned path. This will make therapy more targeted and successful as possible. The scalability and generalizability of machine learning models across many populations have become vital, emphasizing the relevance of inclusion in healthcare operations. This is because these models can be applied to many people. Implementing real-time monitoring and feedback mechanisms could change rehabilitation processes. This advances the field greatly. Wearable technologies and continuous data streams can simplify dynamic intervention modifications to meet patients' changing needs. This can be achieved by adopting these technologies. Despite these technological advances, managing the ethical aspects of machine learning in healthcare is crucial. This is because these apps could change patients' lives. To integrate these technologies responsibly and trustworthily, data privacy, consent, and algorithmic bias must be addressed.
XZ penned the initial draft. All authors partook in the manuscript's development by contributing content and rigorously critiquing it for substantial intellectual contribution. All authors contributed to the article and approved the submitted version.
This research was funded by the Key Project of Guangxi Science and Technology Department (grant no. AB22080096), and the National Natural Science Foundation of China (grant no. 81860274).
The authors declare that the research was conducted in the absence of any commercial or financial relationships that could be construed as a potential conflict of interest.
All claims expressed in this article are solely those of the authors and do not necessarily represent those of their affiliated organizations, or those of the publisher, the editors and the reviewers. Any product that may be evaluated in this article, or claim that may be made by its manufacturer, is not guaranteed or endorsed by the publisher.
1. Ülger Ö, Yıldırım Şahan T, Çelik SE. A systematic literature review of physiotherapy and rehabilitation approaches to lower-limb amputation. Physiother Theory Pract. (2018) 34:821–34. doi: 10.1080/09593985.2018.1425938
2. Todhunter-Brown A, Baer G, Campbell P, Choo PL, Morris J, Langhorne P, et al. Physical rehabilitation approaches for the recovery of function and mobility following stroke. Cochrane Database Syst Rev. (2014) 2023. doi: 10.1002/14651858.CD001920.pub3
3. Duncan PW, Sullivan KJ, Behrman AL, Azen SP, Wu SS, Nadeau SE, et al. Body-weight–supported treadmill rehabilitation after stroke. N Engl J Med. (2011) 364:2026–36. doi: 10.1056/NEJMoa1010790
4. Molteni F, Gasperini G, Cannaviello G, Guanziroli E. Exoskeleton and end-effector robots for upper and lower limbs rehabilitation: narrative review. PM&R. (2018) 10:S174–88. doi: 10.1016/j.pmrj.2018.06.005
5. Gailey R, Gaunaurd I, Raya M, Kirk-Sanchez N, Prieto-Sanchez LM, Roach K. Effectiveness of an evidence-based amputee rehabilitation program: a pilot randomized controlled trial. Phys Ther. (2020) 100:773–87. doi: 10.1093/ptj/pzaa008
6. Das A, Day TW, Kulkarni V, Buchanan A, Cottrell K, John NW, et al. Towards intelligent extended reality in stroke rehabilitation. In: Augmenting Neurological Disorder Prediction and Rehabilitation Using Artificial Intelligence. Cambridge: Academic Press (2022). p. 309–29. doi: 10.1016/B978-0-323-90037-9.00006-0
7. Cui W, Huang L, Tian Y, Luo H, Chen S, Yang Y, et al. Effect and mechanism of mirror therapy on lower limb rehabilitation after ischemic stroke: a fMRI study. NRE. (2022a) 51:65–77. doi: 10.3233/NRE-210307
8. Webster JB, Crunkhorn A, Sall J, Highsmith MJ, Pruziner A, Randolph BJ. Clinical practice guidelines for the rehabilitation of lower limb amputation: an update from the department of veterans affairs and department of defense. Am J Phys Med Rehabil. (2019) 98:820–9. doi: 10.1097/PHM.0000000000001213
9. Esquenazi A, Talaty M, Packel A, Saulino M. The ReWalk powered exoskeleton to restore ambulatory function to individuals with thoracic-level motor-complete spinal cord injury. Am J Phys Med Rehabil. (2012) 91:911–21. doi: 10.1097/PHM.0b013e318269d9a3
10. Alcobendasmaestro M, Esclarínruz A, Casadolópez RM. Lokomat robotic-assisted versus overground training within 3–6 months of incomplete spinal cord lesion: randomized controlled trial. Neurorehabil Neural Repair. (2012) 26(9):1058–63. doi: 10.1177/1545968312448232
11. Chen C, Du Z, He L, Shi Y, Wang J, Dong W. A novel gait pattern recognition method based on LSTM-CNN for lower limb exoskeleton. J Bionic Eng. (2021) 18:1059–72. doi: 10.1007/s42235-021-00083-y
12. Keller U, Rauter G, Riener R. Assist-as-needed path control for the PASCAL rehabilitation robot. 2013 IEEE 13th International Conference on Rehabilitation Robotics (ICORR); Seattle, WA: IEEE (2013). p. 1–7.
13. Coscia M, Wessel MJ, Chaudary U, Millán JDR, Micera S, Guggisberg A, et al. Neurotechnology-aided interventions for upper limb motor rehabilitation in severe chronic stroke. Brain. (2019) 142:2182–97. doi: 10.1093/brain/awz181
14. Mayor-Torres JM, O’Callaghan B, Korik A, Del Felice A, Coyle D, Murphy S, et al. Robotic-assisted gait for lower-limb rehabilitation: evidence of altered neural mechanisms in stroke. medRxiv [preprint]. (2022). doi: 10.1101/2022.02.01.22269218
15. Baud R, Manzoori AR, Ijspeert A, Bouri M. Review of control strategies for lower-limb exoskeletons to assist gait. J NeuroEngineering Rehabil. (2021) 18:119. doi: 10.1186/s12984-021-00906-3
16. Liu M, Peng B, Shang M. Lower limb movement intention recognition for rehabilitation robot aided with projected recurrent neural network. Complex Intell Syst. (2022) 8:2813–24. doi: 10.1007/s40747-021-00341-w
17. Zhang P, Gao X, Miao M, Zhao P. Design and control of a lower limb rehabilitation robot based on human motion intention recognition with multi-source sensor information. Machines. (2022) 10:1125. doi: 10.3390/machines10121125
18. Gao X, Zhang P, Peng X, Zhao J, Liu K, Miao M, et al. Autonomous motion and control of lower limb exoskeleton rehabilitation robot. Front Bioeng Biotechnol. (2023) 11:1223831. doi: 10.3389/fbioe.2023.1223831
19. Luo S, Androwis G, Adamovich S, Su H, Nunez E, Zhou X. Reinforcement learning and control of a lower extremity exoskeleton for squat assistance. Front Robot AI. (2021) 8:702845. doi: 10.3389/frobt.2021.702845
20. Cui Z, Li Y, Huang S, Wu X, Fu X, Liu F, et al. BCI system with lower-limb robot improves rehabilitation in spinal cord injury patients through short-term training: a pilot study. Cogn Neurodyn. (2022b) 16:1283–301. doi: 10.1007/s11571-022-09801-6
21. Shih C-J, Kuo T-Y, Li Y-C, Chen S-F, Lin C-H, Wang F-C. Stroke-gait detection by DNN models employing gait data from various sensor systems. 2021 International Conference on System Science and Engineering (ICSSE); Ho Chi Minh City, Vietnam: IEEE (2021). p. 195–9.
22. Wei Y, Cao Q, Gu J, Otseidu K, Hargrove L. A fully-integrated gesture and gait processing SoC for rehabilitation with ADC-less mixed-signal feature extraction and deep neural network for classification and online training. 2020 IEEE Custom Integrated Circuits Conference (CICC); Boston, MA, USA: IEEE (2020). p. 1–4.
23. Takebayashi T, Uchiyama Y, Okita Y, Domen K. Development of a program to determine optimal settings for robot-assisted rehabilitation of the post-stroke paretic upper extremity: a simulation study. Sci Rep. (2023) 13:9217. doi: 10.1038/s41598-023-34556-3
24. Bouteraa Y, Abdallah IB. Robot-assisted remote rehabilitation. 2019 International Conference on Signal, Control and Communication (SCC); Hammamet, Tunisia: IEEE (2019). p. 337–43.
25. Lee SH, Park G, Cho DY, Kim HY, Lee J-Y, Kim S, et al. Comparisons between end-effector and exoskeleton rehabilitation robots regarding upper extremity function among chronic stroke patients with moderate-to-severe upper limb impairment. Sci Rep. (2020) 10:1806. doi: 10.1038/s41598-020-58630-2
26. Wu J, Cheng H, Zhang J, Yang S, Cai S. Robot-assisted therapy for upper extremity motor impairment after stroke: a systematic review and meta-analysis. Phys Ther. (2021) 101:pzab010. doi: 10.1093/ptj/pzab010
27. Zhang W, Zhang S, Ceccarelli M. Design and Kinematic Analysis of a Novel Metamorphic Mechanism for Lower Limb Rehabilitation. Cham: Springer (2016).
28. Yang J, Sun T, Cheng L, Hou Z-G. Spatial repetitive impedance learning control for robot-assisted rehabilitation. IEEE/ASME Trans Mechatron. (2023) 28:1280–90. doi: 10.1109/TMECH.2022.3221931
29. Nunez EH, Michael PA, Foulds R. 2-DoF ankle-foot system: implementation of balance for lower extremity exoskeletons. 2017 International Symposium on Wearable Robotics and Rehabilitation (WeRob); Houston, TX: IEEE (2017). p. 1–2.
30. Rodríguez-Fernández A, Lobo-Prat J, Font-Llagunes JM. Systematic review on wearable lower-limb exoskeletons for gait training in neuromuscular impairments. J NeuroEngineering Rehabil. (2021) 18:22. doi: 10.1186/s12984-021-00815-5
31. Zhao C, Zhang X, Guo Q. The application of machine-learning on lower limb motion analysis in human exoskeleton system. In: Ge SS, Khatib O, Cabibihan J-J, Simmons R, Williams M-A, editors. Social Robotics Lecture Notes in Computer Science. Heidelberg, Berlin: Springer Berlin Heidelberg (2012). p. 600–11.
32. Yu W, Kumar VC, Turk G, Liu CK. Sim-to-Real transfer for biped locomotion. 2019 IEEE/RSJ International Conference on Intelligent Robots and Systems (IROS); Macau, China: IEEE (2019). p. 3503–10.
33. Guan W, Zhou L, Cao Y. Joint motion control for lower limb rehabilitation based on iterative learning control (ILC) algorithm. Complexity. (2021) 2021:1–9. doi: 10.1155/2021/6651495
34. Choo YJ, Chang MC. Use of machine learning in the field of prosthetics and orthotics: a systematic narrative review. Prosthet Orthot Int. (2023) 47:226–40. doi: 10.1097/PXR.0000000000000199
35. Wang Y, Li Z, Wang X, Yu H, Liao W, Arifoglu D. Human gait data augmentation and trajectory prediction for lower-limb rehabilitation robot control using GANs and attention mechanism. Machines. (2021) 9:367. doi: 10.3390/machines9120367
36. Erdaş ÇB, Sümer E, Kibaroğlu S. Neurodegenerative disease detection and severity prediction using deep learning approaches. Biomed Signal Process Control. (2021) 70:103069. doi: 10.1016/j.bspc.2021.103069
37. Katakam A, Karhade AV, Collins A, Shin D, Bragdon C, Chen AF, et al. Development of machine learning algorithms to predict achievement of minimal clinically important difference for the KOOS-PS following total knee arthroplasty. J Orthop Res. (2022) 40:808–15. doi: 10.1002/jor.25125
38. Oztekin A, Delen D, Turkyilmaz A, Zaim S. A machine learning-based usability evaluation method for eLearning systems. Decis Support Syst. (2013) 56:63–73. doi: 10.1016/j.dss.2013.05.003
39. Vollmer S, Mateen BA, Bohner G, Király FJ, Ghani R, Jonsson P, et al. Machine learning and artificial intelligence research for patient benefit: 20 critical questions on transparency, replicability, ethics, and effectiveness. Br Med J. (2020) 368:l6927. doi: 10.1136/bmj.l6927
40. Lalmuanawma S, Hussain J, Chhakchhuak L. Applications of machine learning and artificial intelligence for COVID-19 (SARS-CoV-2) pandemic: a review. Chaos Solitons Fractals. (2020) 139:110059. doi: 10.1016/j.chaos.2020.110059
41. Whatling GM, Biggs PR, Wilson C, Holt CA. Assessing functional recovery following total knee replacement surgery using objective classification of level gait data and patient-reported outcome measures. Clin Biomech. (2022) 95:105625. doi: 10.1016/j.clinbiomech.2022.105625
42. Hofman JM, Watts DJ, Athey S, Garip F, Griffiths TL, Kleinberg J, et al. Integrating explanation and prediction in computational social science. Nature. (2021) 595:181–8. doi: 10.1038/s41586-021-03659-0
43. Guidotti R, Monreale A, Ruggieri S, Turini F, Giannotti F, Pedreschi D. A survey of methods for explaining black box models. ACM Comput Surv. (2019) 51:1–42. doi: 10.1145/3236009
44. Rudin C. Stop explaining black box machine learning models for high stakes decisions and use interpretable models instead. Nat Mach Intell. (2019) 1:206–15. doi: 10.1038/s42256-019-0048-x
45. the Precise4Q consortium, Amann J, Blasimme A, Vayena E, Frey D, Madai VI. Explainability for artificial intelligence in healthcare: a multidisciplinary perspective. BMC Med Inform Decis Mak. (2020) 20:310. doi: 10.1186/s12911-020-01332-6
46. Stiglic G, Kocbek P, Fijacko N, Zitnik M, Verbert K, Cilar L. Interpretability of machine learning-based prediction models in healthcare. WIRES Data Mining Knowl Discov. (2020) 10:e1379. doi: 10.1002/widm.1379
47. Bhardwaj R, Nambiar AR, Dutta D. A study of machine learning in healthcare. 2017 IEEE 41st Annual Computer Software and Applications Conference (COMPSAC); Turin: IEEE (2017). p. 236–41.
48. Shailaja K, Seetharamulu B, Jabbar MA. Machine learning in healthcare: a review. 2018 Second International Conference on Electronics, Communication and Aerospace Technology (ICECA); Coimbatore: IEEE (2018). p. 910–4.
49. Samarpita S, Satpathy RN. Applications of machine learning in healthcare: an overview. 2022 1st IEEE International Conference on Industrial Electronics: Developments & Applications (ICIDeA); Bhubaneswar, India: IEEE (2022). p. 51–6.
50. Ribeiro MT, Singh S, Guestrin C. “Why should I trust you?”: explaining the predictions of any classifier. Proceedings of the 22nd ACM SIGKDD International Conference on Knowledge Discovery and Data Mining; San Francisco California, USA: ACM (2016). p. 1135–44.
51. Caruana R, Lou Y, Gehrke J, Koch P, Sturm M, Elhadad N. Intelligible models for HealthCare: predicting pneumonia risk and hospital 30-day readmission. Proceedings of the 21th ACM SIGKDD International Conference on Knowledge Discovery and Data Mining; Sydney, NSW, Australia: ACM (2015). p. 1721–30.
52. Taylor RH, Menciassi A, Fichtinger G, Dario P. Medical robotics and computer-integrated surgery. In: Siciliano B, Khatib O, editors. Springer Handbook of Robotics. Berlin, Heidelberg: Springer Berlin Heidelberg (2008). p. 1199–222.
53. Calabrò RS, Müller-Eising C, Diliberti ML, Manuli A, Parrinello F, Rao G, et al. Who will pay for robotic rehabilitation? The growing need for a cost-effectiveness analysis. Innov Clin Neurosci. (2020) 17:14–6.
54. Terranova TT, Simis M, Santos ACA, Alfieri FM, Imamura M, Fregni F, et al. Robot-assisted therapy and constraint-induced movement therapy for motor recovery in stroke: results from a randomized clinical trial. Front Neurorobot. (2021) 15:684019. doi: 10.3389/fnbot.2021.684019
55. Zidaru T, Morrow EM, Stockley R. Ensuring patient and public involvement in the transition to AI-assisted mental health care: a systematic scoping review and agenda for design justice. Health Expect. (2021) 24:1072–124. doi: 10.1111/hex.13299
56. Shi D, Zhang W, Zhang W, Ding X. A review on lower limb rehabilitation exoskeleton robots. Chin J Mech Eng. (2019) 32:74. doi: 10.1186/s10033-019-0389-8
57. Akbari A, Haghverd F, Behbahani S. Robotic home-based rehabilitation systems design: from a literature review to a conceptual framework for community-based remote therapy during COVID-19 pandemic. Front Robot AI. (2021) 8:612331. doi: 10.3389/frobt.2021.612331
58. Aurich-Schuler T, Labruyère R. An increase in kinematic freedom in the lokomat is related to the ability to elicit a physiological muscle activity pattern: a secondary data analysis investigating differences between guidance force, path control, and FreeD. Front Robot AI. (2019) 6:109. doi: 10.3389/frobt.2019.00109
59. Hobbs B, Artemiadis P. A review of robot-assisted lower-limb stroke therapy: unexplored paths and future directions in gait rehabilitation. Front Neurorobot. (2020) 14:19. doi: 10.3389/fnbot.2020.00019
60. Kolakowsky-Hayner SA, Crew J, Moran S. Safety and feasibility of using the EksoTM bionic exoskeleton to aid ambulation after spinal cord injury. J Spine. (2013) S4:003. doi: 10.4172/2165-7939.S4-003
61. Yu H, Huang S, Chen G. Human–robot interaction control of rehabilitation robots with series elastic actuators. IEEE Trans Robot. (2015) 31(5):1089–100. doi: 10.1109/TRO.2015.2457314
62. Mohebbi A. Human-robot interaction in rehabilitation and assistance: a review. Curr Robot Rep. (2020) 1:131–44. doi: 10.1007/s43154-020-00015-4
63. Urrutia R, Miren Gutiérrez-Muto A, Sanz-Morère CB, Gómez A, Politi AM, Lunardini F, et al. Spasticity evaluation with the amadeo tyromotion device in patients with hemispheric stroke. Front Neurorobot. (2023) 17:1172770. doi: 10.3389/fnbot.2023.1172770
64. Dalvi-Esfahani M, Mosharaf-Dehkordi M, Leong LW, Ramayah T, Jamal Kanaan-Jebna AM. Exploring the drivers of XAI-enhanced clinical decision support systems adoption: insights from a stimulus-organism-response perspective. Technol Forecast Soc Change. (2023) 195:122768. doi: 10.1016/j.techfore.2023.122768
Keywords: lower limb rehabilitation, machine learning, rehabilitation robotics, personalized therapy, algorithm challenges
Citation: Zhang X, Rong X and Luo H (2024) Optimizing lower limb rehabilitation: the intersection of machine learning and rehabilitative robotics. Front. Rehabil. Sci. 5:1246773. doi: 10.3389/fresc.2024.1246773
Received: 24 June 2023; Accepted: 15 January 2024;
Published: 26 January 2024.
Edited by:
Ahmad Athif Mohd Faudzi, Universiti Teknologi Malaysia, MalaysiaReviewed by:
Anwar P. P. Abdul Majeed, Xi’an Jiaotong-Liverpool University, China© 2024 Zhang, Rong and Luo. This is an open-access article distributed under the terms of the Creative Commons Attribution License (CC BY). The use, distribution or reproduction in other forums is permitted, provided the original author(s) and the copyright owner(s) are credited and that the original publication in this journal is cited, in accordance with accepted academic practice. No use, distribution or reproduction is permitted which does not comply with these terms.
*Correspondence: Hanwen Luo bHVvaGFud2VuNjZAMTYzLmNvbQ==
†These authors have contributed equally to this work
Disclaimer: All claims expressed in this article are solely those of the authors and do not necessarily represent those of their affiliated organizations, or those of the publisher, the editors and the reviewers. Any product that may be evaluated in this article or claim that may be made by its manufacturer is not guaranteed or endorsed by the publisher.
Research integrity at Frontiers
Learn more about the work of our research integrity team to safeguard the quality of each article we publish.