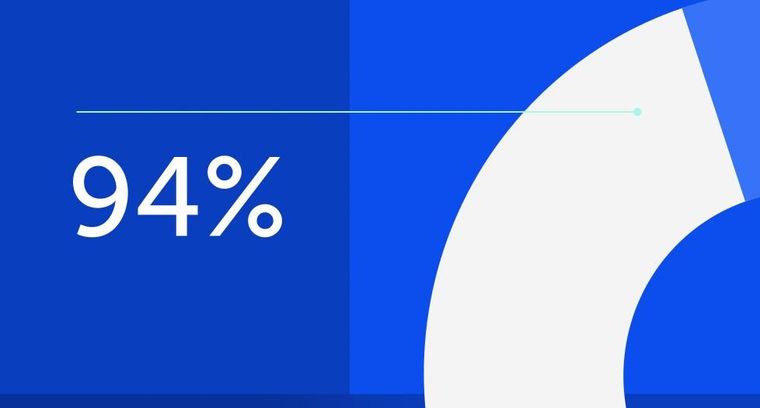
94% of researchers rate our articles as excellent or good
Learn more about the work of our research integrity team to safeguard the quality of each article we publish.
Find out more
ORIGINAL RESEARCH article
Front. Radiol.
Sec. Artificial Intelligence in Radiology
Volume 5 - 2025 | doi: 10.3389/fradi.2025.1457173
The final, formatted version of the article will be published soon.
Select one of your emails
You have multiple emails registered with Frontiers:
Notify me on publication
Please enter your email address:
If you already have an account, please login
You don't have a Frontiers account ? You can register here
Introduction: COVID-19 is an infectious respiratory disease that is caused by the SARS-CoV-2 virus. It is mainly transmitted by respiratory droplets that may produce mild symptoms to severe sickness or death. COVID-19 attacks both the trachea and lungs. High-resolution computed tomography (HRCT) scans are preferred for diagnosing several respiratory disorders at an early stage and more accurately.Accurate detection of COVID-19 using CT images is still challenging due to several factors, such as low-quality images, illumination, and variation in the shape and size of the COVID-19 lesions. Therefore, the main objective is to design a deep learning model for accurate detection at an early stage.Method: A method is proposed for classifying and segmenting COVID-19 lesions. First, preprocessing is performed on axial, coronial, and sagittal views of CT slices using statistical methods based on volumetric analysis to compute the scores. These are used to assign the grades based on the severity level of each lung lobe such as mild, moderate, and healthy. The graded slices are input to the proposed classification model, and N × 1000 features are extracted at the fully connected (FC-1000) layer of the pre-trained ResNet-18 model. Out of these N × 469 features are selected through the Harris Hawks Optimization (HHO) model. Finally, classification is performed using variational quantum, neural network, and Naïve Bayes classifiers. To segment the classified images, fine-tuned U-net model is designed having 47 layers such as 1 input, 5 dropout, 11 conv, 9 ReLU, 9 Batch-normalization, 4 Conv2DTranspo, 4 Concatenate, and 4 max-pooling. The model is trained from scratch based on optimal hyperparameters such as binary cross-entropy loss, 16 filter size, 0.005 drop-out, 8 batch size, and Adam optimizer.The proposed method is evaluated on local and publicly available UCSD-AI4H/COVID-CT datasets and provides a mean-ROC of 0.98 ± 0.01 that strengthen the novelty of the proposed method.
Keywords: UNET, DICOM, ResNet-18, FC-1000, scores
Received: 30 Jun 2024; Accepted: 14 Mar 2025.
Copyright: © 2025 Amin, gul, Sharif and Fernandes. This is an open-access article distributed under the terms of the Creative Commons Attribution License (CC BY). The use, distribution or reproduction in other forums is permitted, provided the original author(s) or licensor are credited and that the original publication in this journal is cited, in accordance with accepted academic practice. No use, distribution or reproduction is permitted which does not comply with these terms.
* Correspondence: Steven Fernandes, Department of Journalism, Media & Computing, College of Arts and Sciences, Creighton University, Omaha, United States
Disclaimer: All claims expressed in this article are solely those of the authors and do not necessarily represent those of their affiliated organizations, or those of the publisher, the editors and the reviewers. Any product that may be evaluated in this article or claim that may be made by its manufacturer is not guaranteed or endorsed by the publisher.
Supplementary Material
Research integrity at Frontiers
Learn more about the work of our research integrity team to safeguard the quality of each article we publish.