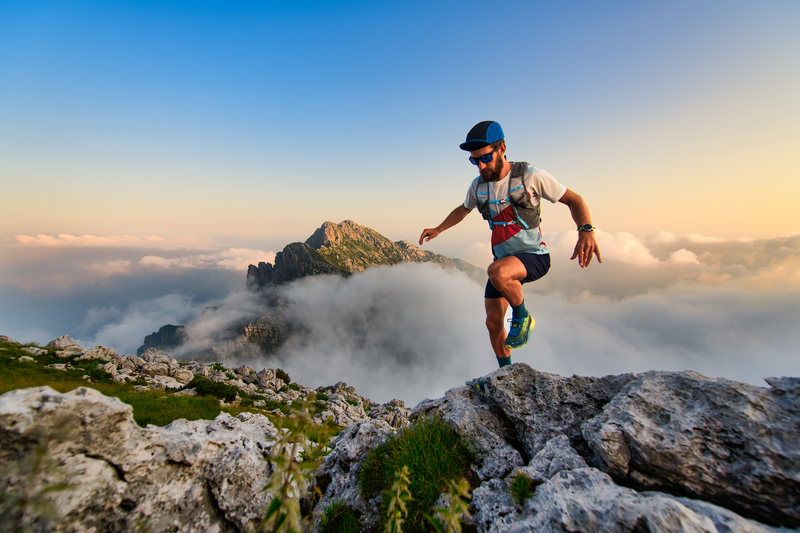
95% of researchers rate our articles as excellent or good
Learn more about the work of our research integrity team to safeguard the quality of each article we publish.
Find out more
ORIGINAL RESEARCH article
Front. Public Health
Sec. Health Economics
Volume 13 - 2025 | doi: 10.3389/fpubh.2025.1588270
The final, formatted version of the article will be published soon.
You have multiple emails registered with Frontiers:
Please enter your email address:
If you already have an account, please login
You don't have a Frontiers account ? You can register here
The rapid advancement of Artificial Intelligence (AI)-driven recommendation systems in healthcare presents significant economic implications, particularly in the context of neurological disorders. These systems offer opportunities to enhance diagnostic accuracy, optimize resource allocation, and improve patient outcomes. However, conventional economic models fail to address the dynamic complexities of AI integration in healthcare, including market inefficiencies and stakeholder behaviors. Methods: To bridge this gap, we propose a Dynamic Equilibrium Model for Health Economics (DEHE), incorporating reinforcement learning and stochastic optimization. This model captures uncertainty in healthcare decision-making and includes dynamic pricing, behavioral incentives, and adaptive insurance premium mechanisms. Findings: Our experimental results demonstrate that DEHE improves economic efficiency by optimizing AI-driven recommendations while balancing healthcare cost and accessibility. Through multi-agent simulations, the model shows strong real-world applicability and stability. It effectively addresses asymmetric information, moral hazard, and market dynamics. Conclusion: This study offers a novel economic framework for integrating AI-driven systems in neurological healthcare.We recommend the adoption of adaptive policy mechanisms and stakeholder-specific incentives to enhance cost-effectiveness and equitable access. These insights contribute to the development of more sustainable and inclusive AI-based healthcare policies.
Keywords: AI-Driven Recommendation Systems, healthcare economics, neurological disorders, Dynamic equilibrium model, machine learning, Cost-Effectiveness, policy optimization
Received: 07 Mar 2025; Accepted: 03 Apr 2025.
Copyright: © 2025 Li. This is an open-access article distributed under the terms of the Creative Commons Attribution License (CC BY). The use, distribution or reproduction in other forums is permitted, provided the original author(s) or licensor are credited and that the original publication in this journal is cited, in accordance with accepted academic practice. No use, distribution or reproduction is permitted which does not comply with these terms.
* Correspondence:
Li Li, Taizhou Vocational College of Science and Technology, Taizhou, China
Disclaimer: All claims expressed in this article are solely those of the authors and do not necessarily represent those of their affiliated organizations, or those of the publisher, the editors and the reviewers. Any product that may be evaluated in this article or claim that may be made by its manufacturer is not guaranteed or endorsed by the publisher.
Research integrity at Frontiers
Learn more about the work of our research integrity team to safeguard the quality of each article we publish.