- 1Department of Health Management, School of Medicine and Health Management, Tongji Medical College, Huazhong University of Science and Technology, Wuhan, China
- 2Key Research Institute of Humanities and Social Sciences of Hubei Provincial, Department of Education, Research Centre for Rural Health Service, Wuhan, China
Background: An increasing number of middle-aged and older adults are migrating from rural to urban areas for employment, to care for their younger generation and due to old age. As these age groups move into urban areas, their healthcare service utilization are directly related to their health status and basic rights to survival. It also places higher demands on China’s healthcare service provision.
Objectives: This study aims to examine effects of rural-to-urban migration on healthcare utilization among middle-aged and older adults in China.
Methods: Panel data from Waves 3, 4, and 5 of the China Health and Retirement Longitudinal Study were used. We included 318 participants in the exposure group and 7,525 participants in the control group. The study employed propensity score matching (PSM) and Difference in Difference (DID) analysis.
Results: Difference in difference regression results showed that middle-aged and older adults reduced number of hospitalizations when they moved to a city with DID values of −0.092 (p < 0.10) for the period 2015–2018 and − 0.135 (p < 0.05) for the period 2015–2020. No significant effects were observed regarding the number of outpatient visits (p > 0.05).
Conclusion: Middle-aged and older migrants who migrated to cities reduced inpatient healthcare utilization, possibly due to lower hospitalization reimbursement rates, financial burden, and lower social integration. Policies enhancing health insurance reimbursement rates for migrants, integrated community support programs, and strengthening health education to promote health equity may provide remedy.
Introduction
In recent years, an increasing number of middle-aged and older adults have been migrating from rural to urban areas or from economically underdeveloped to economically developed areas (1). This trend is driven by various factors, including global industrialization and urbanization, better employment opportunities, expectations of higher income, and improved social services (2). Since 2015, the Chinese migrant population has stabilized at around 245 million, while the number of older migrants continues to rise (3). Compared to younger migrants, middle-aged and older adults are more likely to have diagnosed chronic diseases and higher healthcare utilization (4). Despite the potential for improved healthcare access in urban areas, many middle-aged and older migrants continue to face significant barriers. These include geographic distance from health insurance schemes, higher out-of-pocket medical expenses, and limited access to specialized medical services (5). These challenges highlight the urgent need to understand the healthcare utilization patterns of middle-aged and older migrants, particularly in the context of their migration from rural to urban areas.
To better understand these challenges, previous studies have explored various aspects of migrants’ health and healthcare access. Most existing research has focused on health status (6), health awareness (7), inequalities (8), and respective determinants in migrant populations. Corresponding evidence suggests that migrants’ health is shaped by individual characteristics such as age, gender, education, and marital status (9). Additionally, the timing and extent of migration significantly impact migrants’ health outcomes (10). A key structural barrier is China’s household registration system (hukou), which ties health insurance benefits to an individual’s registered residence, resulting in higher out-of-pocket costs and complex reimbursement procedures for rural-to-urban migrants (2). Research from Germany indicated, for instance, that immigrants utilize healthcare services less frequently and face greater inequalities than non-immigrants (11); and a study from Switzerland found that regularizing the status of undocumented migrants increased their utilization of health services (12). However, most previous studies have utilized cross-sectional data or compared migrant populations with residents (8, 13), leaving a significant gap in understanding how healthcare utilization changes before and after urban relocation among middle-aged and older migrants.
This study aims to close this gap by evaluating the effects of rural-to-urban migration on healthcare utilization among middle-aged and older adults using panel data from the China Health and Retirement Longitudinal Study (CHARLS) for the years 2015, 2018, and 2020. Specifically, we hypothesize that middle-aged and older migrants who move from rural to urban areas will experience a decrease in the number of outpatient visits (NOO) and hospitalizations (NOH). The findings will inform strategies to improve the health of middle-aged and older migrants and guide the development of health insurance policies, thereby promoting healthy aging and advancing health equity.
Methods
Data sources
CHARLS aims to collect high-quality microdata that are representative of Chinese households and adults aged 45 years and older. Being a nationally representative survey of the older population, it covers 150 district units, 450 village units, and approximately 17,000 adults in 10,000 households at baseline (14). Participants are followed up every 2–3 years. CHARLS collects data on consumption, work, income and assets, health status, health insurance, and information on type of residence and health services utilization. Overall, the CHARLS survey provides a comprehensive depiction of the older population in China. To enhance the representativeness of our findings, we applied longitudinal sampling weights from the CHARLS database, which account for non-response at both household and individual levels. Additionally, we used multiple imputation with chained equations and performed 5 imputations to address item-missing data.
Sample selection
This longitudinal study used data from the third (2015), fourth (2018), and fifth (2020) waves of CHARLS. The data were matched by respondent ID to include participants surveyed in all three waves. Next, we excluded participants who lived in towns, moved from urban to rural areas, or lived in combined townships in 2015, were younger than 45 years old, or had missing data regarding date of birth. Additionally, participants with non-agricultural hukou (household registration), those who did not live in the same rural area all the time, those who returned to rural areas after migrating to cities, and those who migrated to cities after 2015, that is during 2018–2020, were also excluded. This process resulted in 23,529 observations for 7,843 adults, divided into the rural-to-urban group (exposure group, 954 observations for 318 adults) and the group that remained in rural areas (control group, 22,575 observations for 7,525 adults). Figure 1 illustrates the data selection process.
Variables
Outcomes of this study were NOO in the previous month and NOH in the last year. NOO represents the total number of outpatient visits to various medical institutions over the last month, while NOH represents the total number of inpatient visits over the past year.
Region was set as an independent variable based on two questions from CHARLS’ basic information section: ‘What is your current address?’, and ‘Is it a village or a city/town?’. Participants who lived in towns in 2015 were excluded, ensuring that all participants lived in villages at the present study’s baseline.
For control variables, the Anderson model of healthcare utilization was consulted. This model includes predisposing, enabling, and need factors and is a well-established framework for analyzing healthcare service use with a behavioral measurement model (15, 16). This study began by selecting covariates that may influence rural-to-urban migration and healthcare utilization, guided by the Anderson service model and relevant literature (17, 18). Predisposing factors included age, gender (male = 1, female = 0), education (illiterate = 1, primary = 2, junior high school = 3, senior high school = 4, bachelor’s degree and above = 5), and marital status (divorced, widowed, and never married = 0, married or cohabitation = 1). Enabling factors consisted of economic status (measured by per capita household expenditure, which is logarithmic to reduce the effect of outliers and make the results more stable) and health insurance (no = 1, employee health insurance = 2, urban and rural resident health insurance = 3, others = 4), social activities (participating in social activities = 1, none = 0), household size (number of people living in the household). Need factors included self-assessed health (very good and good = 1, fair, poor, and very poor = 0), chronic disease prevalence (with chronic disease = 1, without = 0), smoking (current or previous smoker = 1, never smoked = 0), and alcohol consumption (consumes alcohol = 1, abstinent = 0), activities of daily living (ADL) included dressing, bathing, eating, getting into or out of bed, using the bathroom, controlling urination and defecation (having no difficulty = 0, having difficulty but still able to perform independently =1, having difficulty and needing help = 2, unable to perform activity = 3, with a total score ranging from 0 to 18), and instrumental activities of daily living (IADL) included doing household chores, cooking, shopping, making phone calls, taking medication, managing money (scales as for ADL, with a total score also ranging from 0 to 18). Table 1 presents variables categorized and described.
Statistical analysis
We used the PSM-DID method to assess the effect of migration to cities on the NOO and NOH among middle-aged and older adults in the sample. The PSM method effectively addresses issues of endogeneity and self-selection within samples (19–21) and is widely used to evaluate the impacts of health and other policies (22, 23). The PSM-DID can address selection bias by matching treated and control groups on observed covariates, thereby ensuring baseline comparability. Moreover, it relaxes the assumption of parallel trends, which extends the traditional DID framework and makes it more suitable for real-world applications. Additionally, PSM-DID is particularly robust in non-experimental settings, as it provides transparent treatment effect estimates by attributing outcome differences to the treatment rather than pre-existing confounders.
First, propensity scores were calculated. The propensity score is a balancing score: conditional on the propensity score, the distribution of measured baseline covariates is similar between treated and untreated adults. Common support refers to the overlapping region in the propensity score distribution between the exposure and control groups. A balancing test was conducted to detect an equilibrium of covariates for the exposure and control group and to confirm the reduction of sampling bias through matching. Nearest neighbor matching with caliper was employed for our primary analysis, each treated adult was matched with three untreated adults, as frequently mentioned in the literature (24, 25). After matching, the deviation should be less than or equal to 5 percent, or p > 0.10, to confirm proper matching. The adequacy of the matching was evaluated based on the mean reduction in bias for each covariate and the overall mean reduction in bias. Samples outside the common support were excluded.
Secondly, DID model was constructed after PSM matching while controlling for individual and time effects. As expressed in Equation 1, the model is specified as follows:
Where represents an individual i’s healthcare utilization in year t. is a dummy variable for grouping. It is defined as exposure group = 1 and control group = 0. In the current study, 1 denotes migrating to a city (exposure group), and 0 denotes always living in a rural area (control group). is a time dummy variable that defines 2015 = 0, 2018 = 1, and 2020 = 1. is the coefficient of the core explanatory variable in this study, which is the net effect of middle-aged and older adults migrating to the city on the utilization of healthcare services. It reflects the change in the difference between the exposure group (those who migrated to urban areas) and the control group (those who remained in rural areas). represent the effect of time-varying covariates on the outcome . is the error term, and is a constant term. is an individual fixed effect, and is a time-fixed effect. Statistical analyses were conducted using Stata 16.0. To validate the robustness of the DID results after PSM, we conducted a comparative analysis using the radius matching method and performed placebo tests. Furthermore, we conducted heterogeneity analyses to examine the differential impacts of rural-to-urban migration on healthcare utilization across different demographic groups. Chronic diseases, self-assessed health, and marital status were selected for heterogeneity analyses because chronic diseases influence healthcare needs, self-assessed health is a key predictor of healthcare utilization, and marital status affects social support and access to resources. These variables were chosen to provide a comprehensive understanding of the differences in healthcare utilization among rural-to-urban migrants, while also considering data availability and study scope constraints.
Results
Table 1 presents descriptive statistics for main study variables at the 2015 baseline, prior to rural to urban migration of the exposure group. Exposure and control group exhibited differences in age, education, social activities, per capita household expenditure, health insurance, IADL, and household size. Specifically, the mean age of the exposure group was approximately 2.8 years younger than that of the control group. The exposure group further had a higher average education level, engaged in more social activities, had higher per capita household expenditure, and reported better ability to perform instrumental activities of daily living. Moreover, more people in the exposure group were enrolled in employee health insurance and other insurance schemes than in the control group. In contrast, the exposure group’s average household size was smaller than that of the control group. Although there were no significant differences in self-assessed health between exposure and control groups initially, further analysis (see Table 2) revealed that by 2020, middle-aged adults in the exposure group reported poorer self-assessed health compared to the control group. In addition, the exposure group showed reduced participation in social activities after a period of migration to urban areas compared to the control group. In 2018, the exposure group exhibited slightly better ADL than the control group, while by 2020, there were no significant differences between the two groups. Similarly, the exposure group performed better in IADL in 2018; by 2020, the gap between the two groups gradually narrowed, with the exposure group showing a slight advantage in IADL.
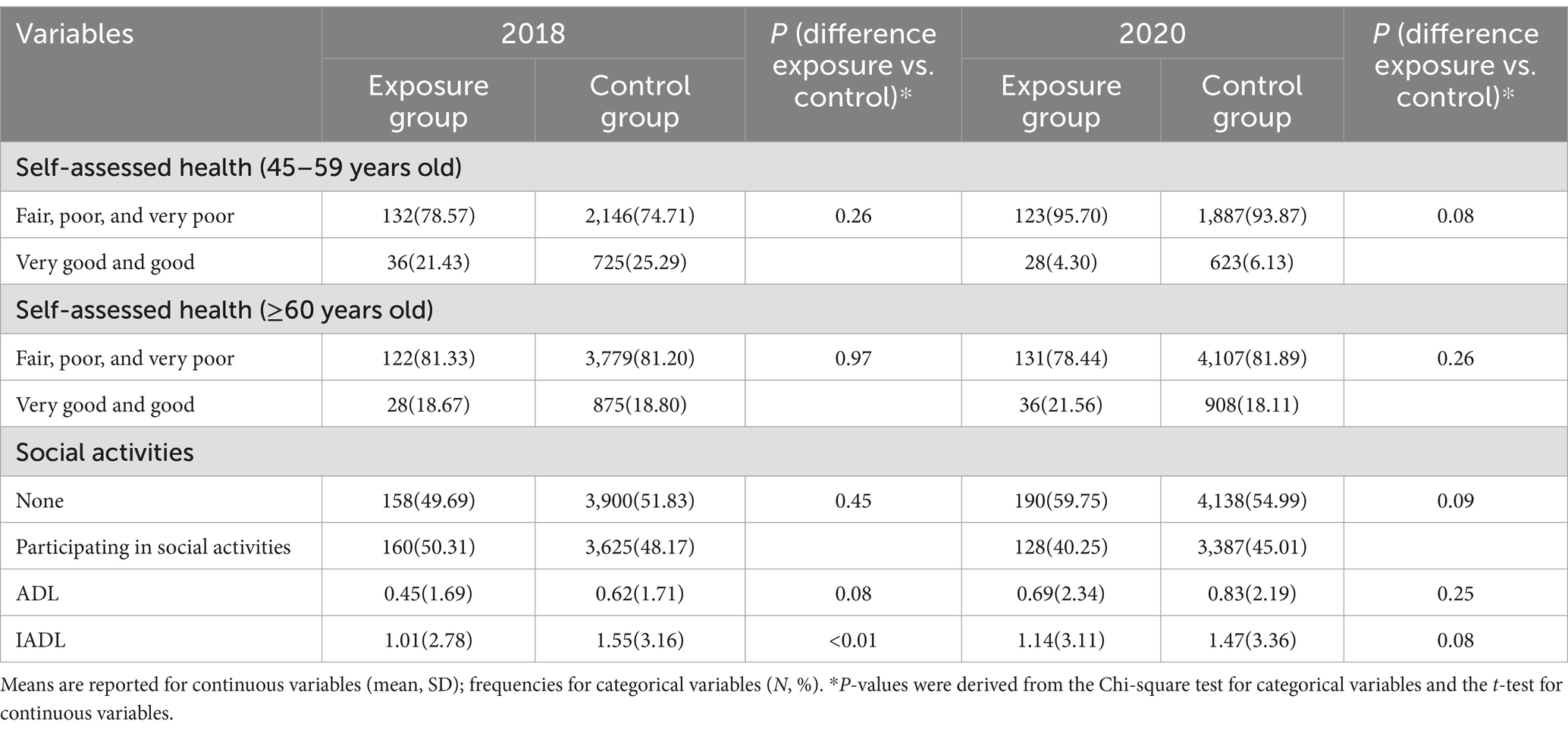
Table 2. Comparison of self-assessed health, social activities, ADL, and IADL between the exposure and control group (China, 2018 and 2020).
PSM results
Table 3 displays the results of the balance test using 1:3 nearest neighbor matching with a caliper. We performed caliper nearest-neighbor matching on the sample from the baseline of 2015 (i.e., before migration). As shown in Table 3, the standard error for each control variable was less than 10% after matching, and no significant differences were found at the 5% level between the means of the variables for the two samples. Additionally, the likelihood ratio chi-square test results using PSM were significantly smaller than those of the original outcomes, further indicating that PSM enhanced overall balance after matching. The matched samples were then aligned with 2018 and 2020 data using respondents’ IDs. Consequently, the final sample included 954 observations in the exposure group and 2,661 observations in the control group.
Parallel trend test
A fundamental assumption of the DID approach is that the exposure and control groups follow parallel trends in the outcome variable prior to the intervention. This requires at least two pre-treatment periods for a formal parallel trend test. However, as this study only includes one pre-treatment period, we follow the approach used in previous research (26), which considers the absence of a significant difference in the outcome variable between the exposure and control groups, after controlling for other factors, as indicative of parallel trends. As shown in Table 4, after adjusting for potential confounders, there is no statistically significant difference in outpatient visits and hospitalizations between the exposure and control groups before migration, suggesting that the parallel trend assumption holds. Therefore, the DID model is appropriate for this analysis.
DID results
First, the DID method was applied to analyze NOO and NOH using the 2015 and 2018 data. The results revealed no significant negative effect on NOO and NOH for middle-aged and older adults migrating to cities (see Table 5). Second, NOO and NOH were analyzed using DID for the 2015 and 2020 data. The DID coefficient for NOH was −0.135, with a p-value<0.05, indicating that compared to the control group, middle-aged and older adults in the exposure group had 0.135 fewer hospitalizations after migrating to an urban area. In contrast, the DID coefficient for NOO was −0.032 with a p-value > 0.05 for 2015–2020. These findings suggest that migration to cities did not significantly affect NOO for middle-aged and older adults.
Sensitivity analyses
Replacement of the matching method
To ensure the robustness of the PSM-DID results, an alternative matching method, specifically radius matching, was employed. The DID regressions were re-estimated using this different matching method, as presented in Table 6. The results remained consistent with the initial findings, suggesting that the empirical analyses demonstrate strong reliability.
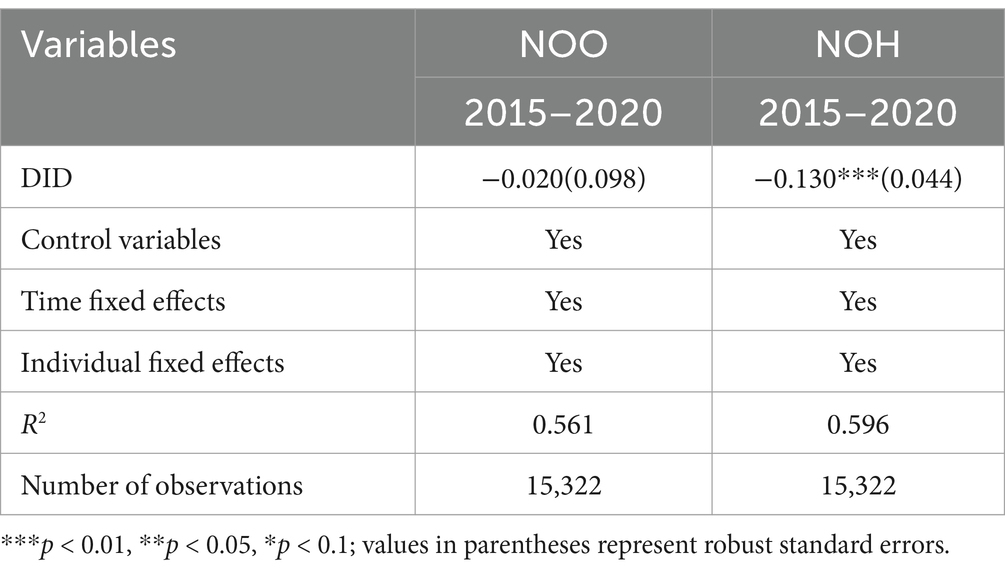
Table 6. Difference-in-differences estimation results after changing the matching method (China, 2015 -2020).
Placebo testing
To control for the potential bias arising from omitted variables in the above results, referring to a previous study (27), a placebo test was performed. The placebo test is a regression in which any of the dummy exposure groups in the sample are randomly selected and the process is repeated 500 times. The results of the placebo test for 2015–2020 are shown in Figure 2. Figure 2a displays the estimated coefficients for the number of hospitalizations, which are centered around zero and follow a normal distribution, indicating a robust model. The dotted lines represent the true estimated coefficients, which are far from these virtual estimates, indicating that the result is not sensitive to unobserved factors or the effects of random interventions. Figure 2b displays the estimated coefficients for the number of outpatient visits. The dotted line remains insignificant within the normal value range, demonstrating the robustness of the study’s results.
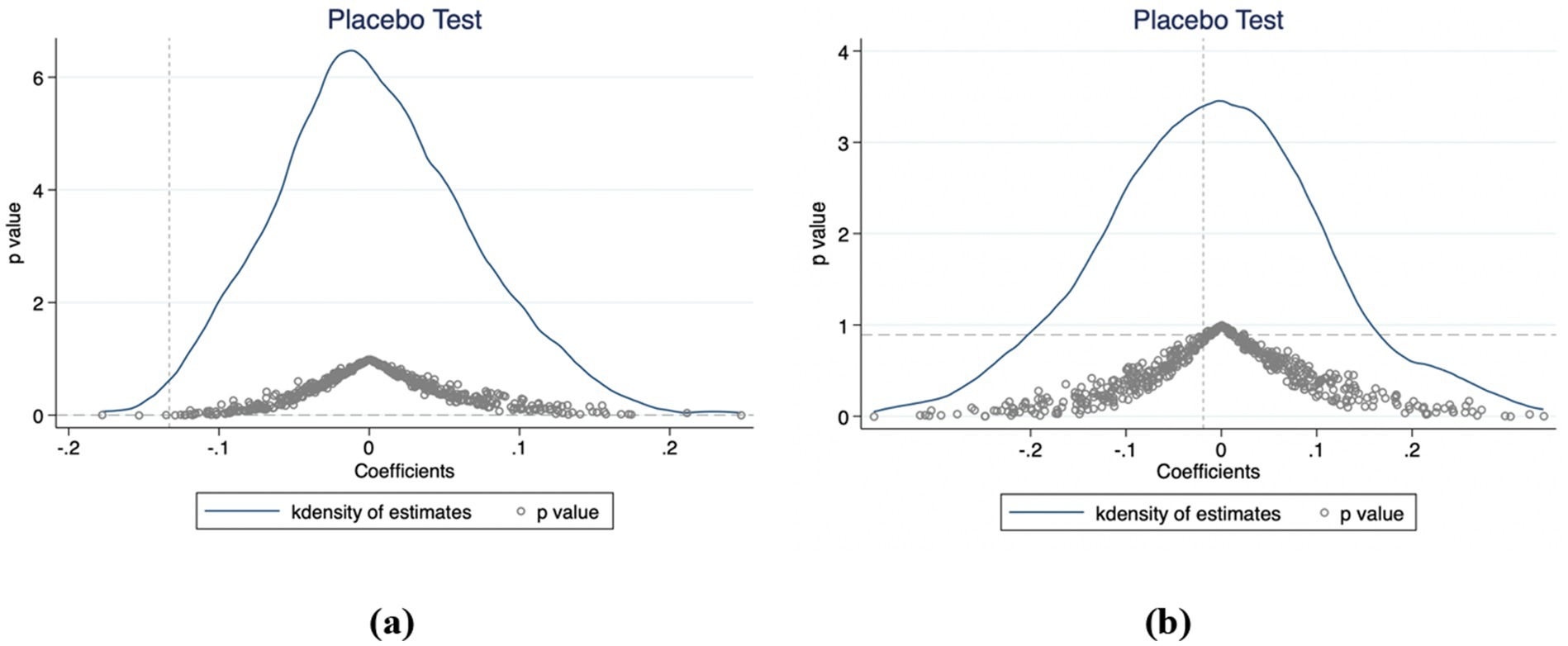
Figure 2. Placebo test results for the coefficient on the number of hospitalizations (a) and outpatient visits (b) (China, 2015–2020).
Winsorization
To enhance the robustness of the results, we used the Winsorization process. The sample values for the lowest and highest 1% of each of the number of hospitalizations and outpatient visits were replaced with 1st percentile and 99th percentile values. Through this process, we aim to minimize the impact of outliers, resulting in more robust results. This method helps to ensure that estimates of NOO and NOH more accurately reflect respondents’ healthcare attendance and avoid bias in the results due to extreme observations. As shown in Table 7, the results are consistent with the previous findings.
Excluding the impact of the COVID-19 pandemic
The global spread of the COVID-19 pandemic in 2020 impacted the utilization of medical services. To account for this, we excluded responses to the survey question, “During the pandemic, were there any medical services or treatments you needed, such as hospitalization for major surgeries or outpatient care, that were delayed or canceled?” Specifically, we removed samples indicating the need for “major surgeries requiring hospitalization” and “outpatient services” affected by delays or cancelations. After excluding these cases, the results remained consistent with the above findings (Table 8).
Heterogeneity analyses
Heterogeneity analyses indicated that healthcare utilization varied among different subgroups of middle-aged and older migrants. These analyses revealed how rural-to-urban migration affected healthcare service utilization across various self-assessed health conditions, age, and chronic disease groups, providing valuable insights for policymakers to formulate more targeted recommendations. Table 9 showed a significant reduction in NOH for migrants with chronic diseases who moved to cities. Additionally, the same table highlighted a significant reduction in NOH for migrants who self-assessed their health as fair, poor, or very poor following their migration to urban areas. Furthermore, Table 9 indicated that 60–74-year-olds who moved to cities had fewer hospitalizations.
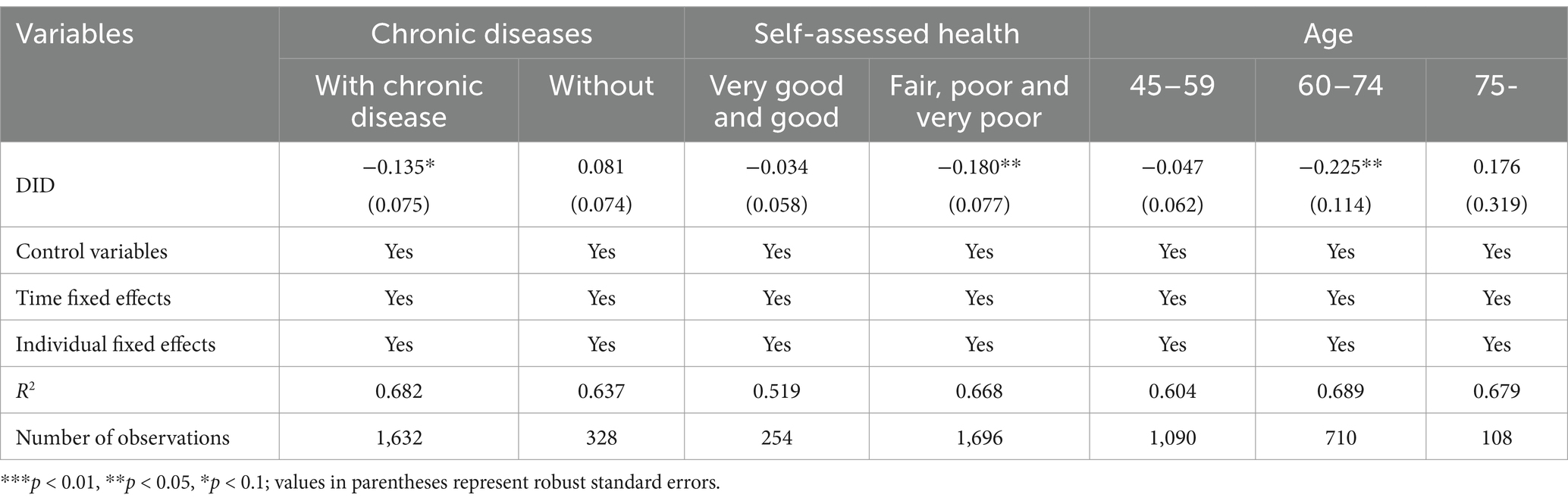
Table 9. Results of the test for heterogeneity in the number of hospitalizations (China, 2015–2020).
Discussion
At present, few studies have used longitudinal data to examine healthcare utilization among middle-aged and older rural-to-urban migrants in China. While previous research has primarily relied on cross-sectional data or compared migrants with residents (8, 13), this study provides novel insights by tracking changes in healthcare utilization before and after migration. Consistent with previous studies (28, 29), our research demonstrated that rural middle-aged and older migrants who moved to urban areas experienced a reduction in hospitalization services utilization. However, our study found that this effect becomes more pronounced with longer durations of urban residence and further extended this understanding by revealing nuanced heterogeneity effects based on individual characteristics. Specifically, we found that migration to urban areas has a more pronounced impact on healthcare utilization among adults with chronic diseases, those with fair or poor self-assessed health, and those aged 60–74. However, contrary to the findings of the previous study (30), the change in residence did not significantly affect the number of outpatient visits, which remains stable. These findings highlight the importance of considering demographic and health status variations when designing policies for migrant populations, a point that has been largely overlooked in prior research.
First, the reduction of hospitalizations may be attributed to healthcare policies related to reimbursement for medical treatment outside one’s registered area (8, 29, 31). Despite ongoing improvements in China’s reimbursement policies, the rates for medical treatment outside one’s enrolment area remain lower than those for local treatment (32). For example, in Qingyuan City, Guangdong Province, the reimbursement rates for hospitalization are 90% for level 1 hospitals, 75% for level 2, and 65% for level 3 if the treatment is received within the enrolment area. In contrast, when treatment is sought outside the enrolment area, the reimbursement rates drop to 70% for level 1, 60% for level 2, and 50% for level 3 (33). Consequently, the reimbursement rates for equivalent medical services are reduced by 15–20 percentage points when treatment is provided elsewhere. Furthermore, before the implementation of direct remote settlement of medical expenses, China’s reimbursement process was complex, requiring upfront payment and subsequent reimbursement at the enrolment location. Similarly, a study conducted in Kenya found that despite national treaties advocating for equal healthcare rights, immigrants often face higher healthcare costs than local residents (34). In the Greater Mekong Subregion, migrants encounter elevated health risks but are inadequately covered, leading to high out-of-pocket payments for health services (35). When designing a high-quality universal health coverage system, it is essential to enable middle-aged and older migrants to enroll in insurance at their place of migration, expand the level and scope of reimbursement for medical treatment in other locations, and reduce the disparity between treatments received within and outside the insured area.
Second, according to Anderson’s model, enabling factors such as financial resources and social capital influence healthcare utilization. Relevant studies indicate that most rural-to-urban migrants forego hospitalization because of poor affordability (36, 37). This is particularly true for the middle-older age group (60–74 years), who may be between retirement and intergenerational care. Compared to their rural counterparts, this group is more susceptible to chronic diseases (38), they tend to prioritize financial stability over personal health to avoid burdening their children, often choosing to overlook their illnesses (13, 39). Although their presence in urban areas increases demand for healthcare services, these adults often underutilize healthcare services due to financial difficulties and restrictive insurance policies (40). Furthermore, lower levels of social integration among middle-aged and older migrants may lead to negative attitudes toward healthcare and inadequate access to preventive and primary public health services (41, 42). To alleviate the financial burden associated with hospitalization, policymakers should consider modest improvements in healthcare treatment for vulnerable migrants. Addressing these challenges also requires fostering a supportive environment at both community and household levels to facilitate the integration of middle-aged and older migrants into urban communities (43, 44).
Third, the effect of moving to an urban area on hospitalization among middle-aged and older adults may take time to become significant. This delay could be attributed to the fact that some adults in these groups have recently migrated and may still be adapting to the new living environment and healthcare system. As a result, they may continue to rely on their established habits for seeking medical care. Steventon similarly observed that hospital admission rates among migrants remained low for several years following their arrival in the UK (45). However, contrary to the findings of the previous study (30), migration to cities did not significantly affect the NOO among these age groups. This may be due to the low reimbursement rates and limited caps on outpatient service reimbursements under many Chinese insurance schemes, which lead to high out-of-pocket costs for middle-aged and older adults. Policymakers should consider enhancing the medical insurance system for treatments received in other locations, integrating community support programs, and promoting health literacy to improve health outcomes for migrant populations (46, 47).
Several limitations should be acknowledged. Firstly, the implementation of PSM-DID relies on the assumption that all relevant covariates are included in the model. Any unobserved covariates can introduce bias by causing different trends between the treatment and control groups. Future research should consider using alternative methods to mitigate the risk of bias. Additionally, the limitations of the CHARLS database restrict our ability to perform in-depth analyses of migration destinations. Adults are nested within provinces, and the development of healthcare services varies significantly across different regions of China, complicating the analysis of healthcare utilization patterns. Future research should employ alternative methods and utilize more detailed datasets that incorporate a broader range of health assessments and regional characteristics to enhance our understanding of healthcare utilization among rural-to-urban migrants.
Conclusion
This study demonstrates that migration from rural to urban areas significantly reduces hospital admissions among middle-aged and older migrants. However, utilization of outpatient services remains unchanged. These findings highlight the need for policymakers to address the specific healthcare challenges faced by this demographic group. In China, where rapid urbanization and economic transformation have led to substantial internal migration, policymakers should enhance the medical insurance system for treatment outside the registered area, develop community support programs, and strengthen health education to improve the well-being of middle-aged and older migrants while ensuring equitable access to care.
Data availability statement
The original contributions presented in the study are publicly available. This data can be found at: https://charls.pku.edu.cn/en/.
Ethics statement
The data used in this study were retrieved from the CHARLS. This survey was endorsed by the Biomedical Ethics Committee of Peking University (No. IRB00001052–11015). All participants in the study signed or marked (if illiterate) the informed consent forms. All methods were carried out according to relevant guidelines and regulations.
Author contributions
LX: Writing – original draft, Writing – review & editing. YC: Funding acquisition, Writing – review & editing.
Funding
The author(s) declare that financial support was received for the research and/or publication of this article. This study was supported by grants from the National Natural Science Foundation of China (72374076).
Acknowledgments
We thank Peking University, the CHARLS research and field team and every respondent in the study for their contributions.
Conflict of interest
The authors declare that the research was conducted in the absence of any commercial or financial relationships that could be construed as a potential conflict of interest.
Generative AI statement
The authors declare that no Gen AI was used in the creation of this manuscript.
Publisher’s note
All claims expressed in this article are solely those of the authors and do not necessarily represent those of their affiliated organizations, or those of the publisher, the editors and the reviewers. Any product that may be evaluated in this article, or claim that may be made by its manufacturer, is not guaranteed or endorsed by the publisher.
Abbreviations
PSM-DID, Difference-in-Differences Propensity Score Matching estimator; CHARLS, China health and retirement longitudinal study; PSM, Propensity Score Matching; DID, Difference-in-Differences estimator.
References
1. Xing, Y, Zhang, L, Zhang, Y, and He, R. Relationship between social interaction and health of the floating elderly population in China: an analysis based on interaction type, mode and frequency. BMC Geriatr. (2023) 23:662. doi: 10.1186/s12877-023-04386-z
2. Gong, P, Liang, S, Carlton, EJ, Jiang, Q, Wu, J, Wang, L, et al. Urbanisation and health in China. Lancet. (2012) 379:843–52. doi: 10.1016/S0140-6736(11)61878-3
3. Gao, L, Penning, MJ, Wu, Z, Sterrett, SJ, and Li, S. Internal migration and the health of middle-aged and older persons in China: the healthy migrant effect reconsidered. Res Aging. (2021) 43:345–57. doi: 10.1177/0164027520958760
4. Zhong, BL, Liu, TB, Chan, SSM, Jin, D, Hu, CY, Dai, J, et al. Common mental health problems in rural-to-urban migrant workers in Shenzhen, China: prevalence and risk factors. Epidemiol Psychiatr Sci. (2018) 27:256–65. doi: 10.1017/S2045796016001141
5. Xie, Y, Guo, Q, and Meng, Y. The health service use of aged rural-to-urban migrant workers in different types of cities in China. BMC Health Serv Res. (2021) 21:606. doi: 10.1186/s12913-021-06638-3
6. Fu, Y, Lin, W, Yang, Y, Du, R, and Gao, D. Analysis of diverse factors influencing the health status as well as medical and health service utilization in the floating elderly of China. BMC Health Serv Res. (2021) 21:438. doi: 10.1186/s12913-021-06410-7
7. Hai, Y, Wu, WL, Yu, LW, and Wu, L. Health literacy and health outcomes in China's floating population: mediating effects of health service. BMC Public Health. (2021) 21:691. doi: 10.1186/s12889-021-10662-7
8. Xi, S, Song, Y, Li, X, Li, M, Lu, Z, Yang, Y, et al. Local-migrant gaps in healthcare utilization between older migrants and local residents in China. J Am Geriatr Soc. (2020) 68:1560–7. doi: 10.1111/jgs.16421
9. Wang, Q. Health of the elderly migration population in China: benefit from individual and local socioeconomic status? Int J Environ Res Public Health. (2017) 14:370. doi: 10.3390/ijerph14040370
10. Niu, L, Liu, Y, and Wang, X. Using nomogram to predict the hospitalization forgone among internal migrants in China: a nationally representative cross-sectional secondary data analysis. Risk Manag Healthc Policy. (2021) 14:3945–54. doi: 10.2147/RMHP.S301234
11. Klein, J, and von dem Knesebeck, O. Inequalities in health care utilization among migrants and non-migrants in Germany: a systematic review. Int J Equity Health. (2018) 17:160. doi: 10.1186/s12939-018-0876-z
12. Fakhoury, J, Burton-Jeangros, C, Consoli, L, Duvoisin, A, and Jackson, Y. Association between residence status regularization and access to healthcare for undocumented migrants in Switzerland: a panel study. Front Public Health. (2022) 10:832090. doi: 10.3389/fpubh.2022.832090
13. Wang, Y, Jing, Z, Ding, L, Tang, X, Feng, Y, Li, J, et al. Socioeconomic inequity in inpatient service utilization based on need among internal migrants: evidence from 2014 national cross-sectional survey in China. BMC Health Serv Res. (2020) 20:984. doi: 10.1186/s12913-020-05843-w
14. Zhao, Y, Hu, Y, Smith, JP, Strauss, J, and Yang, G. Cohort profile: the China health and retirement longitudinal study (CHARLS). Int J Epidemiol. (2014) 43:61–8. doi: 10.1093/ije/dys203
15. Andersen, RM. Revisiting the behavioral model and access to medical care: does it matter? J Health Soc Behav. (1995) 36:1–10. doi: 10.2307/2137284
16. Peng, B, and Ling, L. Health service behaviors of migrants: a conceptual framework. Front Public Health. (2023) 11:1043135. doi: 10.3389/fpubh.2023.1043135
17. Zhou, M, Zhu, W, Sun, X, and Huang, L. Internet addiction and child physical and mental health: evidence from panel dataset in China. J Affect Disord. (2022) 309:52–62. doi: 10.1016/j.jad.2022.04.115
18. Xie, L, Yao, Y-D, Tang, L-l, Zhang, S, Yang, H-L, Zhang, S-Q, et al. Effect of working after retirement on the mental health of older people: evidence from China. Front Psych. (2021) 12:12. doi: 10.3389/fpsyt.2021.731378
19. Nolan, A. Evaluating the impact of eligibility for free care on the use of general practitioner (GP) services: a difference-in-difference matching approach. Soc Sci Med. (2008) 67:1164–72. doi: 10.1016/j.socscimed.2008.06.021
20. Baser, O. Too much ado about propensity score models? Comparing methods of propensity score matching. Value Health. (2006) 9:377–85. doi: 10.1111/j.1524-4733.2006.00130.x
21. Austin, PC. An introduction to propensity score methods for reducing the effects of confounding in observational studies. Multivariate Behav Res. (2011) 46:399–424. doi: 10.1080/00273171.2011.568786
22. Su, D, Chen, YC, Gao, HX, Li, HM, Chang, JJ, Jiang, D, et al. Effect of integrated urban and rural residents medical insurance on the utilisation of medical services by residents in China: a propensity score matching with difference-in-differences regression approach. BMJ Open. (2019) 9:e026408. doi: 10.1136/bmjopen-2018-026408
23. Yang, S, Guo, D, Bi, S, and Chen, Y. The effect of long-term care insurance on healthcare utilization of middle-aged and older adults: evidence from China health and retirement longitudinal study. Int J Equity Health. (2023) 22:228. doi: 10.1186/s12939-023-02042-x
24. Ma, X, Feng, W, Shi, C, Wang, Y, Gao, Q, Cai, W, et al. Association between the location of social medical insurance and social integration among China’s elderly rural migrants: a nationwide cross-sectional study. BMC Public Health. (2023) 23:2108. doi: 10.1186/s12889-023-16956-2
25. Antu, JF, Parvin, K, Sujan, HM, Mamun, MA, and Naved, RT. Effect of rural-urban migration on age at marriage among adolescent girls in Bangladesh. Front Public Health. (2022) 10:840145. doi: 10.3389/fpubh.2022.840145
26. Li, P, Lu, Y, and Wang, J. Does flattening government improve economic performance? Evidence from China. J Dev Econ. (2016) 123:18–37. doi: 10.1016/j.jdeveco.2016.07.002
27. He, Y, Ouyang, W, Li, Z, and Wei, B. Impact of the industrialization of older adult care services on older Individuals' physical and mental health: evidence from China's quasi-natural experiment. J Multidiscip Healthc. (2023) 16:3017–33. doi: 10.2147/JMDH.S426710
28. Shaaban, AN, Morais, S, and Peleteiro, B. Healthcare services utilization among migrants in Portugal: results from the National Health Survey 2014. J Immigr Minor Health. (2019) 21:219–29. doi: 10.1007/s10903-018-0744-3
29. Zhang, X, Yu, B, He, T, and Wang, P. Status and determinants of health services utilization among elderly migrants in China. Glob Health Res Policy. (2018) 3:8. doi: 10.1186/s41256-018-0064-0
30. Li, D, Yang, J, Liu, H, Ma, Y, and Jiang, J. Comparing income-related inequality on health service utilisation between older rural-to-urban migrant workers and older rural residents in China: a cross-sectional study. BMJ Open. (2023) 13:e060581. doi: 10.1136/bmjopen-2021-060581
31. Han, J, and Meng, Y. Institutional differences and geographical disparity: the impact of medical insurance on the equity of health services utilization by the floating elderly population – evidence from China. Int J Equity Health. (2019) 18:91. doi: 10.1186/s12939-019-0998-y
32. Chen, S, Chen, Y, Feng, Z, Chen, X, Wang, Z, Zhu, J, et al. Barriers of effective health insurance coverage for rural-to-urban migrant workers in China: a systematic review and policy gap analysis. BMC Public Health. (2020) 20:408. doi: 10.1186/s12889-020-8448-8
33. Qingyuan Municipal People's Government. How is medical insurance reimbursement for off-site treatment handled for urban and rural residents? (2024). Available online at: https://www.gdqy.gov.cn/gdqy/newxxgk/rdhy/content/post_1858168.html (Accessed September 10, 2024)
34. Arnold, C, Theede, J, and Gagnon, A. A qualitative exploration of access to urban migrant healthcare in Nairobi, Kenya. Soc Sci Med. (2014) 110:1–9. doi: 10.1016/j.socscimed.2014.03.019
35. McMichael, C, and Healy, J. Health equity and migrants in the greater Mekong subregion. Glob Health Action. (2017) 10:1271594. doi: 10.1080/16549716.2017.1271594
36. Zhang, X, Wu, Q, Shao, Y, Fu, W, Liu, G, and Coyte, PC. Socioeconomic inequities in health care utilization in China. Asia Pacific J Public Health. (2015) 27:429–38. doi: 10.1177/1010539514565446
37. Song, X, Zou, G, Chen, W, Han, S, Zou, X, and Ling, L. Health service utilisation of rural-to-urban migrants in Guangzhou, China: does employment status matter? Trop Med Int Health. (2017) 22:82–91. doi: 10.1111/tmi.12801
38. Li, D, Zhou, Z, Shen, C, Zhang, J, Yang, W, and Nawaz, R. Health disparity between the older rural-to-urban migrant workers and their rural counterparts in China. Int J Environ Res Public Health. (2020) 17:17. doi: 10.3390/ijerph17030955
39. Zhong, BL, Liu, TB, Huang, JX, Fung, HH, Chan, SS, Conwell, Y, et al. Acculturative stress of Chinese rural-to-urban migrant workers: a qualitative study. PLoS One. (2016) 11:e0157530. doi: 10.1371/journal.pone.0157530
40. Li, L, Yang, J, Zhai, S, and Li, D. Determinants of differences in health service utilization between older rural-to-urban migrant workers and older rural residents: evidence from a decomposition approach. Int J Environ Res Public Health. (2022) 19:6245. doi: 10.3390/ijerph19106245
41. Li, J, and Rose, N. Urban social exclusion and mental health of China's rural-urban migrants – a review and call for research. Health Place. (2017) 48:20–30. doi: 10.1016/j.healthplace.2017.08.009
42. Tang, S, Long, C, Wang, R, Liu, Q, Feng, D, and Feng, Z. Improving the utilization of essential public health services by Chinese elderly migrants: strategies and policy implication. J Glob Health. (2020) 10:010807. doi: 10.7189/jogh.10.010807
43. Eckman, MH, Wise, R, Leonard, AC, Dixon, E, Burrows, C, Khan, F, et al. Impact of health literacy on outcomes and effectiveness of an educational intervention in patients with chronic diseases. Patient Educ Couns. (2012) 87:143–51. doi: 10.1016/j.pec.2011.07.020
44. Tian, Y, Luo, T, and Chen, Y. The promotional effect of health education on the medical service utilization of migrants: evidence from China. Front Public Health. (2021) 9:818930. doi: 10.3389/fpubh.2021.818930
45. Steventon, A, and Bardsley, M. Use of secondary care in England by international immigrants. J Health Serv Res Policy. (2011) 16:90–4. doi: 10.1258/jhsrp.2010.010097
46. Kim, M, and Gu, H. Relationships between health education, health behaviors, and health status among migrants in China: a cross-sectional study based on the China migrant dynamic survey. Healthcare. (2023) 11:11. doi: 10.3390/healthcare11121768
Keywords: China, healthcare utilization, migrants, middle-aged, older adults
Citation: Xu L and Chen Y (2025) Effects of rural-to-urban migration on healthcare utilization of middle-aged and older adults: evidence from the China health and retirement longitudinal study. Front. Public Health. 13:1576285. doi: 10.3389/fpubh.2025.1576285
Edited by:
Roberta Zupo, University of Bari Aldo Moro, ItalyReviewed by:
Ailin Mao, Capital University of Economics and Business, ChinaBodi Shu, Queensland University of Technology, Australia
Copyright © 2025 Xu and Chen. This is an open-access article distributed under the terms of the Creative Commons Attribution License (CC BY). The use, distribution or reproduction in other forums is permitted, provided the original author(s) and the copyright owner(s) are credited and that the original publication in this journal is cited, in accordance with accepted academic practice. No use, distribution or reproduction is permitted which does not comply with these terms.
*Correspondence: Yingchun Chen, Y2hlbnlpbmdjaHVuaHVzdEAxNjMuY29t