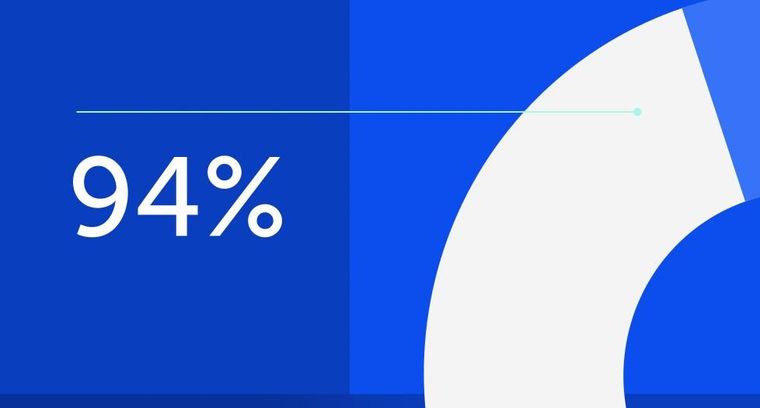
94% of researchers rate our articles as excellent or good
Learn more about the work of our research integrity team to safeguard the quality of each article we publish.
Find out more
ORIGINAL RESEARCH article
Front. Public Health
Sec. Public Health Policy
Volume 13 - 2025 | doi: 10.3389/fpubh.2025.1573783
This article is part of the Research TopicSystem Dynamics Modeling in Public Health: Implementations and ImplicationsView all articles
The final, formatted version of the article will be published soon.
Select one of your emails
You have multiple emails registered with Frontiers:
Notify me on publication
Please enter your email address:
If you already have an account, please login
You don't have a Frontiers account ? You can register here
This technical report addresses the predictive validity of long-term epidemiological forecasting based upon dynamic causal modelling. It uses complementary prospective and retrospective analyses. The prospective analysis completes a series of (annual) reports comparing predictions with subsequent outcomes (i.e., cases, deaths, hospital admissions and Long COVID) reported a year later. Predictive validity is then addressed retrospectively by examining predictions at various points during the pandemic, in relation to actual outcomes at three, six and 12 months after the predictions were evaluated. This analysis suggests that -with a sufficiently expressive dynamic causal model -three, six and 12 month projections can be remarkably accurate (to within 10% or less of observed outcomes) at certain phases of the epidemic: namely, the initial phase -before the emergence of highly transmissible variants -and towards the end of the pandemic, when slow fluctuations in transmissibility and virulence can be estimated more precisely. However, the predictive accuracy in the intervening periods are compromised, to the extent that some forecasts only remain within their Bayesian credible intervals for three months. We provide a quantitative analysis of predictive accuracy for future reference and discuss the implications for epidemiological modelling, and forecasting, of this sort.
Keywords: Coronavirus, compartmental models, epidemiology-descriptive, Incidence, Public health methodology, dynamic causal modelling
Received: 09 Feb 2025; Accepted: 15 Apr 2025.
Copyright: © 2025 Bowie and Friston. This is an open-access article distributed under the terms of the Creative Commons Attribution License (CC BY). The use, distribution or reproduction in other forums is permitted, provided the original author(s) or licensor are credited and that the original publication in this journal is cited, in accordance with accepted academic practice. No use, distribution or reproduction is permitted which does not comply with these terms.
* Correspondence: Cam Bowie, Retired, Devon, United Kingdom
Disclaimer: All claims expressed in this article are solely those of the authors and do not necessarily represent those of their affiliated organizations, or those of the publisher, the editors and the reviewers. Any product that may be evaluated in this article or claim that may be made by its manufacturer is not guaranteed or endorsed by the publisher.
Supplementary Material
Research integrity at Frontiers
Learn more about the work of our research integrity team to safeguard the quality of each article we publish.