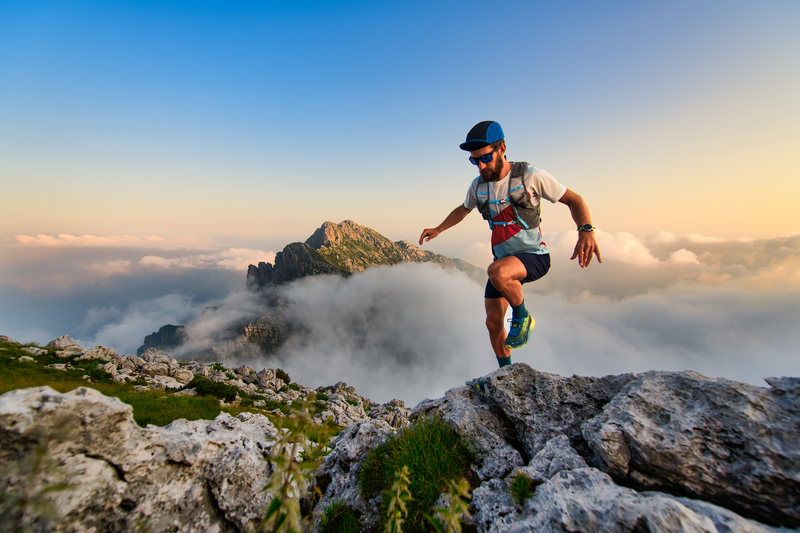
95% of researchers rate our articles as excellent or good
Learn more about the work of our research integrity team to safeguard the quality of each article we publish.
Find out more
ORIGINAL RESEARCH article
Front. Public Health
Sec. Public Mental Health
Volume 13 - 2025 | doi: 10.3389/fpubh.2025.1571522
The final, formatted version of the article will be published soon.
You have multiple emails registered with Frontiers:
Please enter your email address:
If you already have an account, please login
You don't have a Frontiers account ? You can register here
Introduction: Postpartum depression (PPD) has numerous adverse impacts on the families of new mothers and society at large. Early identification and intervention are of great significance. Although there are many existing machine learning classifiers for PPD prediction, the requirements for high accuracy and the interpretability of models present new challenges. Methods: This paper designs an ensemble neural network model for predicting PPD, which combines a Fully Connected Neural Network (FCNN) and a Neural Network with Dropout mechanism (DNN). The weights of FCNN and DNN in the proposed model are determined by their accuracies on the training set and respective Dropout values. The structure of the FCNN is simple and straightforward. The connection pattern among the neurons of the FCNN makes it easy to understand the relationship between the features and the target feature, endowing the proposed model with interpretability. Results: The proposed model achieved the following performance metrics on the PPD dataset: accuracy of 0.933, precision of 0.958, recall of 0.939, F1-score of 0.948, Matthews Correlation Coefficient (MCC) of 0.855, specificity of 0.923, Negative Predictive Value (NPV) of 0.889, False Positive Rate (FPR) of 0.077, and False Negative Rate (FNR) of 0.061. Compared with 10 classic machine learning classifiers, under different dataset split ratios, the proposed model outperforms in terms of indicators such as accuracy, precision, recall, and F1-score, and also has high stability.Discussion: The research results show that the proposed model effectively improves the prediction performance of PPD, which can provide guiding suggestions for relevant medical staff and postpartum women in clinical decision-making. In the future, plans include collecting more disease datasets, using the proposed model to predict these diseases, and constructing an online disease prediction platform to embed the proposed model, which will help with real-time disease prediction.
Keywords: postpartum depression, neural networks, machine learning, clinical decision-making, postpartum women
Received: 05 Feb 2025; Accepted: 31 Mar 2025.
Copyright: © 2025 Lin and Zhou. This is an open-access article distributed under the terms of the Creative Commons Attribution License (CC BY). The use, distribution or reproduction in other forums is permitted, provided the original author(s) or licensor are credited and that the original publication in this journal is cited, in accordance with accepted academic practice. No use, distribution or reproduction is permitted which does not comply with these terms.
* Correspondence:
DongQin Zhou, Wenzhou People’s Hospital, Wenzhou, China
Disclaimer: All claims expressed in this article are solely those of the authors and do not necessarily represent those of their affiliated organizations, or those of the publisher, the editors and the reviewers. Any product that may be evaluated in this article or claim that may be made by its manufacturer is not guaranteed or endorsed by the publisher.
Research integrity at Frontiers
Learn more about the work of our research integrity team to safeguard the quality of each article we publish.