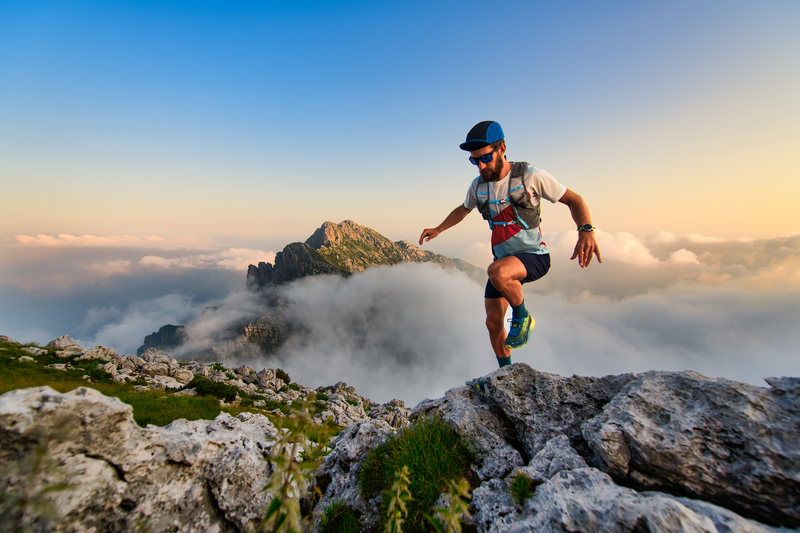
94% of researchers rate our articles as excellent or good
Learn more about the work of our research integrity team to safeguard the quality of each article we publish.
Find out more
ORIGINAL RESEARCH article
Front. Public Health
Sec. Public Health Policy
Volume 13 - 2025 | doi: 10.3389/fpubh.2025.1562191
This article is part of the Research Topic Impact Evaluation using the Translational Science Benefits Model Framework in the National Center for Advancing Translational Science Clinical and Translational Science Award Program View all articles
The final, formatted version of the article will be published soon.
You have multiple emails registered with Frontiers:
Please enter your email address:
If you already have an account, please login
You don't have a Frontiers account ? You can register here
Evaluating large-scale programs designed to transform public health demands innovative approaches for navigating their complexity and scope. The Clinical and Translational Science Awards (CTSA) Program, supported by the NIH's National Center for Advancing Translational Sciences (NCATS), represents a significant national investment with over 60 sites or "hubs" spread across the country. Assessing an initiative of this size and complexity requires measures that balance local flexibility with national coherence. To that end, this study used concept mapping, a mixed-methods approach integrating qualitative brainstorming and sorting with quantitative multidimensional scaling and cluster analysis. Participation across the CTSA was unprecedented. Over 100 evaluation stakeholders were engaged across the network of hubs, leading to the identification of more than 80 measures, which were then organized into thematic clusters that reflect a logical progression from CTSA activities to outcomes and impacts, as well as critical foundational factors such as collaboration and education. The results also revealed a pattern where long-term impacts were ranked among the highest in importance but among the lowest in feasibility, particularly for measures tied to the Translational Science Benefits Model (TSBM), a new evaluation framework gaining popularity across the CTSA. The findings of this study underscore the efficacy of concept mapping in incorporating wide-ranging perspectives, identifying areas of consensus, and informing leadership in the development of unified, data-driven evaluation frameworks -such as TSBM and/or a CTSA logic model-critical to maximizing the CTSA's transformative potential for public health.
Keywords: Clinical and Translational Science Awards (CTSA), concept mapping, evaluation study, stakeholder participation, Mixed-methods Research, translational science benefits model (TSBM), Longitudinal impact, National institutes of health (NIH)
Received: 17 Jan 2025; Accepted: 10 Mar 2025.
Copyright: © 2025 Kane, Trochim, Barr, Vaught, Baker, Khan, Wagner, Herzog, Holmes and Doyle. This is an open-access article distributed under the terms of the Creative Commons Attribution License (CC BY). The use, distribution or reproduction in other forums is permitted, provided the original author(s) or licensor are credited and that the original publication in this journal is cited, in accordance with accepted academic practice. No use, distribution or reproduction is permitted which does not comply with these terms.
* Correspondence:
Cathleen T. Kane, Institute of Clinical & Translational Science,School of Medicine, New York University, New York, 10016, United States
Disclaimer: All claims expressed in this article are solely those of the authors and do not necessarily represent those of their affiliated organizations, or those of the publisher, the editors and the reviewers. Any product that may be evaluated in this article or claim that may be made by its manufacturer is not guaranteed or endorsed by the publisher.
Research integrity at Frontiers
Learn more about the work of our research integrity team to safeguard the quality of each article we publish.