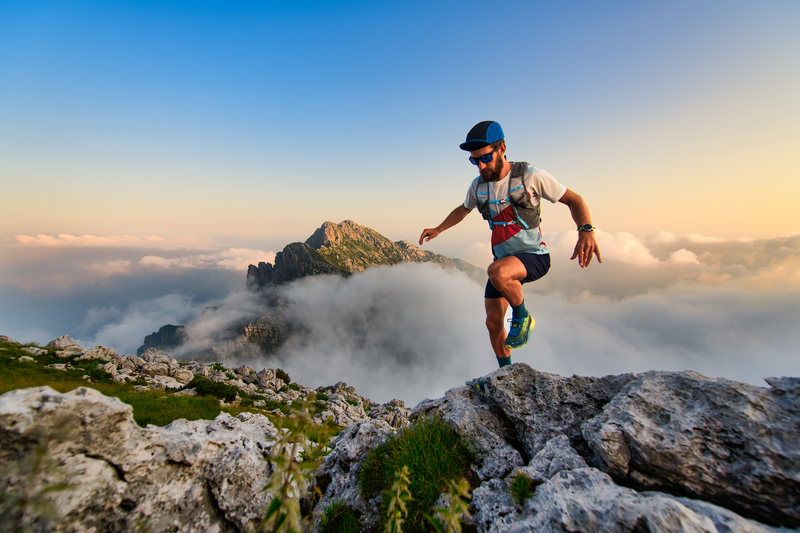
94% of researchers rate our articles as excellent or good
Learn more about the work of our research integrity team to safeguard the quality of each article we publish.
Find out more
ORIGINAL RESEARCH article
Front. Public Health
Sec. Public Health Policy
Volume 13 - 2025 | doi: 10.3389/fpubh.2025.1556737
The final, formatted version of the article will be published soon.
You have multiple emails registered with Frontiers:
Please enter your email address:
If you already have an account, please login
You don't have a Frontiers account ? You can register here
Hospital service efficiency is a vital indicator of the effectiveness of a country's healthcare system. This study utilized the Meta-Frontier Slack-Based Measure (SBM) model to evaluate the efficiency of hospital services across various Chinese provinces from 2009 to 2022. Efficiency comparisons were made using regional and common frontiers, while the technical gap and its decomposition index were applied to identify the sources of efficiency disparities among regions.The Spatial Durbin Model was then employed to analyze the spatial spillover effects of hospital service efficiency. The findings reveal that under the common frontier, the Central South regions demonstrate the highest efficiency, averaging 0.7549, followed by East China at 0.7184, the Southwest at 0.6245, the Northwest at 0.5497, North China at 0.4884, and the Northeast at 0.3571.Technical disparities among China's regional hospital services form three distinct tiers: the first tier includes Central South China, the second tier comprises the Southwest, Northwest, and North China, and the third tier is the Northeast. Management inefficiency predominantly affects the first tier, whereas both management and technical inefficiencies impact the second and third tiers. Furthermore, hospital service efficiency has significant spatial spillover effects, notably enhancing the efficiency of neighboring provinces.
Keywords: Hospital service efficiency, technological heterogeneity, Meta-frontier SBM, spatial Durbin model, regional differences
Received: 07 Jan 2025; Accepted: 25 Feb 2025.
Copyright: © 2025 Song, Ye, Zhou, Chen and jiang. This is an open-access article distributed under the terms of the Creative Commons Attribution License (CC BY). The use, distribution or reproduction in other forums is permitted, provided the original author(s) or licensor are credited and that the original publication in this journal is cited, in accordance with accepted academic practice. No use, distribution or reproduction is permitted which does not comply with these terms.
* Correspondence:
Qianwen Song, Shuangliu Maternal and Child Health Care Hospital, Chengdu, China
Disclaimer: All claims expressed in this article are solely those of the authors and do not necessarily represent those of their affiliated organizations, or those of the publisher, the editors and the reviewers. Any product that may be evaluated in this article or claim that may be made by its manufacturer is not guaranteed or endorsed by the publisher.
Research integrity at Frontiers
Learn more about the work of our research integrity team to safeguard the quality of each article we publish.