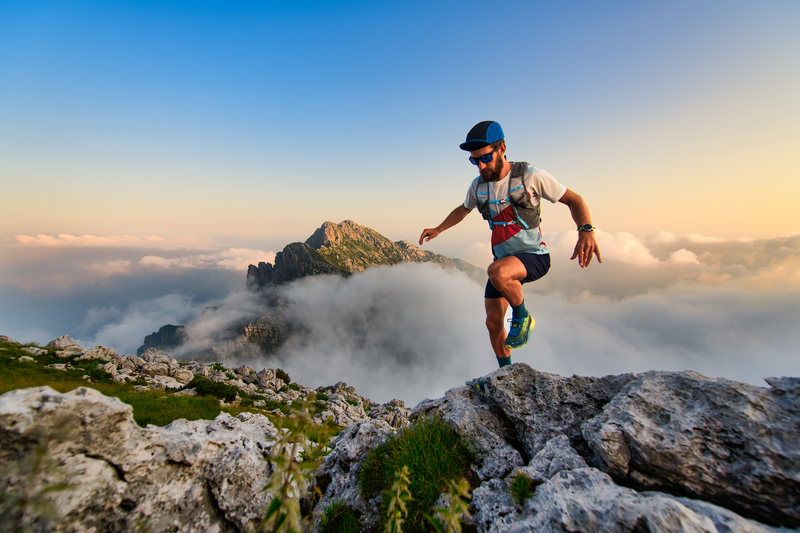
94% of researchers rate our articles as excellent or good
Learn more about the work of our research integrity team to safeguard the quality of each article we publish.
Find out more
ORIGINAL RESEARCH article
Front. Public Health
Sec. Disaster and Emergency Medicine
Volume 13 - 2025 | doi: 10.3389/fpubh.2025.1555735
The final, formatted version of the article will be published soon.
You have multiple emails registered with Frontiers:
Please enter your email address:
If you already have an account, please login
You don't have a Frontiers account ? You can register here
Mass gathering activities are often accompanied by safety risks especially overcrowding, since the characteristics of risk factors may differ under various organizational patterns. However, this issue has not been sufficiently studied, which could lead to cognitive biases in understanding the risks associated with various mass gathering activities, thereby affecting the effectiveness of preventive measures. This study investigates the risk factors of human stampede in mass gathering activities, with a particular focus on analyzing the influence of different organizational patterns on these risks and their implications for safety management. By combining Grounded Theory and Iterative Self-Organizing Clustering Algorithm (ISODATA), 209 overcrowding cases were coded to create a dataset containing risk factors. The organization coefficient was proposed to characterize the degree of risk in different clusters, and the clustering results revealed three types of organizational patterns including organized(O_31=1.9174), applied(O_82=2.9831), and spontaneous(O_73=4.4327). The results indicate significant differences between the three organizational patterns in terms of aggregation causes, layout characteristics, and risk levels, which directly impacts the safety of mass gathering activities. Furthermore, similarities are observed in terms of triggering behaviors, knowledge, awareness, and management across the four categories. This study provides theoretical evidence for risk prevention and safety management of human stampede in mass gathering.
Keywords: Mass gathering1, Organizational pattern2, Spontaneous gathering3, Risk Factors4, Unsupervised Clustering Algorithms5
Received: 05 Jan 2025; Accepted: 24 Mar 2025.
Copyright: © 2025 Lu, Wang, Dai, Zou, Lu and Hong. This is an open-access article distributed under the terms of the Creative Commons Attribution License (CC BY). The use, distribution or reproduction in other forums is permitted, provided the original author(s) or licensor are credited and that the original publication in this journal is cited, in accordance with accepted academic practice. No use, distribution or reproduction is permitted which does not comply with these terms.
* Correspondence:
Ying Lu, College of Resources and Environment Engineering, Wuhan University of Science and Technology, Wuhan, 430081, Hubei Province, China
Weihui Dai, School of Management, Fudan University, Shanghai, Shanghai Municipality, China
Disclaimer: All claims expressed in this article are solely those of the authors and do not necessarily represent those of their affiliated organizations, or those of the publisher, the editors and the reviewers. Any product that may be evaluated in this article or claim that may be made by its manufacturer is not guaranteed or endorsed by the publisher.
Research integrity at Frontiers
Learn more about the work of our research integrity team to safeguard the quality of each article we publish.