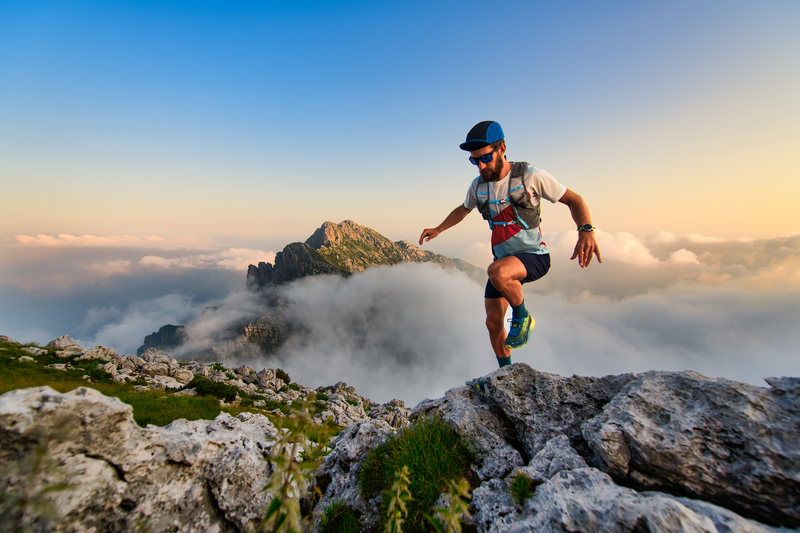
94% of researchers rate our articles as excellent or good
Learn more about the work of our research integrity team to safeguard the quality of each article we publish.
Find out more
ORIGINAL RESEARCH article
Front. Public Health
Sec. Life-Course Epidemiology and Social Inequalities in Health
Volume 13 - 2025 | doi: 10.3389/fpubh.2025.1547946
The final, formatted version of the article will be published soon.
You have multiple emails registered with Frontiers:
Please enter your email address:
If you already have an account, please login
You don't have a Frontiers account ? You can register here
Objective: To create county-, 5-digit ZIP code (ZIP-5)-, and 3-digit ZIP code (ZIP-3)-level datasets of the Social Vulnerability Index (SVI) and its components for 2016-2022 to validate the methodology beyond county level, explore trends in SVI over time and space, and demonstrate its usage in an enrichment exercise with health plan claims. Materials and Methods: The SVI consolidates 16 structural, economic, and demographic variables from the American Community Survey (ACS) into 4 themes: socioeconomic status, household characteristics, racial and ethnic minority status, and housing type and transportation. ACS estimates of the 16 variables for 2016-2022 were extracted for counties and ZIP code tabulation areas, and for ZIP code geographies, crosswalked to ZIP-5, and aggregated to ZIP-3. Areas received a percentile ranking (range, 0 to 1) for SVI and each variable and composite theme, with higher values indicating greater social vulnerability. Results: SVI estimates were produced for up to 3143 counties, 32,243 ZIP-5s, and 886 ZIP-3s. SDoH trends across the US were largely consistent from 2016-2022 despite slight local changes over time. SVI varied across regions, with generally higher vulnerability in the South and lower vulnerability in the North and Northeast. When linked with health plan claims data, higher SVI (i.e., higher vulnerability) was associated with greater comorbidity burden. Conclusion: SVI can be estimated at the ZIP-3 and ZIP-5 levels to provide area-level context, allowing for more routine integration of socioeconomic and health equity-related concepts into health claims and other datasets.
Keywords: Social vulnerability index (SVI), social determinants of health, real-world data (RWD), health equity, Geography
Received: 19 Dec 2024; Accepted: 13 Feb 2025.
Copyright: © 2025 Ng, Zhang and Kowal. This is an open-access article distributed under the terms of the Creative Commons Attribution License (CC BY). The use, distribution or reproduction in other forums is permitted, provided the original author(s) or licensor are credited and that the original publication in this journal is cited, in accordance with accepted academic practice. No use, distribution or reproduction is permitted which does not comply with these terms.
* Correspondence:
Stacey Kowal, Genentech Inc., San Francisco, United States
Disclaimer: All claims expressed in this article are solely those of the authors and do not necessarily represent those of their affiliated organizations, or those of the publisher, the editors and the reviewers. Any product that may be evaluated in this article or claim that may be made by its manufacturer is not guaranteed or endorsed by the publisher.
Research integrity at Frontiers
Learn more about the work of our research integrity team to safeguard the quality of each article we publish.