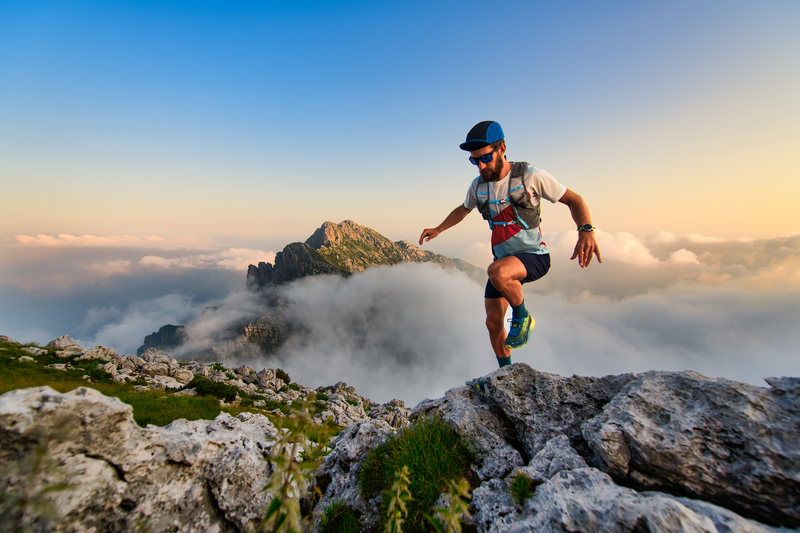
95% of researchers rate our articles as excellent or good
Learn more about the work of our research integrity team to safeguard the quality of each article we publish.
Find out more
ORIGINAL RESEARCH article
Front. Public Health
Sec. Digital Public Health
Volume 13 - 2025 | doi: 10.3389/fpubh.2025.1544904
This article is part of the Research Topic Unlocking the Potential of Health Data: Interoperability, Security, and Emerging Challenges in AI, LLM, Precision Medicine, and Their Impact on Healthcare and Research View all articles
The final, formatted version of the article will be published soon.
You have multiple emails registered with Frontiers:
Please enter your email address:
If you already have an account, please login
You don't have a Frontiers account ? You can register here
Background: Disease presentation and progression can vary greatly in heterogeneous diseases, such as COVID-19, with variability in patient outcomes, even within the hospital setting. This variability underscores the need for tailored treatment approaches based on distinct clinical subgroups.Objectives: This study aimed to identify COVID-19 patient subgroups with unique clinical characteristics using real-world data (RWD) from electronic health records (EHRs) to inform individualized treatment plans.Materials and Methods: A Factor Analysis of Mixed Data (FAMD)-based agglomerative hierarchical clustering approach was employed to analyze the real-world data, enabling the identification of distinct patient subgroups. Statistical tests evaluated cluster differences, and machine learning models classified the identified subgroups.Results: Three clusters of COVID-19 inpatients with unique clinical characteristics were identified. The analysis revealed significant differences in hospital stay durations and survival rates among the clusters, with more severe clinical features correlating with worse prognoses and machine learning classifiers achieving high accuracy in subgroup identification.Conclusions: By leveraging RWD and advanced clustering techniques, the study provides insights into the heterogeneity of COVID-19 presentations. The findings support the development of classification models that can inform more individualized and effective treatment plans, improving patient outcomes in the future.
Keywords: Real-world data, Clustering analysis, Factor analysis of mixed data, Classification, Critical Care
Received: 13 Dec 2024; Accepted: 31 Mar 2025.
Copyright: © 2025 Anderson, Gould, Patil, Mohr, Dodd, Boyce, Dasher, Guerin, Khan, Cheruku, Kumar, Mathe, Mehta, Michelson, Williams, Heavner and Podichetty. This is an open-access article distributed under the terms of the Creative Commons Attribution License (CC BY). The use, distribution or reproduction in other forums is permitted, provided the original author(s) or licensor are credited and that the original publication in this journal is cited, in accordance with accepted academic practice. No use, distribution or reproduction is permitted which does not comply with these terms.
* Correspondence:
Wesley Anderson, Critical Path Institute, Tucson, United States
Disclaimer: All claims expressed in this article are solely those of the authors and do not necessarily represent those of their affiliated organizations, or those of the publisher, the editors and the reviewers. Any product that may be evaluated in this article or claim that may be made by its manufacturer is not guaranteed or endorsed by the publisher.
Research integrity at Frontiers
Learn more about the work of our research integrity team to safeguard the quality of each article we publish.