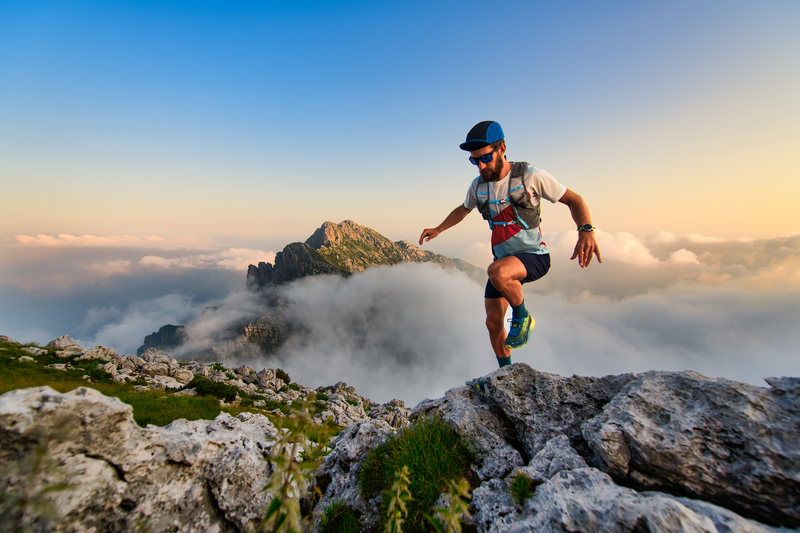
94% of researchers rate our articles as excellent or good
Learn more about the work of our research integrity team to safeguard the quality of each article we publish.
Find out more
ORIGINAL RESEARCH article
Front. Public Health
Sec. Digital Public Health
Volume 13 - 2025 | doi: 10.3389/fpubh.2025.1544894
This article is part of the Research Topic Diagnosing and Treating Frailty and Sarcopenia in Middle-aged and Older Adults View all 6 articles
The final, formatted version of the article will be published soon.
You have multiple emails registered with Frontiers:
Please enter your email address:
If you already have an account, please login
You don't have a Frontiers account ? You can register here
The elderly are at high risk of sarcopenia, making early identification and scientific intervention crucial for healthy aging. This study utilized data from the China Health and Retirement Longitudinal Study (CHARLS), including a cohort of 2,717 middle-aged and elderly participants. Ten machine learning algorithms, such as CatBoost, XGBoost, and NGBoost, were used to construct predictive models. Among them, the XGBoost model performed the best with an ROC-AUC of 0.7, and it was selected as the final predictive model for sarcopenia risk. To enhance model interpretability, SHAP technology was used to visualize the prediction results, and the system was implemented on a web platform. The system provides the probability of sarcopenia onset within four years based on input variables and identifies critical influencing factors, facilitating understanding and use by medical professionals. This system supports early identification and scientific intervention for sarcopenia in the elderly, with significant clinical value and application potential.
Keywords: Sarcopenia, risk prediction, Visualized, machine learning, CHARLS
Received: 13 Dec 2024; Accepted: 24 Feb 2025.
Copyright: © 2025 Du, Tao, Zhu, Wang, Qi, Min, Wei, Zhang and Liu. This is an open-access article distributed under the terms of the Creative Commons Attribution License (CC BY). The use, distribution or reproduction in other forums is permitted, provided the original author(s) or licensor are credited and that the original publication in this journal is cited, in accordance with accepted academic practice. No use, distribution or reproduction is permitted which does not comply with these terms.
* Correspondence:
Qiang Liu, The First Affiliated Hospital of Nanchang University, Nanchang, 330006, Jiangxi Province, China
Disclaimer: All claims expressed in this article are solely those of the authors and do not necessarily represent those of their affiliated organizations, or those of the publisher, the editors and the reviewers. Any product that may be evaluated in this article or claim that may be made by its manufacturer is not guaranteed or endorsed by the publisher.
Research integrity at Frontiers
Learn more about the work of our research integrity team to safeguard the quality of each article we publish.