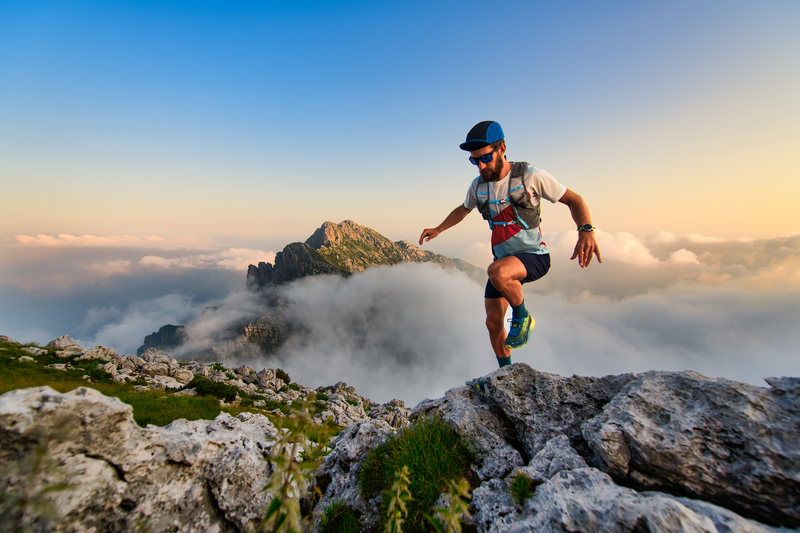
95% of researchers rate our articles as excellent or good
Learn more about the work of our research integrity team to safeguard the quality of each article we publish.
Find out more
METHODS article
Front. Public Health
Sec. Public Health Policy
Volume 13 - 2025 | doi: 10.3389/fpubh.2025.1536147
The final, formatted version of the article will be published soon.
You have multiple emails registered with Frontiers:
Please enter your email address:
If you already have an account, please login
You don't have a Frontiers account ? You can register here
Reducing low-value care by deprescribing is a priority. However, current policies often fail due to healthcare provider resistance, lack of incentives, and poor patient engagement. We believe this is due to insufficient focus on the relationships between key agents in the deprescribing process, making 'one-size-fits-all' interventions ineffective.Our goal is to understand 'how, why, for whom, and in what contexts' policies affect large-scale deprescribing.To achieve our research aim, we propose an inductive an abductive and retroductive phases.The inductive phase involved an exploratory review of the literature, leading to the development of an initial theory presented in this article. The initial theory includes three interconnected hypotheses:(a) the success of deprescription depends on the behaviors and interactions of specific agents within the deprescription process. (b) These interactions are influenced by power and trust dynamics, creating either a vicious or virtuous causal loop. (c) The impact of policy on the deprescription chain depends on its ability to address the diversity of the deprescription process and the agents within a given territory, considering dimensionality, ruggedness, and context-specificity.The abductive phase will test the initial theory's applicability, while the retroductive phase will propose new mechanisms to update it. This forms the core of our realist literature review. After screening, selected articles will assess how well the initial theory explains policy impacts on the deprescription process in specific context. Our article analysis will combine semantic and latent content analysis for the abductive phase, and retroductive analysis. Finally, we will compare the updated theory with existing frameworks.The ultimate purpose of this study is to guide policymakers by providing a framework for informed decision-making before implementing large-scale deprescription policies. Its originality lies in emphasizing the relational nature of the deprescription process and examining policy implementation through the lens of complexity.
Keywords: Deprescription, policies, realist review, protocol, initial theory
Received: 28 Nov 2024; Accepted: 28 Mar 2025.
Copyright: © 2025 Macq, Lopez Toribio and Spinewine. This is an open-access article distributed under the terms of the Creative Commons Attribution License (CC BY). The use, distribution or reproduction in other forums is permitted, provided the original author(s) or licensor are credited and that the original publication in this journal is cited, in accordance with accepted academic practice. No use, distribution or reproduction is permitted which does not comply with these terms.
* Correspondence:
Jean Macq, Université Catholique de Louvain, Louvain-la-Neuve, Belgium
Disclaimer: All claims expressed in this article are solely those of the authors and do not necessarily represent those of their affiliated organizations, or those of the publisher, the editors and the reviewers. Any product that may be evaluated in this article or claim that may be made by its manufacturer is not guaranteed or endorsed by the publisher.
Research integrity at Frontiers
Learn more about the work of our research integrity team to safeguard the quality of each article we publish.