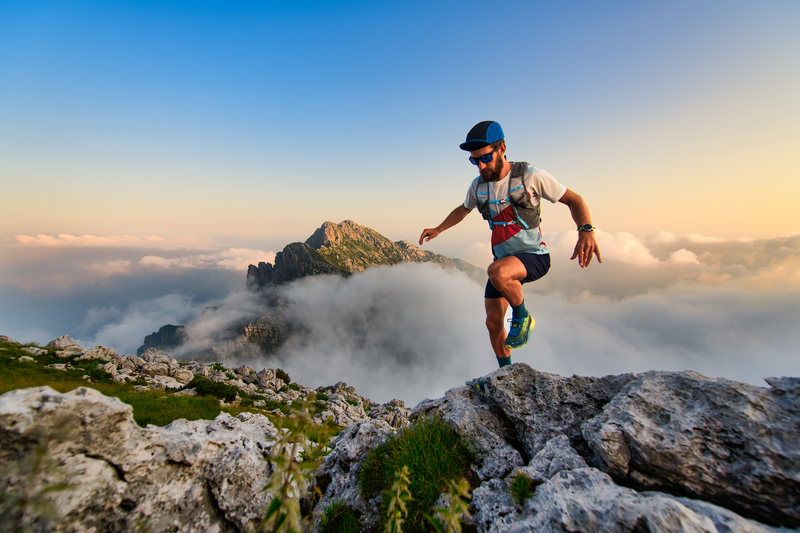
94% of researchers rate our articles as excellent or good
Learn more about the work of our research integrity team to safeguard the quality of each article we publish.
Find out more
ORIGINAL RESEARCH article
Front. Public Health
Sec. Occupational Health and Safety
Volume 13 - 2025 | doi: 10.3389/fpubh.2025.1531541
The final, formatted version of the article will be published soon.
You have multiple emails registered with Frontiers:
Please enter your email address:
If you already have an account, please login
You don't have a Frontiers account ? You can register here
Background: With the rapid development of global digital economy, burnout among food delivery riders has become an important public health issue. Although burnout has been widely studied, research on burnout among food delivery riders, particularly the impact of algorithmic management on riders' burnout remains limited.This study adopts a novel perspective on the intersection of algorithmic management and burnout, offering an in-depth examination of the burnout levels of food delivery riders under the strict control of algorithmic management and identifying its influencing factors.Methods: A survey of 953 food delivery riders was conducted using the Maslach Burnout Inventory-General Survey (MBI-GS). SPSS was used to conduct independent sample t-tests, one-way ANOVA, Pearson correlation, and multiple linear regression to investigate burnout status and identify factors affecting riders' burnout.The findings indicate that food delivery riders are experiencing moderate level of burnout, with Emotional Exhaustion, Depersonalization, and Reduced Personal Accomplishment as the primary dimensions.In the context of algorithmic management, key factors affecting riders' burnout include gender, age, working years, ranking system, Punishment system, work rules, Work monitoring mechanism, workflow design, customer feedback, and restaurant preparation time.Under algorithmic management, burnout is prevalent among China's food delivery riders and influenced by multiple factors. Individualized support, humane organizational systems, satisfied work mechanism, and supportive social environment can help lessen algorithmic management's negative effects on food delivery riders and reduce their burnout.This study provides theoretical recommendations to protect occupational health of gig workers in platform economy, and offers valuable guidance for practical application.
Keywords: algorithmic management, food delivery platform, food delivery riders, burnout, influencing factor
Received: 20 Nov 2024; Accepted: 28 Mar 2025.
Copyright: © 2025 Dong, Zhang and Wu. This is an open-access article distributed under the terms of the Creative Commons Attribution License (CC BY). The use, distribution or reproduction in other forums is permitted, provided the original author(s) or licensor are credited and that the original publication in this journal is cited, in accordance with accepted academic practice. No use, distribution or reproduction is permitted which does not comply with these terms.
* Correspondence:
Lizhi Wu, Shandong University of Science and Technology, Qingdao, China
Disclaimer: All claims expressed in this article are solely those of the authors and do not necessarily represent those of their affiliated organizations, or those of the publisher, the editors and the reviewers. Any product that may be evaluated in this article or claim that may be made by its manufacturer is not guaranteed or endorsed by the publisher.
Research integrity at Frontiers
Learn more about the work of our research integrity team to safeguard the quality of each article we publish.