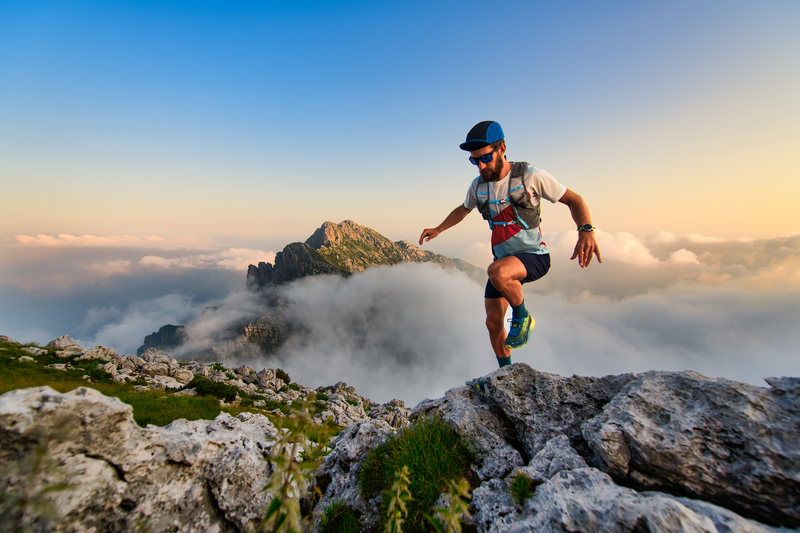
94% of researchers rate our articles as excellent or good
Learn more about the work of our research integrity team to safeguard the quality of each article we publish.
Find out more
ORIGINAL RESEARCH article
Front. Public Health
Sec. Public Mental Health
Volume 13 - 2025 | doi: 10.3389/fpubh.2025.1523691
This article is part of the Research Topic The Intersection of Psychology, Healthy Behaviors, and its Outcomes View all 66 articles
The final, formatted version of the article will be published soon.
You have multiple emails registered with Frontiers:
Please enter your email address:
If you already have an account, please login
You don't have a Frontiers account ? You can register here
Background: Covid-19 measures in Germany varied during the pandemic, and it seems natural that in addition to factors such as incidence, health system capacity, etc., these interventions and their social and economic consequences had an impact on the evolution of the population's well-being. Since the beginning of the pandemic, there has been a suspicion that the health burden would fall mainly on population groups with a lower socio-economic status, and that Covid-19, including the policy measures, could therefore contribute to increasing social inequalities in health. We examine several indicators of well-being over the course of the pandemic, analyse the effect of the stringency of the measures on subjective well-being and the extent to which certain social groups were particularly affected.Methods: Our analyses are based on 2020 and 2021 data from the German Socio-Economic Panel (SOEP), complemented by various regional indicators, including the Covid-19 measures. Data on subjective well-being during the pandemic phases were regressed on the phases, socio-demographic, economic and health-related indicators, stringency of measures and other regional indicators in multilevel models with the district as the top level. Up to N = 29,871 observations in 401 districts were included.Results: Overall, there was little decline in well-being up to the end of the observation period, and even some increase. When the effect of the stringency of the measures was taken into account, the changes were partially attenuated. However, stringency had little direct effect on well-being. People with disabilities and chronic pre-existing conditions were particularly affected by a reduction in wellbeing. In some cases, Covid-19 measures had slightly different effects in these groups.The effects of socio-economic indicators were not strong enough to suggest that lower social status is generally associated with a negative trend in well-being. According to our results, people with disabilities and chronic diseases, including severe obesity, should be given more attention in the future. A change in time-related outcomes when considering Covid-19 measures could indicate adjustment effects on well-being.
Keywords: COVID-19, Subjective well-being, life satisfaction, non-pharmacological interventions, Preventive measures, Regional deprivation
Received: 06 Nov 2024; Accepted: 10 Feb 2025.
Copyright: © 2025 Finne, Nowak and Razum. This is an open-access article distributed under the terms of the Creative Commons Attribution License (CC BY). The use, distribution or reproduction in other forums is permitted, provided the original author(s) or licensor are credited and that the original publication in this journal is cited, in accordance with accepted academic practice. No use, distribution or reproduction is permitted which does not comply with these terms.
* Correspondence:
Anna Christina Nowak, Bielefeld University, Bielefeld, Germany
Disclaimer: All claims expressed in this article are solely those of the authors and do not necessarily represent those of their affiliated organizations, or those of the publisher, the editors and the reviewers. Any product that may be evaluated in this article or claim that may be made by its manufacturer is not guaranteed or endorsed by the publisher.
Research integrity at Frontiers
Learn more about the work of our research integrity team to safeguard the quality of each article we publish.