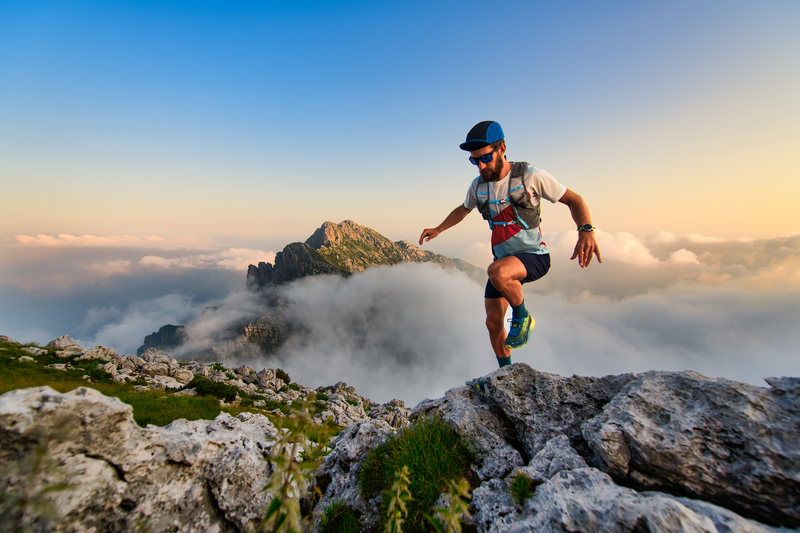
94% of researchers rate our articles as excellent or good
Learn more about the work of our research integrity team to safeguard the quality of each article we publish.
Find out more
ORIGINAL RESEARCH article
Front. Public Health
Sec. Public Mental Health
Volume 13 - 2025 | doi: 10.3389/fpubh.2025.1522877
This article is part of the Research Topic Youth Mental Health, Particularly in Asian Populations View all 56 articles
The final, formatted version of the article will be published soon.
You have multiple emails registered with Frontiers:
Please enter your email address:
If you already have an account, please login
You don't have a Frontiers account ? You can register here
The network theory of mental disorders offers a new perspective for the understanding of comorbidities, but the research on the comorbidities among depression, anxiety, and post-traumatic stress disorder (PTSD) is still insufficient. The aim of this study was to explore the internal relationship by establishing and analyzing the comorbidity networks, and to provide suggestions for the intervention after traumatic events.We utilized data from the second and third wave of the Chengdu Positive Child Development cohort (N = 3,189, 47.79% female), we estimated to network models of depression, anxiety and PTSD.To assess difference in global connectivity between the two networks, we conducted invariance test.Results: K27 (Somatic 10), K37 (Generalized Anxiety 9), K15 (Somatic 5), K33 (Generalized Anxiety 7), K24 (Somatic 9) were the most central nodes in both networks, P13 (Sleep problem) had the highest Bridge Expected Influence value. The structural difference between the two networks was statistically significant (M = 0.229, P = 0.010), and the global strength of the network at wave 2 was higher than the network at wave 3 (35.1 vs. 33.9, S = 1.20, P = 0.010).The correlation in symptoms of the three disorders underscores the need for more comprehensive treatment options for intervention after traumatic events. Central and bridge nodes could inform targeted interventions or policy decisions. Anxiety disorders, especially Som and Gen dimensions, should be the focus of intervention. The Arousal dimension in PTSD, especially sleep disorders, may contribute to the comorbidities. In addition, this study highlights the importance of staged post-traumatic interventions.
Keywords: longitudinal study, Network analysis, Post-traumatic stress disorder, Depression, Anxiety, Adolescent Health, COVID-19, comorbidities
Received: 03 Dec 2024; Accepted: 26 Feb 2025.
Copyright: © 2025 Li, Liu, Wang, Li, Mei, Zhang, Fan and Jiang. This is an open-access article distributed under the terms of the Creative Commons Attribution License (CC BY). The use, distribution or reproduction in other forums is permitted, provided the original author(s) or licensor are credited and that the original publication in this journal is cited, in accordance with accepted academic practice. No use, distribution or reproduction is permitted which does not comply with these terms.
* Correspondence:
Lihua Jiang, Sichuan University, Chengdu, China
Disclaimer: All claims expressed in this article are solely those of the authors and do not necessarily represent those of their affiliated organizations, or those of the publisher, the editors and the reviewers. Any product that may be evaluated in this article or claim that may be made by its manufacturer is not guaranteed or endorsed by the publisher.
Research integrity at Frontiers
Learn more about the work of our research integrity team to safeguard the quality of each article we publish.